2024 One-way ANOVA (PDF)
Document Details
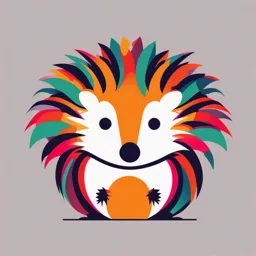
Uploaded by WellBredTurtle345
Melbourne School of
2024
Daniel Little
Tags
Summary
These lecture notes provide an introduction to one-way ANOVA, covering topics like its purpose, applications, statistical analysis, and assumptions. Included are explorations into statistical concepts like inferences, comparisons, decision-making, and the relationship between ANOVA and t-tests.
Full Transcript
DIFFERENCES BETWEEN GROUPS: INTRODUCTION TO ONE-WAY ANOVA PSYC20007 Cognitive Psychology A/Prof Daniel Little Learning Objectives 1. Define One-Way ANOVA and explain its primary purpose in comparing group means 2. Identify and describe real-world applications of One- Way AN...
DIFFERENCES BETWEEN GROUPS: INTRODUCTION TO ONE-WAY ANOVA PSYC20007 Cognitive Psychology A/Prof Daniel Little Learning Objectives 1. Define One-Way ANOVA and explain its primary purpose in comparing group means 2. Identify and describe real-world applications of One- Way ANOVA 3. Distinguish between populations and samples in the context of statistical analysis 4. Calculate and interpret the p-value for a single sample to assess statistical significance 5. Compute and compare p-values when analyzing data from two samples 6. Explain the relationship between One-Way ANOVA and the t-test 7. Calculate the F-statistic for One-Way ANOVA and explain its role in hypothesis testing 8. Describe the concept of degrees of freedom and their impact on the F-distribution in One-Way ANOVA 9. Identify and explain the assumptions necessary for valid One-Way ANOVA results 10. Perform and evaluate post-hoc tests to determine specific group differences following a significant ANOVA result Overview Definition and purpose of ANOVA Applications of one-way ANOVA Populations versus Samples p-value for a single sample p-value for two samples Relation to the t-test Computing the F-statistic Degrees of Freedom ANOVA Assumptions Post-hoc Tests Why statistics? 1. Inference: Making conclusions about a population based on sample data. 2. Comparison: Assessing whether there are significant differences between groups. 3. Decision Making: Using statistical tests to inform decisions in various fields (e.g., education, medicine, marketing). 4. Generalization: Applying findings from a sample to the broader population. 5. Quantifying Uncertainty: Providing a measure of confidence in the results and understanding the role of variability in data. Why statistics? 1. Inference: Making conclusions about a population based on sample data. 2. Comparison: Assessing whether there are significant differences between groups. Sample 1 3. Decision Making: Using statistical tests to inform decisions in various fields (e.g., education, medicine, marketing). 4. Generalization: Applying findings from a sample to the broader population. Sample 2 5. Quantifying Uncertainty: Providing a measure of confidence in the results and understanding the role of variability in data. Why statistics? 1. Inference: Making conclusions about a population based on sample data. 2. Comparison: Assessing whether there are significant differences between groups. 3. Decision Making: Using statistical tests to inform decisions in various fields (e.g., education, medicine, marketing). 4. Generalization: Applying findings from a sample to the broader population. 5. Quantifying Uncertainty: Standardized Testing Providing Clinical Trials a measure of A/B Testing confidence in the results and understanding the role of variability in data. Why statistics? 1. Inference: Making conclusions about a population based on sample data. 2. Comparison: Assessing whether there are significant differences between groups. 3. Decision Making: Using statistical tests to inform decisions in various fields (e.g., education, medicine, marketing). 4. Generalization: Applying findings from a sample to the broader population. 5. Quantifying Uncertainty: Providing a measure of confidence in the results and understanding the role of variability in data. Why statistics? 1. Inference: Making conclusions about a population based on sample data. 2. Comparison: Assessing whether there are significant differences between groups. 3. Decision Making: Using statistical tests to inform decisions in various fields (e.g., education, medicine, marketing). 4. Generalization: Applying findings from a sample to the broader population. 