Statistics for Economics PDF
Document Details
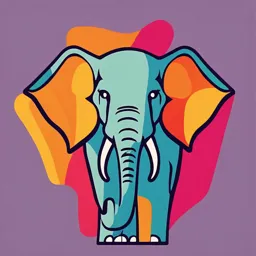
Uploaded by CourteousPoisson6443
Tags
Related
Summary
This document provides a general overview of statistics in economics. It discusses the concepts of statistics, its features, its importance in economics, and some limitations of statistical tools. The concepts of primary/secondary data, types of data and various techniques are discussed in the file.
Full Transcript
Paper [STATISTICS FOR ECONOMICS] SEM-III MODULE - 1 What is Statistics in Economics? Generally, the subject matter of statistics deals with the quantification of data. It revolves around concrete figures to represent qualitative information. Si...
Paper [STATISTICS FOR ECONOMICS] SEM-III MODULE - 1 What is Statistics in Economics? Generally, the subject matter of statistics deals with the quantification of data. It revolves around concrete figures to represent qualitative information. Simply, it is a collection of data. But that’s not all. As economics students, we need to learn about the techniques of dealing with a collection of data, tabulation, classification, and presentation of data. Further, we need to learn about reduction and condensation of data. Lastly, we also need to gain insights into the techniques for analysis and interpretation of data. Features of Statistics in its Plural Sense It is numerically expressed: Statistics in economics deals with numbers and is quantitative. Qualitative adjectives like rich, poor, tall etc. have no attached significance in the statistical universe. Reasonably accurate: A statistical conclusion should be reasonably accurate which depends on the purpose of an investigation, its nature, size and available resources. Can involve estimation: If the field of study is large, for example, the number of people attending a rally, then a fair bit of estimation can do the trick. However for small fields of study, take, for example, the number of students in each field of study in a college, exact number calculation is easy and essential. Systematic collection of data: Collection of data should be done systematically, which means, accumulating just raw data without any information about its origin, purpose etc is not valid in the statistical universe. Relative: Statistics in economics in its plural sense has the feature of comparability. This means that the same kind of data from different sources can be compared. Multiple factors: Statistics is affected by a large number of factors and not just a single factor. For example rise in the price of a commodity is not because of a change in one factor but it is an effect of a large number of factors. Aggregation: Statistics is a game of averages or aggregates. A number expressed for a single entity is no way related to statistics. For example, the height of a single student is not a statistical data but the average height of students in a class is. Statistics in Singular Sense In its singular sense, statistics involves the gathering, presentation, analysis, and interpretation of numerical information for research purposes. Key Characteristics of Statistics in the Singular Sense: Data Collection: Start by deciding how to gather information for your study, then collect the data accordingly. Data Organization: Simplify the collected data for easy comparison by sorting it based on time and place. Data Presentation: Display the organized data in an appealing way, utilizing graphs, charts, diagrams, and tables. Data Analysis: After presentation, analyze the data for accurate results using methods like Measures of Dispersion, Measures of Central Tendency, Interpolation, etc. Data Interpretation: Conclude your findings by using comparisons and making reliable forecasts based on the interpreted data. This step ensures that the collected information is not just numbers but contributes meaningfully to the overall understanding of the subject. Importance of Statistics in Economics In Economics: Statistics contribute to the formulation of economic laws, including the Law of Demand, Law of Supply, Elasticity of Demand, and Elasticity of Supply, using inductive generalization. Economic problems like unemployment and poverty are better understood and addressed through statistical techniques. The study of different market structures, such as perfect competition and monopoly, is facilitated by statistics, which compare the costs, profits, and prices of firms. Economists can estimate mathematical relationships between various economic variables. Statistics help analyze the behavior of economic concepts, such as the laws of supply and demand, providing insights into consumer behavior. Statistical Limitations Similar to everything, statistics in economics are also not free of limitations. These are as follows: 1. Only Quantitative Study The biggest advantage of statistics is also one limitation of the same. Although it is good at studying quantitative data, it fails at analyzing qualitative entities like honesty, wisdom, health etc. 2. Study of Aggregates Another shortcoming of statistics is that it deals only with aggregates and cannot handle data about a single entity. 3. Homogeneous Data One essential requirement for statistics is that data should be uniform and homogeneous. As statistics involves comparison, heterogeneous data cannot be compared. 4. Specific Usage Statistics can be used specifically by people who possess knowledge about the statistical methods. Interestingly, it makes no sense to those who have no knowledge of statistical methods. The field of statistics is concerned with collecting, analyzing, interpreting, and presenting data. In the field of economics, statistics is important for the following reasons: Reason 1: Statistics allows economists to understand the state of the economy using descriptive statistics. Reason 2: Statistics allows economists to spot trends in the economy using data visualizations. Reason 3: Statistics allows economists to quantify the relationship between variables using regression models. Reason 4: Statistics allows economists to forecast trends in the economy. What is a Population vs Sample? Population vs sample is a crucial distinction in statistics. Typically, researchers use samples to learn about populations. Let’s explore the differences between these concepts! o Population: The whole group of people, items, or element of interest. o Sample: A subset of the population that researchers select and include in their study. Researchers might want to learn about the characteristics of a population, such as its mean and standard deviation. Unfortunately, they are usually too large and expensive to study in their entirety. Instead, the researchers draw a sample from the population to learn about it. Collecting data from a subset can be more efficient and cost- effective. Inferential statistics use sample statistics, like the mean and standard deviation, to draw inferences about the corresponding population characteristic If we had to measure entire populations, we’d never be able to answer our research questions because they tend to be too large and unwieldy. Fortunately, we can use a subset to move forward. Definition of Frequency Distribution Frequency distribution is a statistical tool used to organize and analyze a set of data. It shows how frequently each unique value occurs within a dataset. Essentially, it is a summary of the data that provides a snapshot of the patterns of values or intervals. Frequency distributions can be displayed in several formats, including tables, histograms, or pie charts. Example Consider a teacher who wants to analyze the distribution of scores for a recent class test. The test scores of 30 students are as follows: 55, 70, 85, 90, 75, 60, 80, 95, 70, 55, 80, 75, 65, 70, 60, 85, 100, 90, 70, 55, 75, 80, 65, 95, 60, 85, 75, 90, 65, and 75. To create a frequency distribution, the teacher first decides the