Year 12 Research Methods Study Guide 2024-5 PDF
Document Details
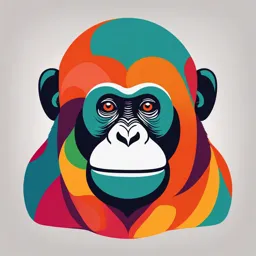
Uploaded by SimplerAllegory473
2024
Dr Doug Cullen
Tags
Summary
This is a study guide in psychology, discussing research methods, ethics, and sampling techniques. The document cover topics like validity and reliability of research.
Full Transcript
**Student name:** Abstract Word Cloud For Experimental Psychology With Related \... **Year 12 Research Methods Study Guide 2024-5** **Dr Doug Cullen 2024** **An Introduction to Research Methods** Half of Paper 2 is made up of research method (including some GCSE level maths questions) and up to...
**Student name:** Abstract Word Cloud For Experimental Psychology With Related \... **Year 12 Research Methods Study Guide 2024-5** **Dr Doug Cullen 2024** **An Introduction to Research Methods** Half of Paper 2 is made up of research method (including some GCSE level maths questions) and up to a third of your total marks (96 marks) across the three papers can be from research methods. Therefore, the research methods topic is the **most important part** of the Psychology A-level. **What does the phrase Research Methods mean?** Psychology is a science, like Biology, Chemistry and Physics, because it uses the scientific method to collect and analyse data. This high quality and reliable data is then used to create theories that are reliable and valid and can make accurate predictions about what should happen in a situation when something changes. You started learning something about the scientific method when you learnt about experiments in science GCSE class. We will learn about the scientific method in detail later in Year 13. In psychology we use a range of different research methods to gather data about how people think and behave and why they act in certain ways. The different methods cover a wide range of techniques, and we will not study all of them in this A-level; however, we will cover the following: Experiments, Observations, (Self-report) Interviews and Questionnaires, Correlational analysis, Content analysis, Thematic Analysis, Meta-analysis, and Case Studies. **\ ** Before we start learning about the research techniques, it is important that we first learn about the guidelines that protect both the participants and researchers when data is being collected and used. In the UK, these guidelines are written by the British Psychological Society (BPS) and can be seen in full on the BPS website. This code of ethics offers guidance on what Psychologists can and cannot do when working with patients and members of the public and what protections participants can expect. The full ethical code is lengthy and is most often simplified into six ethical principles for this A-level. **The six ethical principles/rules:** **Informed consent** -- participants (ppt) must be given all the relevant information for the study *before* they take part in the study (they are 'informed'). To show that the ppt understands and consents to the study and the use of the data that will be collected, and understand the ethical code that is protecting them, the ppt signs what is called a 'consent form' which includes a description of the study, a description of the relevant ethical code and a section where the ppt can write their full name, sign and the date of giving consent. **No deception** -- There is a standard rule that ppts must never be deceived during a study. However, sometimes researchers must deceive participants to prevent the ppt from changing their normal behaviour due to something like *social desirability bias* or the *screw you* effect. If the ppts were told the truth they would likely change their behaviour, and this would damage the quality of the data collected during the study (e.g. the validity would be reduced). In studies where deception is necessary to protect the validity of the study, it is essential that the participants are debriefed (told what really happened and why the researcher had to deceive them). During the debrief the ppts will be told the true purpose of the study, given an explanation about why they were deceived, and they will be reminded that they have the right to withdraw their data from the research. When deception has occurred, there is also an issue with informed consent as the ppt did not consent to the real purpose of the study. **No harm (physical or psychological)** -- Participants should never be subjected to any form of harm during the study. However, some pieces of research cannot avoid harming the ppt because they are investigating things like the body's response to fear or previous harm that the ppt has experienced. Where harm is caused, it must be carefully monitored, and counselling/guidance offered afterwards. Psychological support can be extremely expensive and studies that cause psychological harm are not conducted lightly and will have gone through several layers of checks, including being reviewed by a university ethics board. If harm is unexpectedly occurring during a study, the research should be stopped immediately. **Right to withdraw** -- Participants must be informed that they have the right to withdraw from the research at any time. This is always true, so even if you have spent two hours setting up a piece of research (like an EEG study) and the participant decides that they would like to withdraw before you have finished collecting the data, the study should be stopped. Participants also have the right to withdraw their data from the research after they have completed the data collection part. When you have deceived your participants, you