Chapter 10 Introduction to Simple Experiments PDF
Document Details
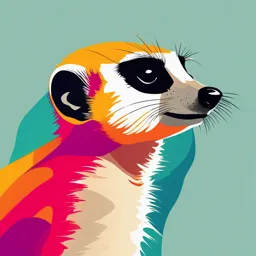
Uploaded by NonViolentVeena
Tags
Summary
This chapter introduces simple experiments in psychology, focusing on evaluating causal claims. It discusses independent and dependent variables, experimental design types, and potential threats to internal validity. Examining examples from studies on note-taking and infant persistence illustrates the application of these principles.
Full Transcript
PART V Tools for Evaluating Causal Claims A person’s hand writing on a notebook. There is a grid system superimposed on the image. 10 Introduction to Simple Experiments More Information A Learning Secret: Don’t Take Notes with a Laptop SCIENTIFIC AMERICAN, 2014 More Information ...
PART V Tools for Evaluating Causal Claims A person’s hand writing on a notebook. There is a grid system superimposed on the image. 10 Introduction to Simple Experiments More Information A Learning Secret: Don’t Take Notes with a Laptop SCIENTIFIC AMERICAN, 2014 More Information Infants Can Learn the Value of Perseverance by Watching Adults THE ATLANTIC, 2017 LEARNING OBJECTIVES A year from now, you should still be able to: 1. Apply the three criteria for establishing causation to experiments and explain why experiments can support causal claims. 2. Identify an experiment’s independent, dependent, and control variables. 3. Classify experiments as independent-groups and within-groups designs and explain why researchers might conduct each type of study. 4. Evaluate three potential threats to internal validity in an experiment— design confounds, selection effects, and order effects—and explain how experimenters usually avoid them. 5. Interrogate an experimental design using the four validities. A CAUSAL CLAIM IS the boldest kind of claim a scientist can make. A causal claim replaces verb phrases such as related to, is associated with, or linked to with powerful verbs such as makes, influences, or affects. Causal claims are special: When researchers make a causal claim, they are also stating something about interventions and treatments. The advice to not take notes with a laptop is based on a causal inference: Taking notes on a laptop causes something negative. Similarly, if babies are influenced by watching adults, those adults should think carefully about what behaviors they model. Interventions are often the ultimate goal of psychological studies, and they must be based on sound experimental research. Experiments are the only way to investigate such causal issues. TWO EXAMPLES OF SIMPLE EXPERIMENTS Let’s begin with two examples of experiments that supported valid causal claims. As you read about the two studies, consider how each one differs from the bivariate correlational studies in Chapter 8. What makes each of these studies an experiment? How does the experimental design allow the researchers to support a causal claim rather than an association claim? Example 1: Taking Notes Do you bring a pen to class for taking notes on what your professor is saying? Or do you open your laptop and type? If you’re like most students, you use the notetaking habit you think works for you. But should you trust your own experience? Maybe one way of taking notes is actually better than the other (Figure 10.1). More Information Figure 10.1 Take note. Which form of notetaking would lead to better learning? Researchers Pam Mueller and Daniel Oppenheimer (2014) decided to conduct an experiment that compared the two practices. When they considered the processes involved, both approaches seemed to have advantages. Typing is faster than longhand, they reasoned, so students can easily transcribe the exact words and phrases a professor is saying, resulting in seemingly more complete notes. However, students might not think about the material when they’re typing. When taking handwritten notes, in contrast, students can summarize, paraphrase, or make drawings to connect ideas—even if they record fewer words than they would on a computer. Longhand notes could result in deeper processing of the material and more effective comprehension. Which way would be better? Sixty-seven college students were recruited to come to a laboratory classroom, usually in pairs. The classroom was prepared in advance: Half the time it contained laptops; the other half, notebooks and pens. Having selected five TED talks on interesting topics, the researchers showed one of the lectures on a video screen. They told the students to take notes on the lectures using their assigned method (Mueller & Oppenheimer, 2014). After the lecture, students spent 30 minutes doing another activity meant to distract them from thinking about the lecture. Then they were tested on what they had learned from the TED talk. The essay questions asked about straightforward factual information (e.g., “Approximately how many years ago did the Indus civilization exist?”) as well as conceptual information (e.g., “How do Japan and Sweden differ in their approaches to equality in their societies?”). Their answers were scored by a research assistant who did not know which form of notetaking each participant had used. The results Mueller and Oppenheimer obtained are shown in Figure 10.2. Students in both the laptop and the longhand groups scored about equally on the factual questions, but the longhand group scored higher on the conceptual questions. More Information Figure 10.2 The effect of laptop and longhand notetaking on test performance. In this study, performance on factual questions was the same in the laptop and longhand groups, but performance on conceptual questions was better for those who took handwritten notes. The error bars represent standard error of each mean. (Source: Adapted from Mueller & Oppenheimer, 2014.) Mueller and Oppenheimer didn’t stop at just one study. They wanted to demonstrate that the original result could happen again. Their journal article reports two other studies, each of which compared longhand to laptop notetaking, and each of which showed the same effect: The longhand group performed better on conceptual test questions. (The two other studies, unlike the first, showed that longhand notetakers did better on factual questions, too.) The authors made a causal claim: Taking notes in longhand causes students to better understand what they hear. Do you think their study supports the causal claim? Example 2: Motivating Babies In an article with this headline—“Infants can learn the value of perseverance by watching adults”—journalist Ed Yong (2017) summarized a series of studies on how watching adult models can motivate babies to persist at difficult tasks. What were the studies behind this science writer’s story? The studies took place at a play lab at the Boston Children’s Museum. The researchers (Leonard et al., 2017) recruited more than 100 babies, aged 13 to 18 months, to participate. Parents sat next to their babies during the study but were asked not to help. Behind the scenes, the researchers had flipped a coin to assign half of the babies to an “effort” condition and half to a “no-effort” condition. In the effort condition, the babies watched a model try to get a toy frog out of a plastic box. The model kept repeating, “How do I get this out?” After trying several ways, she finally opened the box’s secret door, saying, “I got it out!” Then the model tried to unhook a toy from a carabiner, saying, “How do I get this off?” After several tries, she finally released the toy and said, “Yay!” In the no-effort condition, the model worked with the same toys for the same amount of time. But in this condition, she simply took the toy frog out of the box three times in a row and easily took the toy off the carabiner three times. After modeling effort or no effort, the model handed the baby a cube- shaped toy that played music (see Figure 10.3). The toy had a large white button that looked like it should start the music, but it was actually inert. The researchers recorded how long the babies spent playing with the toy. How many times would the babies try the inert button? More Information Figure 10.3 Measuring persistence. A baby tries to get the toy to play music in the persistence study. The researchers measured how many times the baby pressed the large, inert button on the toy. The results of the study are depicted in Figure 10.4. The researchers found that babies in the effort condition pressed the inert button about 11 times more than babies in the no-effort condition. The researchers wrote, “Seeing just two examples of an adult working hard to achieve her goals can lead infants to work harder at a novel task relative to infants who see an adult succeed effortlessly” (Leonard et al., 2017, p. 357). What do you think: Do the results of this study support the researcher’s causal claim? More Information Figure 10.4 The results of Leonard et al.’s study on persistence in babies. The error bars represent standard error of each mean. (Source: Adapted from Leonard et al., 2017.) EXPERIMENTAL VARIABLES The word experiment is common in everyday use. Colloquially, “to experiment” means to try something out. A cook might say they experimented with a recipe by replacing the eggs with applesauce. A friend might say they experimented with a different driving route to the beach. In psychological science, however, the term experiment specifically means that the researchers manipulated at least one variable and measured another (as you learned in Chapter 3). Experiments can take place in a laboratory, a school, or just about anywhere a researcher can manipulate one variable and measure another. A manipulated variable is a variable that is controlled, such as when the researchers assign participants to a particular level (value) of the variable. For example, Mueller and Oppenheimer (2014) manipulated notetaking by flipping a coin to determine whether a person would take notes with a laptop or in longhand. (In other words, the participants did not get to choose which form they would use.) Notetaking method was a variable because it had more than one level (laptop and longhand), and it was a manipulated variable because the experimenter assigned each participant to a particular level. The Leonard team (2017) similarly manipulated which model the babies watched by flipping a coin ahead of time to decide which session participants were in. (Parents did not choose which type of model their babies would see.) Measured variables take the form of records of behavior or attitudes, such as self-reports, behavioral observations, or physiological measures (see Chapter 5). After an experimental situation is set up, the researchers simply record what happens. In their first study, Mueller and Oppenheimer measured student performance on the essay questions. After manipulating the notetaking method, they watched and recorded—that is, they measured —how well people answered the factual and conceptual questions. The Leonard team manipulated the adult model’s effort behavior and then measured how many times each baby pressed the inert button. Independent and Dependent Variables In an experiment, the manipulated (causal) variable is the independent variable. The name comes from the fact that the researcher has some “independence” in assigning people to different levels of this variable. A study’s independent variable should not be confused with its levels, which are also referred to as conditions. The independent variable in the Leonard study was the adult model’s effort behavior, which had two conditions: effort and no effort. The measured variable is the dependent variable, or outcome variable. How a participant acts on the measured variable depends on the level of the independent variable. Researchers have less control over the dependent variable; they manipulate the independent variable and then watch what happens to people’s self-reports, behaviors, or physiological responses. A dependent variable is not the same as its levels, either. The dependent variable in the Leonard study was the number of button presses (not “25 presses”). Experiments must have at least one independent variable and one dependent variable, but they often have more than one