Statistics 1 PDF Study Guide 2024 - University of London
Document Details
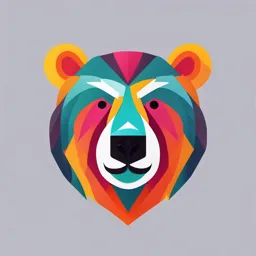
Uploaded by DesirousManticore5313
University of London
2024
James S. Abdey
Tags
Related
Summary
This Statistics 1 study guide, published by the University of London, covers key statistical concepts for undergraduate students in Economics, Management, Finance, and the Social Sciences. The guide, authored by James S. Abdey, includes topics such as data visualization, probability theory, hypothesis testing and sample questions. Also includes a PDF version.
Full Transcript
Undergraduate study in Economics, Management, Finance and the Social Sciences Statistics 1 J.S. Abdey ST104a 2024 Statistics 1 J.S. Abdey ST104a 2024 Undergraduate study in Economics, Management, Finance and the Social Sciences This subject guide is for a 100 course offered as part of the Uni...
Undergraduate study in Economics, Management, Finance and the Social Sciences Statistics 1 J.S. Abdey ST104a 2024 Statistics 1 J.S. Abdey ST104a 2024 Undergraduate study in Economics, Management, Finance and the Social Sciences This subject guide is for a 100 course offered as part of the University of London’s undergraduate study in Economics, Management, Finance and the Social Sciences. This is equivalent to Level 4 within the Framework for Higher Education Qualifications in England, Wales and Northern Ireland (FHEQ). For more information see: london.ac.uk This guide was prepared for the University of London by: James S. Abdey, BA (Hons), MSc, PGCertHE, PhD, Department of Statistics, London School of Economics and Political Science. This is one of a series of subject guides published by the University. We regret that due to pressure of work the author is unable to enter into any correspondence relating to, or arising from, the guide. If you have any comments on this subject guide, please communicate these through the discussion forum on the virtual learning environment. University of London Publications Office Stewart House 32 Russell Square London WC1B 5DN United Kingdom london.ac.uk Published by: University of London © University of London 2024 The University of London asserts copyright over all material in this subject guide except where otherwise indicated. All rights reserved. No part of this work may be reproduced in any form, or by any means, without permission in writing from the publisher. We make every effort to respect copyright. If you think we have inadvertently used your copyright material, please let us know. 4 Contents Contents 0 Preface 1 0.1 Route map to the subject guide....................... 1 0.2 Introduction to the subject area....................... 1 0.3 Syllabus.................................... 2 0.4 Aims and objectives............................. 2 0.5 Learning outcomes.............................. 2 0.6 Employability outcomes........................... 3 0.7 Overview of learning resources........................ 3 0.7.1 The subject guide........................... 3 0.7.2 Mathematical background...................... 4 0.7.3 Essential reading........................... 4 0.7.4 Further reading............................ 5 0.7.5 Online study resources........................ 5 0.7.6 The VLE............................... 6 0.7.7 Making use of the Online Library.................. 7 0.8 Examination advice.............................. 7 1 Mathematics primer and the role of statistics in the research process 9 1.1 Synopsis of chapter.............................. 9 1.2 Learning outcomes.............................. 9 1.3 Recommended reading............................ 9 1.4 Introduction.................................. 9 1.5 Arithmetic operations............................ 10 1.6 Squares and square roots........................... 11 1.7 Fractions and percentages.......................... 11 1.8 Some further notation............................ 12 1.8.1 Absolute value............................ 12 1.8.2Inequalities.............................. 12 P 1.9 Summation operator,........................... 13 1.10 Graphs..................................... 14 i Contents 1.11 The graph of a linear function........................ 15 1.12 The role of statistics in the research process................ 17 1.13 Overview of chapter.............................. 20 1.14 Key terms and concepts........................... 20 1.15 Sample examination questions........................ 20 1.16 Solutions to Sample examination questions................. 21 2 Data visualisation and descriptive statistics 23 2.1 Synopsis of chapter.............................. 23 2.2 Learning outcomes.............................. 23 2.3 Recommended reading............................ 23 2.4 Introduction.................................. 23 2.5 Types of variable............................... 24 2.5.1 Categorical variables......................... 25 2.6 Data visualisation............................... 26 2.6.1 Presentational traps......................... 26 2.6.2 Dot plot................................ 27 2.6.3 Histogram............................... 27 2.6.4 Stem-and-leaf diagram........................ 29 2.7 Measures of location............................. 31 2.7.1 Mean.................................. 31 2.7.2 Median................................ 32 2.7.3 Mode.................................. 34 2.8 Measures of dispersion............................ 35 2.8.1 Range................................. 35 2.8.2 Boxplot................................ 36 2.8.3 Variance and standard deviation................... 37 2.9 Test your understanding........................... 42 2.10 Overview of chapter.............................. 46 2.11 Key terms and concepts........................... 46 2.12 Sample examination questions........................ 46 2.13 Solutions to Sample examination questions................. 47 3 Probability theory 51 3.1 Synopsis of chapter.............................. 51 3.2 Learning outcomes.............................. 51 ii Contents 3.3 Recommeded reading............................. 51 3.4 Introduction.................................. 52 3.5 The concept of probability.......................... 52 3.6 Relative frequency.............................. 54 3.7 ‘Randomness’................................. 55 3.8 Properties of probability........................... 56 3.8.1 Notational vocabulary........................ 57 3.8.2 Venn diagrams............................ 57 3.8.3 The additive law........................... 58 3.8.4 The multiplicative law........................ 60 3.9 Conditional probability and Bayes’ formula................. 61 3.9.1 Bayes’ formula............................ 62 3.9.2 Total probability formula....................... 62 3.9.3 Independent events (revisited).................... 65 3.10 Probability trees............................... 65 3.11 Overview of chapter.............................. 66 3.12 Key terms and concepts........................... 67 3.13 Sample examination questions........................ 67 3.14 Solutions to Sample examination questions................. 68 4 Random variables, the normal and sampling distributions 69 4.1 Synopsis of chapter.............................. 69 4.2 Learning outcomes.............................. 69 4.3 Recommended reading............................ 69 4.4 Introduction.................................. 69 4.5 Discrete random variables.......................... 71 4.6 Continuous random variables........................ 72 4.7 Expectation of a discrete random variable................. 73 4.8 Functions of a random variable....................... 75 4.9 Variance of a discrete random variable................... 77 4.10 The normal distribution........................... 79 4.10.1 Standard normal statistical tables.................. 80 4.10.2 The general normal distribution................... 83 4.11 Sampling distributions............................ 83 4.12 Sampling distribution of X̄.......................... 85 4.13 Overview of chapter.............................. 88 iii Contents 4.14 Key terms and concepts........................... 88 4.15 Sample examination questions........................ 88 4.16 Solutions to Sample examination questions................. 89 5 Interval estimation 91 5.1 Synopsis of chapter.............................. 91 5.2 Learning outcomes.............................. 91 5.3 Recommended reading............................ 91 5.4 Introduction.................................. 91 5.4.1 Principle of confidence intervals................... 92 5.5 Interval estimation for a population mean................. 94 5.5.1 Variance known (σ 2 known)..................... 94 5.5.2 Variance unknown (σ 2 unknown).................. 95 5.5.3 Student’s t distribution........................ 96 5.5.4 Confidence interval for a single mean (σ 2 known)......... 98 5.5.5 Confidence interval for a single mean (σ 2 unknown)........ 99 5.6 Confidence interval for a single proportion................. 100 5.7 Sample size determination.......................... 101 5.8 Estimation of differences between parameters of two populations..... 103 5.9 Difference between two population means.................. 104 5.9.1 Unpaired samples: variances known................. 104 5.9.2 Unpaired samples: variances unknown and unequal........ 106 5.9.3 Unpaired samples: variances unknown and equal.......... 107 5.9.4 Paired (dependent) samples..................... 109 5.10 Difference between two population proportions............... 111 5.11 Overview of chapter.............................. 113 5.12 Key terms and concepts........................... 113 5.13 Sample examination questions........................ 114 5.14 Solutions to Sample examination questions................. 