will need to remind them during the debriefing that they have the right to withdraw from the study now that they know the true purpose of the study. **Confidentiality** -- The participant's name must never be published. Instead of using a participant's real name the psychologist will use a pseudonym (fake name) or initials, or even a number. It is exceedingly rare for a participant's real name to be used and only occurs when they have given their permission, or they have passed away. An example of a participant's real name being known by the public is the case of Henry Molaison who was known as HM in the medical literature before his death. **Privacy** -- researchers should only collect data that they have specific consent to collect. Psychologists must not invade the privacy of their participants and collect data that has not been consented to by the ppts. This is remarkably similar to the GDPR rules that are included in apps and on the internet and, depending on the nature of the infraction, a psychologist who invaded the privacy of a ppt could be at risk of prosecution. **Dealing with ethical issues** **Breaking the informed consent rule:** If a researcher needs to break the informed consent rule to prevent damage to the study, it is important that the ppt is told as soon as possible. When **deception** has occurred the ppt should be debriefed at the end of the study. In a debrief the ppt is told the truth and asked if they want to keep their data in the study and a full consent will be taken. **Children under 16** cannot give consent to take part in a study. If the researcher needs to collect data from ppts under 16 years of age they will need each child's parent's permission. You do not need to collect consent from participants who are being observed in the normal world going about their everyday lives, for example, on the street or in a shop. Anywhere that you could expect others to see you that is not a private space, the researcher will not need to collect consent. Sometimes you cannot collect consent because it is impossible or doing so would damage the research. In these cases, it might be possible to collect **presumptive consent**. In presumptive consent, you find a similar type of person to your potential ppts, and you ask them if they would be happy to take part in the study if it was run on them. If the person says that they would be happy to take part, you can **presume** that the actual ppt would also consent. **Emotional harm** If emotional harm occurs, the ppt will need to be supported with counselling or therapy to ensure that they are able to return to where they were mentally before the study took place. Therapy and counselling can be expensive, so researchers need to include this in their budgets for the study. **Confidentiality and privacy issues** See the main ethical rules section **TOPIC 2: the different research methods used in Psychology** You need to know the strengths and weaknesses for each research method and be able to plan a piece of research using each research type. **Overview of the different methods for collecting data in Psychology** **Experiments** look for a difference between groups or conditions. A common difference is exploring the effectiveness of group collaboration against individual performance. **Observations** are used to study human behaviour in different situations. For example, observing infant responses to their primary carer leaving the infant in a strange environment. **Correlations** look for relationships between linked variables, for example, the weight and height of the same group of ppts. **Content analyses** investigate the number of times distinct categories occur in recorded information. **Self-report or survey methods include questionnaires and interviews** and allow psychologists to investigate people's thoughts, feelings, and opinions. **Thematic analysis** is the only technique that analyses qualitative data and leaves it as qualitative. It is similar to analysing a book in English Literature. **Case studies** allow in depth detailed study of a single individual, who often is displaying some form of unique behaviour or brain damage. **Meta-analyses** allow a researcher to combine the results of several different studies and reanalyse them all together. This gives greater power to the research because there are many more participants included in the statistical test. **Longitudinal** studies show changes over an extended period of time, for example the impact of childhood neglect on adult relationships and mental health. **\ EXPERIMENTS** **Experiments** are especially useful research tools, as they allow a researcher to determine **cause and effect.** Because the researcher controls every variable and only changes one variable (IV) at a time, the researcher can conclude that any change in the variable being measured (DV) must be because of the manipulation of the independent variable. *Example of cause and effect*: Researchers might want to determine if administering a certain type of medicine leads to an improvement of symptoms. In a simple experiment, participants are randomly assigned to one of two groups. One group is given the medicine, and the other group is given a placebo (control, sugar pill). If the experimental group (medicine) improves more than the control group (placebo), the researcher concludes that the manipulation (medicine versus placebo) caused the difference. **Laboratory (lab) experiments** take place in a controlled environment where all the potential variables are under the control of the experimenter. The variables include