PS219: Research Methods in Psychology - One-way ANOVA PDF
Document Details
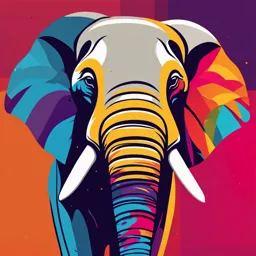
Uploaded by CommendableSitar412
University of Galway
2025
Rory Coyne
Tags
Related
- BEHL 2005/2019 (UO) Introductory Research Methods PDF
- Experimental Design I: Single Factor Designs Chapter 7 PDF
- Statistical Analysis PDF
- Activity 13 Quiz 4 (20 Points) PDF
- Covariance Research Design PDF
- HPS3U34 – CM 10-11-12 : Tester les effets principaux, d'interaction, et simples dans les différents plans factoriels (PDF)
Summary
This document contains lecture slides for PS219: Research Methods in Psychology, focusing on between-subjects design and one-way ANOVA, presented by Rory Coyne in 2025. The lecture covers topics such as one-factor, multiple group designs, assumptions of ANOVA, and analysis of variance.
Full Transcript
PS219: Research Methods in Psychology Between-subjects design Part 2: One-way between-subjects ANOVA February 11th, 2025 Rory Coyne PS219 Module Coordinator [email protected] When separate subjects (participants) must be used for each experimental condition, the design is called a be...
PS219: Research Methods in Psychology Between-subjects design Part 2: One-way between-subjects ANOVA February 11th, 2025 Rory Coyne PS219 Module Coordinator [email protected] When separate subjects (participants) must be used for each experimental condition, the design is called a between subjects design. Recap on yesterday... In a non-equivalent group design, different groups of participants are formed under circumstances that do not permit the researcher to control the assignment of individuals to groups. The independent-samples t-test is used to test the difference in means between two independent groups and is used in a between-subjects design. Check the p-value to see if there is a significant difference between groups (p <.05). The larger the t score, the bigger the difference between groups. Today we will cover... 01 One-factor, multiple group designs 02 One-way Analysis of Variance 03 Assumptions of ANOVA 04 One-way between-subjects ANOVA Between Groups Design: One Factor Multiple Group Designs A one-factor multiple group design is a research design where a single measure (the dependent variable) is recorded for more than two levels of one independent variable. One of the typical conditions (levels) is a control group where the value of the independent variable remains neutral. The remaining groups are defined by their respective levels of change introduced along the dimension of the independent variable. Analysis of Variance In statistics, variance is basically how spread out your data is. If scores across your participants are similar, then the variance is small (as shown by the narrow blue curve) If scores differ vastly from person to person, however, then there is a large variance (as shown by the wide orange curve). Why should we analyse variance when we're interested in comparing means? Suppose a researcher is interested in comparing pain scores after completing three different yoga routines. Here, the researcher can ask themselves two questions: 1. Was one routine more successful than another at reducing pain? 2. Or were the differences just due to individual difference? The first question refers to variance between groups - that is, the difference in pain scores between the three routines. The second question refers to overall variance among individuals - how different were people from one another (regardless of group)? If a lot more of the difference in pain reduction is due to which group you got assigned to, compared to random individual differences, then we say that there is a significant difference between the three groups in the average amount of pain reduction. Analysis of variance ANOVA compares the amount of group variation to the amount individual variation, allowing us to determine if groups are actually different or not, on average. It does so using something called the F-test Just like with a t-test, when we perform an F-test, we’ll obtain a test statistic (in this case, F) and a p-value. The F-statistic is constructed by dividing the average amount of group variance by the average amount of individual variance. One-way between-groups design One-way Analysis of Variance The null hypothesis in the analysis of variance is that the three or more populations being compared all have no differences This basic question about means is answered by analysing the different variances involved. Two types of variance are analysed: Within group (individual) variance - this is unaffected by whether or not the null hypothesis is true. Between group variance - this represents differences between the three groups. One-way Analysis of Variance Advantages: Why not just do loads of t-tests? If t-tests are used repeatedly, they have the potential to lose reliability (think 5%) The ANOVA enables one to compare more than two sets of data. Assumptions of ANOVA 1. Normal distribution of variables. 2. Homogeneity of variance 3. Equal interval measurement at least 4. Independence of observations 5. Equal numbers in each cell (or proportionality) 1. Normal distribution of variables The dependent variable should follow a normal distribution (i.e., there should be no skew present in the data). 