114 6 Hypothesis testing principles 117 6.1 Synopsis of chapter.............................. 117 6.2 Learning outcomes.............................. 117 6.3 Recommended reading............................ 117 6.4 Introduction.................................. 117 6.5 Types of error................................. 119 iv Contents 6.6 Significance level............................... 121 6.7 Critical values................................. 123 6.7.1 Rejection region for two-tailed tests................. 123 6.7.2 Rejection region for upper-tailed tests................ 124 6.7.3 Rejection region for lower-tailed tests................ 124 6.8 P -values.................................... 126 6.8.1 Interpretation of p-values....................... 129 6.8.2 Statistical significance versus practical significance......... 131 6.9 Overview of chapter.............................. 131 6.10 Key terms and concepts........................... 132 6.11 Sample examination questions........................ 132 6.12 Solutions to Sample examination questions................. 132 7 Hypothesis testing of means and proportions 135 7.1 Synopsis of chapter.............................. 135 7.2 Learning outcomes.............................. 135 7.3 Recommended reading............................ 135 7.4 Introduction.................................. 136 7.5 Testing a population mean claim...................... 137 7.6 Hypothesis test for a single mean (σ 2 known)............... 139 7.7 Hypothesis test for a single mean (σ 2 unknown).............. 140 7.8 Hypothesis test for a single proportion................... 143 7.9 Hypothesis testing of differences between parameters of two populations. 145 7.10 Difference between two population means.................. 146 7.10.1 Unpaired samples: variances known................. 146 7.10.2 Unpaired samples: variances unknown and unequal........ 147 7.10.3 Unpaired samples: variances unknown and equal.......... 149 7.10.4 Paired (dependent) samples..................... 151 7.11 Difference between two population proportions............... 153 7.12 Overview of chapter.............................. 155 7.13 Key terms and concepts........................... 156 7.14 Sample examination questions........................ 156 7.15 Solutions to Sample examination questions................. 157 8 Contingency tables and the chi-squared test 159 8.1 Synopsis of chapter.............................. 159 v Contents 8.2 Learning outcomes.............................. 159 8.3 Recommended reading............................ 159 8.4 Introduction.................................. 159 8.5 Association versus correlation........................ 160 8.6 Tests for association............................. 160 8.6.1 Contingency tables.......................... 161 8.6.2 Expected frequencies......................... 161 8.6.3 Test statistic............................. 162 8.6.4 The chi-squared, χ2 , distribution.................. 163 8.6.5 Degrees of freedom.......................... 163 8.6.6 Performing the test.......................... 164 8.7 Goodness-of-fit tests............................. 166 8.7.1 Observed and expected frequencies................. 166 8.7.2 The goodness-of-fit test........................ 167 8.8 Overview of chapter.............................. 169 8.9 Key terms and concepts........................... 169 8.10 Sample examination questions........................ 169 8.11 Solutions to Sample examination questions................. 170 9 Sampling and experimental design 173 9.1 Synopsis of chapter.............................. 173 9.2 Learning outcomes.............................. 173 9.3 Recommended reading............................ 173 9.4 Introduction.................................. 173 9.5 Motivation for sampling........................... 174 9.6 Types of sampling techniques........................ 175 9.6.1 Non-random sampling........................ 175 9.6.2 Random sampling........................... 178 9.7 Sources of error................................ 181 9.8 Non-response bias............................... 183 9.9 Method of contact.............................. 184 9.10 Experimental design............................. 187 9.11 Observational studies and designed experiments.............. 188 9.11.1 Observational study......................... 188 9.12 Longitudinal surveys............................. 189 9.13 Overview of chapter.............................. 190 vi Contents 9.14 Key terms and concepts........................... 190 9.15 Sample examination questions........................ 191 9.16 Solutions to Sample examination questions................. 192 10 Correlation and linear regression 195 10.1 Synopsis of chapter.............................. 195 10.2 Learning outcomes.............................. 195 10.3 Recommended reading............................ 195 10.4 Introduction.................................. 196 10.5 Scatter diagrams............................... 196 10.6 Causal and non-causal relationships..................... 198 10.7 Correlation coefficient............................ 199 10.7.1 Spearman rank correlation...................... 201 10.8 Linear regression............................... 203 10.8.1 The simple linear regression model................. 203 10.8.2 Parameter estimation......................... 204 10.8.3 Prediction............................... 205 10.8.4 Points to watch about linear regression............... 206 10.9 Overview of chapter.............................. 207 10.10 Key terms and concepts.......................... 208 10.11 Sample examination questions....................... 208 10.12 Solutions to Sample examination questions................ 209 A Mathematics primer and the role of statistics in the research process 211 A.1 Worked examples............................... 211 A.2 Practice problems............................... 215 A.3 Solutions to Practice problems........................ 216 B Data visualisation and descriptive statistics 221 B.1 Worked examples............................... 221 B.2 Practice problems............................... 233 B.3 Solutions to Practice problems........................ 235 C Probability theory 239 C.1 Worked examples............................... 239 C.2 Practice problems............................... 256 C.3 Solutions to Practice problems........................ 258 vii Contents D Random variables, the normal and sampling distributions 263 D.1 Worked examples............................... 263 D.2 Practice problems............................... 276 D.3 Solutions to Practice problems........................ 277 E Interval estimation 283 E.1 Worked examples............................... 283 E.2 Practice problems............................... 291 E.3 Solutions to Practice problems........................ 292 F Hypothesis testing principles 295 F.1 Worked examples............................... 295 F.2 Practice problems............................... 298 F.3 Solutions to Practice problems........................ 298 G Hypothesis testing of means and proportions 301 G.1 Worked examples............................... 301 G.2 Practice problems............................... 309 G.3 Solutions to practice problems........................ 310 H Contingency tables and the chi-squared test 313 H.1 Worked examples............................... 313 H.2 Practice problems............................... 318 H.3 Solutions to Practice problems........................ 320 I Sampling and experimental design 323 I.1 Worked examples............................... 323 I.2 Practice problems............................... 326 I.3 Solutions to Practice problems........................ 327 J Correlation and linear regression 333 J.1 Worked examples............................... 333 J.2 Practice problems............................... 342 J.3 Solutions to Practice problems........................ 343 K Examination formula sheet 345 viii Contents L Sample examination paper 347 M Sample examination paper – Solutions 353 ix Contents x Chapter 0 Preface 0.1 Route map to the subject guide This subject guide provides you with a framework for covering the syllabus of the ST104A Statistics 1 course and directs you to additional resources such as readings and the virtual learning environment (VLE). The material in this half course is necessary as preparation for other courses you may study later on as part of your degree. You may choose to take ST104B Statistics 2 so that you can study the concepts introduced here in greater depth. A natural continuation of this half course and ST104B Statistics 2 are the advanced half courses ST2133 Advanced statistics: distribution theory and ST2134 Advanced statistics: statistical inference. Two applied statistics courses for which this half course is a prerequisite are ST2187 Business analytics, applied modelling and prediction and ST3188 Statistical methods for market research. You may wish to develop your economic statistics by taking EC2020 Elements of econometrics, which requires ST104B Statistics 2 as well. The chapters are not a series of self-contained topics, rather they build on each other sequentially. As such, you are strongly advised to follow the subject guide in chapter order. There is little point in rushing past material which you have only partially understood in order to reach the final chapter. Once you have completed your work on all of the chapters, you will be ready for examination revision. A good place to start is the sample examination paper which you will find at the end of the subject guide. Colour has been included in places to emphasise important items. Formulae in the main body of chapters are in blue – these exclude formulae used in examples. Key terms and concepts when introduced are shown in magenta. References to other courses and half courses are shown in purple (such as above). Terms in italics are shown in purple for emphasis. References to chapters, sections, figures and tables are shown in teal. 0.2 Introduction to the subject area Welcome to the wonderful world of statistics! This discipline has unparalleled applicability in a wide range of areas such as finance, business, management, economics and other fields in the social sciences. ST104A Statistics 1 provides you with the opportunity to understand the fundamentals and gain the vital quantitative skills and powers of analysis that are highly sought-after by employers in many sectors. 