Lecture 4 - Structural Bioinformatics PDF
Document Details
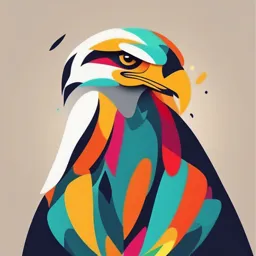
Uploaded by ConvincingOak
Imperial College London
Mike Sternberg
Tags
Summary
This document provides a lecture on structural bioinformatics, focusing on protein function and gene ontology. It details different types of homologous proteins, such as orthologs and paralogs, and approaches to predict protein function. The document also discusses various methods for identifying and analyzing protein structure.
Full Transcript
Lecture 4 – Structural Bioinforma0cs Protein Func1on Gene Ontology The enzyme classificaBon system is only enzyme funcBons, whereas gene ontology gives func3ons beyond enzymes. It is a controlled vocabulary that can be applied to all organisms, and can be used to describe gene products (proteins and...
Lecture 4 – Structural Bioinforma0cs Protein Func1on Gene Ontology The enzyme classificaBon system is only enzyme funcBons, whereas gene ontology gives func3ons beyond enzymes. It is a controlled vocabulary that can be applied to all organisms, and can be used to describe gene products (proteins and RNA) in any organism. All descripBons are supported by some level of evidence. It captures informaBon about 3 important features of funcBon: - What does the gene product do? – Molecular funcBon - Why does it perform these acBviBes? – Biological process - Where does it act? – Cellular component 3 Gene Ontologies - Molecular Func3on = biological funcBon - the tasks performed by individual gene products, for example, GTPase acBvity or carbohydrate binding. - goes from a general funcBon to a specific funcBon (there are different levels) - Biological Process = biological goal/objecBve (higher level funcBon) - broad biological goals, such as mitosis or purine metabolism - Cellular Component = acBve locaBon - subcellular structures, locaBons and macromolecular complexes, for example, nucleus, telomere and RNA polymerase II holoenzyme. Evidence Codes The annota3on source is important, it enables you to assess how confident the annotaBon is. GO associates annotaBons with an evidence code that indicates its score. Sources for AnnotaBons - Papers - Experiments - ComputaBonal analysis - Automated predicBons/analysis The first two are the most reliable as they have the most robust informaBon, and we would only use these as training data for an algorithm. Uses of GO Enhanced predictors of protein funcBon return predic3on of GO terms. Common features in a set of over- expressed genes can be reported as belonging to a common GO group. If you do RNA-seq, look if you have a set of highly expressed genes and whether they all belong to a similar GO term, this can give you can idea of related func3on or where the acBvity is. Clustering terms is oYen used to get a picture of an integrated view of protein funcBon. Func%on Predic%on There are about 240 million sequences, where the Bme taken experimentally to determine funcBon can be several years. Thus, to predict funcBon, we use methods that exploit protein homology and protein families. Approaches: General homology search - BLAST (single sequence informaBon) - PSIBLAST (mulBple sequence informaBon) - HMMs scan (mulBple sequence informaBon in HMM) - Pfam library Method: Query sequence + Database of sequences à Comparison algorithm (BLASTP, PSIBLAST, HMM) à list of similar protein sequences à infer homologues, similar structure and oYen similar funcBon. Types of Homologous Proteins Orthologs These are homologues created by specia3on, and typically have the same func3on in different species. You can transfer the func3on with a high degree of confidence, as they usually have almost idenBcal funcBons and the same 4 digits of EC if they are enzymes. Also, will have a closer sequence iden3ty than paralogs. Example: Human chymotrypsin and Bovine chymotrypsin – preferenBally cleave pepBdes aYer aromaBc Leu, have the same 4 digit EC code and have 85% sequence idenBty. Paralogues These are homologues created by gene duplica3on within a species from which they evolve independently, these typically have related funcBons. Example: Human trypsinogen / Human chymotrypsin, have different 4th digit of EC and only 38% idenBty. %ID and Func+onal Transfer To some extent these concepts about EC numbers apply to all types of funcBon but there are no clear-cut rules, and funcBon is challenging to quanBfy. Start with a query sequence and perform a BLASTP search: - If you find a protein from another species with >~50% iden3ty then it could easily be an orthologue with the same funcBon. - If you find a protein from another species with >~30% iden3ty then it could be a paralogue and have a related funcBon. - If you find a protein from the same species with >~30% idenBty then it could be a paralogue and have a related funcBon. These concepts are incorporated into soYware, where it is safe to transfer func3on between orthologues. Domains Another complicaBon of automaBc transfer is that proteins have domains. Homology-Based Predic>ons Search programs idenBfy local