dependent variable. For example, the notetaking study had two dependent variables: performance on factual questions and performance on conceptual questions. Similarly, the baby persistence study’s main dependent variable was the number of button presses, but the researchers also measured how long each baby played with the toy during a 2-minute interval. When the dependent variables are measured on different scales (e.g., button presses and seconds), they are usually presented on separate graphs. (Experiments can also have more than one independent variable; Chapter 12 introduces this type of experiment.) Here’s a way to tell the two kinds of variables apart. When researchers graph their results, the independent variable is almost always on the x- axis, and the dependent variable is almost always on the y-axis (see Figures 10.2 and 10.4 for examples). A mnemonic for remembering the two types of variables is that the independent variable comes first in time (and the letter I looks like the number 1), and the dependent variable is measured afterward (or second). Control Variables When researchers are manipulating an independent variable, they need to make sure they are varying only one thing at a time—the potential causal force or proposed “active ingredient” (e.g., only the form of notetaking, or only the amount of effort the adult model displays). Therefore, besides the independent variable, researchers also control potential third variables (or nuisance variables) in their studies by holding all other factors constant between the levels of the independent variable. For example, Mueller and Oppenheimer (2014) manipulated the method people used to take notes, but they held constant several other potential variables: People in both groups watched lectures in the same room and had the same experimenter. They watched the same videos and answered the same questions about them, and so on. Any variable that an experimenter holds constant on purpose is called a control variable. In the Leonard et al. study (2017), one control variable was the toys the model was using. In both conditions, she modeled the same frog-in-the- box and carabiner toys. She used the same cheerful, enthusiastic voice. The researchers also kept constant how long the model demonstrated each toy (30 seconds each), the gender of the model (always female), the chair the infant sat in, the cubicle where the experiment took place, and so on. Control variables are not really variables at all because they do not vary; experimenters keep the levels the same for all participants. Clearly, control variables are essential in experiments. They allow researchers to separate one potential cause from another and thus eliminate alternative explanations for results. Control variables are therefore important for establishing internal validity. CHECK YOUR UNDERSTANDING 1. What are the minimum requirements for a study to be an experiment? 2. Define independent variable, dependent variable, and control variable, using your own words. 1. A manipulated variable and a measured variable; see p. 281. 2. See pp. 281–282. Glossary experiment A study in which at least one variable is manipulated and another is measured. manipulated variable A variable in an experiment that a researcher controls, such as by assigning participants to its different levels (values). See also measured variable. measured variable A variable in a study whose levels (values) are observed and recorded. See also manipulated variable. independent variable In an experiment, a variable that is manipulated. In a multiple- regression analysis, a predictor variable used to explain variance in the criterion variable. See also dependent variable. condition One of the levels of the independent variable in an experiment. dependent variable In an experiment, the variable that is measured. In a multiple- regression analysis, the single outcome, or criterion variable the researchers are most interested in understanding or predicting. Also called outcome variable. See also independent variable. control variable In an experiment, a variable that a researcher holds constant on purpose. WHY EXPERIMENTS SUPPORT CAUSAL CLAIMS In both of the examples above, the researchers manipulated one variable and measured another, so both studies can be considered experiments. But are these researchers really justified in making causal claims on the basis of these experiments? Yes. To understand how experiments support causal claims, you can first apply the three rules for causation to the baby persistence study. The three rules should be familiar to you by now: 1. Covariance. Do the results show that the causal variable is related to the outcome variable? Are distinct levels of the independent variable associated with different levels of the dependent variable? 2. Temporal precedence. Does the study design ensure that the causal variable comes before the outcome variable in time? 3. Internal validity. Does the study design rule out alternative explanations for the results? Experiments Establish Covariance The results of the experiment by Leonard and her colleagues did show covariance between the causal variable (the independent variable: model’s behavior) and the outcome variable (the dependent variable: button presses). On average, babies who saw the “effort” model pressed the button 11 times more often than babies who saw the “no-effort” model (see Figure 10.4). In this case, covariance is indicated by a difference in the group means: The number of button presses was different in the effort condition than it was in the no-effort condition. The notetaking study’s results also showed covariance, at least for conceptual questions: Longhand notetakers had higher scores than laptop notetakers on conceptual questions. INDEPENDENT VARIABLES ANSWER “COMPARED TO WHAT?” The covariance criterion might seem obvious. In our everyday reasoning, though, we tend to ignore its importance because most of our personal experiences do not have the benefit of a comparison group, or comparison condition. For instance, you might have wondered if your painstaking, handwritten notes are making you learn more, but without comparing longhand with laptop notetaking for the same class session, you cannot know for sure. An experiment, in contrast, provides the comparison group you need. Therefore, an experiment is a better source of information than your own experience because an experiment allows you to ask and answer: Compared to what? (For a review of experience versus empiricism, see Chapter 2.) If independent variables did not vary, a study could not establish covariance. For example, a few years ago, a psychology blogger described a study he had conducted informally, concluding that dogs don’t like being hugged (Coren, 2016). The press widely covered the conclusion, but the study behind it was flawed. Having collected Internet photos of people hugging their dogs, the researcher reported that 82% of the hugged dogs showed signs of stress. However, this study did not have a comparison group: Coren did not collect photos of dogs not being hugged. Therefore, we cannot know, based on this study, if signs of stress are actually higher in hugged dogs than not-hugged dogs. In contrast, true experiments manipulate an independent variable. Because every independent variable has at least two levels, true experiments are always set up to look for covariance. COVARIANCE: IT’S ALSO ABOUT THE RESULTS Manipulating the independent (causal) variable is necessary for establishing covariance, but the results matter, too. Suppose the baby researchers had found no difference in how babies behaved in the two conditions. In that case, the study would have found no covariance, and the experimenters would have had to conclude that persistent adult models do not cause babies to persist more. After all, if button presses did not covary with the effort/no-effort conditions, there is no causal impact to explain. CONTROL GROUPS, TREATMENT GROUPS, AND COMPARISON GROUPS There are a couple of ways an independent variable might be designed to show covariance. Your early science classes may have emphasized the importance of a control group in an experiment. A control group is a level of an independent variable that is intended to represent “no treatment” or a neutral condition. When a study has a control group, the other level or levels of the independent variable are usually called the treatment group(s). For example, if an experiment is testing the effectiveness of a new medication, the researchers might assign some participants to take the medication (the treatment group) and other participants to take an inert sugar pill (the control group). When the control group is exposed to an inert treatment such as a sugar pill, it is called a placebo group, or a placebo control group. ❮❮ For more details on the placebo effect and how researchers control for it, see Chapter 11, pp. 335–337. Not every experiment has—or needs—a control group, and often, a clear control group does not even exist. The Mueller and Oppenheimer notetaking study (2014) had two comparison groups—laptop and longhand —but neither was a control group, in the sense that neither of them clearly established a “no notetaking” condition. Also consider the experiment by Harry Harlow (1958), discussed in Chapter 1, in which baby monkeys were put in cages with artificial “mothers” made of either cold wire or warm cloth. There was no control group, just a carefully designed comparison condition. When a study uses comparison groups, the levels of the independent variable differ in some intended and meaningful way. All experiments need a comparison group so the researchers can compare one condition to another, but the comparison group may not need to be a control group. Experiments Establish Temporal Precedence The experiment by Leonard’s team also established temporal precedence. The experimenters manipulated the causal (independent) variable (adult model’s effort behavior) to ensure that it came first in time. Then the babies took the musical cube and pressed its button. The causal variable clearly did come before the outcome (dependent) variable. This ability to establish temporal precedence, by controlling which variable comes first, is a strong advantage of experimental designs. By manipulating the independent variable, the experimenter virtually ensures that the cause comes before the effect (or outcome). The ability to establish temporal precedence is a feature that makes experiments superior to correlational designs. A simple correlational study is a snapshot—all variables are measured at the same time, so when two variables covary (such as time spent sitting and measured cortical thickness, or deep conversations and well-being), it’s impossible to tell which variable came first. In contrast, experiments unfold over time, and the experimenter makes sure the independent variable comes first. Well-Designed Experiments Establish Internal Validity Did the Mueller and Oppenheimer study establish internal validity? Are there any alternative explanations for why students in the longhand condition scored better on conceptual tests than students in the laptop condition? A well-designed experiment establishes internal validity, which is one of the most important validities to interrogate when you encounter causal claims. To be internally valid, a study must ensure that the causal variable (the active ingredient), and not other factors, is responsible for the change in the outcome variable. You can interrogate internal validity by exploring potential alternative explanations. For example, you might ask whether the students in the laptop notes group were given more difficult test questions than those in the handwritten notes group. If so, the difficulty of the test would be an alternative explanation for why laptop students did worse (Figure 10.5). However, the researchers gave the same test to both groups. In fact, the difficulty of the test was a control variable: It was held constant for all participants, for just this reason. More Information Figure 10.5 A threat to internal validity. If the test questions for the laptop group had been harder than the