1 0. Preface Statistics forms a core component of our programmes. All of the courses mentioned above require an understanding of the concepts and techniques introduced in this course. You will develop analytical skills on this course that will help you with your future studies and in the world of work. 0.3 Syllabus The up-to-date course syllabus for ST104A Statistics 1 can be found in the course information sheet, which is available on the course VLE page. 0.4 Aims and objectives The emphasis of this half course is on the application of statistical methods in management, economics and the social sciences. We will focus on the interpretation of tables and results, as well as the appropriate way to approach statistical problems. Note that this course is at an elementary mathematical level. We will introduce ideas of probability, inference and multivariate analysis which we will further develop in the half course ST104B Statistics 2. 0.5 Learning outcomes At the end of this half course, and having completed the essential reading and activities, you should: be familiar with the key ideas of statistics that are accessible to a student with a moderate mathematical competence be able to routinely apply a variety of methods for explaining, summarising and presenting data and interpreting results clearly using appropriate diagrams, titles and labels when required be able to summarise the ideas of randomness and variability, and the way in which these link to probability theory to allow the systematic and logical collection of statistical techniques of great practical importance in many applied areas have a grounding in probability theory and some grasp of the most common statistical methods be able to perform inference to test the significance of common measures such as means and proportions and conduct chi-squared tests of contingency tables be able to use correlation analysis and simple linear regression and know when it is appropriate to do so. 2 0.6. Employability outcomes 0.6 Employability outcomes Below are the three most relevant skill outcomes for students undertaking this course which can be conveyed to future prospective employers: 1. complex problem-solving 2. decision making 3. communication. 0.7 Overview of learning resources 0.7.1 The subject guide The subject guide is a self-contained resource, i.e. the content provided here is sufficient to prepare for the examination. We will discuss in detail all examinable topics with numerous worked examples and practice problems. It is essential to study extensively using the subject guide in order to perform well in the final examination. You do not need to buy a textbook, although you may want to read about the same topics written by different authors. See the suggested ‘Further reading’ below. The subject guide provides a range of activities that will enable you to test your understanding of the basic ideas and concepts. We want to encourage you to try the exercises that you encounter throughout the material before working through the solutions. With statistics, the motto is ‘practise, practise, practise...’. It is the best way to learn the material and prepare for examinations. The course is rigorous and demanding, but the skills you will be developing will be rewarding and well recognised by future employers. A suggested approach for studying ST104A Statistics 1 is to split the material into 10 weeks as follows: Week Chapter 1 Chapter 1: Mathematics primer and the role of statistics in the research process 2 Chapter 2: Data visualisation and descriptive statistics 3 Chapter 3: Probability theory 4 Chapter 4: Random variables, the normal and sampling distributions 5 Chapter 5: Interval estimation of means and proportions 6 Chapter 6: Hypothesis testing principles 7 Chapter 7: Hypothesis testing of means and proportions 8 Chapter 8: Contingency tables and the chi-squared test 9 Chapter 9: Sampling and experimental design 10 Chapter 10: Correlation and linear regression 3 0. Preface We recommend the following procedure: 1. Read the introductory comments. 2. Study the chapter content and practice problems. 3. Go through the learning outcomes carefully. 4. Refer back to this subject guide, or to supplementary texts, to improve your understanding until you are able to work through the problems confidently. The last step is the most important. It is easy to think that you have understood the material after reading it, but working through problems is the crucial test of understanding. Problem-solving should take up most of your study time. To prepare for the examination, you will only need to read the material in the subject guide, but it may be helpful from time to time to look at the suggested ‘Further reading’ below. 0.7.2 Mathematical background To study and understand statistics you will need to be familiar with some simple abstract mathematical concepts and apply common sense to see how to use these ideas in real-life applications. The concepts needed for probability and statistical inference are impossible to absorb by just reading them in a book – although you may find you need to do this more than once! You need to read, then think, then try some problems, then read and think some more. This process should be repeated until you find the problems easy to do. You will also need to use high-school arithmetic and understand some basic algebraic ideas. These ideas are very important. Starting with them should help you feel comfortable with this half course from the outset and they are therefore introduced to you in Chapter 1. Calculators A calculator may be used when answering questions on the examination paper for ST104A Statistics 1. It must comply in all respects with the specification given in the Regulations. You should also refer to the admission notice you will receive when entering the examination and the ‘Notice on permitted materials’. 0.7.3 Essential reading This subject guide is ‘self-contained’ meaning that this is the only resource which is essential reading for ST104A Statistics 1. Throughout the subject guide there are many worked examples and sample examination questions replicating resources typically provided in statistical textbooks. 4 0.7. Overview of learning resources Statistical tables In the examination you will be provided with relevant extracts of: Lindley, D.V. and W.F. Scott New Cambridge Statistical Tables. (Cambridge: Cambridge University Press, 1995) 2nd edition [ISBN 9780521484855]. The relevant extracts can be found at the end of this subject guide, and are the same as those distributed for use in the examination. It is advisable that you become familiar with them, rather than those at the end of a textbook which may differ in presentation. 0.7.4 Further reading As mentioned above, this subject guide is sufficient for study of ST104A Statistics 1. Of course, you are free to read around the subject area in any text, paper or online resource. You should support your learning by reading as widely as possible and by thinking about how these principles apply in the real world. To help you read extensively, you have free access to the virtual learning environment (VLE) and University of London Online Library (see below). Numerous titles are available covering the topics frequently covered in foundation statistics courses such as ST104A Statistics 1. Due to the inevitable heterogeneity among students taking this half course, some may find one author’s style easier to understand than another’s. That said, the recommended textbook for this course is: Abdey, J. Business Analytics: Applied Modelling and Prediction. (London: SAGE Publications, 2023) 1st edition [ISBN 9781529774092]. This textbook shows many real-world business applications of all statistical methods covered in ST104A Statistics 1. The textbook is also useful for ST2187 Business analytics, applied modelling and prediction and ST3188 Statistical methods for market research so if you study either of these courses you can benefit from a single textbook! 0.7.5 Online study resources In addition to the subject guide and the Essential reading, it is crucial that you take advantage of the study resources that are available online for this course, including the VLE and the Online Library. You can access the VLE, the Online Library and your University of London email account via the Student Portal at: http://my.london.ac.uk You should have received your login details for the Student Portal with your official offer, which was emailed to the address that you gave on your application form. You have probably already logged into the Student Portal in order to register! As soon as you registered, you will automatically have been granted access to the VLE, Online Library and your fully functional University of London email account. 