BMS2043 Statistics & Data Analysis Lecture 3 2024 PDF
Document Details
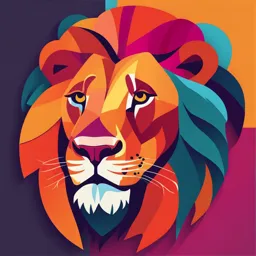
Uploaded by CongratulatoryIntelligence5915
University of Surrey
2024
University of Surrey
Youngchan Kim
Tags
Related
- BMS2043 Statistics & Data Analysis Lecture 1 2024 PDF
- Statistics & Data Analysis Lecture 2 2024 PDF
- BMS2043 Statistics & Data Analysis Lecture 4 2024 PDF
- Statistical Analysis of Twin Data PDF
- Biochemistry of Nutrition Topic 12 Nutrition Methodology PDF
- Principles and Techniques of Biochemistry and Molecular Biology PDF
Summary
This document is a lecture from the university of surrey on statistics and data analysis. It covers the concepts of one-tailed hypothesis testing, and statistical models including linear regression.
Full Transcript
Statistics & Data Analysis Analytical and Clinical Biochemistry (BMS2043) Spring 2024 Lecture 3 Youngchan Kim, PhD Lecturer in Quantum Biology University of Surrey youngchan.kim@surrey.ac.uk / 01AZ04 Inferential statistics, part 1, recap Steps in performing a statistical test 1. Formulate null and a...
Statistics & Data Analysis Analytical and Clinical Biochemistry (BMS2043) Spring 2024 Lecture 3 Youngchan Kim, PhD Lecturer in Quantum Biology University of Surrey youngchan.kim@surrey.ac.uk / 01AZ04 Inferential statistics, part 1, recap Steps in performing a statistical test 1. Formulate null and alternative hypotheses (H0 vs. H1) 2. Evaluate the data and choose an appropriate statistical test for the data 3. Perform the statistical test 4. Obtain test statistic and P-value 5. Evaluate the statistical significance of the result 6. Reject or accept null hypothesis BMS2043 – Statistics and Data Analysis, 2024 When is it appropriate to use a one-tail P value? A one-tailed test is appropriate when previous data, physical limitations, or common sense tells you that the difference, if any, can only go in one direction. You should only choose a one-tail P value when both of the following are true. ü You predicted which group will have the larger mean (or proportion) before you collected any data. If you only made the "prediction" after seeing the data, don't even think about using a one-tail P value. ü If the other group had ended up with the larger mean – even if it is quite a bit larger – you would have attributed that difference to chance and called the difference 'not statistically significant'. https://www.graphpad.com/guides/prism/latest/statistics/one-tail_vs__two-tail_p_values.htm BMS2043 – Statistics and Data Analysis, 2024 Example 1: FTO and BMI Based on previous research a scientist has an a priori hypothesis that body mass index (BMI) in Europeans is increased due to variations in FTO gene. The scientist has data on UK population to study the effects of FTO on BMI. Which is the null hypothesis here? After testing the effect of variation in the FTO gene on BMI the scientist gets a test statistic with an associated Pvalue=0.004. What is your conclusion? BMI in Europeans is either decreased or not changed due to variations in FTO gene. Which is the alternative hypothesis? BMI in Europeans is increased due to variations in FTO gene. Is the test one or two-tailed? Now we have a “increase” in the alternative hypothesis. This means that instead of performing a twotailed test, we will perform a left-sided one-tailed test. BMS2043 – Statistics and Data Analysis, 2024 Another example for a one-tailed t-test You are testing whether a new antibiotic impairs renal function, as measured by serum creatinine. Many antibiotics poison kidney cells, resulting in reduced glomerular filtration and increased serum creatinine. As far as I know, no antibiotic is known to decrease serum creatinine, and it is hard to imagine a mechanism by which an antibiotic would increase the glomerular filtration rate. Before collecting any data, you can state that there are two possibilities: Either the drug will not change the mean serum creatinine of the population, or it will increase the mean serum creatinine in the population. You consider it impossible