similari3es. Two sequences may share a region/domain of similarity but also other domains that are different. As not all domains are shared the funcBon the funcBon is probably different. This is the danger of automated predicBons based on homology, so you should do a full-length match and not just a local match you get from BLAST or PSIBLAST. Pfam is a domain-based approach and can circumvent this problem by searching specific domain libraries. Specific Domain Libraries Specific libraries with func3onal annota3on can be searched via InterPro at EBI. Avoid problem of inheri3ng func3on from a homologue where we only match one domain by looking at: - Prosite mo3fs (short pakerns good for enzyme acBve sites) - Profite profiles (more extensive profiles – e.g. a carrier protein will have a sequence pakern for the funcBon that extends across the sequence) - Pfam (HMMs of domains) However, all of this requires that the family has been added. It is generally easier to interpret than homology transfer, but there is less coverage. Advanced Sequence-Based Approaches Groups develop approaches based on sequence concepts but integraBng different sources of informa3on. One concept is to say that proteins that interact will have similar func3ons (i.e. use the interactome), an example of this is the STRING database. Other approaches integrate many sources of informaBon e.g. NetGo. String Database This idenBfies proteins that interact with a query protein. It uses different sources of informaBon with different reliability. It gets known interacBons from curated databases that have been experimentally determined. It also contains predicted interacBons from a variety of bioinformaBcs methods and text mining (finding in literature). NetGo2.0 This is a machine learning approach, where you start with the query sequence and it uses k-nearest neighbour using the BLAST result, InterPro features, frequency of amino acids and k-nearest neighbour of STRING. This integrates lots of sources of informa3on and did quite well. There is a blind test for funcBon predicBon known as CAFA, where they try to predict GO terms, and NetGo2.0 performed well. NetGo3.0 Added in a protein language model that replaces some of the earlier components, made very li[le difference – didn’t transform the accuracy. Structure-Based Approaches Match 3D structures (superpose them) and if there are similar func3onal residues this suggests related funcBon. This way you can find hits that you can’t idenBfy by sequence because they have such a remote rela3onship. Example: Integrase and Ribonuclease H, where their acBve sites match so we can transfer the funcBon. NOTE: Structure-based approaches enhance your confidence in funcBonal annotaBon. Structural Searching Take a structure, align it with a database of structures and you get a score. Use structural searching to idenBfy similar fold (DALI, CATH or Foldseek), as a similar 3D structure may suggest a related funcBon. If the func3onal site in the match is known, then examine if there are similar residues in your newly determined protein. This gives a higher degree of confidence of funcBonal transfer, even though you can never be certain. FoldSeek As structure predicBon methods are generaBng millions of publicly available protein structures, searching these databases is becoming a bo[leneck. Foldseek aligns the structure of a query protein against a database by describing terBary amino acid interacBons within proteins as sequences over a structural alphabet. Foldseek decreases computa3on 3mes by four to five orders of magnitude with 86%, 88% and 133% of the sensiBviBes of Dali, TM-align and CE, respecBvely. Convergence of Ac+ve Sites Cannot do this if you have convergent evolu3on. For example, even with gamma-chymotrypsin and sub3lisin, structure-based approaches looking at the global fold would not find this relaBonship. People have tried to search for the local 3D pa[erns, but it gives a lot of false posi3ves. The way you would tackle this would be via STRING, where you look at the interacBons, but it wouldn’t give you any specific data. Predic1on Non-Globular Regions Apart from the ends, the protein chain in the membrane is in a non-polar (hydrophobic) environment, thus, the sidechains tend to be hydrophobic. Main-chain cannot have NH and CO groups not forming hydrogen bonds – they do not like to be uncompensated for in a hydrophobic environment, so they form an alpha-helix. However, note that not all membrane bound proteins are formed of alpha-helical segments. Bacteriorhodopsin This was the first membrane protein revealed by Cryo-EM and is a classic example of a 7 TM GPCR, with 7 alpha helices. Porin This is a beta-barrel, and is the only other structure where you can sa3sfy all the H bonding other than alpha- helices. Although, they are found more in bacteria. Transmembrane Helices These span the hydrophobic secBon of the membrane about 35-50A wide. This secBon is not translocated (fixed) that abuts membrane oYen contains +ve charges. Each residue in an alpha helix advances the structure