noise, light, temperature (if relevant), people coming and going, equipment (computers, monitors, recording devices), medicines, etc. **Note:** validity = testing what you intended to test **Field experiments** have variables and are manipulated by the experimenter; however, instead of taking place in a lab they take place in the real world. **Natural experiments** are not 'set up' by an experimenter. They are situations that occur naturally and are only measured by a researcher. **Quasi-experiments** occur whenever the researcher is using an independent variable that exists naturally. The most common quasi variables are gender and age. However, any comparison where the IV is something that already exists and the researcher could not allocate the ppts to a group is a quasi-experiment. Any study that includes a comparison of males versus females is a quasi-experiment. **Note**: Quasi-experiments can occur in a lab field or natural experiment, the key thing to look for is if the IV is a comparison of a naturally occurring variable. For example, the correct term for a lab experiment with a quasi IV is a 'quasi-experiment based in a lab,' you could also call it a 'quasi-lab experiment.' The most common areas for quasi questions are gender, age (old versus young), ethnicity, race, and intelligence. -*strengths*: the only way to study naturally occurring IVs. -*Weakness*: the researcher has lost some of the control by using a quasi IV. This means that in some ways it is not considered a true experiment. Some researchers believe that this means that quasi-experiments lose the ability to make cause and effect conclusions. **Cause and effect** A particularly important concept in psychology is whether the researcher has taken enough control of the variables in a study to say that the study has cause and effect. When a researcher claims that they have cause and effect they are saying that they are very sure that the manipulation of the IV was the cause of the change in the DV and that any changes are not due to other uncontrolled variables. This claim can only be made when the IV, DV and extraneous variables (what you called control variables at GCSE) are fully controlled. Lab and field experiments are the only types of research that take enough control to allow a cause-and-effect conclusion to be made. **Extraneous variables** **Extraneous variables** are things that should be controlled to make the experiment reliable and valid, for example, temperature, lighting, and noise. In some research, the Psychologist might even go as far as to control things like the amount of food eaten before the experiment or the amount of sleep a participant has had. A failure to control important extraneous variables (EV) could mean that the EV changes the DV instead of the IV. If the EV does have an impact on the study, there will be a loss in validity. Losing validity is a significant issue because it means that the research has failed which is an expensive waste of time, resources, and money. **Confounding variables** occur when the researcher has failed to control a variable that has impacted on the DV. Confounding variables 'confuse' the control and outcomes of the study so that it is not clear if the manipulation of the IV or the uncontrolled confounding variable was responsible for the change in the DV. Confounding variables damage the validity of the experiment and mean that the research result cannot be trusted which is a waste of time, resources, and money. Researchers running experiments spend a lot of time making sure that they have controlled every variable that might impact the DV instead of the manipulation of the IV. **EXPERIMENTAL DESIGNS: RIM** **Repeated measures design**: In a repeated measures design there is only one group of participants, and each participant completes all the tasks. For example, a participant's reaction time for pressing a button (DV) to both angry and happy faces (IV) is recorded and then a comparison is made between the reaction times for each condition (angry versus happy). *Strengths*: there are no participant differences because each participant is compared with their own performance on each of the tasks. This design can be quicker and cheaper to run because you need fewer participants. *Weaknesses*: Because the same participants complete more than one task there may be an issue with **order effects**. There are three order effects that you need to learn for the A-level: **boredom, fatigue, and practice**. For example, boredom might make a participant perform poorly because they are not focused on the task; fatigue could make the participant perform poorly because they are tired; and practice could make the participant perform better on the second task/condition. Each of these order effects would reduce the validity of the study. *Dealing with order effects*: Order effects can be controlled through **counter balancing.