that the drug will truly decrease mean serum creatinine of the population and plan to attribute any observed decrease to random sampling. Accordingly, it makes sense to calculate a one-tailed P value. BMS2043 – Statistics and Data Analysis, 2024 Statistical methods to be covered in these lectures Correlation and associated P-value Test of frequencies: χ2 – test and Fisher’s exact test Quantitative normally distributed data: Student’s t-test Quantitative non-normal data: Mann-Whitney U-test More than 2 groups: ANOVA – Analysis of variance More than 2 groups, non-normal data: Kruskal-Wallis test Linear regression (simple and multiple) Logistic regression BMS2043 – Statistics and Data Analysis, 2024 Inferential statistics, part 2 What is a statistical model? A simplified representation of reality A model for the association structure in the data Most common ones are regression models A regression model represents how a dependent (outcome) variable Y depends on one or more independent variables (covariates) X George E.P. Box: “All models are wrong, but some are useful.” BMS2043 – Statistics and Data Analysis, 2024 Linear regression (simple and multiple) Linear Regression analysis is used to create a model that describes the relationship between a dependent variable and one or more independent variables. https://datatab.net/tutorial/linear-regression BMS2043 – Statistics and Data Analysis, 2024 Linear regression (simple and multiple) Linear regression seeks to identify the line that minimizes the sum of the squared differences between observed and predicted values. This optimization technique is known as the least squares method. Linear regression is built upon several crucial assumptions. It assumes that there is a linear relationship between the variables being analysed, that the errors in predictions are normally distributed, and that the variance of these errors remains constant (homoscedasticity). Simple Linear Regression https://www.linkedin.com/pulse/understanding-linear-regression-basics-divyesh-sonar-snv4c/ BMS2043 – Statistics and Data Analysis, 2024 Multiple Linear Regression Example: body height predicted by weight Y = Outcome/dependent variable (Y) Body height (cm) X = Predictors/explanatory variables/independent variables Weight: kg Sex: 1 = male, 2 = female Mathematically: Y = 𝛼 + 𝛽1 X1 + 𝛽2 X2 + 𝜺 i.e. Height = 𝛼 + 𝛽1 Weight + 𝛽2 Sex + 𝜺 BMS2043 – Statistics and Data Analysis, 2024 Intercept, 𝛽, standard error (SE), test statistic, P-value Intercept 𝛼: the value of the outcome when X=0 Regression coefficient 𝛽: the slope for X tells about the unit change in Y per a unit change in X 𝛽=0 means that there is no association between X and Y Standard error (SE) of 𝛽: the standard deviation of the estimate Measures how precisely the model estimates the unknown 𝛽 Affected by sample size Test statistic t = 𝛽/SE(𝛽) P-value R2: Variance explained in the outcome by explanatory variables. BMS2043 – Statistics and Data Analysis, 2024 Mean ± SD for symmetric distributions Mean ± 1 SD includes 68.2% of cases Mean ± 2 SD includes 95.4% of cases Mean ± 3 SD includes 99.7% of cases BMS2043 – Statistics and Data Analysis, 2024 Confidence intervals (CI) The point estimate 𝛽 has an error (SE) associated with it telling about its variability if we repeated the test multiple times (remember SEM) We can use the SE to estimate where the point estimate is with a certain confidence (e.g. 90%, 95%, 99%) if we were to repeat the test in multiple samples from the population (ideally infinite times) Calculation: 𝛽 ± z𝛼*SE(𝛽) When calculating 95% CI, z𝛼 = 1.96 à 95% CI: 𝛽 ± 1.96*SE(𝛽) BMS2043 – Statistics and Data Analysis, 2024 Confidence intervals (CI) Example: body height was associated with sex (𝛽=-11.05, SE=0.18). The 95% CIs are: 95% CI: 𝛽 ± 1.96*SE(𝛽) Lower: -11.05-1.96*0.18 = -11.40 Upper: -11.05+1.96*0.18 = -10.70 We get 𝛽=-11.05 (-11.40, -10.70) BMS2043 – Statistics and Data Analysis, 2024 Confidence intervals (CI) If 95% CIs do not include 0, there is a statistically significant association (i.e. P