5 0. Preface If you have forgotten these login details, please click on the ‘Forgot Password’ link on the login page. 0.7.6 The VLE The VLE, which complements this subject guide, has been designed to enhance your learning experience, providing additional support and a sense of community. It forms an important part of your study experience with the University of London and you should access it regularly. The VLE provides a range of resources for EMFSS courses: Course materials: Subject guides and other course materials available for download. In some courses, the content of the subject guide is transferred into the VLE and additional resources and activities are integrated with the text. Readings: Direct links, wherever possible, to essential readings in the Online Library, including journal articles and ebooks. Video content: Including introductions to courses and topics within courses, interviews, lessons and debates. Screencasts: Videos of PowerPoint presentations, animated podcasts and on-screen worked examples. External material: Links out to carefully selected third-party resources. Self-test activities: Multiple-choice, numerical and algebraic quizzes to check your understanding. Collaborative activities: Work with fellow students to build a body of knowledge. Discussion forums: A space where you can share your thoughts and questions with fellow students. Many forums will be supported by a ‘course moderator’, a subject expert employed by LSE to facilitate the discussion and clarify difficult topics. Past examination papers: We provide up to three years of past examinations alongside Examiners’ commentaries that provide guidance on how to approach the questions. Study skills: Expert advice on getting started with your studies, preparing for examinations and developing your digital literacy skills. Some of these resources are available for certain courses only, but we are expanding our provision all the time and you should check the VLE regularly for updates. 6 0.8. Examination advice 0.7.7 Making use of the Online Library The Online Library (https://onlinelibrary.london.ac.uk) contains a huge array of journal articles and other resources to help you read widely and extensively. To access the majority of resources via the Online Library you will either need to use your University of London Student Portal login details, or you will be required to register and use an Athens login. The easiest way to locate relevant content and journal articles in the Online Library is to use the Summon search engine. If you are having trouble finding an article listed in a reading list, try removing any punctuation from the title, such as single quotation marks, question marks and colons. For further advice, please use the online help pages (https://onlinelibrary.london.ac.uk/resources/summon) or contact the Online Library team using the ‘Chat with us’ function. 0.8 Examination advice Important: The information and advice given here are based on the examination structure used at the time this subject guide was written. Please note that subject guides may be used for several years. Because of this we strongly advise you to always check both the current Programme regulations for relevant information about the examination, and the VLE where you should be advised of any forthcoming changes. You should also carefully check the rubric/instructions on the paper you actually sit and follow those instructions. The examination is by a two-hour unseen question paper. No books may be taken into the examination, but the use of calculators is permitted, and statistical tables and a formula sheet are provided (the formula sheet can be found at the end of the subject guide). Section A, worth 50 marks, is compulsory with several short questions covering a wide range of the syllabus. In Section B two out of three longer questions must be answered, also worth 50 marks in total. You may use your calculator whenever you feel it is appropriate, always remembering that the examiners can give marks only for what appears on the examination script. Therefore, it is important to always show your working. In terms of the examination, as always, it is important to manage your time carefully and not to dwell on one question for too long – move on and focus on solving the easier questions, coming back to harder ones later. Remember, it is important to check the VLE for: up-to-date information on examination and assessment arrangements for this course where available, past examination papers and Examiners’ commentaries for the course which give advice on how each question might best be answered. 7 0. Preface 8 1 Chapter 1 Mathematics primer and the role of statistics in the research process 1.1 Synopsis of chapter This chapter outlines the essential mathematical building blocks which you will need to work with in this half course. Most of these will likely be revision to you, but some new material may be introduced. There is also a general introduction to some of the statistical ideas which you will be learning about in this half course. It should enable you to link different parts of the syllabus and see their relevance to each other and also to the other courses you are studying. 1.2 Learning outcomes By the end of this chapter, and having completed the recommended reading and activities, you should be able to: manipulate arithmetic and algebraic expressions using simple rules recall and use common signs: square, square root, ‘greater than’, ‘less than’ and absolute value demonstrate use of the summation operator and work with the ‘i’, or index, of x draw the straight line for a linear function explain the role of statistics in the research process. 1.3 Recommended reading Abdey, J. Business Analytics: Applied Modelling and Prediction. (London: SAGE Publications, 2023) first edition [ISBN 9781529774092] Chapter 1. 1.4 Introduction This opening chapter introduces some basic concepts and mathematical tools upon which the rest of the half course is built. Before proceeding to the rest of the subject 9 1. Mathematics primer and the role of statistics in the research process 1 guide, it is essential that you have a solid understanding of these fundamental concepts and tools. You should be a confident user of the basic mathematical operations (addition, subtraction, multiplication and division) and be able to use these operations on a calculator. The content of this chapter is expected to be a ‘refresher’ of the elementary algebraic and arithmetic rules from schooldays. Some material featured in this chapter may be new to you, such as the summation operator and graphs of linear functions. If so, you should master these new ideas before progressing. Finally, remember that although it is unlikely that an examination question would test you on the topics in this chapter alone, the material covered here may well be an important part of the answer! 1.5 Arithmetic operations We begin with elementary arithmetic operations which will be used when working with data in ST104A Statistics 1. Often students understand the statistical concepts but fail to manage a problem because they cannot do the required arithmetic. Although this is not primarily an arithmetic paper, many calculations will be used, so it is vital to ensure that you are comfortable with the examples and activities in this subject guide. The acronym to remember is BODMAS, which tells us the correct order (that is, the priority) in which mathematical operations are performed: Brackets Order (i.e. powers, square roots etc.) Division Multiplication Addition Subtraction. You should also know that: the sum of a and b means a + b the difference between a and b means either a − b or b − a the product of a and b means a × b = a · b the quotient of a and b means a divided by b, i.e. a/b. 10 1.6. Squares and square roots 1 Example 1.1 What is (35 ÷ 7 + 2) − (42 − 8 × 3)? BODMAS tells us to work out the brackets first. Here there are two sets of brackets, so let us deal with them one at a time. First bracket: 35 ÷ 7 + 2 do division first: 35 ÷ 7 + 2 = 5 + 2 then perform the addition: 5 + 2 = 7. Second bracket: 42 − 8 × 3 do order first: 42 − 8 × 3 = 16 − 8 × 3 next do multiplication: 16 − 8 × 3 = 16 − 24 then perform the subtraction: 16 − 24 = −8. Now the problem has been simplified we complete the calculation with the final subtraction: 7 − (−8) = 7 + 8 = 15. Note that the two negatives become positive! 1.6 Squares and square roots The power is the number of times a quantity is to be multiplied by itself. For example, 34 = 3 × 3 × 3 × 3 = 81. Any number raised to the power 2 is called ‘squared’, hence x2 is ‘x squared’, which is simply x × x. Remember that squared values, such as x2 , are always non-negative. This is important, for example, when we compute the quantity s2 in Chapter 2 which involves squared terms, so a negative answer should ring alarm bells telling us a mistake has been made! √ It might be helpful to √ of the square root of x (denoted by x) as the reverse of think √ √ such that x × x = x. Note that positive real numbers have two square the square, roots: ± 81 = ±9, although the positive square root will typically be used in ST104A Statistics 1. In practice, the main problems you will encounter involve taking square roots of numbers with decimal places. Be careful that you understand that 0.9 is the square root of 0.81, and that 0.3 is the square root of 0.09 (and not 0.9!). Of course, in the examination you can perform such calculations on your calculator, but it always helps to have an idea of what the answer should be as a feasibility check of your answer! 1.7 Fractions and percentages A fraction is part of a whole and can be expressed as either: a common fraction: for example, 1/2 or 3/8 a decimal fraction: for example, 0.50 or 0.375. In the common fraction, the top number is the numerator and the bottom number is the denominator. In practice, decimal fractions are more commonly used. 11 1. Mathematics primer and the role of statistics in the research process 1 When multiplying fractions together, just multiply all the numerators together to obtain the new numerator, and do the same with the denominators. For example: 4 1 2 4×1×2 8 × × = =. 