test questions for the handwritten notes group, that would have been an alternative explanation for the study’s results. (Study design and results are fabricated for illustration purposes.) You also might be wondering whether the experimenters in the Leonard et al. study treated the babies in the two groups differently. Maybe the model acted more cheerful with the babies in one condition than she did with those in the other. That would have been another threat to internal validity, so it’s important to know whether the models knew the hypothesis of the study. For any given research question, there can be several possible alternative explanations, which are known as confounds, or potential threats to internal validity. The word confound can mean “confuse”: When a study has a confound, you are confused about what is causing the change in the dependent variable. Is it the intended causal variable (such as the effort shown by the model)? Or is there some alternative explanation (such as the model’s cheerful attitude)? Internal validity is subject to a number of distinct threats, three of which—design confounds, selection effects, and order effects—are discussed in this chapter, and the rest in Chapter 11. As experimenters design and interpret studies, they keep these threats to internal validity in mind and try to avoid them. DESIGN CONFOUNDS A design confound is an experimenter’s mistake in designing the independent variable; it occurs when a second variable happens to vary systematically along with the intended independent variable. The accidental second variable is therefore an alternative explanation for the results. As such, a design confound is a classic threat to internal validity. If the adult models in the baby study had accidentally exhibited more cheerful attitudes in the effort than the no-effort condition, the study would have a design confound because the second variable (model’s cheerfulness) would have systematically varied along with the independent variable (effort versus no effort). If the test for the laptop group was harder than the test for the longhand notes group, then test difficulty would have been a design confound, too. However, the researchers did not make these errors. The models in the Leonard team’s study were carefully trained to express the same emotion in both conditions. And the notetaking researchers gave the same questions to all participants, no matter what condition they were in, so there would be no systematic differences between the groups. When an experiment has a design confound, it has poor internal validity and cannot support a causal claim. Because the Leonard et al. study did not have any apparent design confounds, its internal validity is sound. The researchers carefully thought about confounds in advance and turned them into control variables instead. Similarly, Mueller and Oppenheimer controlled for a number of potential design confounds, such as question difficulty, experimenter expectations, room conditions, and so on. In both cases, the researchers took steps to help them justify making a causal claim. Systematic Variability Is the Problem. You need to be careful before accusing a study of having a design confound. Not every potentially problematic variable is a confound. Consider the example of the baby persistence study. It might be the case that some of the adult models were cheerful and others were more reserved. The emotional expression of the models is a problem for internal validity only if it shows systematic variability with the independent variable. Did the cheerful models work only in the effort condition and the reserved ones only in the no-effort condition? Then it would be a design confound. However, if the adult models’ demeanor showed unsystematic variability (random or haphazard) across both groups, then their emotional expression would not be a confound. Here’s another example. Perhaps some of the participants in the notetaking study were interested in the video lectures and others were not. This variability in interest would not be a design confound unless it varied systematically with the notetaking condition to which they were assigned. If those in the longhand group all happened to be very interested in the lectures and those in the laptop group were all uninterested, level of interest would vary systematically with the notetaking condition and would be a confound. But if some participants in each condition were interested and some were not, that would be unsystematic variability and would not be a confound. Unsystematic variability can lead to other problems in an experiment. Specifically, it can obscure, or make it difficult to detect differences in, the dependent variable, as discussed fully in Chapter 11. However, unsystematic variability should not be called a design confound (Figure 10.6). More Information Figure 10.6 Unsystematic variability is not the same as a confound. Some babies like music more than others, some babies can sit still longer than others, and some babies just had a nap while others are tired. But individual differences don’t become a confound unless one type of baby ends up in one group systematically more than another group. If individual differences are distributed evenly in both groups, they are not a confound. Some babies like music more than others, some babies can sit still longer than others, and some babies just had a nap while others are tired. But individual differences don’t become a confound unless one type of baby ends up in one group systematically more than another group. If individual differences are distributed evenly in both groups, they are not a confound. SELECTION EFFECTS In an experiment, when the kinds of participants in one level of the independent variable are systematically different from those in the other, selection effects can result. They can also happen when the experimenters let participants choose (select) which group they want to be in. A selection effect may result if the experimenters assign one type of person (e.g., all the women, or all who sign up early in the semester) to one condition, and another type of person (e.g., all the men, or all those who wait until later in the semester) to another condition. Here’s a real-world example. A study was designed to test a new intensive therapy for autism, involving one-on-one sessions with a therapist for 40 hours per week (Lovaas, 1987; see Gernsbacher, 2003). To determine whether this therapy would cause a significant improvement in children’s autism symptoms, the researchers recruited 38 families that had children with autism and arranged for some children to receive the new intensive treatment while others received their usual treatment. The researchers initially intended to randomly assign families to either the intensive- treatment group or the treatment-as-usual group. However, some of the families lived too far away to receive the new treatment; other parents protested that they preferred to be in the intensive-treatment group. Thus, not all the families were randomly assigned to the two groups. At the end of the study, the researchers found that the symptoms of the children in the intensive-treatment group had improved more than the symptoms of those who received their usual treatment. However, this study suffered from a clear selection effect: The families in the intensive- treatment group were probably systematically different from the treatment-as-usual group because the groups self-selected. Many parents in the intensive-treatment group were placed there because of their eagerness to try a focused, 40-hour-per-week treatment regimen. Therefore, parents in that group may have been more motivated to help their children, so there was a clear threat to internal validity. Because of the selection effect, it’s impossible to tell the reason for the results (Figure 10.7). Did the children in that group improve because of the intensive treatment? Or did they improve because the families who selected the new therapy were simply more engaged in their children’s treatment? Of course, in any study that tests a therapy, some participants will be more motivated than others. This variability in motivation becomes a confound only when the more motivated folks tend to be in one group— that is, when the variability is systematic. More Information Figure 10.7 Selection effects. In a study for treating autism, some parents insisted that their children be in the new intensive-treatment group rather than the treatment-as- usual group. Because they had this choice, it’s not possible to determine whether the improvement in the intensive group was caused by the treatment itself or by the fact that the more motivated parents chose it. (Data and error bars are fabricated for illustration purposes.) Avoiding Selection Effects with Random Assignment. Well-designed experiments often use random assignment to avoid selection effects. In the baby study, an experimenter flipped a coin to determine which participants would be in each group, so each one had an equal chance of being in the effort or no-effort condition. What does this mean? Suppose that, of the 100 babies in the study, 20 were exceptionally focused. Probabilistically speaking, the flips of the coin would have placed about 10 of these very focused babies in the effort condition and about 10 in the no-effort condition. Similarly, if 12 of the babies were acting fussy that day, random assignment would place about 6 of them in each group. In other words, since the researchers used random assignment, it’s very unlikely, given the random (deliberately unsystematic) way people were assigned to each group, that all the focused or fussy babies would have been clustered in the same group. ❯❯ To review the difference between random assignment and random sampling, see Chapter 7, pp. 190–191. Assigning participants at random to different levels of the independent variable—by flipping a coin, rolling a die, or using a random number generator—controls for all sorts of potential selection effects (Figure 10.8). Of course, random assignment may not always create numbers that are perfectly even. The 20 exceptionally focused babies may be distributed as 9 and 11, or 12 and 8, rather than exactly 10 and 10. However, random assignment almost always works. In fact, simulations have shown that random assignment creates similar groups up to 98% of the time, even when there are as few as 4 people in each group (Sawilowsky, 2005; Strube, 1991). More Information Figure 10.8 Random assignment. Random assignment ensures that every participant in an experiment has an equal chance to be in each group. Random assignment is a way of desystematizing the types of participants who end up in each level of the independent variable. It creates a situation in which the experimental groups will become virtually equal, on average, before the independent variable is applied. After random assignment (and before manipulating the independent variable), researchers should be able to test the experimental groups for intelligence, extroversion, motivation, and so on, and averages of each group should be comparable on these traits. Avoiding Selection Effects with Matched Groups. In the simplest type of random assignment, researchers assign participants at random to one condition or another in the experiment. For certain studies, researchers may wish to be absolutely sure the experimental groups are as equal as possible before they administer the independent variable. In these cases, they may choose to use matched groups, or matching. To create matched groups from a sample of 30, the researchers would first measure the participants on a particular variable that might matter to the dependent variable. Student achievement, operationalized by GPA, for instance, might matter in the study of notetaking. The researchers would next match up participants in pairs, starting with the two having the highest GPAs, and within that matched set, randomly assign one of them to each of the two notetaking conditions. They would then take the pair with the next-highest GPAs and within that set again assign randomly to the two groups. They would continue this process until they reach the participants with the lowest GPAs and assign them at random too (Figure 10.9). More Information Figure 10.9 Matching groups to eliminate selection effects. To create matched groups, participants are sorted from lowest to highest on some variable and grouped into sets of two. Individuals within each set are then assigned at random to the two experimental groups. Matching has the advantage of randomness. Because each member of the matched pair is randomly assigned, the technique prevents selection effects. This method also ensures that the groups are equal on some important variable, such as GPA, before the manipulation of the independent variable. The disadvantage is that the matching process requires an extra step—in this case, finding out people’s GPA before assigning to groups. Matching therefore requires more time and often more resources than random assignment. CHECK YOUR UNDERSTANDING 1. Why do experiments usually satisfy the three causal criteria? 