5. Quantifying Uncertainty: Providing a measure of confidence in the results and understanding the role of variability in data. Definition of ANOVA ANOVA stands for ANalysis Of VAriance One-Way ANOVA: Statistical technique to determine if two or more groups are statistically different from one another Works by testing the probability of the null hypothesis that all group Purpose Are there statistical differences between multiple groups? Helps determine if at least one group has a different mean from the other groups Variables with distinct groups but no inherent orderi Assesses the effect of a single categorical independent variable on a single Variables continuous dependent variable that can take on any value within a given range Key concepts Null hypothesis – all group means are equal A limitation of ANOVA is that it does not tell you which Alternative hypothesis group is different – at least one group mean is different Between-group variance – Variability attributed to between group differences Within-group variance – Variability within each group F-statistic – Ratio of between-group variance to within-group variance p-value – Probability of observing the test statistic, F, assuming that the null hypothesis is true Key concepts Null hypothesis – all group means are equal Alternative hypothesis – at least one group mean is different ANOVA works by comparing between group variance Between-group variance (which comes from your – Variability attributed to between group differences experimental manipulation) to within-group variance Within-group variance (which comes from noise or – Variability within each group randomness alone) F-statistic – Ratio of between-group variance to within-group variance p-value – Probability of observing the test statistic, F, assuming that the null hypothesis is true Key concepts Null hypothesis – all group means are equal Alternative hypothesis – at least one group mean is different Between-group variance – Variability attributed to between group differences Within-group variance The values which come – Variability within each group out of the ANOVA analysis are an F- F-statistic statistic, which in turn – Ratio of between-group variance to within-group varianceallows you to determine a p-value p-value – Probability of observing the test statistic, F, assuming that the null hypothesis is true Why do we need statistical tests? In any dataset, there is variability due to: – Measurement error – Experimental or Observational conditions – Individual differences – Random fluctuations Statistical tests help quantify variability and distinguish what is due to randomness (within- group variance) and what is due to effects we might be interested in (between-group variance) Applications of One-Way ANOVA Are there differences in final exam scores between students taught using traditional lectures, online courses, or blended learning? DV: IV: Applications of One-Way ANOVA A medical facility is trialling three different medications to examine the reduction in blood pressure DV: IV: Applications of One-Way ANOVA Netflix compares three different front-page organisation strategies for presenting new content DV: IV: Applications of One-Way ANOVA Comparing scores on an anxiety test from patients receiving CBT, medication, or a combination of both DV: IV: Applications of One-Way ANOVA Applying different training methods for detecting fake news and then comparing identification accuracy DV: IV: Experiment Each of these applications involves an experiment – Some independent variable is manipulated across several categorical conditions – Outcomes are recorded on one continuous dependent variable The outcome of the experiment is data Goals of Statistical Inference From the data, we want to: – Infer something about the population Is our sample representative of the population? – Determine whether multiple samples come from the same or different populations? Is there a significant difference between groups? – Predict what the population The effect size will look like describes the if we apply our manipulation? magnitude of the observed differences Did our manipulation work? Data are noisy and variable – reflect the process we’re interested in (our experiment) but also random variation How can you determine whether the data were generated by the same underlying process or by different processes? – If data in different conditions is generated from the same process then we would assume that the data should “look the same” in both conditions – What does this imply for our manipulation? How should we compare groups? In psychology, the easiest way to compare data from different groups is to compare the mean or average score within each group