2. Homogeneity of variance The variances of the groups must be similar. In an independent-samples t-test and a one-way between-subjects ANOVA, we test for the presence of homogeneity of variances using a test called Levene's test for homogeneity (or equality) of variances. If the p-value yielded from a Levene's test is less than.05, then the assumption of homogeneity of variance has been violated. 3. Equal interval measurement at least The dependent variable (the variable of interest) needs a continuous scale (i.e., the data needs to be at either an interval or ratio measurement). 4. Independence of observations Each group should contain a set of scores which are totally independent of scores in the other groups. In other words, the dataset should not contain data from participants who are in more than one group. 5. Equal numbers in each cell (or proportionality) There should be a (roughly) equal number of participants in each of the groups. If group sizes are very unequal, this makes it difficult to obtain a valid result for the ANOVA. Unequal group sizes is also a threat to the assumption of homogeneity of variance - particularly with large samples. Violations of the assumptions of ANOVA The assumption of equal variance is not a problem unless they are very disparate. As a rule, the largest variance should not be more than 4 or 5 times the smallest when the sample sizes are equal. It may be assumed that the scores are at least symmetrical as opposed to normally distributed in the use of the ANOVA. Violations of the assumptions of ANOVA ANOVA can still be used validly if there is a slightly unequal number of scores in each cell/group. ANOVA cannot be used validly if both (a)heterogeneity of variance (b)unequal sample sizes exist One-way between-subjects ANOVA - example Research question: Is there a difference in frisbee throwing distance between secondary school students, undergraduate students, and postgraduate students? Mean score of the Standard deviation score three groups (M) of the three groups (SD) p-value: Tells you whether or not the assumption of homogeneity of variance has been violated. If p <.05, the assumption has been violated. If p >.05, equal variances is assumed. Levene statistic (F) F -statistic p-value Degrees of freedom: report both between groups and total df. F-statistic F-statistic: Variation between sample means / Variation within samples. The larger the F-statistic, the greater the variation between sample means relative to the variation within the samples. Thus, the larger the F-statistic, the greater the evidence that there is a difference between the group means. Interpreting the results of a one-way between-subjects ANOVA F(2, 29) = 0.35, p =.709 Interpreting the results of a one-way between-subjects ANOVA F(2, 29) = 0.35, p =.709 This is telling us there was no significant difference in frisbee throwing distance between the three groups. Writing up the results of a one-way between-subjects ANOVA Step 1: State the name and purpose of the test. "A one-way between-subjects ANOVA was conducted to examine if there was a significant difference in frisbee throwing distance between secondary school students, undergraduate students, and postgraduate students." Writing up the results of a one-way between-subjects ANOVA Step 2: State the independent and dependent variables. "The independent variable was education level, with three levels: secondary school, undergraduate, and postgraduate. The dependent variable was frisbee throwing distance." Writing up the results of a one-way between-subjects ANOVA Step 3: Report Levene's test for homogeneity of variances "Levene's test for homogeneity of variances was not significant (F = 0.19 , p =.828), therefore the assumption of homogeneity of variances was met." Writing up the results of a one-way between-subjects ANOVA Step 4: Report the results of the ANOVA "Analysis of variance revealed that there was no significant difference in frisbee throwing distance between the three groups (F(2, 29) = 0.35, p =.709)." Putting it all together: "A one-way between-subjects ANOVA was conducted to examine if there was a significant difference in frisbee throwing distance between secondary school students, undergraduate students, and postgraduate students. The independent variable was education level, with three levels: secondary school, undergraduate, and postgraduate. The dependent variable was frisbee throwing distance. Levene's test for homogeneity of variances was not significant (F = 0.19 , p =.828), therefore the assumption of homogeneity of variances was met. Analysis of variance revealed that there was no significant difference in frisbee throwing distance between the three groups (F(2, 29) = 0.35, p =.709)." Post-hoc analysis for a one-way between- subjects ANOVA If there had been a significant difference between the three groups, we would need to conduct post-hoc analysis to find where those significant differences were. Post-hoc analysis: Additional statistical tests conducted after the primary analysis. The post-hoc analysis for a one-way between-groups ANOVA is called the Tukey HSD - we will cover this in week 12. Recommended Reading Chapter 20 – ‘The variance ratio test’ Chapter 21 – ‘Analysis of variance (ANOVA): Introduction to the one-way unrelated or uncorrelated ANOVA’ Thank you! For questions, email me at [email protected]