2. How are design confounds and control variables related? 3. How does random assignment prevent selection effects? 4. How does using matched groups prevent selection effects? 1. See pp. 283–286. 2. See pp. 286–287; control variables are used to eliminate potential design confounds. 3. See pp. 290–291. 4. See p. 291. Glossary comparison group A group in an experiment whose levels on the independent variable differ from those of the treatment group in some intended and meaningful way. Also called comparison condition. control group A level of an independent variable that is intended to represent “no treatment” or a neutral condition. Also called control condition. placebo group A control group in an experiment that is exposed to an inert treatment, such as a sugar pill. Also called placebo control group. confound A general term for a potential alternative explanation for a research finding; a threat to internal validity. design confound A threat to internal validity in an experiment in which a second variable happens to vary systematically along with the independent variable and therefore is an alternative explanation for the results. systematic variability In an experiment, a description of when the levels of a variable coincide in some predictable way with experimental group membership, creating a potential confound. See also unsystematic variability. unsystematic variability In an experiment, a description of when the levels of a variable fluctuate independently of experimental group membership, contributing to variability within groups. See also systematic variability. selection effect A threat to internal validity that occurs in an independent-groups design when the kinds of participants at one level of the independent variable are systematically different from those at the other level. random assignment The use of a random method (e.g., flipping a coin) to assign participants into different experimental groups. matched groups An experimental design technique in which participants who are similar on some measured variable are grouped into sets; the members of each matched set are then randomly assigned to different experimental conditions. Also called matching. treatment group The participants in an experiment who are exposed to the level of the independent variable that involves a medication, therapy, or intervention. INDEPENDENT-GROUPS DESIGNS Although the minimum requirement for an experiment is that researchers manipulate one variable and measure another, experiments can take many forms. One of the most basic distinctions is between independent-groups designs and within-groups designs. Independent-Groups Versus Within-Groups Designs In the notetaking and baby persistence studies, there were different participants at each level of the independent variable. In the notetaking study, some participants took notes on laptops and others took notes in longhand. In the persistence study, some babies were in the effort condition and others were in the no-effort condition. Both of these studies used an independent-groups design, in which separate groups of participants are placed into different levels of the independent variable. This type of design is also called a between-subjects design or between-groups design. In a within-groups design, or within-subjects design, each person is presented with all levels of the independent variable. For example, Mueller and Oppenheimer (2014) used an independent-groups design. But they might have run their study as a within-groups design if they had asked each participant to take notes on two videos—using a laptop for one and handwriting their notes for the other. Two basic forms of independent-groups designs are the posttest-only design and the pretest/posttest design. The two types of designs are used in different situations. Posttest-Only Design The posttest-only design, also known as an equivalent groups, posttest- only design, is one of the simplest independent-groups experimental designs. In this design, participants are randomly assigned to independent variable groups and are tested on the dependent variable once (Figure 10.10). The notetaking study is an example of a posttest-only design, with two independent variable levels (Mueller & Oppenheimer, 2014). Participants were randomly assigned to a laptop condition or a longhand condition (Figure 10.11), and they were tested only once on the video they watched. More Information Figure 10.10 A posttest-only design. More Information Figure 10.11 Studying notetaking: a posttest-only design. Posttest-only designs satisfy all three criteria for causation. They allow researchers to test for covariance by detecting differences in the dependent variable. (Having at least two groups makes it possible to do so.) They establish temporal precedence because the independent variable comes first in time. And when they are conducted well, they establish internal validity. When researchers use appropriate control variables, there should be no design confounds, and random assignment takes care of selection effects. Pretest/Posttest Design In a pretest/posttest design, or equivalent groups, pretest/posttest design, participants are randomly assigned to at least two groups and are tested on the key dependent variable twice—once before and once after exposure to the independent variable (Figure 10.12). More Information Figure 10.12 A pretest/posttest design. A study on the effects of mindfulness training, introduced in Chapter 1, is an example of a pretest/posttest design. In this study, 48 students were randomly assigned to participate in either a 2-week mindfulness class or a 2-week nutrition class (Mrazek et al., 2013). One week before starting their respective classes, all students completed a verbal-reasoning section of a GRE test. One week after their classes ended, all students completed another verbal-reasoning GRE test of the same difficulty. The results, shown in Figure 10.13, revealed that, while the nutrition group did not improve significantly from pretest to posttest, the mindfulness group scored significantly higher at posttest than at pretest. STRAIGHT FROM THE SOURCE More Information Figure 10.13 Results using a pretest/posttest design. In this study, mindfulness training caused students to improve their GRE verbal scores. (Source: Mrazek et al., 2013, Fig. 1A.) Researchers might use a pretest/posttest design when they want to be sure random assignment made groups equal. In this case, a pretest/posttest design means researchers can be absolutely sure there is no selection effect in a study. If you examine the white pretest bars in Figure 10.13, you’ll see the nutrition and mindfulness groups had almost identical pretest scores, indicating that random assignment worked as expected. In addition, pretest/posttest designs enable researchers to track people’s change in performance over time. Although the two groups started out, as expected, with about the same GRE ability, only the mindfulness group improved their GRE scores. Which Design Is Better? Why might researchers choose to do a posttest-only experiment rather than a pretest/posttest design? Shouldn’t they always make sure groups are equal on GRE ability or persistence before they experience a manipulation? Not necessarily. In some situations, it is problematic to use a pretest/posttest design. Imagine that the Leonard team had pretested the babies to see how persistent they were at pressing an inert button. If they had, the babies might have become too frustrated to continue. (Studies with babies need to be short!) Instead, the researchers trusted in random assignment to create equivalent groups. More persistent and less persistent babies all had an equal chance of being in either one of the two groups, and if they were distributed evenly across both groups, their effects would cancel each other out. Therefore, any observed difference in the number of button presses between these two groups of babies should be attributable only to the two model conditions. In other words, “being a persistent baby” was a potential selection effect, but random assignment helped avoid it. In contrast, a pretest/posttest design made sense for the Mrazek team’s study. They could justify giving their sample of students the GRE test two times because they had told participants they were studying ways of “improving cognitive performance.” In short, the posttest-only design may be the most basic type of independent-groups experiment, but its combination of random assignment plus a manipulated variable can lead to powerful causal conclusions. The pretest/posttest design adds a pretesting step to the most basic independent-groups design. Researchers might use a pretest/posttest design if they want to study improvement over time, or to be extra sure that two groups are equivalent at the start—as long as the pretest does not make the participants change their subsequent behavior. CHECK YOUR UNDERSTANDING 1. What is the difference between independent-groups and within-groups designs? Use the term levels in your answer. 2. Describe why posttest-only and pretest/posttest designs are both independent-groups designs. Explain how they differ. 1. See p. 292. 2. See pp. 292–294. Glossary independent-groups design An experimental design in which different groups of participants are exposed to different levels of the independent variable, such that each participant experiences only one level of the independent variable. Also called between-subjects design, between-groups design. within-groups design An experimental design in which each participant is presented with all levels of the independent variable. Also called within-subjects design. posttest-only design An experiment using an independent-groups design in which participants are tested on the dependent variable only once. Also called equivalent groups, posttest-only design. pretest/posttest design An experiment using an independent-groups design in which participants are tested on the key dependent variable twice: once before and once after exposure to the independent variable. WITHIN-GROUPS DESIGNS There are two basic types of within-groups designs. When researchers expose participants to all levels of the independent variable, they might do so by repeated exposures, over time, to different levels, or they might do so concurrently. Repeated-Measures Design A repeated-measures design is a type of within-groups design in which participants are measured on a dependent variable more than once, after exposure to each level of the independent variable. Here’s an example. Humans are social animals, and we know that many of our thoughts and behaviors are influenced by the presence of other people. Happy times may be happier, and sad times sadder, when experienced with others. Researchers Erica Boothby and her colleagues used a repeated-measures design to investigate whether a shared experience would be intensified even when people do not interact with the other person (Boothby et al., 2014). They hypothesized that sharing a good experience with another person makes it even better than it would have been if experienced alone, and that sharing a bad experience would make it even worse. They recruited 23 college women to