number of intervals or “bins.” Let’s say they choose bins of width 10, starting from 50-59, 60-69, and so on up to 100. The frequency of scores within each bin would then be tallied. For example, the 50-59 interval includes three scores (55, 55, and 55), the 60-69 interval includes four scores (60, 60, 60, and 65), and so on. This process results in a table that clearly shows how the test scores are distributed across these intervals. Why Frequency Distribution Matters Frequency distributions are invaluable in the field of statistics and data analysis because they allow researchers and analysts to quickly view the distribution of a data set and make inferences about the population from which the data was drawn. For example, a frequency distribution can show whether the data are skewed toward high or low values or if they are uniformly distributed. Additionally, this analysis can reveal outliers, peaks, or clusters in the data, offering insights into patterns or tendencies that might not be immediately obvious. Visualizing the frequency distribution, such as through a histogram, can further enhance understanding by providing a graphical representation of data distribution. This can make it easier to see the shape of the data distribution, be it normal, bimodal, or skewed, facilitating more informed decision-making and analysis. Types of Frequency Distribution The frequency distribution is further classified into five. These are: Exclusive Series In such a series, for a particular class interval, all the data items having values ranging from its lower limit to just below the upper limit are counted in the class interval. In other words, we do not include the items that have values less than the lower limit, equal to the upper limit and greater than the upper limit. Note that here the upper limit of a class repeats itself in the lower limit of the next interval. This is the most used type of frequency distribution. Weight Frequency 40-50 2 50-60 10 60-70 5 70-80 3 Inclusive Series On the contrary to exclusive series, an inclusive series includes both its upper and lower limit. Of course, this means that we do not include the items with values less than the lower limit and greater than the upper limit. Marks Frequency 10-19 5 20-29 13 30-39 6 Open End Series In an open-end series, the lower limit of the first class in the series and the upper limit of the last class in the series is missing. Instead, there is ‘below the lower limit’ of the first class and ‘lower limit and above the lower limit’ of the last class. Age Frequency Below 4 5 5-10 6 10-20 10 20 and 8 above Cumulative Frequency Series In a cumulative frequency series, we either add or subtract the frequencies of all the preceding class intervals to determine the frequency for a particular class. Further, the classes are converted into either ‘less than the upper limit’ or ‘more than the lower limit’. Mid-Values Frequency Series A mid-value frequency series is the one in which we have the mid values of class intervals and the corresponding frequencies. In other words, the mid values represent the range of a particular class interval. To determine the upper and lower limits of a class represented by its mid- value we can use the following formulas: Lower Limit= m – (1\2)×i Upper Limit= m – (1\2)×i Here, m= The mid value of the class i= Difference between the mid-values What is Cumulative Frequency? Cumulative frequency is the running total of frequencies in a table. Use cumulative frequencies to answer questions about how often a characteristic occurs above or below a particular value. It is also known as a cumulative frequency distribution. For example, how many students are in the 4th grade or lower at a school? Cumulative frequency builds on the concepts of frequency and frequency distribution. o Frequency: The number of times a value occurs in a dataset. For example, there are 12 4th graders in the school. o Frequency distribution: A table that lists all values in the dataset and how many times each one occurs. Learn more about Frequency Tables. In this post, learn how to find and construct cumulative frequency distributions for both discrete and continuous data. I’ll also show you how to create less than and greater than versions of these tables. Definition of Cumulative Frequency In statistics, the frequency of the first-class interval is added to the frequency of the second class, and this sum is added to the third class and so on then, frequencies that are obtained this way are known as cumulative frequency (c.f.). A table that displays the cumulative frequencies that are distributed over various classes is called a cumulative frequency distribution or cumulative frequency table. There are two types of cumulative frequency - lesser than type and greater than type. Cumulative frequency is used to know the number of observations that lie above (or below) a particular frequency in a given data set. Let us look at a few examples that are used in many real-world situations. What is Cumulative Frequency? Cumulative frequency is the running total of frequencies in a table. Use cumulative frequencies to answer questions about how often a characteristic occurs above or below a particular value. It is also known as a cumulative frequency distribution. For example, how many students are in the 4th grade or lower at a school? Cumulative frequency builds on the concepts of frequency and frequency distribution. o Frequency: The number of times a value occurs in a dataset. For example, there are 12 4th graders in the school. o Frequency distribution: A table that lists all values in the dataset and how many times each one occurs. Learn more about Frequency Tables. In this post, learn how to find and construct cumulative frequency distributions for both discrete and continuous data. I’ll also show you how to create less than and greater than versions of these tables. How to Find Cumulative Frequency Finding a cumulative frequency distribution makes the most sense when your data have a natural order. The natural ordering allows the cumulative running total to be meaningful. With a minor change, the process works with both discrete and continuous data. Learn more about the differences between Discrete vs. Continuous Data. For example, the grades in a school, months of a year, or age in years are discrete values with a logical order. Alternatively, when you have continuous data, you can create ranges of values known as classes. In this case, frequencies are counts of how often continuous data fall within each class. Calculate cumulative frequency by starting at the top of a frequency table and working your way down. Take each row’s frequency and add all preceding rows. By summing the current and previous rows, you calculate the running total. TYPES OF DATA 1. Primary data: The data which are collected by an investigator originally from its basic source for the first time for any statistical inquiry are known as primary data. The primary data are also called first-hand data. As it is collected directly from the informants. Primary data are generally used in those cases where the secondary data do not provide an adequate basis for analysis. Primary data are also called field source. Example: Data obtained in a population census by C.B.S (Central Bureau of statistics) are primary data of the same organization. 