L&M Concept Learning PDF
Document Details
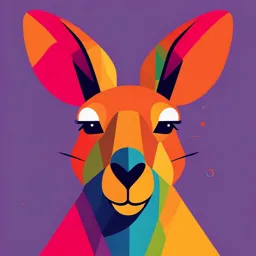
Uploaded by EnthusiasticErbium3720
L&M
Tags
Summary
This document provides notes on concept learning, specifically focusing on semantic memory, priming, and word recognition. It discusses how semantic knowledge is organized in hierarchical networks and how retrieval occurs via spreading activation.
Full Transcript
Concept Learning (Lecture 13 & 14) Semantic memory ○ Semantic knowledge allows us to predict, understand, and respond to new experiences ○ Semantic knowledge often arises from common elements across multiple (episodic) experiences ○ Scientists h...
Concept Learning (Lecture 13 & 14) Semantic memory ○ Semantic knowledge allows us to predict, understand, and respond to new experiences ○ Semantic knowledge often arises from common elements across multiple (episodic) experiences ○ Scientists have formal methods to organize knowledge about how the world works ○ How is semantic, declarative memory organized by the mind? Must support retrieval of facts we learned Must support reasoning about about relationships between those facts Must allow us to make inferences about new experiences Semantic networks and spreading activation ○ The amount of time to verify a sentence depends on distance in semantic network ○ Semantic knowledge is organized in hierarchical networks Distance between concepts (nodes) in the network represents their relatedness ○ Retrieval occurs via spreading activation through the network ○ Semantic (or category) fluency test: write down as many items from a category as possible within a short period of time Order of retrieval reflects semantic relatedness between items ○ Based on responses to semantic fluency, we can reconstruct how animal concepts are organized in the mind Size: how often people generated the animal Distance: how often two connected concepts (nodes) were generated one after another Priming in semantic memory ○ Priming: exposure to a stimulus influences the response to a subsequent stimulus, often in the absence of explicit awareness ○ Typical procedure: Present a prime stimulus (usually during unrelated task) The prime activates an internal representation in memory Activation spreads to other, related concepts in memory Activated representations affect behavior Recall: distributed representations ○ Mental representation of a visual object (like a face) involves layers of increasingly complex or abstract features as information flows through the visual system Word recognition ○ Words are objects composed of a fixed set of features (letters) ○ Comprehending text requires you to recognize words and retrieve their meaning from semantic memory ○ Words can be recognized relatively easy even if many features (letters) are in scrambled order ○ Interactive activation theory of word recognition Words are represented as combinations of letters, which are represented as combinations of line segments (features) Activations spreads through the network in both directions so that representations of words and letters interact Recognizing words in context ○ Word superiority effect: individual letters are recognized faster and more accurately when presented in the context of a real world According to Interactive Activation theory, activation at the word level spreads to the letter level, making retrieval faster Same effect doesn’t happen when letter is presented alone or in context of an unfamiliar nonsense word ○ Interactive activation allows us to fill in the gaps when input is ambiguous or incomplete (example of pattern of completion) Summary ○ Conceptual knowledge is organized in hierarchical associative networks ○ Retrieval of semantic memory occurs via spreading activation through those networks ○ According to interaction activation theory, representations of lower level features and higher level concepts interact ○ Word superiority effect: activation of word in memory facilitates detection of its features (individual level) ○ Activation of higher level concepts allows us to generalize in conditions where input is scrambled, distorted, or dissimilar to prototypical experiences Semantic memory ○ Episodic memory Organized by spatial and temporal context ○ Semantic memory De-contextualized Organized by meaning Generalizable knowledge ○ Concept learning and generalization Concept: a psychological representation of a category of objects, events, or people in the world Category learning: the process by which animals and human learn to classify stimuli into categories Generalization: the transfer of past learning to new situations and problems Predicting similar consequences based on perceived similarity to past experiences ○ Generalization gradient Pigeons trained to peck at yellow light to get reward, then tested on response to range of colors Generalization gradient: graph showing how physical change in stimuli correspond to changes in behavioral responses Not a result of confusing different stimuli or being unable to perceive differences Strength of response represents prediction that similar stimuli will lead to the same outcome ○ Generalization in associative networks What does generalization tell us about how different stimuli are