Introduction-to-Bioinformatics-Ontology.pptx
Document Details
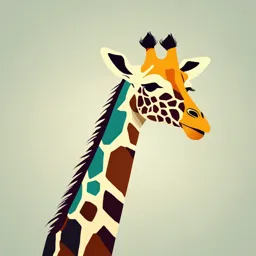
Uploaded by IntegratedFife
Tags
Related
- Computational Molecular Microbiology (MBIO 4700) Lecture Notes PDF
- Computational Molecular Microbiology (MBIO 4700) Lecture Notes PDF
- Computational Molecular Microbiology (MBIO 4700) PDF
- Bioinformatics Lecture Slides PDF
- Lecture 4 - Structural Bioinformatics PDF
- Bioinformatica e Oncologia Molecolare PDF
Full Transcript
Bioinformatics (Ontology) by Galal AL Marzoqi Agenda Introduction to Bioinformatics Ontology Importance of Ontologies in Bioinformatics Key Concepts and Terminology Ontology Development Methodologies Ontology Representation Languages Ontology Alig...
Bioinformatics (Ontology) by Galal AL Marzoqi Agenda Introduction to Bioinformatics Ontology Importance of Ontologies in Bioinformatics Key Concepts and Terminology Ontology Development Methodologies Ontology Representation Languages Ontology Alignment and Mapping Ontology-based Data Integration Challenges in Bioinformatics Ontology Development Future Trends and Directions Introduction to Bioinformatics Ontology Bioinformatics ontology is a critical tool for organizing and analyzing biological data. It provides a standardized framework for representing and understanding the relationships between biological entities. This framework is important for integrating and interpreting data from different sources, such as genome sequences, protein structures, and experimental results. Introduction to Bioinformatics Ontology Ontologies are hierarchical structures that define terms and their relationships. Each term represents a concept, and the relationships between terms specify how concepts are related. For example, a bioinformatics ontology power define the terms "gene," "protein," and "metabolic Importance of Ontologies in Bioinformatics Ontologies provide a standardized terminology for describing biological data, enabling consistent interpretation and analysis through different studies and databases. They enable data integration by providing a common framework for linking various datasets, improving our understanding of complex biological Importance of Ontologies in Bioinformatics Ontologies are important for For example, ontologies can be used to understanding the relationships identify genes that are difficult in a between different biological entities, specific disease, predict the effects of such as genes, proteins, pathways, mutations on protein function, or discover and diseases. new drug targets. By providing a hierarchical structure, In short, ontologies are a powerful tool ontologies allow us to reason about for forward our understanding of biology these relationships and based on and developing new solutions to Key Concepts and Terminology Bioinformatics ontology is a structured terminology that describes biological concepts and their relationships. It provides a common language for representing and integrating data across different sources and disciplines. Ontologies use a hierarchical structure, with more general concepts at the top and more specific concepts at the bottom. Key Concepts and Terminology Key concepts in bioinformatics ontology include classes, properties, and individuals. Classes represent categories of entities, such as genes, proteins, or diseases. Properties describe characteristics of entities, such as gene function or protein localization. Individuals are specific instances of classes, such as a particular gene or protein. Ontology Development Methodologies Knowledge Acquisition 1 This phase includes gathering domain knowledge. This can be done through interviews with experts, reviewing Formalization 2 existing literature, and analyzing data. The goal is to The knowledge acquired is then formalized into a create a complete and accurate representation of the structured representation. This includes defining domain. concepts, relationships, and properties. Domain experts play a central tical role in confirming the Ontology languages, such as OWL and RDF, provide tools ontology accurately reflects their knowledge. for representing this knowledge in a machine-readable format. Evaluation and Refinement 3 These formal languages ensure stability and enable Ontology is formalized, it needs to be evaluated and refined. computer-based reasoning. This includes testing. The ontology may also need to be adapted based on feedback from domain experts. This iterative process confirms the ontology remains related and useful over time. Ontology Representation Language Web Ontology Language (OWL) OWL is a standard language for representing ontologies on the Semantic Web. It provides a rich set of concepts for defining classes, properties, and relationships between entities. OWL suitable for various bioinformatics applications. Ontology Alignment and Mapping Ontology alignment and mapping are central tasks in bioinformatics. They include identifying concepts between different ontologies, enabling data integration and knowledge sharing through different sources. Structural Alignment 1 Identifies relationships between concepts based on their structure and organization within the ontology. Instance-based Alignment 2 Aligns concepts by examining their instances or examples, identifying commonalities based on observed data. Label-based Alignment 3 Compares concept labels or names, and semantic relationships to identify possible alignments. Ontology-based Data Integration Ontologies play a central role in integrating data from diverse biological databases. They provide a standardized vocabulary and hierarchical structure for representing biological concepts, helping joined data exchange and analysis across different By mapping data to ontologies, platforms. researchers can match data from different sources, enabling cross-database queries and comparisons. This approach helps the discovery of novel relationships and patterns of biomedical research. Challenges in Bioinformatics Ontology Development Developing complete and accurate ontologies for complex biological processes is a important challenge. There are many factors to consider, such as the diversity of biological data, the dynamic nature of biological systems, and the need for interoperability between different ontologies. Another challenge is maintaining and updating ontologies as new scientific discoveries are made and our understanding of biological systems evolves. Ontologies must be kept up to date to reflect the latest research and ensure that they are still relevant and useful. Ensuring that ontologies are widely adopted and used by the bioinformatics community is also crucial. This requires clear communication and collaboration among researchers, developers, and users of ontologies. Future Trends and Directions Bioinformatics ontology Furthermore, there is a growing highlighting on developing development is continuously ontologies for specific biological domains, such as cancer developing, driven by improvements in genomics and microbiome research. The data science, artificial intelligence, and development of standardized ontologies across different high-throughput technologies. research areas will help data sharing and interoperability, Future trends include the collaboration and scientific discovery. integration of ontologies with machine learning algorithms to enable data analysis and prediction.