come to a laboratory. Each participant was joined by a female confederate (a research assistant pretending to be a participant). The two sat side-by-side, facing forward, and never spoke to each other. The experimenter explained that each person in the pair would do a variety of activities, including tasting some dark chocolates and viewing some paintings. During the experiment, the order of activities was determined by drawing cards, but the drawings were rigged so that the real participant’s first two activities were always tasting chocolates. In addition, the real participant tasted one chocolate at the same time the confederate was also tasting it, but she tasted the other chocolate while the confederate was viewing a painting. The participant was told that the two chocolates were different, but in fact they were exactly the same. After tasting each chocolate, participants rated how much they liked it. The results showed that people liked the chocolate more when the confederate was also tasting it (Figure 10.14). More Information Figure 10.14 Testing the effect of sharing an experience using a repeated-measures design. (A) The design of the study. (B) The results of the study. Error bars represent 95% CIs. (Source: Adapted from Boothby et al., 2014.) In this study, the independent variable had two levels: sharing and not sharing an experience. Participants experienced both levels, making it a within-groups design. The dependent variable was participants’ rating of the chocolate. It was a repeated-measures design because each participant rated the chocolate twice (i.e., repeatedly). Concurrent-Measures Design In a concurrent-measures design, participants are exposed to all the levels of an independent variable at roughly the same time, and a single attitudinal or behavioral preference is the dependent variable. An example is a study investigating infant cognition, in which infants were shown a male face and a female face at the same time, and an experimenter recorded which face they looked at the longest (Quinn et al., 2002). The independent variable was the gender of the face, and babies experienced both levels (male and female) at the same time. The baby’s looking preference was the dependent variable (Figure 10.15). This study found that babies show a preference for looking at female faces, unless their primary caretaker is male. More Information Figure 10.15 A concurrent-measures design for an infant cognition study. Babies saw two faces simultaneously, and the experimenters recorded which face they looked at more. Harlow also used a concurrent-measures design when he presented baby monkeys with both a wire and a cloth “mother” (Harlow, 1958). The monkeys indicated their preference by spending more time with one mother than the other. In Harlow’s study, the type of mother was the independent variable (manipulated as within-groups), and each baby monkey’s clinging behavior was the dependent variable. Advantages of Within-Groups Designs The main advantage of a within-groups design is that it ensures the participants in the two groups will be equivalent. After all, they are the same participants! For example, some people really like dark chocolate and others do not. But in a repeated-measures design, people bring their same liking of chocolate to both conditions, so their individual liking for the chocolate stays the same. The only difference between the two conditions can be attributed to the independent variable (whether people were sharing the experience with the confederate or not). In a within- groups design such as the chocolate study, researchers say that each woman “acted as her own control” because individual or personal variables are kept constant. Similarly, when the Quinn team (2002) studied whether infants prefer to look at male or female faces as a within-groups design, they did not have to worry (for instance) that all the girl babies would be in one group or the other, or that babies with older siblings or who go to daycare would be in one group or the other. Every baby saw both types of faces, which kept any extraneous personal variables constant across the two facial gender conditions. ❮❮ To review matched-groups designs, see p. 291. The idea of “treating each participant as his or her own control” also means matched-groups designs can be treated as within-groups designs. As discussed earlier, in a matched-groups design, researchers carefully match sets of participants on some key control variable (such as GPA) and assign each member of a set to a different group. The matched participants in the groups are assumed to be more similar to each other than in a more traditional independent-groups design, which uses random assignment. ❯❯ For more on measurement error and noise in a study, see Chapter 11, pp. 347–350. Besides providing the ability to use each participant as his or her own control, within-groups designs also enable researchers to make more precise estimates of the differences between conditions. Statistically speaking, when extraneous differences (unsystematic variability) in personality, food preferences, gender, ability, and so on are held constant across all conditions, researchers can estimate the effect of the independent variable manipulation more precisely—there is less extraneous error in the measurement. Having extraneous differences between conditions in a study is like being at a noisy party: Your ability to understand somebody’s exact words is hampered when many other conversations are going on around you. A within-groups design can also be attractive because it generally requires fewer participants overall. Suppose a team of researchers is running a study with two conditions. If they want 50 participants in each condition, they will need a total of 100 people for an independent-groups design. However, if they run the same study as a within-groups design, they will need only 50 participants because each participant experiences all levels of the independent variable (Figure 10.16). In this way, a repeated-measures design can be much more efficient. More Information Figure 10.16 Within-groups designs require fewer participants. If researchers want a certain number of participants in each of two experimental conditions, a within-groups design is more efficient than an independent-groups design. Although only 40 participants are shown here (for reasons of space), psychologists usually need to use larger samples than this in their studies. Covariance, Temporal Precedence, and Internal Validity in Within-Groups Designs Do within-groups designs allow researchers to make causal claims? In other words, do they stand up to the three criteria for causation? Because within-groups designs enable researchers to manipulate an independent variable and incorporate comparison conditions, they provide an opportunity for establishing covariance. The Boothby team (2014) observed, for example, that the chocolate ratings covaried with whether people shared the tasting experience or not. A repeated-measures design also establishes temporal precedence. The experimenter controls the independent variable and can ensure that it comes first. In the chocolate study, each person tasted chocolate as either a shared or an unshared experience and then rated the chocolate. In the infant cognition study, the researchers presented the male and female faces first and then measured looking time. What about internal validity? For a within-groups design, researchers don’t have to worry about selection effects because participants are exactly the same in the two conditions. They do need to avoid design confounds, however. For example, Boothby’s team made sure both chocolates were exactly the same. If the chocolate that people tasted in the shared condition was of better quality, the experimenters would not know if it was the chocolate quality or the shared experience that was responsible for higher ratings. Similarly, Quinn’s team made sure the male and female faces they presented to the babies were equally attractive and of the same ethnicity. INTERNAL VALIDITY: CONTROLLING FOR ORDER EFFECTS Within-groups designs have the potential for a particular threat to internal validity: Sometimes, being exposed to one condition first changes how participants react to the later condition. Such responses are called order effects, and they happen when exposure to one level of the independent variable influences responses to the next level. An order effect in a within- groups design is a confound, meaning that behavior at later levels of the independent variable might be caused not by the experimental manipulation but rather by the sequence in which the conditions were experienced. Order effects can include practice effects, also known as fatigue effects, in which a long sequence might lead participants to get better at the task or to get tired or bored toward the end. Order effects also include carryover effects, in which some form of contamination carries over from one condition to the next. For example, imagine sipping orange juice right after brushing your teeth; the first taste contaminates your experience of the second one. An order effect in the chocolate-tasting study could have occurred if people rated the first chocolate higher than the second simply because the first bite of chocolate is always the best; subsequent bites are never quite as good. That would be an order effect and a threat to internal validity because the order of tasting chocolate is confounded with the condition (shared versus unshared experiences). AVOIDING ORDER EFFECTS BY COUNTERBALANCING Because order effects are potential internal validity problems in a within- groups design, experimenters want to avoid them. When researchers use counterbalancing, they present the levels of the independent variable to participants in different sequences. With counterbalancing, any order effects should cancel each other out when all the data are combined. Boothby and her colleagues (2014) used counterbalancing in their experiment (Figure 10.17). Half the participants tasted their first chocolate in the shared condition followed by a second chocolate in the unshared condition. The other half tasted chocolate in the unshared followed by the shared condition. Therefore, the potential order effect of “first taste of chocolate” was present for half of the people in each condition. When the data were combined from these two sequences, any order effect dropped out of the comparison between the shared and unshared conditions. As a result, the researchers knew that the difference they noticed was attributable only to the shared (versus unshared) experiences, and not to practice, carryover, or some other order effect. More Information Figure 10.17 Counterbalanced design. Using counterbalancing in an experiment will cancel out any order effects in a repeated-measures design. Procedures Behind Counterbalancing. When researchers counterbalance conditions (or levels) in a within-groups design, they split their participants into groups, and each group receives one of the condition sequences. How do the experimenters decide which participants receive the first order of presentation and which ones receive the second? Through random assignment, of course! They might recruit, say, 50 participants to a study and randomly assign 25 of them to receive the order A then B, and assign 25 of them to the order B then A. There are two methods for counterbalancing an experiment: full and partial. When a within-groups experiment has only two or three levels of an independent variable, researchers can use full counterbalancing, in which all possible condition orders are represented. For example, a repeated-measures design with two conditions is easy to counterbalance because there are only two orders (A → B and B → A). In a repeated- measures design with three conditions—A, B, and C—each group of participants could be randomly assigned to one of the six following sequences: A→B→C A→C→B B→A→C B→C→A C→A→B C→B→A As the number of conditions increases, however, the number of possible orders needed for full counterbalancing increases dramatically. For example, a study with four conditions requires 24 possible