2. Secondary data: Those data which are collected by one agency organization or person but used by other agency, organization or person is called secondary data. These types of data are not original for the user. These are also called second-hand data. The data which are already collected by someone but obtained from some published and unpublished sources are called secondary data. Example: For Central Bureau of statistics, the census data are primary whereas, for all others we use, such data are secondary. Differences Between Primary and Secondary Data: Primary data Secondary data Data collected the first time Data that are already collected and from the field of study are used by others are called secondary called primary data. data. They are first hand or original They are second hand in nature. in nature. It gives more accurate Sometimes secondary data may not information. be accurate. They are like raw materials and They are found in ready-made form they have to be processed just like finished goods. after collection. Collection of primary data Secondary data save money, time takes a large amount of money and efforts because these are used and efforts. from the existing sources. There is no need to worry Secondary data should be carefully about while using primary data and critically examined before they from the investigator. are used. Techniques Methods of Data Collection. There are two types of techniques of data collection: 1. Census: A census is a technique of data collection where the information is collected from each and every unit of the population associated with the subject matter of enquiry. In Nepal, census occurs every ten years. In such census information about each and every individual of the country is collected. Not a single individual is left out in such a census. Merits: a) It gives complete information about the population. b) This method is more suitable for a limited area. Demerits: a) It is more expensive, labour requiring and time-consuming. b) This method is impossible if the population size is infinite. 2. Sampling method: In this method, only the part of population units is selected as a representative of the whole population. The selected part of the units is called sample and the method of selecting a sample is called a sample method. The number of items in the sample is known as sample size. For example, 15 people are drawn from a population of 250 people from a village to know the drinking habits of those 15 people are the sample for the study. Merits: a) This method is less time consuming, less labour requiring and cheaper. b) In the case of infinite population, it is a suitable method. Demerits: a) The sample units may not represent the population. b) Due to the biases of the indicator, the result obtained may be misleading. MODULE – 2 Measures of Central Tendency The measurable characteristics such as rainfall, elevation, density of population, levels of educational attainment or age groups vary. If we want to understand them, how would we do ? We may, perhaps, require a single value or number that best represents all the observations. This single value usually lies near the centre of a distribution rather than at either extreme. The statistical techniques used to find out the centre of distributions are referred as measures of central tendency. The number denoting the central tendency is the representative figure for the entire data set because it is the point about which items have a tendency to cluster. Measures of central tendency are also known as statistical averages. There are a number of the measures of central tendency, such as the mean, median and the mode. Mean The mean is the value which is derived by summing all the values and dividing it by the number of observations Presentation of Data 1. Arithmetic Mean – In statistics, the Arithmetic Mean (AM), also called an average, is the ratio of the sum of all observations to the total number of observations. The arithmetic mean can also inform or model concepts outside of statistics. In a physical sense, the arithmetic mean can be thought of as a centre of gravity. From the mean of a data set, one can think of the average distance the data points are from the mean as standard deviation. The square of standard deviation (i.e. variance) is analogous to the moment of inertia in the physical model. Arithmetic mean represents a number that is obtained by dividing the sum of the elements of a set by the number of values in the set. 2. Geometric Mean – The Geometric Mean (GM) is the average value or mean, which signifies the central tendency of the set of numbers by finding the product of their values. Basically, one multiplies the numbers altogether and takes the nth root of the multiplied numbers, where n is the total number of data values. For example, for a given set of two numbers, such as 3 and 1, the geometric mean is equal to √(3×1) = √3 = 1.732. In other words, the geometric mean is defined as the nth root of the product of n numbers. It is noted that the geometric mean is different from the arithmetic mean. 3. Harmonic Mean – The Harmonic Mean (HM) is defined as the reciprocal of the average of the reciprocals of the data values. It is based on all the observations, and it is rigidly defined. The harmonic mean gives less weightage to the large values and large weightage to the small values to balance the values correctly. In general, the harmonic mean is used when there is a necessity to give greater weight to the smaller items. It is applied in the case of times and average rates. 4. Quartiles – Quartiles divide the entire set into four equal parts. So, there are three quartiles, first, second, and third, represented by Q1, Q2, and Q3, respectively. Q2 is nothing but the median, since it indicates the position of the item in the list and, thus, is a positional average. To find quartiles of a group of data, one has to arrange the data in ascending order. 5. Percentile – A percentile is defined as the percentage of values found under specific values. Percentiles are mostly used in the ranking system. It is based on dividing up the normal distribution of the values. Percentile is represented as xth, where x is a number. For example, assume that a student has the 80th percentile on a test of 150. By this, one can understand the term percentile better and know that by scoring 150 in the exam, a student has beaten 80% of the remaining class in the exam. Good Average (i) Good Average should be based on all the observations: Only those averages, where all the data are used give best result, whereas the averages which use less data are not representative of the whole group. (ii) Good Average should not be unduly affected by extreme value: No term should affect the average too much. If one or two very small or very large items unduly affect the average, then the average cannot be really typical of the entire group. Thus extreme terms may distort the average and reduce its usefulness. As if in example given, we are to find average of 6, 8, 10, we get mean as 8, but if another item, having value 200 is taken, the average comes out to be 56. Hence it can’t be called a good average as with only one new item, it has increased from 8 to 56. (iii) Good Average should be rigidly defined: There should be no confusion about the meaning or description of an average. It must have a rigid or to the point definition. (iv) Good Average should be easy to calculate and simple to understand: If the calculation of an average involves too much mathematical processes, it will not be easily understood and its use will be limited only to a limited number of persons. This average cannot be a popular average. It should be easy to understand. (v) Good Average should be capable of further algebraic treatment: Measures of central tendency are used in many other techniques of statistical analysis like measures of Dispersion, Correlation etc. (vi) Good Average should be found by graphic methods also: That average is considered a good average which can be found by arithmetic as well as by graphic method. (vii) Good Average should not be affected by variations of sampling: A good average will be least affected by sampling fluctuations. If a few samples are taken from the same universe, the average should be such as has the least variation in values derived in the individual samples. The results obtained will be considered to be the true representative of the universe in this case. (viii) Good Average should not be affected by skewness: We will not call an average good one if it is affected by skewness present in the distribution. Comparison between Mean, Median, and Mode The decision of which approach to employ for a particular collection of data depends on a number of factors that can be grouped into the following major categories: 1. Rigidly Defined: Mean and median are defined rigidly; on the other hand, mode is not always rigidly defined. 