9 3 5 9×3×5 135 Percentages give an alternative way of representing fractions by relating a particular quantity to the whole in parts per hundred. For example, 60% is 60 parts per 100, which, as a common fraction, is simply 60/100. 1.8 Some further notation 1.8.1 Absolute value One useful sign in statistics is | | which denotes the absolute value. This is the numerical value of a real number regardless of its sign (positive or negative). The absolute value of x, sometimes referred to as the modulus of x, or ‘mod x’, is |x|. So |7.1| = |−7.1| = 7.1. Statisticians sometimes want to indicate that they only want to use the positive value of a number. For example, let the distance between town X and town Y be 5 miles. Suppose someone walks from X to Y – a distance of 5 miles. A mathematician would write this as +5 miles. Later, after shopping, the person returns to X and the mathematician would record him as walking −5 miles (taking into account the direction of travel). Hence this way the mathematician can show the person ended up where they started. We, however, may be more interested in the fact that the person has had some exercise that day! So, we need notation to indicate this. The absolute value enables us to take only the positive values of our variables. The distance, d, from Y to X may well be expressed mathematically as −5 miles, but you will probably be interested in the absolute amount, so |−d| = d. 1.8.2 Inequalities An inequality is a mathematical statement that one quantity is greater or less than another: x > y means ‘x is greater than y’ x ≥ y means ‘x is greater than or equal to y’ x < y means ‘x is less than y’ x ≤ y means ‘x is less than or equal to y’ x ≈ y means ‘x is approximately equal to y’. 12 P 1.9. Summation operator, P 1 1.9 Summation operator, P The summation operator, , is likely to be new to many of you. It is widely used in statistics and you will come across it frequently in ST104A Statistics 1, so make sure you are comfortable using it before proceeding further! Statistics involves data analysis, so to use statistical methods we need data! Individual observations are typically represented using a subscript notation. For example, the heights of n people1 would be represented by x1 , x2 ,... , xn , where the subscript denotes the order in which the heights are observed (x1 represents the height of the first observed person, x2 the height of the second observed person etc.). Hence xi represents the height of the ith individual and, in order to list them all, the subscript i must take all integer values from 1 to n, inclusive. So, the whole set of observations is {xi : i = 1, 2,... , n} which can be read as ‘a set of observations xi such that i goes from 1 to n’. P Summation operator, The sum of a set of n observations, that is x1 + x2 + · · · + xn , may be written as: n X xi (1.1) i=1 P where is the summation operator, which can be read as ‘the sum of’. Therefore, Pn xi is read as ‘the sum of xi , for i equals 1 to n’. i=1 We see that the summation is said to be over i, where i is the index of summation and the range of i, in P (1.1), is from 1 to n. The lower bound of the range is the value of i written underneath , and the upper bound is written above it. Note that the lower bound can be any integer (positive, negative or zero), such that the summation is over all values of the index of summation in step increments of size one from the lower bound to the upper bound, inclusive. P As stated above, appears frequently in statistics. For example, in Chapter 2 you will meet descriptive statistics including the arithmetic mean of observations which is defined as: n 1X x̄ = xi. n i=1 n P Rather than write out xi in full, when all the xi s are summed we sometimes write i=1 n P P short-cuts, such as xi , or (when the range of summation is obvious) just xi. 1 Note that the resulting sum does not involve i in any form. Hence the sum is unaffected by (or invariant to) the choice of letter used for the index of summation. Hence, for 1 Throughout this half course, n will denote a sample size. 13 1. Mathematics primer and the role of statistics in the research process 1 example, the following summations are all equal: n X n X n X xi = xj = xk i=1 j=1 k=1 since each represents x1 + x2 + · · · + xn. Sometimes the way that xi depends on i is known. For example, if xi = i, we have: 3 X 3 X xi = i = 1 + 2 + 3 = 6. i=1 i=1 However, do not always assume that xi = i! Example 1.2 If {xi : i = 1, 2,... , n} is a set of observations, we might observe x1 = 4, x2 = 5, x3 = 1, x4 = −2 and x5 = 9. Therefore: 4 X x2i = 42 + 52 + 12 + (−2)2 = 46 i=1 and: 5 X 5 X xi (xi − 2) = (x2i − 2xi ) = ((−2)2 − 2 × −2) + (92 − 2 × 9) = 71 i=4 i=4 remembering to use BODMAS in the second example. 1.10 Graphs In Chapter 2 you will spend some time learning how to present data in graphical form, and also in the representation of the normal distribution in Chapter 4. You should make sure you have understood the following material. If you are taking MT105A Mathematics 1, you will need to use these ideas there as well. When a variable y depends on another variable x, we can represent the relationship mathematically using functions. In general we write this as y = f (x), where f is the rule which allows us to determine the value of y when we input the value of x. Graphs are diagrammatic representations of such relationships, using coordinates and axes. The graph of a function y = f (x) is the set of all points in the plane of the form (x, f (x)). Sketches of graphs can be very useful. To sketch a graph, we begin with the x-axis and y-axis as shown in Figure 1.1. We then plot all points of the form (x, f (x)). Therefore, at x units from the origin (the point where the axes cross), we plot a point whose height above the x-axis (that is, whose y coordinate) is f (x), as shown in Figure 1.2. Joining all points together of the form (x, f (x)) results in a curve (or sometimes a straight line), which is called the graph of f (x). A typical curve might look like that shown in Figure 1.3. 14 1.11. The graph of a linear function 1 Figure 1.1: Graph axes. Figure 1.2: Example of a plotted coordinate. However, you should not imagine that the correct way to sketch a graph is to plot a few points of the form (x, f (x)) and join them up – this approach rarely works well in practice and more sophisticated techniques are needed. There are two function types which you need to know about for this half course: linear functions (i.e. the graph of a straight line, see below) normal functions (which we shall meet frequently in later chapters). 1.11 The graph of a linear function Linear functions are those of the form: f (x) = a + bx and their graphs are straight lines which are characterised by a gradient (or slope), b, and a y-intercept (where x = 0) at the point (0, a). A sketch of the function y = 3 + 2x is provided in Figure 1.4, and the function y = 2 − x is shown in Figure 1.5. 15 1. Mathematics primer and the role of statistics in the research process 1 Figure 1.3: The graph of a generic function, y = f (x). y 3 -1.5 x Figure 1.4: A sketch of the linear function y = 3 + 2x. y 2 2 x Figure 1.5: A sketch of the linear function y = 2 − x. 16 1.12. The role of statistics in the research process 1 1.12 The role of statistics in the research process Before we get into details, let us begin with the ‘big picture’. First, some definitions. Research: trying to answer questions about the world in a systematic (scientific) way. Empirical research: doing research by first collecting relevant information (data) about the world. Research may be about almost any topic: physics, biology, medicine, economics, history, literature etc. Most of our examples will be from the social sciences: economics, management, finance, sociology, political science, psychology etc. Research in this sense is not just what universities do. Governments, businesses, and all of us as individuals do it too. Statistics is used in essentially the same way for all of these. Example 1.3 It all starts with a question. Can labour regulation hinder economic performance? Understanding the gender pay gap: what has competition got to do with it? Children and online risk: powerless victims or resourceful participants? Refugee protection as a collective action problem: is the European Union (EU) shirking its responsibilities? Do directors perform for pay? Heeding the push from below: how do social movements persuade the rich to listen to the poor? Does devolution lead to regional inequalities in welfare activity? The childhood origins of adult socio-economic disadvantage: do cohort and gender matter? Parental care as unpaid family labour: how do spouses share? Key stages of the empirical research process We can think of the empirical research process as having five key stages. 1. Formulating the research question. 2. Research design: deciding what kinds of data to collect, how and from where. 3. Collecting the data. 4. Analysis of the data to answer the research question. 5. Reporting the answer and how it was obtained. 