by 1.8A, and transmembrane helices tend to be between 20-30 residues long. Methods to Iden%fy TM Regions Hydrophobic Residue Search Early methods searched for runs of very non-polar (hydrophobic) residues along a sequence. Then use a scale of how hydrophobic each residue is using either the Hopp and Woods scale or the Kyte and Doolitle scale, calculated typically over a window of 11 residues. Hydrophobic Plots The red line is the threshold. Here there are two well predicted membrane spanning regions. Signal Pep+des Signal pepBdes refer to the sequence at the start of proteins ranging from 15-60 residues, that direct the protein to the correct cellular loca3on, these are typically cleaved off. They oYen have a hydrophobic region followed by a pakern typical of the cleavage site, however, in predicBons we need to try and dis3nguish transmembrane regions from signal pepBdes. DeepTMHMM UnBl recently, predicBon methods developed HMMs based on aligned sequences. The state of the art now is DeepTMHMM, which is a deep learning approach that predicts transmembrane structures and signal pepBdes. The algorithm predicts how the sequence maps onto the different potenBal states from the N- to the C- terminus, with a stepwise approach. Low Complexity Regions Regions with composiBon biased strongly to a small number of amino acids are very uninformaBve, but occur in the sequences of a significant number of proteins (oYen in disordered regions). They distort the staBsBcal significance scores of alignments, they pollute the PSSM and lead you into the wrong part of space. The program SEG is oYen used, where it replaces low complexity regions with lower case in BLAST searches at NCBI. Coiled-Coils These are two or three intertwined alpha helices and be a short segment or far longer. They are idenBfied using COILS. Disordered Proteins Some Globular proteins have small regions which are disordered and cannot be idenBfied with crystallography or NMR, oYen at the N- or C- terminus or a long loop. Other proteins do not adopt a single structural conformaBon but are instead highly flexible, this flexibility allows protein recogni3on. OYen, proteins become structured when a protein binds to another protein. Predic+on of Disorder Based on principles, they tend to have a lower fracBon of hydrophobic residues than the folded protein with a hydrophobic core. PredicBon is machine learning based, as most programs are now based on neural networks or support vector machines. Examples are PONDR-FIT and DISOPRED2. You can also use AlphaFold predicBon where the confidence given by pLDDT < 70%. fIDPnn Disorder Predic+on flDPnn produced the most accurate predic3ons of disorder (AUC = 0.814) and the fully disordered proteins (i.e., proteins for which disorder covers at least 95% of their sequences) in CAID. Moreover, flDPnn generates puta3ve func3ons for the predicted IDRs covering the four most commonly annotated funcBons, including protein-binding, DNA-binding, RNA-binding, and linkers. NOTE: AlphaFold pLDDT indicates disorder. DisProt - Database of Disordered Proteins DisProt is the gold standard database for intrinsically disordered proteins and regions, providing valuable informaBon about their func3ons. They show that DisProt's curated annotaBons strongly correlate with disorder predicBons inferred from AlphaFold2 pLDDT (predicted Local Distance Difference Test) confidence scores. Drug Discovery Pipeline Defini%ons Target A protein or molecule whose acBvity is modified to achieve therapeuBc effects. Hit A small molecule idenBfied through biological or computaBonal screening with the desired effect (typically IC₅₀ ≤ 1 µM). o Hits may come from commercially available compounds or custom chemical libraries. o Limita3on: If purchasable, the compound lacks novelty, meaning you cannot secure a ‘composi+on of maSe’r patent for it. Lead A chemically opBmized version of a hit, designed to: o Enhance therapeuBc efficacy. o Minimize adverse effects, including toxicity and poor absorpBon. Clinical Development Phases 1. Clinical Phase I o Purpose: Determine a safe dosage and assess side effects. o Par3cipants: 20–80 individuals (oYen healthy volunteers). o Method: Gradual dose escalaBon with close monitoring of absorpBon and adverse effects. 2. Clinical Phase II o Purpose: Refine Phase I results, focusing on side effects and iniBal effecBveness. o Par3cipants: 100–300 paBents (usually with the target condiBon). o Outcome: IdenBfies potenBal therapeuBc benefits. 