2. Based on all Observations: A suitable average should be calculated based on all observations. This attribute is only met by mean and not by median or mode. 3. Possess Sampling Stability: Mean should be preferred when the criteria of least sampling variability is to be attained. 4. Additional Algebraic Treatment: It should be able to get additional mathematical treatment. This attribute can only be satisfied by the mean, hence the majority of statistical theories utilise the mean as a measure of central tendency. 5. Simple to Calculate and Understand: It should be simple to understand and interpret an average. All three averages; i.e., mean, median, and mode satisfy this attribute. 6. Not significantly Impacted by Extreme Values: The appropriate average shouldn’t be significantly impacted by extreme observations. From this perspective, the mode acts as the most appropriate average. The existence of extreme observations has a very small effect on the median but a large impact on the mean. What is Dispersion in Statistics? Dispersion is the state of getting dispersed or spread. Statistical dispersion means the extent to which numerical data is likely to vary about an average value. In other words, dispersion helps to understand the distribution of the data. Measures of Dispersion In statistics, the measures of dispersion help to interpret the variability of data i.e. to know how much homogenous or heterogeneous the data is. In simple terms, it shows how squeezed or scattered the variable is. Types of Measures of Dispersion There are two main types of dispersion methods in statistics which are: Absolute Measure of Dispersion Relative Measure of Dispersion Absolute Measure of Dispersion An absolute measure of dispersion contains the same unit as the original data set. The absolute dispersion method expresses the variations in terms of the average of deviations of observations like standard or means deviations. It includes range, standard deviation, quartile deviation, etc. The types of absolute measures of dispersion are: 1. Range: It is simply the difference between the maximum value and the minimum value given in a data set. Example: 1, 3,5, 6, 7 => Range = 7 -1= 6 2. Variance: Deduct the mean from each data in the set, square each of them and add each square and finally divide them by the total no of values in the data set to get the variance. Variance (σ2) = ∑(X−μ)2/N 3. Standard Deviation: The square root of the variance is known as the standard deviation i.e. S.D. = √σ. 4. Quartiles and Quartile Deviation: The quartiles are values that divide a list of numbers into quarters. The quartile deviation is half of the distance between the third and the first quartile. 5. Mean and Mean Deviation: The average of numbers is known as the mean and the arithmetic mean of the absolute deviations of the observations from a measure of central tendency is known as the mean deviation (also called mean absolute deviation). Relative Measure of Dispersion The relative measures of dispersion are used to compare the distribution of two or more data sets. This measure compares values without units. Common relative dispersion methods include: 1. Co-efficient of Range 2. Co-efficient of Variation 3. Co-efficient of Standard Deviation 4. Co-efficient of Quartile Deviation 5. Co-efficient of Mean Deviation Co-efficient of Dispersion The coefficients of dispersion are calculated (along with the measure of dispersion) when two series are compared, that differ widely in their averages. The dispersion coefficient is also used when two series with different measurement units are compared. It is denoted as C.D. The common coefficients of dispersion are: C.D. in terms of Coefficient of dispersion Range C.D. = (Xmax – Xmin) ⁄ (Xmax + Xmin) Quartile Deviation C.D. = (Q3 – Q1) ⁄ (Q3 + Q1) C.D. in terms of Coefficient of dispersion Standard Deviation (S.D.) C.D. = S.D. ⁄ Mean Mean Deviation C.D. = Mean deviation/Average MODULE-III What is Correlation? A statistical tool that helps in the study of the relationship between two variables is known as Correlation. It also helps in understanding the economic behaviour of the variables. Two Variables are said to be Correlated if: The two variables are said to be correlated if a change in one causes a corresponding change in the other variable. For example, A change in the price of a commodity leads to a change in the quantity demanded. An increase in employment levels increases the output. When income increases, consumption increases as well. The degree of correlation between various statistical series is the main subject of analysis in such circumstances. Significance of Correlation 1. It helps determine the degree of correlation between the two variables in a single figure. 2. It makes understanding of economic behaviour easier and identifies critical variables that are significant. 3. When two variables are correlated, the value of one variable can be estimated using the value of the other. This is performed with the regression coefficients. 4. In the business world, correlation helps in taking decisions. The correlation helps in making predictions which helps in reducing uncertainty. It is so because the predictions based on correlation are probably reliable and close to reality. Types of Correlation Correlation can be classified based on various categories: Based on the direction of change in the value of two variables, correlation can be classified as: 1. Positive Correlation: When two variables move in the same direction; i.e., when one increases the other also increases and vice-versa, then such a relation is called a Positive Correlation. For example, Relationship between the price and supply, income and expenditure, height and weight, etc. 2. Negative Correlation: When two variables move in opposite directions; i.e., when one increases the other decreases, and vice-versa, then such a relation is called a Negative Correlation. For example, the relationship between the price and demand, temperature and sale of woollen garments, etc. Based on the ratio of variations between the variables, correlation can be classified as: 1. Linear Correlation: When there is a constant change in the amount of one variable due to a change in another variable, it is known as Linear Correlation. This term is used when two variables change in the same ratio. If two variables that change in a fixed proportion are displayed on graph paper, a straight- line will be used to represent the relationship between them. As a result, it suggests a linear relationship. In the above graph, for every change in the variable X by 5 units there is a change of 10 units in variable Y. The ratio of change of variables X and Y in the above schedule is 1:2 and it remains the same, thus there is a linear relationship between the variables. 2. Non-Linear (Curvilinear) Correlation: When there is no constant change in the amount of one variable due to a change in another variable, it is known as a Non-Linear Correlation. This term is used when two variables do not change in the same ratio. This shows that it does not form a straight-line relationship. For example, the production of grains would not necessarily increase even if the use of fertilizers is doubled. In the above schedule, there is no specific relationship between the variables. Even though both change in the same direction i.e. both are increasing, they change in different proportions. The ratio of change of variables X and Y in the above schedule is not the same, thus there is a non-linear relationship between the variables. Based on the number of variables involved, correlation can be classified as: 1. Simple Correlation: Simple correlation implies the study between the two variables only. For example, the relationship between price and demand, and the relationship between price and money supply. 