sequences. If experimenters want to put at least a few participants in each order, the need for participants can quickly increase, counteracting the typical efficiency of a repeated-measures design. Therefore, they might use partial counterbalancing, in which only some of the possible condition orders are represented. One way to partially counterbalance is to present the conditions in a randomized order for every subject. (This is easy to do when an experiment is administered by a computer; the computer delivers conditions in a new random order for each participant.) Another technique for partial counterbalancing is to use a Latin square, a formal system to ensure that every condition appears in each position at least once. A Latin square for six conditions (conditions 1 through 6) looks like this: 1 2 6 3 5 4 2 3 1 4 6 5 3 4 2 5 1 6 4 5 3 6 2 1 5 6 4 1 3 2 6 1 5 2 4 3 The first row is set up according to a formula, and then the conditions simply go in numerical order down each column. Latin squares work differently for odd and even numbers of conditions. If you wish to create your own, you can find formulas for setting up the first rows of a Latin square online. Disadvantages of Within-Groups Designs Within-groups designs are true experiments because they involve a manipulated variable and a measured variable. They potentially establish covariance, they ensure temporal precedence, and when experimenters control for order effects and design confounds, they can establish internal validity, too. So why wouldn’t a researcher choose a within-groups design all the time? Within-groups designs have three main disadvantages. First, as noted earlier, repeated-measures designs have the potential for order effects, which can threaten internal validity. But a researcher can usually control for order effects by using counterbalancing, so they may not be much of a concern. A second possible disadvantage is that a within-groups design might not be possible or practical. Suppose someone has devised a new way of teaching children how to ride a bike, called Method A. She wants to compare Method A with the older method, Method B. Obviously, she cannot teach a group of children to ride a bike with Method A and then return them to baseline and teach them again with Method B. Once taught, the children are permanently changed. In such a case, a within-groups design, with or without counterbalancing, would make no sense. The study on mindfulness training and GRE scores fits in this category. Once people had participated in mindfulness training, they presumably could apply their new skill indefinitely. A third problem occurs when people see all levels of the independent variable and then change the way they would normally act. Imagine a study that asks people to rate the attractiveness of two photographed people— one Black and one White. Participants in such a study might think, “I know I’m participating in a study at the moment; seeing both a White and a Black person makes me wonder whether it has something to do with prejudice.” As a result, they might change their spontaneous behavior. A cue that can lead participants to guess an experiment’s hypothesis is known as a demand characteristic, or an experimental demand. Demand characteristics create an alternative explanation for a study’s results. You would have to ask: Did the manipulation really work, or did the participants simply guess what the researchers expected them to do and change their behavior accordingly? Is Pretest/Posttest a Repeated-Measures Design? You might wonder whether pretest/posttest independent-groups design should be considered a repeated-measures design. After all, in both designs, participants are tested on the dependent variable twice. In a true repeated-measures design, however, participants are exposed to all levels of a meaningful independent variable, such as a shared or unshared experience, or the gender of the face they’re looking at. The levels of such independent variables can also be counterbalanced. In contrast, in a pretest/posttest design, participants see only one level of the independent variable, not all levels (Figure 10.18). Table 10.1 summarizes the four types of experimental designs covered in this chapter. More Information Figure 10.18 Pretest/posttest design versus repeated-measures design. In a pretest/posttest design, participants see only one level of the independent variable, but in a repeated-measures design, they see all the levels. (DV = dependent variable. IV = independent variable.) TABLE 10.1 Two Independent-Groups Designs and Two Within-Groups Designs Independent-Groups Designs Within-Groups Designs Definition: Different Definition: Same participants participants at each level of see all levels of independent independent variable variable Repeated- Posttest-only Pretest/posttest Concurrent- measures design design measures design design Glossary repeated-measures design An experiment using a within-groups design in which participants respond to a dependent variable more than once, after exposure to each level of the independent variable. concurrent-measures design An experiment using a within-groups design in which participants are exposed to all the levels of an independent variable at roughly the same time, and a single attitudinal or behavioral preference is the dependent variable. order effect In a within-groups design, a threat to internal validity in which exposure to one condition changes participant responses to a later condition. See also carryover effect, practice effect, testing threat. practice effect A type of order effect in which participants’ performance improves over time because they become practiced at the dependent measure (not because of the manipulation or treatment). Also called fatigue effect. See also order effect, testing threat. carryover effect A type of order effect, in which some form of contamination carries over from one condition to the next. counterbalancing In a repeated-measures experiment, presenting the levels of the independent variable to participants in different sequences to control for order effects. See also full counterbalancing, partial counterbalancing. full counterbalancing A method of counterbalancing in which all possible condition orders are represented. See also counterbalancing, partial counterbalancing. partial counterbalancing A method of counterbalancing in which some, but not all, of the possible condition orders are represented. See also counterbalancing, full counterbalancing. Latin square A formal system of partial counterbalancing to ensure that every condition in a within-groups design appears in each position at least once. demand characteristic A cue that leads participants to guess a study’s hypotheses or goals; a threat to internal validity. Also called experimental demand. INTERROGATING CAUSAL CLAIMS WITH THE FOUR VALIDITIES To interrogate an experimental design using the four big validities as a framework, what questions should you ask, and what do the answers mean? Let’s use Mueller and Oppenheimer’s (2014) study on notetaking as an illustration. Construct Validity: How Well Were the Variables Measured and Manipulated? In an experiment, researchers operationalize two constructs: the independent variable and the dependent variable. When you interrogate the construct validity of an experiment, you should ask about the construct validity of each of these variables. DEPENDENT VARIABLES: HOW WELL WERE THEY MEASURED? Chapters 5 and 6 explained in detail how to interrogate the construct validity of a dependent (measured) variable. To interrogate construct validity in the notetaking study, you would start by asking how well the researchers measured their dependent variables: factual knowledge and conceptual knowledge. ❮❮ To review interrater reliability, see Chapter 5, pp. 125–126. One aspect of good measurement is face validity. Mueller and Oppenheimer (2014) provided examples of the factual and conceptual questions they used, so you can examine these and evaluate whether they actually constitute good measures of factual learning (e.g., “What is the purpose of adding calcium propionate to bread?”) and conceptual learning (e.g., “If a person’s epiglottis was not working properly, what would be likely to happen?”). These two examples do seem to be appropriate types of questions because the first asks for direct recall of a lecture’s factual information, and the second requires people to understand the epiglottis and make an inference. The researchers also noted that each of these open- ended questions was graded by two coders. The two sets of scores, they reported, showed good interrater reliability (.89). In this study, the strong interrater reliability indicates that the two coders agreed about which participants got the right answers and which ones did not. INDEPENDENT VARIABLES: HOW WELL WERE THEY MANIPULATED? To interrogate the construct validity of the independent variables, you would ask how well the researchers manipulated (or operationalized) them. In the Mueller and Oppenheimer study, this was straightforward: People were given either a pen or a laptop. This operationalization clearly manipulated the intended independent variable. Manipulation Checks and Pilot Studies. In other studies, researchers need to use manipulation checks to collect empirical data on the construct validity of their independent variables. A manipulation check is an extra dependent variable that researchers can insert into an experiment to convince them that their experimental manipulation worked. A manipulation check was not necessary in the notetaking study because research assistants could simply observe participants to make sure they were actually using the laptops or pens they had been assigned. Manipulation checks are more likely to be used when the intention is to make participants think or feel certain ways. For example, researchers may want to manipulate feelings of anxiety by telling some students they have to give a public speech. Or they may wish to manipulate people’s empathy by showing a poignant film. They may manipulate amusement by telling jokes. In these cases, a manipulation check can help researchers determine whether the operationalization worked as intended. Here’s an example. Researchers were interested in investigating whether humor would improve students’ memory of a college lecture (Kaplan & Pascoe, 1977). Students were randomly assigned to listen to a serious lecture or one punctuated by humorous examples, and the key dependent variable was their memory for the material. In addition, to ensure they actually found the humorous lecture funnier than the serious one, students rated the lecture on how “funny” and “light” it was. As expected, the students in the humorous lecture condition rated the speaker as funnier and lighter than students in the serious lecture condition. The researchers concluded that the manipulation worked as expected. A similar procedure, called a pilot study, is a simple study, using a separate group of participants, that is completed before (or sometimes after) the study of primary interest to confirm the effectiveness of the manipulations. Kaplan and Pascoe (1977) might have exposed a separate group of students to either a serious or a humorous lecture and then asked them how amusing they found it. CONSTRUCT VALIDITY AND THEORY TESTING Experiments are designed to test theories. Therefore, interrogating the construct validity of an experiment requires you to evaluate how well the measures and manipulations researchers used in their study capture the conceptual variables in their theory. Recall that Mueller and Oppenheimer (2014) originally proposed that laptop notetaking would let students more easily take notes verbatim, compared with taking handwritten notes. In fact, their study included measures of “verbatim overlap” so they could test their theory about why laptop notetakers might perform worse. After transcribing each person’s notes, they measured how closely the notes overlapped verbatim with the video lecture narration. It turned out that people in the laptop condition had, in fact, written more verbatim notes than people in the longhand condition. In