represented in memory? If different stimuli are represented independently, no generalization would occur When the output node is activated, a response is generated An internal layer contains nodes that receive input from multiple, similar stimuli Input nodes are activated when corresponding stimulus is presented Distributed representation: a representation in which information is coded as a pattern of activation distributed across many different nodes To be able to generalize, we can’t treat every experience as a one-time, unique event, must be able to recognize shared elements across experiences Perceived similarity between different stimuli depends on whether they have overlapping representations in memory ○ Category learning Classical view of category learning: learn the set of features that define members of the category Categorization involves comparing a new object to the list of features necessary for category membership ○ Category learning: prototype theory For many natural concepts, there is no simple set of conditions to determine membership (contradicting the classical view) Category membership is graded, not all-or-nothing (we view some items to be better examples of a category than others) Family resemblance property: category members tend to have overlapping sets of features in common Prototype: the central tendency or idealized version of a concept or category According to the prototype theory of category learning, a learned prototype combines the most common features across experiences examples of the category Categorization of a new stimulus occurs by evaluating its similarity to the stored prototype Features can be physical or non-physical Typicality effect: objects that are more similar to the prototype are judges as better, or more typical, examples of the category (and are classified more quickly) Ambiguous, difficult to classify objects tend to be equidistant to prototypes of multiple categories Solso and McCarthy experiment Participants studied a set of faces for 10 seconds each Studied faces were all highly similar to a prototype face which was never shown After a delay, presented a new set of items that included the unseen prototype, the previously seen faces, and new, dissimilar faces Asked people whether they recognize each test face (and how confident they were) Participants confidently recognized the prototype face even though it had never been shown Among new (unstudied) faces, confidence was highest for faces with higher proportion of shared features ○ Configural representations in face recognition Inversion effect: loss of ability to recognize abnormal features when upside-down (changing the configuration) People can recognize faces from caricatures more quickly than from real photographs Caricatures emphasize configurations of features that are present in representation of the person (and unlikely in memories of other faces) Summary We use perceived similarity to generalize from existing conceptual knowledge to new events (generalization gradient) ○ Generalization provides evidence of distributed representations which allow us to recognize shared features According to prototype theory, memory for each concept is stored as a prototype that averages across experience ○ New objects/events are classified by comparing distances to stored prototypes Memory of a concept incorporates not only the set of features, but also how those features are arranged (configural representations) Discrimination learning Generalization: the transfer of past learning to new situations and problems ○ Predicting similar consequences based on perceived similarity to past experiences Discrimination learning: the process by which animals or people learn to respond to differently to different stimuli Discrimination learning is important for learning how natural categories should be further divided in meaningful ways (based on differences in experienced outcomes) Through experience, you may learn that category members are associated with different outcomes based on one feature dimension Pigeons were trained to peck to get reward based on frequency of a one Two conditions ○ Standard training: responding to a single tone is always reinforced ○ Discrimination training: responding to a 1000-Hz tone is always reinforced; responding to a 950-Hz tone is never reinforced After training, given a generalization test: play a wide range of frequencies and measure whether pigeon responds by pecking After discrimination training, pigeons learned to treat 950-Hz and 1000-Hz differently Discrimination training led to less generalization overall compared to standard training ○ Summary We use perceived similarity to generalize from existing conceptual knowledge to new events (generalization gradients) Generalization based on distributed representations in memory which allow us to recognize shared features But studies of discrimination learning show that generalization is not determined solely by perceived similarity Quickly adjust generalization gradients in response to differing outcomes Discrimination training tends to produce more narrow generalization gradients, in effect signaling that the world is more complex than it appears based on similarity alone