2. Partial Correlation: Partial correlation implies the study between the two variables keeping other variables constant. For example, the production of wheat depends upon various factors like rainfall, quality of manure, seeds, etc. But, if one studies the relationship between wheat and the quality of seeds, keeping rainfall and manure constant, then it is a partial correlation. 3. Multiple Correlation: Multiple correlation implies the study between three or more three variables simultaneously. The entire set of independent and dependent variables is studied simultaneously. For example, the relationship between wheat output with the quality of seeds and rainfall. What is Karl Pearson’s Coefficient of Correlation? The first person to give a mathematical formula for the measurement of the degree of relationship between two variables in 1890 was Karl Pearson. Karl Pearson’s Coefficient of Correlation is also known as Product Moment Correlation or Simple Correlation Coefficient. This method of measuring the coefficient of correlation is the most popular and is widely used. It is denoted by ‘r’, where r is a pure number which means that r has no unit. According to Karl Pearson, “Coefficient of Correlation is calculated by dividing the sum of products of deviations from their respective means by their number of pairs and their standard deviations.” Karl Pearson’s Coefficient of Correlation(r)=Sum of Products of Deviatio ns from their respective meansNumber of Pairs×Standard Deviations of both SeriesKarl Pearson’s Coefficient of Correlation(r)=Number of Pairs ×Standard Deviations of both SeriesSum of Products of Deviations from their respective means Or r=∑xyN×σx×σyr=N×σx×σy∑xy Where, N = Number of Pair of Observations x = Deviation of X series from Mean (X−Xˉ)(X−Xˉ) y = Deviation of Y series from Mean (Y−Yˉ)(Y−Yˉ) σx σx = Standard Deviation of X series (∑x2N)(N∑x2) σy σy = Standard Deviation of Y series (∑y2N)(N∑y2) r = Coefficient of Correlation Example 1: Determine the Coefficient of Correlation between X and Y. The summation of the product of deviations of Series X and Y from their respective means is 200. Solution: The figures given are: N = 30, σx = 4, σy = 3, and ∑xy = 200 r=∑xyN×σx×σyr=N×σx×σy∑xy =20030×4×3=5090=0.5=30×4×3200=9050=0.5 Coefficient of Correlation = 0.5 It means that there is a positive correlation between X and Y. Features of Karl Pearson’s Coefficient of Correlation The main features of Karl Pearson’s Coefficient of Correlation are as follows: 1. Knowledge of Direction of Correlation: This method of measuring coefficient of correlation gives us knowledge about the direction of the relationship between two variables. In other words, it tells us whether the relationship between two variables is positive or negative. 2. Size of Correlation: Karl Pearson’s Coefficient of Correlation indicates the size of the relationship between two variables. Besides, Correlation Coefficient ranges between -1 and +1. 3. Indicates Magnitude and Direction: This method not only specifies the magnitude of the correlation between two variables but also specifies its direction. It means that, if two variables are directly related, then the correlation coefficient between the variables will be a positive value. However, if two variables are inversely related, then the correlation coefficient between the variables will be a negative value. 4. Ideal Measure: As this method is based on the most essential statistical measure, such as standard deviation and mean, it is an ideal/appropriate measure. Spearman’s Correlation Explained Spearman’s correlation in statistics is a nonparametric alternative to Pearson’s correlation. Use Spearman’s correlation for data that follow curvilinear, monotonic relationships and for ordinal data. Statisticians also refer to Spearman’s rank order correlation coefficient as Spearman’s ρ (rho). In this post, I’ll cover what all that means so you know when and why you should use Spearman’s correlation instead of the more common Pearson’s correlation. To learn more about correlation in general, and Pearson’s correlation in particular, read my post about Interpreting Correlation Coefficients. Throughout this post, I graph the data. Graphing is crucial for understanding the type of relationship between variables. Seeing how variables are related helps you choose the correct analysis! Spearman’s rank correlation coefficient, denoted by 𝑟 ,is a measure of the tendency for one variable to increase or decrease as the other does within a monotonic (entirely increasing or entirely decreasing) relationship, such that −1≤𝑟≤1. If one variable always increases as the other does, we can say that the value of 𝑟 is positive and there is a direct association between the variables. On the other hand, if one variable always decreases as the other increases, then we can say that the value of 𝑟 is negative and this indicates an inverse association. Rank correlation coefficient values of 1 or −1 describe a perfectly associated monotonic relationship. This means that either the ranks agree entirely (𝑟=1) or they are direct opposites (𝑟=−1).Unlike with Pearson’s correlation coefficient, a perfect 𝑟 value of −1 or 1 can occur regardless of whether the quantitative data pairs in a set are linearly related or not. Not only can 𝑟 be 1 or −1,but also it can have any value between −1 and 1. A value of 0 for 𝑟 indicates no association between the variables. The closer the value of 𝑟 is to −1 or 1, the stronger the association, and the closer it is to 0, the weaker the association. Definition: Spearman’s Rank Correlation Coefficient Spearman’s rank correlation coefficient, denoted by 𝑟 ,is a numerical value such that −1≤𝑟≤1.It gives a measure of the likelihood of one variable increasing as the other increases (a direct association) or of one variable decreasing as the other increases (an inverse association). Direct associations are indicated by positive values, and inverse associations are indicated by negative values. No association is indicated by a value of 0. The stronger the association, the closer 𝑟 is to −1 or 1, and the weaker the association, the closer it is to 0. Rank correlation coefficient values of 1 or −1 mean that either the ranks agree entirely (𝑟=1) or they are direct opposites (𝑟=−1). Our first step in determining the value of 𝑟 for a set of 𝑛 bivariate data pairs is to rank the values of each variable. In a quantitative data set, the smallest rank for a variable can be assigned to either the least or the greatest data value, but each variable must be ranked in the same way. That is, both must be ranked either from least to greatest or from greatest to least. Also, if two data values are the same, then their ranks must also be the same. Thus, the ranks of two or more identical data values are equal to the average of their places in an ordered list. The identical data values are said to have tied ranks. Formula: Spearman’s Rank Correlation Coefficient The formula for Spearman’s rank correlation coefficient is 𝑟=1−6∑𝑑𝑛(𝑛−1) ,where 𝑟 is the coefficient and 𝑛 is the number of points in the data set. For each point (𝑥,𝑦) ,the square of the difference in the ranks of the two coordinates is represented by 𝑑 ,and the sum of each of these squares is represented by the expression 𝑑. What Is Regression? Regression is a statistical method used in finance, investing, and other disciplines that attempts to determine the strength and character of the relationship between