addition, the more people wrote verbatim notes, the worse they did on the essay test. The researchers supported their theory by measuring key constructs that their theory proposed. Here’s another example of how theory guides the variables researchers manipulate and measure in an experiment. Recall that the chocolate-tasting study was designed to test the theory that sharing an experience makes it more intense (Boothby et al., 2014). In addition to showing that good- tasting chocolate tastes better when another person is tasting it, the researchers also needed to demonstrate the same effect in response to a negative experience. Using the same repeated-measures design, in a second study they used squares of 90% dark chocolate, containing almost no sugar, so it was more bitter than the chocolate in the first study. People rated their liking for the bitter chocolate lower when the experience was shared, compared with unshared (Figure 10.19). More Information Figure 10.19 Construct validity is theory-driven. (A) When people tasted bitter chocolate in this study, they rated it more negatively when the experience was shared than when it was unshared. They also rated both of the bitter chocolates lower than the sweet chocolates in the first study, providing construct validity evidence that the experience in the second study was negative. (B) People were more absorbed in the shared experience, evidence that the shared versus unshared experience was manipulated as intended. Error bars represent 95% CIs. (Source: Adapted from Boothby et al., 2014.) Two main results of the chocolate studies support their construct validity: (1) People in the first study rated the chocolate higher overall than those in the second study, which is what you’d expect if one was supposed to represent a positive experience and the other a negative experience. (2) People reported being more absorbed in the shared experience than the unshared one. This result supports the theory that shared experiences should be more intense (absorbing) than unshared ones. External Validity: To Whom or What Can the Causal Claim Generalize? Chapters 7 and 8 discussed external validity in the context of frequency claims and association claims. Interrogating external validity in the context of causal claims is similar. You ask whether the causal relationship can generalize to other people, places, and times. (Chapter 14 goes into even more detail about external validity questions.) GENERALIZING TO OTHER PEOPLE As with an association claim or a frequency claim, when interrogating a causal claim’s external validity, you should ask how the experimenters recruited their participants. Remember that when you interrogate external validity, you ask about random sampling—randomly gathering a sample from a population. (In contrast, when you interrogate internal validity, you ask about random assignment—randomly assigning each participant in a sample into one experimental group or another.) Were the participants in a study sampled randomly from the population of interest? If they were, you can be relatively sure the results can be generalized, at least to the population of participants from which the sample came. In the Mueller and Oppenheimer study (2014), the 67 students were a convenience sample (rather than a random sample) of undergraduates from Princeton University. Because they were a convenience sample, you can’t be sure that the results would generalize to all Princeton University students, not to mention to college students in general. In addition, because the study was run only on college students, you can’t assume the results would apply to middle school or high school students. The ability of this sample to generalize to other populations is simply unknown. GENERALIZING TO OTHER SITUATIONS External validity also applies to the types of situations to which an experiment might generalize. For example, the notetaking study used five videotaped TED talk lectures. In their published article, Mueller and Oppenheimer (2014) reported two additional experiments, each of which used new video lectures. All three experiments found the same pattern, so you can infer that the effect of laptop notetaking does generalize to other TED talks. However, you can’t be sure from this study whether laptop notetaking would generalize to a live lecture class. You also don’t know whether the effect of laptop notetaking would generalize to other kinds of college teaching, such as team-based learning or lab courses. To decide whether an experiment’s results can generalize to other situations, we need to conduct more research. One experiment, conducted after Mueller and Oppenheimer’s three studies, helped demonstrate that the laptop notetaking effect can generalize to live lecture classes (Carter et al., 2016). College student cadets at West Point were randomly assigned to their real, semester-long economics classes. There were 30 sections of the class, which all followed the same syllabus, used the same textbook, and gave almost the same exams. In 10 of the sections, students were not allowed to use laptops or tablets, and in another 10 sections, they were allowed to use them. In the last 10 sections, students could use tablets as long as they were kept flat on their desk during the class. The results indicated that students in the two computerized sections scored lower on exams than students in the computer-free classrooms. This study helps us generalize from Mueller and Oppenheimer’s short-term lecture situation to a real, semester-long college class. Similarly, you might ask if Boothby et al.’s hypothesis about shared experiences might generalize to other experiences besides tasting chocolate (Figure 10.20). More Information Figure 10.20 Generalizing to other situations. The chocolate-tasting study showed that flavors are perceived as more intense when the experience is shared. A future study might explore whether the shared experiences effect generalizes to other situations, such as watching a happy or sad movie. WHAT IF EXTERNAL VALIDITY IS POOR? Should you be concerned that Mueller and Oppenheimer did not select their participants at random from the population of college students? Should you be concerned that all three of their studies used TED talks instead of other kinds of classroom material? ❮❮ For more discussion on prioritizing validities, see Chapter 14, pp. 438– 452. Remember from Chapter 3 that in an experiment, researchers usually prioritize experimental control—that is, internal validity. To get a clean, confound-free manipulation, they may have to conduct their study in an artificial environment like a university laboratory. Such locations may not represent situations in the real world. Although it’s possible to achieve both internal and external validity in a single study, doing so can be difficult. Therefore, many experimenters decide to sacrifice real-world representativeness for internal validity. Testing their theory and teasing out the causal variable from potential confounds were the steps Mueller and Oppenheimer, like most experimenters, took care of first. In addition, running an experiment on a relatively homogenous sample (such as college students) meant that the unsystematic variability was less likely to obscure the effect of the independent variable (see Chapter 11). Replicating the study using several samples in a variety of contexts is a step saved for later. Although Mueller and Oppenheimer sampled only college students and ran their studies in a laboratory, at least one other study demonstrated that taking notes by computer can cause lower grades even in real, semester-long courses. Future researchers might also be interested in testing the effect of using laptops among younger students or for other subjects (such as psychology or literature courses). Such studies would demonstrate whether longhand notetaking is more effective than laptop notetaking for all subjects and for all types of students. Statistical Validity: How Much? How Precise? What Else Is Known? Interrogating the statistical validity of an experiment involves asking about effect size, precision of the estimate, and replication. In your statistics class, you will learn how to ask other questions, such as whether the researchers conducted the right statistical tests. HOW LARGE IS THE EFFECT? The first question we can ask is, How large is the difference between the laptop and longhand groups? It appears that longhand groups learned more, but how much more? Asking this question helps you evaluate covariance (i.e., the difference between the experimental groups). In general, the larger the difference, the more important, and the stronger, the causal effect. When we do experiments, we have two ways to express effect size. The first is to use original units. In Mueller and Oppenheimer’s studies, the original units for the dependent variable were the number of points people scored correctly. Participants were tested on both factual and conceptual questions, but we’ll focus on the conceptual questions here. People in the longhand condition earned an average of 4.29 points on the conceptual questions, compared with 3.77 in the laptop condition. Therefore, the effect size in original units is 0.52 points of improvement. On the 7- question test, that might be the difference between a grade of A or B. ❮❮ For more detail on standard deviation and effect size, see Statistics Review: Descriptive Statistics, pp. 472–477 and pp. 484–488. The second way is to use a standardized effect size. In Chapter 8, you learned that the correlation coefficient r helps researchers evaluate the effect size (strength) of an association. When there are two groups in an experiment, we often use an indicator called d. This standardized effect size takes into account both the difference between means and the spread of scores within each group (the standard deviation). When d is large, it means the independent variable caused a large change in the dependent variable, relative to how spread out the scores are. When d is small, it means the scores of participants in the two experimental groups overlap more. Figure 10.21 shows what two d values might look like when a study’s results are graphed, showing all participants. Even though the difference between means is exactly the same in the two graphs, the effect sizes reflect the different degrees of overlap between the group participants. More Information Figure 10.21 Effect size and overlap between groups. Effect sizes are larger when the scores in the two experimental groups overlap less. Overlap is a function of how far apart the group means are as well as how variable the scores are within each group. On both sides of the graph, the two group means (M) are the same distance apart (about 2 units), but the overlap of the scores between groups is greater in the blue scores on the right. Because there is more overlap between groups, the effect size is smaller. ❮❮ For more questions to ask when interrogating statistical validity, see Statistics Review: Inferential Statistics, pp. 491–493. In Mueller and Oppenheimer’s first study (2014), the effect size for the difference in conceptual test performance between the longhand and laptop groups was d = 0.38. This means the laptop group scored 0.38 of a standard deviation higher than the longhand group. Psychologists sometimes start by saying a d of 0.2 should be considered small, a d of 0.5 is moderate, and a d of 0.8 is large (Cohen, 1992; in Chapter 8 you learned that that comparable benchmarks for r were.1,.3, and.5, respectively.) According to these guidelines, a d of 0.38 would be considered small to moderate. However, you also need context. As you learned in Chapter 8, a “small” effect size (such as a tiny adjustment to an athlete’s form) can have a large real-world impact, especially when accumulated over multiple people or situations. Which one should you use—original units or d? It depends on your goal. Original units are useful when you want to estimate the real-world impact of an intervention: How much would taking laptop notes affect a course grade? Standardized effect sizes are useful when you want to compare effect sizes that are based on