If we do find that different conditions have different means, how can we be certain that these averages are reliable and not due to randomness? Data with Different Means Assume we’ve measured anxiety 22 levels after three types 16 of 20 treatment: 14 18 1) 16CBT 12 2) 14Medication 10 3) 12Both Anxiety Anxiety 8 10 The figure tells us that there is a 6 8 decrease in Anxiety levels from 4 CBT6 to Medication to Both 4 2 We2might be tempted to 0 conclude 0 that this difference is CBT Medication Both CBT Medication Both meaningful Data with Different Means 22 16 20 But when 14 we examine the actual 18 variability in the samples, we 12 16 can see that the difference in 14 the means 10 cannot be Anxiety Anxiety 12 interpreted without also thinking 8 10 about the fact that variability 8 increases 6 from CBT to Medication 4 to Both 6 4 2 2 0 0 CBT Medication Both CBT Medication Both Data with Different Means 20 16 18 We need 14 to compare the 16 variability of the samples across 12 14 conditions 10 12 Anxiety Anxiety 10 When 8the variability is 8 consistent, 6 the difference between the means (between 6 4 group differences) can inform us 4 about 2statistical significance 2 0 0 CBT Medication Both CBT Medication Both Why would our data be affected by random variation? Because we’re not dealing directly with populations but only samples from those populations Populations – the entire collection of data (all possible measurements or outcomes) from the group that we are interested in Samples – a random subset of a population which is representative of that population Populations and Samples All adults diagnosed with A randomly selected group depression in Melbourne of 150 adults receiving treatment at different clinics in the area All Instagram users A randomly selected group of 500 users who logged on in the past six months All trees in Victoria 842 randomly selected trees from Victorian forests A randomly selected group All voting age adults in of 300 voting age adults Australia from various electorates Populations and Samples Note how the samples do not perfectly represent the distribution We do not have access 18 This is analogous to how to the population 16 when you don’t always get 5 we examine our data heads out of 10 flips of a 14 fair coin All we have is the12 sample This is RANDOM Count 10 SAMPLING 8 6 4 2 0 -4 -3 -2 -1 0 1 2 3 4 Observed Value Populations and Samples Is the red line our best 18 guess at what the 16 population looks like? 14 Or is it more like the 12 dashed green line? Count 10 8 Or something else? 6 4 2 0 -4 -3 -2 -1 0 1 2 3 4 Observed Value Determining whether two samples were generated from the same population How to determine whether two samples came from the same population? – Did our manipulation have an effect? How? – Look at the data! The first step should always be to look at summaries of the data (means, graphs, etc) – Do the data look different from one another? – If so, how can we tell whether it is due solely to random chance? This is where statistical testing comes in Populations and Samples Null Hypothesis 18 16 14 12 Count 10 8 6 4 2 0 -4 -3 -2 -1 0 1 2 3 4 Observed Value Populations and Samples Alternative Hypothesis 18 16 14 12 Count 10 8 6 4 2 0 -4 -3 -2 -1 0 1 2 3 4 Observed Value Summary Data are noisy We must infer from the sample what we want to know about the population For one-way ANOVA, we have more than two samples and we want to know if they’re different To understand one-way ANOVA, we can generalise from thinking about the probability of a single data point How to decide if a datum comes from a distribution or not For any data point we observe, we work out the probability that it was generated by some hypothetical This is distribution a normal distribution with an arbitrary mean and standard deviation I made up (but it could be based on some principled reasoning – like the group average and standard deviation) For this single data point, we are trying to determine whether it was generated from this distribution This data point is “unusual” because it is far from the mean (how far can the data point be before we conclude that it did not come from this distribution?) The Normal Distribution By integrating “under the curve” we can compute the probabilities in different regions of the normal distribution 34.1% 2.1% 13.6% -3σ -2σ -1σ 0 1σ 2σ 3σ σ – Symbol for standard deviation The Normal Distribution 95% of all scores are within +/- 1.95 standard deviations -3σ -2σ -1σ 0 1σ 2σ 3σ σ – Symbol for standard devia “Unusual” data are rare data Very short and