17 1. Mathematics primer and the role of statistics in the research process 1 The main job of statistics is the analysis of data, although it also informs other stages of the research process. Statistics are used when the data are quantitative, i.e. in the form of numbers. Statistical analysis of quantitative data has the following features. It can cope with large volumes of data, in which case the first task is to provide an understandable summary of the data. This is the job of descriptive statistics. It can deal with situations where the observed data are regarded as only a part (a sample) from all the data which could have been obtained (the population). There is then uncertainty in the conclusions. Measuring this uncertainty is the job of statistical inference. We continue with an example of how statistics can be used to help answer a research question. Example 1.4 CCTV, crime and fear of crime. Our research question is what is the effect of closed-circuit television (CCTV) surveillance on: the number of recorded crimes? the fear of crime felt by individuals? We illustrate this using part of the following study. Gill, M. and A. Spriggs ‘Assessing the impact of CCTV’, Study 292, Home Office Research, 2005. The research design of the study comprised the following. Target area: a housing estate in northern England. Control area: a second, comparable housing estate. Intervention: CCTV cameras installed in the target area but not in the control area. Comparison of measures of crime and the fear of crime in the target and control areas in the 12 months before and 12 months after the intervention. The data and data collection were as follows. Level of crime: the number of crimes recorded by the police, in the 12 months before and 12 months after the intervention. Fear of crime: a survey of residents of the areas. Respondents: random samples of residents in each of the areas. 18 1.12. The role of statistics in the research process 1 In each area, one sample before the intervention date and one about 12 months after. Sample sizes: Before After Target area 172 168 Control area 215 242 Question considered here: ‘In general, how much, if at all, do you worry that you or other people in your household will be victims of crime?’ (from 1 = ‘all the time’ to 5 = ‘never’). Statistical analysis of the data. % of respondents who worry ‘sometimes’, ‘often’ or ‘all the time’: Target Control [a] [b] [c] [d] Confidence Before After Change Before After Change RES interval 26 23 −3 53 46 −7 0.98 (0.55, 1.74) It is possible to calculate various statistics, for example the Relative Effect Size RES = ([d]/[c])/([b]/[a]) = 0.98 is a summary measure which compares the changes in the two areas. RES < 1, which means that the observed change in the reported fear of crime has been a bit less good in the target area. However, there is uncertainty because of sampling: only 168 and 242 individuals were actually interviewed at each time in each area, respectively. The confidence interval for RES includes 1, which means that changes in the self-reported fear of crime in the two areas are ‘not statistically significantly different’ from each other. The number of (any kind of) recorded crimes: Target area Control area [a] [b] [c] [d] Confidence Before After Change Before After Change RES interval 112 101 −11 73 88 15 1.34 (0.79, 1.89) Now the RES > 1, which means that the observed change in the number of crimes has been worse in the control area than in the target area. However, the numbers of crimes in each area are fairly small, which means that these estimates of the changes in crime rates are fairly uncertain. The confidence interval for RES again includes 1, which means that the changes in crime rates in the two areas are not statistically significantly different from each other. In summary, this study did not support the claim that the introduction of CCTV reduces crime or the fear of crime. 19 1. Mathematics primer and the role of statistics in the research process 1 If you want to read more about research of this question, see Welsh, B.C. and D.P. Farrington ‘Effects of closed circuit television surveillance on crime’, Campbell Systematic Reviews 17 2008, pp. 1–73. Many of the statistical terms and concepts mentioned above have not been explained yet – that is what the rest of the course is for! However, it serves as an interesting example of how statistics can be employed in the social sciences to investigate research questions. 1.13 Overview of chapter Much of this material should be familiar to you, but some may be new. Although it is only a language or set of rules to help you deal with statistics, without it you will not be able to make sense of the following chapters. Before you continue, make sure you have completed all the worked examples in Appendix A, and understood what you have done. 1.14 Key terms and concepts Absolute value Numerator BODMAS Percentage Denominator Power Descriptive statistics Product Difference Quantitative Empirical Quotient Fraction Research Graph Square root Index of summation Statistical inference Inequality Sum Linear function Summation operator Modulus 1.15 Sample examination questions 1. Suppose that x1 = −0.2, x2 = 2.5, x3 = −3.7, x4 = 0.8, x5 = 7.4, and y1 = −0.2, y2 = 8.0, y3 = 3.9, y4 = −2.0, y5 = 0. Calculate the following quantities: 5 X (a) x2i i=3 2 X 1 (b) xy i=1 i i 5 X y2 i (c) y43 +. i=4 xi 20 1.16. Solutions to Sample examination questions 1 2. Suppose that y1 = −2, y2 = −5, y3 = 1, y4 = 16, y5 = 10, and z1 = 8, z2 = −5, z3 = 6, z4 = 4, z5 = 10. Calculate the following quantities: 3 X (a) zi2 i=1 5 X √ (b) yi zi i=4 3 X 1 (c) z42 +. y i=1 i 1.16 Solutions to Sample examination questions 1. (a) We have: 5 X x2i = (−3.7)2 + (0.8)2 + (7.4)2 = 13.69 + 0.64 + 54.76 = 69.09. i=3 (b) We have: 2 X 1 1 1 = + = 25 + 0.05 = 25.05. i=1 x i y i (−0.2) × (−0.2) 2.5 × 8.0 (c) We have: 5 y2 (−2.0)2 02 X i y43 + = (−2.0) + 3 + = −8 + 5 = −3. i=4 xi 0.8 7.4 2. (a) We have: 3 X zi2 = 82 + (−5)2 + 62 = 64 + 25 + 36 = 125. i=1 (b) We have: 5 X √ √ √ yi zi = 16 × 4 + 10 × 10 = 8 + 10 = 18. i=4 (c) We have: 3 X 1 1 1 z42 + 2 = 4 + − − + 1 = 16.3. y i=1 i 2 5 21 1. Mathematics primer and the role of statistics in the research process 1 22 Chapter 2 2 Data visualisation and descriptive statistics 2.1 Synopsis of chapter This chapter contains two separate but related themes, both to do with the understanding of data. First, we look at graphical representations for data which allow us to see their most important characteristics. Second, we calculate simple numbers, such as the mean or standard deviation, which will summarise those characteristics. In summary, you should be able to use appropriate diagrams and measures in order to explain and clarify data which you have collected or which are presented to you. 2.2 Learning outcomes After completing this chapter, and having completed the essential reading and activities, you should be able to: draw and interpret density histograms, stem-and-leaf diagrams and boxplots incorporate labels and titles correctly in your diagrams and state the units which you have used calculate the following: arithmetic mean, median, mode, standard deviation, variance, quartiles, range and interquartile range explain the use and limitations of the above quantities. 2.3 Recommended reading Abdey, J. Business Analytics: Applied Modelling and Prediction. (London: SAGE Publications, 2023) 1st edition [ISBN 9781529774092] Chapter 2. 2.4 Introduction Both themes considered in this chapter (data visualisation and descriptive statistics) could be applied to population data, but in most cases (namely here) they are applied to a sample. The notation would change slightly if a population was being represented. 23 2. Data visualisation and descriptive statistics Most visual representations are very tedious to construct in practice without the aid of a computer. However, you will understand much more if you try a few by hand (as is commonly asked in examinations). You should also be aware that spreadsheets do not 2 always use correct terminology when discussing and labelling graphs. It is important, once again, to go over this material slowly and make sure you have mastered the basic statistical definitions introduced here before you proceed to more theoretical ideas. 2.5 Types of variable Data1 are obtained on any desired variable. A variable is something which, well, varies! For quantitative variables, i.e. numerical variables, these can be classified into two types. Types of quantitative variable Discrete variables: These have outcomes you can count. Examples include the number of passengers on a flight and the number of telephone calls received each day in a call centre. Observed values for these will be 0, 1, 2,... (i.e. non-negative integers). Continuous variables: These have outcomes you can measure. Examples include height, weight and time, all of which can be measured to several decimal places, and typically have units of measurement (such as metres, kilograms and hours). Many of the problems for which people use statistics to help them understand and make decisions involve types of variables which can be measured. When we are dealing with a continuous variable – for which there is a generally recognised method of determining its value – we can also call it a measurable variable. The numbers which we then obtain come ready-equipped with an ordered relation, i.e. we can always tell if two measurements are equal (to the available accuracy) or if one is greater or less than the other. Of course, before we do any sort of data analysis, we need to collect data. Chapter 9 will discuss a range of different techniques which can be employed to obtain a sample. For now, we just consider some simple examples of situations where data might be collected, such as a: pre-election opinion poll asking 1,000 people about their voting intentions market research survey asking adults how many hours of television they watch per week census interviewer asking parents how many of their children are receiving full-time education (note that a census is the total enumeration of a population, hence this would not be a sample!). 1 Note that the word ‘data’ is plural, but is very often used as if it was singular. You will probably see both forms used when reading widely. 