a dependent variable and one or more independent variables. Linear regression is the most common form of this technique. Also called simple regression or ordinary least squares (OLS), linear regression establishes the linear relationship between two variables. Linear regression is graphically depicted using a straight line of best fit with the slope defining how the change in one variable impacts a change in the other. The y-intercept of a linear regression relationship represents the value of the dependent variable when the value of the independent variable is zero. Nonlinear regression models also exist, but are far more complex. Key Takeaways Regression is a statistical technique that relates a dependent variable to one or more independent variables. A regression model is able to show whether changes observed in the dependent variable are associated with changes in one or more of the independent variables. It does this by essentially determining a best-fit line and seeing how the data is dispersed around this line. Regression helps economists and financial analysts in things ranging from asset valuation to making predictions. For regression results to be properly interpreted, several assumptions about the data and the model itself must hold. In economics, regression is used to help investment managers value assets and understand the relationships between factors such as commodity prices and the stocks of businesses dealing in those commodities. While a powerful tool for uncovering the associations between variables observed in data, it cannot easily indicate causation. Regression as a statistical technique should not be confused with the concept of regression to the mean, also known as mean reversion. Understanding Regression Regression captures the correlation between variables observed in a data set and quantifies whether those correlations are statistically significant or not. The two basic types of regression are simple linear regression and multiple linear regression, although there are nonlinear regression methods for more complicated data and analysis. Simple linear regression uses one independent variable to explain or predict the outcome of the dependent variable Y, while multiple linear regression uses two or more independent variables to predict the outcome. Analysts can use stepwise regression to examine each independent variable contained in the linear regression model. Regression can help finance and investment professionals. For instance, a company might use it to predict sales based on weather, previous sales, gross domestic product (GDP) growth, or other types of conditions. The capital asset pricing model (CAPM) is an often-used regression model in finance for pricing assets and discovering the costs of capital. Types: 1. Linear Regression Linear regression is used to fit a regression model that describes the relationship between one or more predictor variables and a numeric response variable. Logistic Regression Logistic regression is used to fit a regression model that describes the relationship between one or more predictor variables and a binary response variable. Polynomial Regression Polynomial regression is used to fit a regression model that describes the relationship between one or more predictor variables and a numeric response variable.. Ridge Regression Ridge regression is used to fit a regression model that describes the relationship between one or more predictor variables and a numeric response variable. Quantile Regression Quantile regression is used to fit a regression model that describes the relationship between one or more predictor variables and a response variable. MODULE-IV Meaning of Index Numbers: The value of money does not remain constant over time. It rises or falls and is inversely related to the changes in the price level. A rise in the price level means a fall in the value of money and a fall in the price level means a rise in the value of money. Thus, changes in the value of money are reflected by the changes in the general level of prices over a period of time. Changes in the general level of prices can be measured by a statistical device known as ‘index number.’ Index number is a technique of measuring changes in a variable or group of variables with respect to time, geographical location or other characteristics. There can be various types of index numbers, but, in the present context, we are concerned with price index numbers, which measures changes in the general price level (or in the value of money) over a period of time. Price index number indicates the average of changes in the prices of representative commodities at one time in comparison with that at some other time taken as the base period. According to L.V. Lester, “An index number of prices is a figure showing the height of average prices at one time relative to their height at some other time which is taken as the base period.” Uses of Index Number in Statistics We have known the features and types of the Index numbers. For a further comprehensive study, we will now discuss the uses of Index numbers. Index numbers are useful in many basic to complicated studies. Like it is used in the basic study of human population in a country and also it is used to determine the extinction rate of the rare animals in a particular region. There are many more usages of Index Numbers, let us find out: It helps in measuring changes in the standard of living as well as the price level. Wage rate regulation is consistent with the changes in the price level. With the determination of price levels, wage rates may be revised. Government policies are framed following the index number of prices. This price stability inherent to fiscal and economic policies is based on index numbers. It gives a pointer for international comparison concerning different economic variables—for instance, living standards between two countries. Importance of Index Number Index numbers are most commonly used in the study of the economic status of a particular region. As mentioned, the index number defines the level of a variable relative to the level in a particular period of time span. These index numbers serve as a measure to study the change in the effects of all the factors that cannot be measured or estimated on a direct basis. Thus, Index numbers occupy an important place due to their efficacy in measuring the extent of economic changes across a stipulated period. It helps to study such changes' effects due to factors that cannot be directly measured. Features of Index Numbers: The following are the main features of index numbers: (i) Index numbers are a special type of average. Whereas mean, median and mode measure the absolute changes and are used to compare only those series which are expressed in the same units, the technique of index numbers is used to measure the relative changes in the level of a phenomenon where the measurement of absolute change is not possible and the series are expressed in different types of items. (ii) Index numbers are meant to study the changes in the effects of such factors which cannot be measured directly. For example, the general price level is an imaginary concept and is not capable of direct measurement. But, through the technique of index numbers, it is possible to have an idea of relative changes in the general level of prices by measuring relative changes in the price level of different commodities. (iii) The technique of index numbers measures changes in one variable or group of related variables. For example, one variable can be the price of wheat, and group of variables can be the price of sugar, the price of milk and the price of rice. (iv) The technique of index numbers is used to compare the levels of a phenomenon on a certain date with its level on some previous date (e.g., the price level in 1980 as compared to that in 1960 taken as the base year) or the levels of a phenomenon at different places on the same date (e.g., the price level in India in 1980 in comparison with that in other countries in 1980). Difficulties faced in the Construction of Index Numbers The production of index numbers is fraught with challenges. Following are the difficulties faced in the Construction of Index Numbers – 1. Difficulties in Choosing a Base Period: The first challenge is determining which year to use as the starting point. The foundation year must be standard. However, determining a causal year is tough. Furthermore, a typical year now becomes abnormal after a certain amount of time. As a result, having the same base period for several years is not recommended. 