very long Netflix Viewing durations are rare Durations 90 (relative to 80 What counts as “very average) 70 early” or “very late”? Mean = - 0.3 SD = 5.1 60 More than 2 SD’s ± Frequency 50 mean Range is -15 to 40 +17 30 Mean ± 2 SD = -0.3 ± 2 × 20 5.1 10 2.2% are very early 0 -20 -10 0 10 20 (less than -10.5) Time 2.1% are very late Extreme cases are rare (low probability) (greater than +9.9). Extreme cases are unlikely to have been generated from this distribution “Unusual” data result in a low p-value (when we examine the probability of being generated by some distribution) 0.4 If the data are generated from a 0.3 normal Prob. 0.2 distribution THEN cases that p 0.1 are more than 2 0.0 <.05 -3 -2 -1 0 1 2 3 standard Z Score The bell-shaped normal distribution deviations from Unusual cases occur with a the mean occur probability of less than 5% only 5% of the time Summary Typically, we consider 2 distributions (or less than.05 probability) as being too “unusual” to consider as being generated from that distribution What the p-value means: Part I Dealing with a single sample The p-value means that IF our assumptions about the population distribution are true… – i.e., that the population has a normal distribution with a mean and variance equal to some value Then our sample will occur with a probability equal to the p-value If p <.05, then it is very unlikely that our sample came from that population distribution What the p-value means: Part I Dealing with a single sample The probability of our data being a sample from that population distribution is less than 1 in 20 When we make inferences we have to allow for the possibility that we might be wrong – Being wrong 1 out of 20 times is the level of risk that we (as a discipline) are willing to take.05 is the alpha level or the Type I error- rate (false alarm error rate) What the p-value means: Part I Dealing with a single sample p: Probability that our observed data was generated from some specified distribution p <.05 Alpha level: some arbitrary cutoff that we set to determine statistical significance Summary Random Sampling necessitates that we will sometimes observe data with unlikely values (unusual data) by chance alone We can use the p-value to determine whether or not we think a data point comes from a particular population What if we want to tell whether two (or more) samples come from different distributions? What the p-value means: Part II Dealing with a two samples If our experimental manipulations have no effect then the observed variation is due to chance… …and our groups will not be different enough to say that they are truly different – Chance variation is also known as within-group variation If our experimental manipulation does have an effect, then it will push the groups apart more than just chance What the p-value means: Part II Dealing with two samples 18 18 16 16 14 14 12 12 Count Count 10 10 8 8 6 6 4 4 2 2 0 0 -4 -3 -2 -1 0 1 2 3 4 -4 -3 -2 -1 0 1 2 3 4 Observed Value Observed Value Experimental groups come Experimental groups come from same population from different populations Difference in samples due Difference in samples due to chance only to chance + effect What the p-value means: Part II Dealing with two samples 18 16 14 12 NULL Hypothesis Count 10 8 6 4 Null = no experimental effect 2 Differences due to chance only 0 -4 -3 -2 -1 0 1 2 3 4 Observed Value In a one-way ANOVA, the p-value tells us the probability of observing a difference between our samples (i.e., our data) given that the Null Hypothesis is true p <.05 means that if the Null Hypothesis is true, then we have a less than 5 % chance observing the difference we found between our samples. Relation to t-tests The t-test looks at the distribution of differences between the scores What types of differences are expected under the NULL Hypothesis that there is no difference? – The Null hypothesis predicts the difference should be 0 – But due to random variation there will always be some variation around 0 (the distribution describing this variation is called the t- distribution) – If we observe a difference which is not very probable under the t-distribution, then we say that we have observed a significant difference Relation to t-tests We can compare pairs of typical scores (means) using t-tests. – A t-test compares means for two groups – You can use a one-way ANOVA to compare two groups, but one-way ANOVA can also be compared to 3 or more groups ANOVA: The F-statistic Like the t-test provides a statistic (the t- value) and an associate probability of that t-value (i.e., the p-value) The ANOVA provides a statistics called the F-statistic that has its own associated p- value The F-statistic is also called the F-value or F-ratio A conceptual explanation of ANOVA The F-statistic compares variation between and within groups a large F-statistic suggests that the samples come from different populations Which means that the null hypothesis is less likely to be true Summary Statistical tests like t-tests and one- way ANOVA provide a value of some test statistic These values can be assessed against a statistical distribution to provide a p-value Each statistical test has its own associated distribution How does ANOVA work? I’m going to fabricate two sets of data: – Both are supposed to measure “test scores” within three different groups of 1000 participants each Dataset 1: I assume that mean test score for all 3 groups is about 10, so the only difference among the groups is due to the chance of the simulation. Dataset 2: I assume that mean test score is: – 10 in group 1 – 15 in group 2 – 20 in group 3 I’ll show how ANOVA can detect differences for Dataset 2 but detects no difference for Dataset 1 Dataset 1 Test scores Report anxiety group Mean Std. Deviation 1.00 10.0333 1.92437 2.00 10.0959 2.00327 3.00 10.0292 2.02435 Total 10.0528 1.98404 100 100 100 Group 1 Group 2 Group 3 Frequency Frequency Frequency 50 50 50 0 0 0 0 5 10 15 20 0 5 10 15 20 0 10 20 Anxiety Anxiety Anxiety Test Scores Test Scores Test Scores 300 Total Frequency 200 All scores 100 combined into one histogram 0 0 10 20 Anxiety Test Scores Total (across three groups) 250 200 Mean = 10.08 Std. Dev = 1.99 Frequency 150 N = 3000 100 50 0 0 5 10 15 20 Anxiety Test Scores Total variance = 3.9 Variance = SD Total Sums of Squares (SS) = 1117 SSTotal = Σ(x – m) 100 100 100 Group 1 Group 2 Group 3 Frequency Frequency Frequency 50 50 50 0 0 0 0 5 10 15 20 0 5 10 15 20 0 10 20 Anxiety Anxiety Anxiety Test Scores Test Scores Test Scores 300 Total Group 1 Frequency Group 2 Group 3 200 Var = 3.95 Var = 3.65 Var = 3.56 SS = 3950 100 SS = 3648 SS = 3556 0 0 10 20 Within = 3950 + 3648 + 3556 = 111 Anxiety otal = 11176 Between = SSTotal – SSWithin = 23 Summary SSWithin: One-way ANOVA works by calculating the sum of squares within each group and adding these values together SSTotal: We then compute the total sum of squares after combining all of the data SSBetween: We find the sum of squares between groups by subtracting SSWithin from SSTotal Dataset 2 Test scores Report anxiety group Mean Std. Deviation 1.00 10.0333 1.92437 2.00 15.0959 2.00327 3.00 20.0292 2.02435 Total 15.0528 4.53819 150 Group 1 150 Group 2 150 Group 3 Frequency Frequency Frequency 100 100 100 50 50 50 0 0 0 0 10 20 30 0 10 20 30 0 10 20 30 Anxiety Anxiety Anxiety Test Scores Test Scores Test Scores 150 Total Frequency 100 50 0 0 10 20 30 Anxiety Test Scores Because the means of the three groups are different The variation of the total data is MUCH greater 150 Total Frequency 100 50 0 0 10 20 30 Anxiety Test Scores VarianceTotal = 20.584 SSTotal = 61732 150 Group 1 150 Group 2 150 Group 3 Frequency Frequency Frequency 100 100 100 50 50 50 0 0 0 0 10 20 30 0 10 20 30 0 10 20 30 Anxiety Anxiety Anxiety Test Scores Test Scores Test Scores 150 Total Group 1 Frequency 100 Group 2 Group 3 Var = 3.63 Var = 3.96 Var = 3.86 50 SS = 3631 SS = 3965 SS = 3860 0 0 10 20 30 Within = 3631 + 3965 + 3860 = 114 Anxiety otal = 61732 Between = SSTotal – SSWithin = 50276 Summary Dataset 1 – Variation between groups is much less than variation within groups – No substantial difference among means Dataset 2 – Variation between groups is much greater than variation within groups – Big differences between means of each group F-statistic The F-statistic is a ratio of the variance between to the variance within groups. 𝐵𝑒𝑡𝑤𝑒𝑒𝑛𝐺𝑟𝑜𝑢𝑝𝑠 𝑉𝑎𝑟𝑖𝑎𝑡𝑖𝑜𝑛 𝐹= 𝑊𝑖𝑡h𝑖𝑛𝐺𝑟𝑜𝑢𝑝𝑠 𝑉𝑎𝑟𝑖𝑎𝑡𝑖𝑜𝑛 F-statistic 𝑀𝑆 𝑏𝑒𝑡𝑤𝑒𝑒𝑛 𝐹= 𝑀𝑆 𝑤𝑖𝑡h𝑖𝑛 Dataset 2 𝑆𝑆𝑤𝑖𝑡h𝑖𝑛 11456 𝑀𝑒𝑎𝑛 𝑆𝑞𝑢𝑎𝑟𝑒 𝑊𝑖𝑡h𝑖𝑛= 𝑑𝑓 𝑤𝑖𝑡h𝑖𝑛 ? 