24 2.5. Types of variable 2.5.1 Categorical variables Qualitative data, often referred to as categorical variables, represent characteristics or qualities that can be divided into distinct groups or categories. Unlike quantitative data, 2 which recall are numerical, qualitative data is non-numeric and describes attributes or qualities. Categorical variables can take on different categories or groups, and they are often used to classify items into specific classes or labels based on shared characteristics. A polling organisation might be asked to determine whether, say, the political preferences of voters were in some way linked to their highest level of education – for example, do graduates tend to be supporters of Party XYZ? In consumer research, market research companies might be hired to determine whether users were satisfied with the service they obtained from a business (such as a restaurant) or a department of local or central government (housing departments being one important example). For qualitative variables, these can be classified into two types. Types of qualitative variable Nominal variables: These have categories with no inherent order or ranking. Examples include colours (such as red, blue, green etc.) and types of fruit (such as apple, banana, orange etc.). Ordinal variables: These have categories with a meaningful order or ranking but the intervals between them are not consistent. Examples include highest educational level achieved (such as high school, undergraduate, postgraduate) and degree classification (such as first class, upper second class, lower second class etc.). Example 2.1 Consider the following. (a) The total number of graduates (in a sample). (b) The total number of Party XYZ supporters (in a sample). (c) The number of graduates who support Party XYZ. (d) The number of Party XYZ supporters who are graduates. (e) Satisfaction levels of diners at a restaurant. In cases (a) and (b) we are doing simple counts, within a sample, of a single category – graduates and Party XYZ supporters, respectively – while in cases (c) and (d) we are looking at some kind of cross-tabulation between two categorical variables – a scenario which will be considered in Chapter 8. There is no obvious and generally recognised way of putting political preferences in order (in the way that we can certainly say that 1 < 2). It is similarly impossible to rank (as the technical term has it) many other categories of interest: in combatting discrimination against people, for instance, organisations might want to look at the effects of gender, religion, nationality, sexual orientation, disability etc. but the 25 2. Data visualisation and descriptive statistics whole point of combatting discrimination is that different levels of each category cannot be ranked. Hence these are examples of nominal variables. 2 In case (e), by contrast, there is a clear ranking: the restaurant would be pleased if there were lots of people who expressed themselves as being ‘very satisfied’, rather than merely ‘satisfied’, let alone ‘dissatisfied’ or ‘very dissatisfied’ ! Hence this is an ordinal variable. 2.6 Data visualisation Datasets consist of potentially vast amounts of data. Hedge funds, for example, have access to very large databases of historical price information on a range of financial assets, such as so-called ‘tick data’ – very high-frequency intra-day data. Of course, the human brain cannot easily make sense of such large quantities of numbers when presented with them on a screen. However, the human brain can cope with visual representations of data. By producing various plots, we can instantly ‘eyeball’ to get a bird’s-eye view of the dataset. So, at a glance, we can quickly get a feel for the data and determine whether there are any interesting features, relationships etc. which could then be examined in greater depth. In modelling, for example, we often make distributional assumptions, and a suitable variable plot allows us to easily check the feasibility of a particular distribution by eye. To summarise, plots are a great medium for communicating the salient features of a dataset to a wide audience. The main representations we use in ST104A Statistics 1 are histograms, stem-and-leaf diagrams and boxplots. We will also use scatterplots to visualise the relationship, if any, between two measurable variables (covered in Chapter 10). Note that there are many other representations available from software packages like Tableau, in particular pie charts and standard bar charts which are appropriate when dealing with categorical data, although these will not be considered further in this half course. If interested, you are recommended to study ST2187 Business analytics, applied modelling and prediction. 2.6.1 Presentational traps Before we see our first graphical representation you should be aware when reading articles in newspapers, magazines and even within academic journals, that it is easy to mislead the reader by careless or poorly-defined diagrams. As such, presenting data effectively with diagrams requires careful planning. A good diagram: provides a clear summary of the data is a fair and honest representation highlights underlying patterns allows the extraction of a lot of information quickly. 26 2.6. Data visualisation A bad diagram: confuses the viewer misleads (either accidentally or intentionally). 2 Advertisers and politicians are notorious for ‘spinning’ data to portray a particular narrative for their own objectives! 2.6.2 Dot plot The simplicity of a dot plot makes it an ideal starting point to think about the concept of a sample distribution. For small datasets, this type of plot is very effective for seeing the data’s underlying distribution. We use the following procedure. 1. Obtain the range of the dataset (the values spanned by the data), and draw a horizontal line to accommodate this range. 2. Place dots (hence the name ‘dot plot’ !) corresponding to the values above the line, resulting in the empirical distribution. Example 2.2 Hourly wage rates (in £) for clerical assistants: 12.20 11.50 11.80 11.60 12.10 11.80 11.60 11.70 11.50 11.60 11.90 11.70 11.60 12.10 11.70 11.80 11.90 12.00 11.50 11.60 11.70 11.80 11.90 12.00 12.10 12.20 Instantly, some interesting features emerge from the dot plot which are not immediately obvious from the raw data. For example, most clerical assistants earn less than £12 per hour and nobody (in the sample) earns more than £12.20 per hour. 2.6.3 Histogram Histograms are excellent diagrams to use when we want to visualise the frequency distribution of discrete or continuous variables. Our focus will be on how to construct a density histogram. Data are first organised into a table which arranges the data into class intervals (also called bins) – disjointed subdivisions of the total range of values which the variable takes. Let K denote the number of class intervals. These K class intervals should be mutually exclusive (meaning they do not overlap, such that each observation belongs to at most one class interval) and collectively exhaustive (meaning that each observation belongs to at least one class interval). 27 2. Data visualisation and descriptive statistics Recall that our objective is to represent the distribution of the data. As such, when choosing K, too many class intervals will dilute the distribution, while too few will concentrate it (using technical jargon, will tend to degenerate the distribution). Either 2 way, the pattern of the distribution will be lost – defeating the purpose of the histogram. As a guide, K = 6 or 7 should be sufficient, but remember to always exercise common sense! To each class interval, the corresponding frequency is determined, i.e. the number of observations of the variable which fall within each class interval. Let fk denote the frequency of class interval k, and let wk denote the width of class interval k, for k = 1, 2,... , K. PK The relative frequency of class interval k is rk = fk /n, where n = fk is the sample k=1 size, i.e. the sum of all the class interval frequencies. The density of class interval k is dk = rk /wk , and it is this density which is plotted on the y-axis (the vertical axis). It is preferable to construct density histograms only if each class interval has the same width. Example 2.3 Consider the weekly production output of a factory over a 50-week period (you can choose what the manufactured good is!). Note that this is a discrete variable since the output will take integer values, i.e. something which we can count. The data are (in ascending order for convenience): 350 354 354 358 358 359 360 360 362 362 363 364 365 365 365 368 371 372 372 379 381 382 383 385 392 393 395 396 396 398 402 404 406 410 420 437 438 441 444 445 450 451 453 454 456 458 459 460 467 469 We construct the following table, noting that a square bracket ‘[’ includes the class interval endpoint, while a round bracket ‘)’ excludes the class interval endpoint. Interval Relative Cumulative width, Frequency, frequency, Density, frequency, P Class interval wk fk rk = fk /n dk = rk /wk k fk [340, 360) 20 6 0.12 0.006 6 [360, 380) 20 14 0.28 0.014 20 [380, 400) 20 10 0.20 0.010 30 [400, 420) 20 4 0.08 0.004 34 [420, 440) 20 3 0.06 0.003 37 [440, 460) 20 10 0.20 0.010 40 [460, 480) 20 3 0.06 0.003 50 Note that here we have K = 7 class intervals each of width 20, i.e. wk = 20 for k = 1, 2,... , 7. From the raw data, check to see how each of the frequencies, fk , has been obtained. For example, f1 = 6 represents the first six observations (350, 354, 354, 358, 358 and 359). 28 2.6. Data visualisation We have n = 50, hence the relative frequencies are rk = fk /50 for k = 1, 2,... , 7. For example, r1 = f1 /n = 6/20 = 0.12. The density values can then be calculated. For example, d1 = r1 /w1 = 0.12/20 = 0.006. 