** When a researcher counterbalances, they split the group of participants in half and ask one half to do task A and then task B while the second half of the group complete task B and then task A. Then you put the data from the two halves together and analyse it as one. Although you have not removed the order effects completely, you have balanced the impact of the order effects within the study. **Independent groups design**: In independent groups design there is more than one group of participants, and each group completes just one of the tasks. All experiments comparing males and females are independent groups. *Strength*: there are no order effects because each participant completes just one task A larger number of participants are required because you need different ppts for each task. **Matched pairs design**: The researcher carefully chooses students who can be matched into pairs on relevant variables to the research. For example, the participants might be matched together on their reading age. Each member of a pair completes one of the tasks. The researcher then compares the performance on task A with the performance on task B and treats the two ppts as if they are the same person (because they have been matched). *Strengths*: there are no individual/participant differences AND no order effects. This method has both strengths of repeated measures and independent groups. **Pilot studies** A **pilot study** may be conducted to ensure that the experiment works and that the variables are well controlled. If the pilot study highlights any issues, the experimenter will change the experiment to make sure that it is well controlled and that it is working as expected. A pilot is the first thing that guides the way. When drilling holes in wood or a wall, it is sensible to first drill a pilot hole in the correct position. This makes it easier to drill a larger hole, which is harder to do, in the correct place. The pilot hole will also identify any issues with drilling in that position. In psychology, pilot studies do the same thing as when drilling a hole. They help to make sure that the study is heading in the right direction and check to see if any changes need to be made to the design or methodology. For example, improving the instructions, changing the recording of data technique, changing any stimuli. **TOPIC 3: Hypotheses** A key skill in Psychology is being able to write clear and specific hypotheses. You should remember something about hypotheses from your GCSE science lessons and, in this A-level, you will take the next step in understanding the theory behind hypotheses, how they should be written for each type of research and identifying the distinct types of hypotheses that can be used in research. A hypothesis is a statement predicting what is expected to happen in a research study. They are never written as a question, and you will be awarded zero marks if you do write a hypothesis as a question. Hypotheses help to control the research and organise what should happen. Every scientific study conducted across the planet will have a hypothesis (or several hypotheses) that the researcher is testing. Once the data has been collected from the research it will be analysed to see if the results support the hypothesis enough to conclude that the hypothesis is trustworthy. You need to be able to write hypotheses for experiments, correlations, and observations. You will also need to be able to identify and write directional, non-directional and null hypotheses (more about these later) Below is an example of an experimental hypothesis, it has been fully operationalised which means that the variables and expectations have been clearly stated. **Example experiment hypothesis** "Female participants will score significantly higher scores on the IQ test compared to male participants" A hypothesis describes the connection between the variables and the expected outcome. In this example, the **independent variable** (the thing that is being manipulated) is the gender of the participants. The **dependent variable** (the thing that is being measured) is the IQ score. The outcome that is predicted is that female participants will do better than male participants. Note: the study is a quasi-study because the IV is naturally occurring. Operationalising is a skill that you will develop throughout the course as you write more variables and hypotheses you will get used to writing them clearly and stating what they are and what you expect to find in a specific way. **Directional hypotheses** A directional hypothesis states exactly what the researcher expects to find, including the pattern of the results. In the example above the researcher has stated that they think that the female participants will score higher than the male participants on an IQ test. This is a directional hypothesis, as it states exactly which group will be better than the other. A directional hypothesis is used when there is **previous research** that indicates the direction that the results will take. **Non-directional hypotheses** A non-directional hypothesis states that there will be a difference but not exactly where that difference will be. If the example hypothesis is re-written to be directional it would be written as follows: "There will be a significant difference in the IQ test scores between male and female participants." This time the hypothesis states that there will be a difference, but it does not pinpoint exactly what will happen. A non-directional hypothesis should be chosen when there is no previous research available to the researcher. Researchers usually prefer to use a directional hypothesis because it makes it easier to test the trustworthiness of the data collected. This is something that we will study in year 13. **Null hypotheses** A null hypothesis is the opposite of the experimental (or alternative if you are not running an experiment) hypothesis. Researchers are meant to include a null hypothesis for two main reasons: 1. The null covers the probability that the hypothesis is incorrect 2. The null helps the researcher to stay objective by adding extra distance between themselves and the experimental hypothesis If we return to the same example already used "Female participants will score significantly higher scores on the IQ test