𝑆𝑆𝑏𝑒𝑡𝑤𝑒𝑒𝑛 50276 𝑀𝑒𝑎𝑛 𝑆𝑞𝑢𝑎𝑟𝑒 𝐵𝑒𝑡𝑤𝑒𝑒𝑛= 𝑑𝑓 𝑏𝑒𝑡𝑤𝑒𝑒𝑛 ? How to compute the F- statistic SSWithin: due to chance alone SSBetween: chance plus any experimental effect The sum of squares is measure of variation; however, sum of squares will be sensitive to the sample size – SS grows larger as more scores are added so increasing the sample size will increase the SS We need to correct the sum of squares for the number of groups and the number of subjects Degrees of freedom (df) – the number of independent scores – there are two df for a one-way ANOVA. – you can think of df as a way to account for both the number of groups and the size of the sample in the calculation of the F statistic Degrees of freedom (df) Given a set of numbers One number fixed 4 9 8 1010 7 Total That has some mean degrees of M = 7.6 freedom = 4 If I fix one of the numbers, I can allow all of the other numbers to vary and still recover One number fixed the same mean 10 1 12 1 10 14 Different numbers M = 7.6Same mean Degrees of freedom (df) 3000 participants: 1000 in each of 3 groups dfTotal = N – 1 Number of participants minus 1 dfBetween = k – 1 Number of conditions minus 1 dfWithin = N – k Number of participants minus the number of groups F-statistic 𝑀𝑆 𝑏𝑒𝑡𝑤𝑒𝑒𝑛 𝐹= 𝑀𝑆 𝑤𝑖𝑡h𝑖𝑛 Dataset 2 𝑆𝑆𝑤𝑖𝑡h𝑖𝑛 11456 𝑀𝑒𝑎𝑛 𝑆𝑞𝑢𝑎𝑟𝑒 𝑊𝑖𝑡h𝑖𝑛= 𝑑𝑓 𝑤𝑖𝑡h𝑖𝑛 2997 𝑆𝑆𝑏𝑒𝑡𝑤𝑒𝑒𝑛 50276 𝑀𝑒𝑎𝑛 𝑆𝑞𝑢𝑎𝑟𝑒 𝐵𝑒𝑡𝑤𝑒𝑒𝑛= 𝑑𝑓 𝑏𝑒𝑡𝑤𝑒𝑒𝑛 2 What does the F-ratio mean? Distribution of the F-statistic 95% of the F-ratios are in the main part of the distribution Probability Density 0 1 2 3 4 5 6 F-statistic What does the F-ratio mean? Distribution of the F-statistic 5% of the F-ratios are in the tail The p-value tells us where our F-ratio Probability Density is located in this distribution 0 1 2 3 4 5 6 F-statistic The F statistic The F-statistic is assessed against the F- distribution to see whether it is extreme or not (i.e., less than.05?) – There are two degrees of freedom (df) required – one for the within and one for the between groups variance – df determines the shape of the distribution Summary We can draw conclusions about comparisons of means from sampled data, using null hypothesis significance testing. The basic idea is that if a sample statistic is extreme and unusual, assuming a null hypothesis, then there is evidence that the null hypothesis may not hold. More formally, if the probability of the sample statistic (the F-statistic) is less than.05 reject the null hypothesis. P-values and decisions Type I Error (False Positive) – Concluding there is an effect when there is none – Alpha or significance level Type II Error (False Negative) – Failing to detect an effect when there is one – Statistical Power Assumption I: Independence of Observations – the value of one observation does not influence or is not influenced by another observation – Violating this assumption can lead to increased Type I and Type II error – Typically ensured by using careful data collection and random assignment to conditions Assumption II: Normality – data within each group should be approximately normally distributed – ANOVA relies on this assumption to ensure that the sampling distribution of the mean is normally distributed – This is important for small sample sizes – With larger sample size, ANOVA is robust to violations of normality – Variable transformations can be used to improve normality Assumption III: Homogeneity of Variance – Each group should have approximately the same variance – Unequal variances can affect the validity of the F-statistic and lead to incorrect conclusions – Levene's test or Bartlett's test can be used to assess whether variances are equal across groups – If violated, non-parametric tests that don’t rely on this assumption can be used Structure of the ANOVA table Experimental conditions Within-group variance (or error variance) Residuals are the differences between the observed data points and the group means Structure of the ANOVA table SSBetween SSWithin Structure of the ANOVA table dfBetween dfWithin Structure of the ANOVA table MSBetween MSWithin Structure of the ANOVA table F-statistic Structure of the ANOVA table p-value Structure of the ANOVA table Effect size Effect size will be discussed in your lab class Structure of the ANOVA table There are different methods of partitioning between and within group variance. Type III is the most general and is used as default in most stats packages Post-hoc tests ANOVA tells us that there is a significant difference, but it doesn’t tell us where Common post-hoc tests – Tukey’s HSD – Bonferroni Correction Both methods compare all of the groups against every other group They differ in how they compute the significance between groups Summary For your lab experiment, we will use a one-way ANOVA to compare the 3 conditions from the experiment We will additionally calculate the post-hoc Bonferroni Correction test Beyond One-Way ANOVA Two-way ANOVA – Main Effects – Interactions Three-way ANOVA, … , N-way ANOVA Regression – Linear regression – Multiple regression General Linear Model Multilevel or Hierarchical Modelling Key References Gravetter, F.J. & Wallnau L.B. (2017). Statistics for the Behavioural Sciences (10th ed.) Other editions are also fin