different units. For example, using d you can compare effect sizes for exam points, time spent reading, and words used. Because d is standardized, it also enables you to compare the results found in one study to a body of knowledge. For example, in education research, one review found that the average effect size across experimental tests of educational interventions for high school students was d = 0.27 (Hill et al., 2008). It might be helpful to know that the pen versus laptop effect is in this same ballpark. HOW PRECISE IS THE ESTIMATE? In addition to estimating the size of the effect, we can also compute its 95% confidence interval (CI). Confidence intervals, introduced in Chapters 3 and 8, are computed so that 95% of them will contain the true population difference. Mueller and Oppenheimer calculated the difference (in original units) between longhand and laptop to be 0.52, and one calculation of the 95% CI for this difference is [–0.16, 1.20]. You don’t know the true population difference for longhand versus laptop notes, but 95% of CIs will contain the true difference. This CI also suggests we should not be surprised if the true difference turns out to be as large as a 1.20-point advantage or even that the laptop group scored a bit higher (–0.16 on a 7- point test; Figure 10.22). More Information Figure 10.22 95% CI for the difference in original units. In Mueller and Oppenheimer’s Study 1, the estimate of the difference in conceptual test score between laptop and longhand conditions was 0.52 points (out of 7 possible); here’s one calculation of its 95% CI. (Source: Open data from Mueller & Oppenheimer, 2014; no covariates used.) The width of the 95% CI reflects precision. When a study has a relatively small sample and more variability in the data, the CI will be relatively wide (less precise). When a study has a larger sample and less variability, then the CI will be narrower (more precise). If there were a narrower CI— say, [0.04, 0.72] for the notetaking study—it would suggest that we can probably rule out zero as the true difference between the two conditions; we’d call this result “statistically significant.” ❯❯ For details on how the CI is computed, see Statistics Review: Inferential Statistics, pp. 493–495. Instead of using original units, we can also compute the 95% CI for the d of 0.38, which might be presented like this: 95% CI for d [−0.16, 1.20]. Just as for original scores, 95% of the CIs for d will contain the d for the population. The more people in the study and the less variability, the more precise (narrow) the 95% CI will be. REPLICATION Each experiment we conduct uses data from a sample (something we know) to make an inference about a true population effect (which we may never know). A single 95% CI provides important information about how large the population effect might be. Another step in estimating the population effect is to conduct the study again and find multiple estimates. The baby persistence researchers replicated their study exactly and reported both studies’ data in their empirical journal article. Science journalist Ed Yong interviewed Leonard and reported, “After Leonard had spent a year studying the value of persistence, her advisor Laura Schulz told her to do the experiment again. ‘It was a very meta moment,’ she says. She recruited another 120 infants.... And to her delight, she got exactly the same results” (Yong, 2017). Mueller and Oppenheimer’s study was published alongside two other studies, almost identical to the first, that manipulated pen versus laptop notetaking and measured factual and conceptual knowledge; all three studies found similar effects. Nevertheless, some other researchers have found smaller, and even no, benefits of longhand over laptop notes (Luo et al., 2018; Morehead et al., 2019; Urry et al., 2020). When the studies you encounter have been replicated, you can combine their estimates to get an even better estimate of the true population value. Internal Validity: Are There Alternative Explanations for the Results? ❮❮ For a full discussion of replication, including meta-analysis, see Chapter 14, pp. 437–447. When you are interrogating causal claims, keep in mind that internal validity is often the priority. Experimenters isolate and manipulate a key causal variable, while controlling for all other possible variables, precisely so they can achieve internal validity. If the internal validity of an experiment is sound, you know that a causal claim is almost certainly appropriate. But if there is some confound, a causal claim would be inappropriate. It should instead be demoted to an association claim. Three potential threats to internal validity have already been discussed in this chapter. These fundamental internal validity questions are worth asking of any experiment: 1. Did the experimental design ensure that there were no design confounds, or did some other variable accidentally covary along with the intended independent variable? (Mueller and Oppenheimer made sure people in both groups saw the same video lectures, were in the same room, and so on.) 2. If the experimenters used an independent-groups design, did they control for selection effects by using random assignment or matching? (Random assignment controlled for selection effects in the notetaking study.) 3. If the experimenters used a within-groups design, did they control for order effects by counterbalancing? (Counterbalancing is not relevant in Mueller and Oppenheimer’s design because it was an independent- groups design.) Chapter 11 goes into further detail on these threats to internal validity and covers nine more threats. CHECK YOUR UNDERSTANDING 1. How do manipulation checks provide evidence for the construct validity of an experiment’s independent variable? Why does theory matter in evaluating construct validity? 2. Besides generalization to other people, what other aspect of generalization does external validity address? 3. What does it mean when an effect size is large (as opposed to small) in an experiment? 4. Summarize the three threats to internal validity discussed in this chapter. 1. See pp. 304–306. 2. Generalization to other situations; see pp. 306–307. 3. See pp. 308–309. 4. See p. 311. Glossary manipulation check In an experiment, an extra dependent variable researchers can include to determine how well a manipulation worked. pilot study A study completed before (or sometimes after) the study of primary interest, usually to test the effectiveness or characteristics of the manipulations. CHAPTER REVIEW It’s time to complete your study experience! Go to INQUIZITIVE to practice actively with this chapter’s concepts and get personalized feedback along the way. Summary Causal claims are special because they can lead to advice, treatments, and interventions. The only way to support a causal claim is to conduct a well- designed experiment. TWO EXAMPLES OF SIMPLE EXPERIMENTS An experiment showed that taking notes on a laptop rather than in longhand caused students to do worse on a conceptual test of lecture material. An experiment showed that babies who watch adults being persistent try harder on a subsequent task. EXPERIMENTAL VARIABLES Experiments study the effect of an independent (manipulated) variable on a dependent (measured) variable. Experiments deliberately keep all extraneous variables constant as control variables. WHY EXPERIMENTS SUPPORT CAUSAL CLAIMS Experiments support causal claims because they potentially allow researchers to establish covariance, temporal precedence, and internal validity. The three potential internal validity threats covered in this chapter that researchers work to avoid are design confounds, selection effects, and order effects. INDEPENDENT-GROUPS DESIGNS In an independent-groups design, different participants are exposed to each level of the independent variable. In a posttest-only design, participants are randomly assigned to one of at least two levels of an independent variable and then measured once on the dependent variable. In a pretest/posttest design, participants are randomly assigned to one of at least two levels of an independent variable and are then measured on a dependent variable twice—once before and once after they experience the independent variable. Random assignment or matched groups can help establish internal validity in independent-groups designs by minimizing selection effects. WITHIN-GROUPS DESIGNS In a within-groups design, the same participants are exposed to all levels of the independent variable. In a repeated-measures design, participants are tested on the dependent variable after each exposure to an independent variable condition. In a concurrent-measures design, participants are exposed to at least two levels of an independent variable at the same time and then indicate a preference for one level (the dependent variable). Within-groups designs allow researchers to treat each participant as his or her own control and require fewer participants than independent-groups designs. Within-groups designs also present the potential for order effects and demand characteristics. INTERROGATING CAUSAL CLAIMS WITH THE FOUR VALIDITIES Interrogating construct validity involves evaluating whether the variables were manipulated and measured in ways consistent with the theory behind the experiment. Interrogating external validity involves asking whether the experiment’s results can be generalized to other people or to other situations and settings. Interrogating statistical validity starts by asking about the effect size, precision of the estimate as assessed by the 95% CI, and whether the study has been replicated. Interrogating internal validity involves looking for design confounds and seeing whether the researchers used techniques such as random assignment and counterbalancing. Key Terms experiment, p. 281 manipulated variable, p. 281 measured variable, p. 281 independent variable, p. 281 condition, p. 281 dependent variable, p. 281 control variable, p. 282 comparison group, p. 284 control group, p. 284 treatment group, p. 284 placebo group, p. 285 confound, p. 286 design confound, p. 286 systematic variability, p. 287 unsystematic variability, p. 287 selection effect, p. 289 random assignment, p. 290 matched groups, p. 291 independent-groups design, p. 292 within-groups design, p. 292 posttest-only design, p. 292 pretest/posttest design, p. 293 repeated-measures design, p. 295 concurrent-measures design, p. 296 order effect, p. 299 practice effect, p. 299 carryover effect, p. 299 counterbalancing, p. 299 full counterbalancing, p. 300 partial counterbalancing, p. 301 Latin square, p. 301 demand characteristic, p. 302 manipulation check, p. 304 pilot study, p. 304 To see samples of chapter concepts in the popular media, visit www.everydayresearchmethods.com and click the box for Chapter 10. Review Questions Max ran an experiment in which he asked people to shake hands with an experimenter (played by a female friend) and rate the experimenter’s friendliness using a self-report measure. The experimenter was always the same person and used the same standard greeting for all participants. People were randomly assigned to shake hands with her either after she had cooled her hands under cold water or after she had warmed her hands under warm water. Max’s results found that people rated the experimenter as friendlier when her hands were warm than when they were cold. 1. Why does Max’s experiment satisfy the causal criterion of temporal precedence? 1. Because Max found a difference in rated friendliness between the two conditions, cold hands and warm hands. 2. Because the participants shook the experimenter’s hand before rating her friendliness. 3. Because the experimenter acted the same in all conditions, except having cold or warm hands. 4. Because Max randomly assigned people to the warm hands or cold hands condition. 2. In Max’s experiment, what was a control variable? 1. The participants’ rating of the friendliness of the experimenter. 2. The temperature of the experimenter’s hands (warm or cold). 3. The gender of the students in the study. 4. The standard greeting the experimenter used while shaking hands. 3. What type of design is Max’s experi