2. Problem in Commodity Selection: Another challenge is selecting the index number’s representative commodities. The selection is not a simple task. They must be chosen from a diverse range of things that are consumed. Consumer consumption patterns may change, rendering the number of indexes obsolete. As a result, selecting representative commodities involves significant challenges. 3. Problems in Price Compendium: Another challenge is obtaining enough and correct value. It’s not always possible to obtain from the same location. Furthermore, the issue of deciding middle prices arises. The retail cost differs greatly. As a result, wholesale prices are calculated using index numbers. 4. Difficulty in Choosing a Statistical Approach: Another challenge is deciding on a suitable approach for calculating averages. However, each strategy produces a unique set of findings. As a result, deciding which strategy to use is challenging. 5. Difficulties Resulting from Changes Over Time: In today’s world, changes in commodities occur on a continuous basis because of technological advancements. So, consumers begin to consume them, and instead of the old, new commodities are introduced. Furthermore, commodity prices may fluctuate because of technological advancements. They might fall. However, when calculating the index numbers, new commodities are not added to the list of commodities. As a result, the index figures based on ancient commodities are unreal. 6. It is not possible to make a comparison: Index numbers do not allow for international pricing comparisons. The goods consumed and included in the calculation of an index number vary by country. Meat, eggs, automobiles, and electrical appliances, for example, are included in advanced countries’ price indices but not in backward countries. Similarly, the weights allocated to goods differ. As a result, international comparisons of index numbers are impossible. 7. It is not possible to make comparisons between different locations: Even if various locations within a country are chosen, the same index number cannot be assigned to them. This is due to variances in people’s consumption habits. Individuals in the northern part of India consume different commodities than people in the southern portion of India. As a result, applying the same index number to both is incorrect. 8. Not Appropriate to Individuals: An index number is not applicable to a single person who is a member of the group it was created. A person may not be affected if there is a rise in the price level index number shows. This is since an index number reflects averages. How would You identify an Index Number? – Features and Characteristics of Index Numbers The main highlighting features of index numbers are mentioned as below– It is a special category of average for measuring relative changes in such instances where absolute measurement cannot be undertaken Index number only shows the tentative changes in factors that may not be directly measured. It gives a general idea of the relative changes The method of index number measure alters from one variable to another related variable It helps in the comparison of the levels of a phenomenon concerning a specific date and to that of a previous date It is representative of a special case of averages especially for a weighted average Index numbers have universal utility. The index that is used to ascertain the changes in price can also be used for industrial and agricultural production. Types of Index Numbers There are various types of index numbers that have particular usage. We will study the types of Index numbers to know the same. This section which is related to the types of Index numbers will help the students to understand the importance of each type in regard to the task which is practiced for. Value Index A value index number is formed from the ratio of the aggregate value for a particular period with that of the aggregate value that is found in the base period. The value index is utilized for inventories, sales, and foreign trade, among others. Quantity Index A quantity index number is used to measure changes in the volume or quantity of goods that are produced, consumed, and sold within a stipulated period. It shows the relative change across a period for particular quantities of goods. Index of Industrial Production (IIP) is an example of Quantity Index. Price Index A price index number is used to measure how price alters across a period. It will indicate the relative value and not the absolute value. The Consumer Price Index (CPI) and Wholesale Price Index (WPI) are major examples of a price index. How to Calculate Index Numbers in Economics? There are two ways to construct index numbers in statistics: The aggregative and Averaging relatives methods. 1. Aggregative method of calculating index numbers The aggregative method is commonly used to calculate the price index. In this method, the index number (P) = the sum of all the values of all the commodities in the current year (P1) divided by the sum of all the values of the same commodities in the base year (P0) and multiplied by 100. The formula for Simple Aggregative price index: P01=Σp1/ Σp0x100 Where P01= Current price Index number Σp1 = the total of commodity prices in the current year Σp0 = the total of the same commodity prices in the base year. However, there is a limitation to using the aggregative method to calculate the index numbers in economics. Across a time frame, the quantities of purchased goods change. Hence, if a particular commodity A is bought more than commodity B, the overall change in the index number is affected by this difference in the “weights” of goods. The simple aggregative price index calculates only the “unweighted” index number wherein all commodities are assumed equal weight or importance. The formula to calculate the weighted aggregative price index or Laspeyre’s price index considers the relative importance of all commodities in terms of the quantity (q0) of that product from the base year. The resultant index number answers how much the price has changed from the base year to the selected year if the same quantities were bought as before. Alternatively, Paasche’s price index is calculated by exchanging q0 with quantities bought in the selected year (q1) to see how much price has changed with current quantity consumption since the base year. 1. Averaging relatives method of calculating index numbers In cases when the number(n) of commodities is high, averaging relatives method is used to calculate index numbers in economics. Another kind of price relative index is a weighted relative index wherein weights are the percentage of expenditure done on a given commodity during the base period or current period, using the different formulae. There are two broad categories of Index Numbers: viz., Weighted Index Numbers and Unweighted Index Numbers. Weighted Index Numbers can be determined using three methods; viz., Laspeyre’s, Paasche’s, and Fisher’s Methods. 