3. Clinical Phase III o Purpose: DefiniBvely prove effecBveness and further evaluate safety. o Par3cipants: Thousands of paBents in large-scale trials. o Method: Randomized comparison with placebos or exisBng treatments. o Outcome: Provides the robust data needed for regulatory approval. Post-Clinical Stages Regulatory Phase o Submission of data to obtain approval for the drug’s use and markeBng. Sales and Monitoring Phase (Phase IV) o Purpose: Post-markeBng surveillance to monitor long-term safety and effecBveness. Patent Timing Challenge Risk of Early Paten3ng: The drug might fail clinical trials, leading to wasted resources. Risk of Late Paten3ng: CompeBtors may develop and release similar drugs, undercumng exclusivity. Computer-Based Drug Design There are three types of modelling: - You know the ac3vi3es of a set of ligands but do not know the structure of the receptor. - You know the structure of the receptor but not of the acBve ligands. - You know the structure of the complex of the receptor and the ligand(s). Ligand Screening Step 1 – Derive a QSAR QSAR = QuanBtaBve Structure AcBvity RelaBonship = A mathema3cal rela3onship relaBng acBvity to the structure of a molecule. Start with a set of ligands with known acBvity to derive a QSAR. Step 2 – Use the QSAR to score new molecules. Step 3 – Dock ligands into the receptor using virtual screening. Input: Structure of the target protein + Database of small molecules Dock into site and test the high scoring molecules. Output: Structure determinaBon + new inhibitor design. Docking Methods Tradi+onal Methods - Search by adjusBng the conformaBon of the ligand and the targets side chains (NOT its main chain). - Score using a simplified atom/atom score of energy calculaBons. o We don’t want precise Van der Waals interacBons as they tend to fail when they can’t model conformaBonal change. - Examples are AutoDock Vina and GLIDE. Advanced ML Methods - Some use ML to derive beker scoring func3ons – DeepDock. - Some use ML for searching and scoring – DiffDock o DiffDock uses diffusion models, it takes the known complexes, diffuse it with noise and then denoise it. Evalua%on of Ligand Docking – Redocking Incorrect Approach This involves using the X-ray structure of a protein-ligand complex to test if the docking algorithm can predict the correct pose of the ligand. Typically, the algorithm explores different conformaBons of the ligand while keeping the protein structure mostly rigid, allowing only side-chain adjustments. This "lock-and-key" approach is overly simplis3c and does not reflect biological reality, as proteins oYen undergo backbone conforma3onal changes when binding different ligands. Another issue, parBcularly prevalent in newer machine learning (ML) approaches, is the pracBce of training on one protein and tesBng on a homologous protein. This method does not consBtute de novo docking but instead exploits structural similarity, which undermines the evaluaBon of the model's true predicBve capability. Correct Approach In contrast, the correct approach begins by using the apo structure of the protein, which is the unbound state, to model the holo structure, the protein-ligand complex. The docking process should then evaluate how accurately the ligand can be docked into the binding site. This approach oYen requires the use of sooer poten3als to accommodate the flexibility of the protein. Importantly, homologous proteins should be excluded from both training and tesBng datasets to prevent arBficially high-performance metrics that do not reflect real-world docking challenges. A more refined strategy involves using a protein already known to bind one inhibitor and akempBng to dock a different molecule into the same site. This method beker approximates docking into an unbound state, providing a more realisBc assessment of the docking algorithm's predicBve accuracy. NOTE: When you see ligand docking programs, you must ask these quesBons about re-docking and homology. Addi3onal Note If you use AF2/3 to generate your protein structure, because it has learned both the bound and unbound structures, you tend to get a hybrid conforma3on. This means you don’t actually know if youre docking to the bound or the unbound form. This is an advantage of using template-based approaches like Phyre2.2. Sample Results from AlphaFold3 AF3 yields impressive results on docking. It was used to dock a clinical stage inhibitor to it ligand - AF3 achieves accurate predicBons but docking tools Vina and Gold do not. Discovery of Pfizer’s Nirmatrelvir In March 2020, the 3CL protease was idenBfied as a suitable target for SARs-CoV-2. Pfizer already had an inhouse viable drug against this enzyme from an earlier SARS outbreak. However, this drug has 5 hydrogen bond donors and so is very polar. If administered as a pill it would get trapped in the gut and so could only be used intravenously. The structure of the molecule was examined to see the parts that would bind to the receptor, and then removed the hydrogen bond donor that was not criBcal to this interacBon. This idenBfied Nirmatrelvir, with good an3viral acBvity and can be given orally to rats. They also decided to add ritonavir, which has no acBvity against SARS-CoV-2, but does bind to metabolising enzymes, thereby prevenBng nirmatrelvir from being broken down. The result was Paxlovid, which was approved by the FDA in July 2022. If given to Covid-19 paBents within 3 days of symptoms, then hospital admissions or death was 89% lower than placebo. Due to the emergency situaBon of covid, the drug discovery Bmeline of Paxlovid was 2.25 years compared to an average 13 years.