2 The table above includes an additional column of ‘Cumulative frequency’, which is obtained by simply determining the running total of the class frequencies (for example, the cumulative frequency up to the second class interval is 6 + 14 = 20). Note the final column is not required to construct a density histogram, although the computation of cumulative frequencies may be useful when determining medians and quartiles (to be discussed later in this chapter). To construct the histogram, adjacent bars are drawn over the respective class intervals such that the histogram has a total area of one. The histogram for the above example is shown in Figure 2.1. Figure 2.1: Density histogram of weekly production output for Example 2.3. 2.6.4 Stem-and-leaf diagram A stem-and-leaf diagram uses the raw data. As the name suggests, it is formed using a ‘stem’ and corresponding ‘leaves’. The choice of the stem involves determining a major component of an observed value, such as the ‘10s’ unit if the order of magnitude of the observations were 15, 25, 35 etc., or if data are of the order of magnitude 1.5, 2.5, 3.5 etc. the integer part. The remainder of the observed value plays the role of the ‘leaf’. Applied to the weekly production dataset, we obtain the stem-and-leaf diagram shown below in Example 2.4. 29 2. Data visualisation and descriptive statistics Example 2.4 Continuing with Example 2.3, the stem-and-leaf diagram is: 2 Stem-and-leaf diagram of weekly production output Stem (Tens) Leaves (Units) 35 044889 36 0022345558 37 1229 38 1235 39 235668 40 246 41 0 42 0 43 78 44 145 45 0134689 46 079 Note the informative title and labels for the stems and leaves. For the stem-and-leaf diagram in Example 2.4, note the following points. These stems are formed of the ‘10s’ part of the observations. Leaves are vertically aligned, hence rotating the stem-and-leaf diagram 90 degrees anti-clockwise reproduces the shape of the data’s distribution, similar to what would be revealed with a density histogram. The leaves are placed in ascending order within the stems, so it is a good idea to sort the raw data into ascending order first of all (fortunately the raw data in Example 2.3 were already arranged in ascending order, but for other datasets this may not be the case). Unlike the histogram, the actual data values are preserved. This is advantageous if we want to calculate various descriptive statistics later on. So far we have considered how to summarise a dataset visually. This methodology is appropriate to get a visual feel for the distribution of the dataset. In practice, we would also like to summarise things numerically. There are two key properties of a dataset which will be of particular interest. Key properties of a dataset Measures of location – a central point about which the data tend (also known as measures of central tendency). Measures of dispersion – a measure of the variability of the data, i.e. how spread out the data are about the central point (also known as measures of spread). 30 2.7. Measures of location 2.7 Measures of location The mean, median and mode are the three principal measures of location. In general, 2 these will not all give the same numerical value for a given dataset/distribution.2 These three measures (and, later, measures of dispersion) will now be introduced using the following small sample dataset: 32, 28, 67, 39, 19, 48, 32, 44, 37 and 24. (2.1) 2.7.1 Mean The preferred measure of location/central tendency, which is simply the ‘average’ of the data. It will be frequently applied in various statistical inference techniques in later chapters. (Sample) mean P Using the summation operator, , which remember is just a form of ‘notational shorthand’, we define the sample mean, x̄, as: n 1X x1 + x2 + · · · + xn x̄ = xi =. n i=1 n To note, the notation x̄ will be used to denote an observed sample mean for a sample dataset, while µ will denote its population counterpart, i.e. the population mean. Example 2.5 For the dataset in (2.1) above: 10 1 X 32 + 28 + · · · + 24 370 x̄ = xi = = = 37. 10 i=1 10 10 Of course, it is possible to encounter datasets in frequency form, that is each data value is given with the corresponding frequency of observations for that value, fk , for k = 1, 2,... , K, where there are K different variable values. In such a situation, use the formula: K P fk xk k=1 x̄ = K. (2.2) P fk k=1 Note that this preserves the idea of ‘adding up all the observations and dividing by the total number of observations’. This is an example of a weighted mean, where the weights are the relative frequencies (as seen in the construction of density histograms). 2 These three measures can be the same in special cases, such as the normal distribution (introduced in Chapter 4) which is symmetric about the mean (and so mean = median) and achieves a maximum at this point, i.e. mean = median = mode. 31 2. Data visualisation and descriptive statistics If the data are given in grouped-frequency form, such as that shown in the table in Example 2.3, then the individual data values are unknown3 – all we know is the class interval in which each observation lies. The sensible solution is to use the midpoint of 2 the interval as a proxy for each observation recorded as belonging within that class interval. Hence you still use the grouped-frequency mean formula (2.2), but each xi value will be substituted with the appropriate class interval midpoint. Example 2.6 Using the weekly production data in Example 2.3, the interval midpoints are: 350, 370, 390, 410, 440, 450 and 470, respectively. These will act as the data values for the respective class intervals. The mean is then calculated as: K P 7 P f k xk f k xk k=1 k=1 (6 × 350) + (14 × 370) + · · · + (3 × 470) x̄ = = = = 400.4. PK P7 6 + 14 + · · · + 3 fk fk k=1 k=1 Compared to the true mean of the raw data (which is 399.72), we see that using the midpoints as proxies gives a mean very close to the true sample mean value. Note the mean is not rounded up or down since it is an arithmetic result. A drawback with the mean is its sensitivity to outliers, i.e. extreme observations. For example, suppose we record the net worth of 10 randomly chosen people. If Elon Musk (one of the world’s richest people at time of writing), say, was included, his substantial net worth would pull the mean upward considerably! By increasing the sample size n, the effect of his inclusion, although diluted, would still be non-negligible, assuming we were not just sampling from the population of billionaires! 2.7.2 Median The (sample) median, m, is the middle value of the ordered dataset, where observations are arranged in ascending order. By definition, 50 per cent of the observations are greater than or equal to the median, and 50 per cent are less than or equal to the median. (Sample) median Arrange the n numbers in ascending order, x(1) , x(2) ,... , x(n) , (known as the order statistics, such that x(1) is the first order statistic, i.e. the smallest observed value, and x(n) is the nth order statistic, i.e. the largest observed value), then the sample median, m, depends on whether the sample size is odd or even. If: n is odd, then there is an explicit middle value, so m = x((n+1)/2) n is even, then there is no explicit middle value, so take the average of the values either side of the ‘midpoint’, hence m = (x(n/2) + x(n/2+1) )/2. 3 Of course, we do have the raw data for the weekly production output and so we could work out the exact sample mean, but here suppose we did not have access to the raw data, instead we were just given the table of class interval frequencies as shown in Example 2.3. 32 2.7. Measures of location Example 2.7 For the dataset in (2.1), the ordered observations are: 19, 24, 28, 32, 32, 37, 39, 44, 48 and 67. 2 Here n = 10, i.e. there is an even number of observations, so we compute the average of the fifth and sixth ordered observations, that is: x(n/2) + x(n/2+1) x(5) + x(6) 32 + 37 m= = = = 34.5. 2 2 2 If we only had data in grouped-frequency form (as in Example 2.3), then we can make use of the cumulative frequencies. Since n = 50, the median is the 25.5th ordered observation which must lie in the [380, 400) class interval because once we exhaust the ordered data up to the [360, 380) class interval we have only accounted for the smallest 20 observations, while once the [380, 400) class interval is exhausted we have accounted for the smallest 30 observations, meaning the median must lie in this class interval. Assuming the raw data are not accessible, we could use the midpoint (i.e. 390) as denoting the median. Alternatively, we could use an interpolation method which uses the following ‘general’ formula for grouped data, once you have identified the class which includes the median (such as [380, 400) above): bin width × number of remaining observations endpoint of previous bin +. bin frequency Example 2.8 Returning to the weekly production output data from Example 2.3, the median would be: 20 × (25.5 − 20) 380 + = 391. 10 For comparison, using the raw data, x(25) = 392 and x(26) = 393, gives the ‘true’ sample median of 392.5. Although an advantage of the median is that it is not influenced by outliers (Elon Musk’s net worth would be x(n) and so would not affect the median), in practice it is of limited use in formal statistical inference. For symmetric data, the mean and median are always equal. Therefore, this is a simple way to verify whether a dataset is symmetric. Asymmetric distributions are skewed, where skewness measures the departure from symmetry. Although you will not be expected to compute the coefficient of skewness (its numerical value), you need to be familiar with the two types of skewness. Skewness When mean > median, this indicates a positively-skewed distribution (also, referred to as ‘right-skewed’). When mean < median, this indicates a negatively-skewed distribution (also, referred to as ‘left-skewed’).