Classic Linear Regression Model CLRM PDF
Document Details
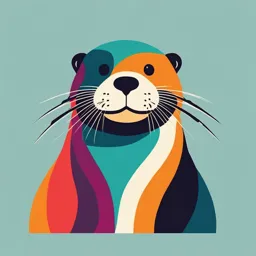
Uploaded by UsableChiasmus
Tags
Summary
This document provides a detailed explanation of the classical linear regression model (CLRM). It outlines the seven key assumptions (including linearity, uncorrelated explanatory variables, zero mean of the disturbance term, constant variance of error terms, no correlation between error terms, correct model specification and normal distribution of error terms) and the properties of Ordinary Least Squares (OLS) estimators. It also discusses the Gauss-Markov theorem. The content is suitable for an undergraduate economics or statistics course.
Full Transcript
The classical linear regression BT22203: Econometrics model (CLRM) The classical linear regression model Assumption 1: Regression model is linear in parameter Assumption 2: The explanatory variable is uncorrelated with the disturbance term and non-stochastic Assumption 3: The mean value of the...
The classical linear regression BT22203: Econometrics model (CLRM) The classical linear regression model Assumption 1: Regression model is linear in parameter Assumption 2: The explanatory variable is uncorrelated with the disturbance term and non-stochastic Assumption 3: The mean value of the disturbance term is zero The other factors or forces are not related to 𝑋𝑖 and therefore, given the value of 𝑋𝑖 , their mean value is zero. Assumption 4: The variance of each 𝑢𝑖 is constant or homoscedastic - The conditional distribution of each Y population corresponding to the given value of X has the same variance. - The individual Y values are spread around their mean values with the same variance Assumption 5: There is no correlation between two error terms - There is no systematic relationship between two error terms - Positive correlation: if one error term (𝑢) is above the mean value, another 𝑢 will also be above the mean value - Negative correlation: if one 𝑢 is below the mean value, another 𝑢 has to be above the mean value, or vice versa Assumption 6: The regression model is correctly specified. Alternatively, there is no specification bias or specification error in the model used in empirical analysis. WHY OLS? THE PROPERTIES OF OLS ESTIMATORS Gauss – Markov Theorem: Given the assumptions of the classical linear regression model, the OLS estimators have minimum variance in the class of linear estimators; that is, they are BLUE (best linear unbiased estimators) Property 1: 𝑏1 and 𝑏2 are linear estimators Property 2: They are unbiased; that is, 𝐸(𝑏1 ) = 𝐵1 and 𝐸(𝑏2 ) = 𝐵2. Property 3: that is, the OLS estimator of the error variance is unbiased. Property 4: 𝑏1 and 𝑏2 are efficient estimators; that is, var (𝑏1 ) and var (𝑏2 ) are less than the variance of any other linear unbiased estimator of 𝐵1 and 𝐵2. The sampling or probability distributions of OLS estimators Assumption 7: The error terms 𝑢𝑖 follows the normal distribution with mean zero and variance 𝜎 2 that is: Central limit theorem: If there is a large number of independent and identically distributed random variables, then, with a few exceptions, the distribution of their sum tends to be a normal distribution as the number of such variables increases indefinitely. 𝑏1 and 𝑏2 that are linear functions of the normally distributed variable 𝑢𝑖 prove that 𝑏1 and 𝑏2 themselves are normally distributed.