1. Laspeyre’s Method The method of calculating Weighted Index Numbers under which the base year quantities are used as weights of different items is known as Laspeyre’s Method. The formula for Laspeyre’s Price Index is: Laspeyre’s Price Index (P01)=∑p1q0∑p0q0×100Laspeyre’s Price Index (P01)=∑p0q0∑p1q0×100 Here, P01 = Price Index of the current year p0 = Price of goods at base year q0 = Quantity of goods at base year p1 = Price of goods at the current year Example: Calculate Price Index Numbers for 2021 with 2014 as base with the help of Laspeyre’s Method. Solution: Laspeyre’s Price Index (P01)=∑p1q0∑p0q0×100Laspeyre’s Price Index (P01)=∑p0q0∑p1q0×100 =8,2806,640×100=6,6408,280×100 = 124.69 Laspyre’s Price Index = 124.69 2. Paasche’s Method The method of calculating Weighted Index Numbers under which the current year’s quantities are used as weights of different items is known as Paasche’s Method. The formula for Paasche’s Price Index is: Paasche’s Index Number (P01)=∑p1q1∑p0q1×100Paasche’s Index Nu mber (P01)=∑p0q1∑p1q1×100 Here, P01 = Price Index of the current year p0 = Price of goods in the base year q1 = Quantity of goods in the base year p1 = Price of goods in the current year Example: Calculate Price Index Numbers for 2021 with 2014 as base with the help of Paasche’s Method. Solution: Paasche’s Index Number (P01)=∑p1q1∑p0q1×100Paasche’s Index Nu mber (P01)=∑p0q1∑p1q1×100 =7,1605,880×100=5,8807,160×100 = 121.76 Paasche’s Index Number = 121.76 3. Fisher’s Method The method of calculating Weighted Index Numbers under which the combined techniques of Paasche and Laspeyre are used is known as Fisher’s Method. In other words, both the base year and current year’s quantities are used as weights. The formula for Fisher’s Price Index is: Fisher’s Price Index (P01)=∑p1q0∑p0q0×∑p1q1∑p0q1×100Fisher’s Pric e Index (P01)=∑p0q0∑p1q0×∑p0q1∑p1q1×100 Here, P01 = Price Index of the current year p0 = Price of goods in the base year q1 = Quantity of goods in the base year p1 = Price of goods in the current year Fisher’s Method is considered the Ideal Method for Constructing Index Numbers. Example: Calculate Price Index Numbers for 2021 with 2014 as the base with the help of Fisher’s Method. Solution: Fisher’s Price Index (P01)=∑p1q0∑p0q0×∑p1q1∑p0q1×100Fisher’s Pric e Index (P01)=∑p0q0∑p1q0×∑p0q1∑p1q1×100 =8,2806,460×7,1605,880×100=6,4608,280×5,8807,160×100 =1.28×1.21×100=1.28×1.21×100 = 124.45 Fisher’s Index Number = 124.45 MODULE – V Time series analysis is a specific way of analyzing a sequence of data points collected over an interval of time. In time series analysis, analysts record data points at consistent intervals over a set period of time rather than just recording the data points intermittently or randomly. However, this type of analysis is not merely the act of collecting data over time. What sets time series data apart from other data is that the analysis can show how variables change over time. In other words, time is a crucial variable because it shows how the data adjusts over the course of the data points as well as the final results. It provides an additional source of information and a set order of dependencies between the data. Time series analysis typically requires a large number of data points to ensure consistency and reliability. An extensive data set ensures you have a representative sample size and that analysis can cut through noisy data. It also ensures that any trends or patterns discovered are not outliers and can account for seasonal variance. Additionally, time series data can be used for forecasting—predicting future data based on historical data. Time series analysis is used for non-stationary data—things that are constantly fluctuating over time or are affected by time. Industries like finance, retail, and economics frequently use time series analysis because currency and sales are always changing. Stock market analysis is an excellent example of time series analysis in action, especially with automated trading algorithms. Likewise, time series analysis is ideal for forecasting weather changes, helping meteorologists predict everything from tomorrow’s weather report to future years of climate change. Examples of time series analysis in action include: Weather data Rainfall measurements Temperature readings Heart rate monitoring (EKG) Brain monitoring (EEG) Quarterly sales Stock prices Automated stock trading Industry forecasts Interest rates Uses of Time Series The most important use of studying time series is that it helps us to predict the future behaviour of the variable based on past experience It is helpful for business planning as it helps in comparing the actual current performance with the expected one From time series, we get to study the past behaviour of the phenomenon or the variable under consideration We can compare the changes in the values of different variables at different times or places, etc. Components for Time Series Analysis The various reasons or the forces which affect the values of an observation in a time series are the components of a time series. The four categories of the components of time series are Trend Seasonal Variations Cyclic Variations Random or Irregular movements Seasonal and Cyclic Variations are the periodic changes or short-term fluctuations. Trend The trend shows the general tendency of the data to increase or decrease during a long period of time. A trend is a smooth, general, long-term, average tendency. It is not always necessary that the increase or decrease is in the same direction throughout the given period of time. It is observable that the tendencies may increase, decrease or are stable in different sections of time. But the overall trend must be upward, downward or stable. The population, agricultural production, items manufactured, number of births and deaths, number of industry or any factory, number of schools or colleges are some of its example showing some kind of tendencies of movement. Linear and Non-Linear Trend If we plot the time series values on a graph in accordance with time t. The pattern of the data clustering shows the type of trend. If the set of data cluster more or less round a straight line, then the trend is linear otherwise it is non-linear (Curvilinear). Seasonal Variations These are the rhythmic forces which operate in a regular and periodic manner over a span of less than a year. They have the same or almost the same pattern during a period of 12 months. This variation will be present in a time series if the data are recorded hourly, daily, weekly, quarterly, or monthly. These variations come into play either because of the natural forces or man-made conventions. The various seasons or climatic conditions play an important role in seasonal variations. Such as production of crops depends on seasons, the sale of umbrella and raincoats in the rainy season, and the sale of electric fans and A.C. shoots up in summer seasons. The effect of man-made conventions such as some festivals, customs, habits, fashions, and some occasions like marriage is easily noticeable. They recur themselves year after year. An upswing in a season should not be taken as an indicator of better business conditions. Cyclic Variations The variations in a time series which operate themselves over a span of more than one year are the cyclic variations. This oscillatory movement has a period of oscillation of more than a year. One complete period is a cycle. This cyclic movement is sometimes called the ‘Business Cycle’. It is a four-phase cycle comprising of the phases of prosperity, recession, depression, and recovery. The cyclic variation may be regular are not periodic. The upswings and the downswings in business depend upon the joint nature of the economic forces and the interaction between them. Random or Irregular Movements There is another factor which causes the variation in the variable under study. They are not regular variations and are purely random or irregular. These fluctuations are unforeseen, uncontrollable, unpredictable, and are erratic. These forces are earthquakes, wars, flood, famines, and any other disasters.