compared to male participants" It can be re-written as a null hypothesis by changing the words slightly. Instead of making a prediction of what will be found in the research the null hypothesis states that there will be nothing found in the research. "There will be no difference in the IQ test scores between male and female participants." When we analyse data (something we will come back to in year 13) we use statistical tests to tell us how trustworthy our data is. If we find that our data is trustworthy, we can reject the null hypothesis and say that we have found something. If we find that our data is not trustworthy, we must accept the null hypothesis, and we would have to say that we did not find anything. Note: the whole purpose of scientific research is finding new data that helps to explain the world around us and to then share that data so that other scientists can use it to make better predictions about what will happen in different situations. If a piece of research is shown to be untrustworthy the scientist will not publish it because they have nothing to share with other researchers to help improve predictions. **The steps that you should go through when writing a hypothesis** So far, we have looked at experimental hypotheses and you also need to be familiar with correlational and associational hypotheses. **Correlational hypotheses** are different to experimental hypotheses because you are looking for a relationship instead of a difference. This means that a correlational hypothesis must predict that there will be some form of relationship between the two covariables. Correlational hypotheses usually start will the words "There will be a relationship between... ". For example, "there will be a **relationship** between the height of participants in cm and their UK shoe size" If we have previous research that suggests the direction of the hypothesis then we can use a directional correlational hypothesis. To write these we add either the word positive or negative to describe the type of hypothesis we expect to find. For example, "there will be a **positive relationship** between height of participants in cm and their UK shoe size." Sometimes researchers look for an association between different unrelate categories. This is different from a relationship, which is why we use the words association. We will learn more about these in Year 13 as they do not come up during year 12. The Chi Square statistical test is a test of association or difference and compares distinct categories. For example, "there will be an association or difference between birth order (first of second) and career choice (academic or vocational)" **OBSERVATIONS** **Observations** are an excellent way to directly identify and record human behaviour. There is no independent variable, so they are not experiments. They can take place in a lab or in the real world (natural). Observations are a particularly effective way at recording complex human behaviour, for example, how a child responds to their mother, or how ppts act in a pretend prison. Observations are made up of a combination of design factors and observations can be described in terms of which factors have been chosen **Design factor one: Lab versus Natural** 1. **Lab observations** take place in a lab and allow the researcher to control the environment and manipulate some of the variables a. Strengths: high control and often easier to record the information. b. Weaknesses: lacks ecological validity 2. **Natural observations** take place in the real world c. Strengths: they are very realistic; you can study behaviours that would not be possible in the lab d. Weaknesses: there is low control, they are harder to replicate. **Design factor two: Participant versus Non-participant** 1. **Participant observer**: where the observer actively participates in what they are observing. a. **Strength**: they will first hand see the fine detail involved and they will experience the emotions involved in the activity b. **Weakness**: it is hard to see the whole picture because they are involved at a fine detail level. 2. **Non-participant observer**: where the observer does not participate in the activities they are observing. c. **Strength**: they will be able to see the whole picture more clearly, d. **Weakness**: they will miss the fine detail and may not fully understand what is happening from the participant's perspective. **Design factor three: Covert versus Overt** 1. **Covert observation**: where the researcher is hidden and cannot be seen -- this means the people being observed should behave more naturally because they do not know that they are being observed. There is an issue of consent with covert observation because the ppts do not know that they are being watched. If the observation is happening in a public place, you do not need to collect consent because it is expected that other people might be watching you in a public space. 2. **Overt observation**: where the researcher is in the open and people know they are being observed. The participants may change their behaviour because they are being observed, so there is a risk of the **Hawthorne effect** and **social desirability bias (SDB)**. ***Recording data/information during an observation -- sampling data*** It is impossible to write everything down during an observation because as you look down to write you could be missing an important behaviour. Observers have two options in the way that they sample the data during an observation. **Option 1 -- Episodic sampling** -- behaviour categories are created and then each time one of the behaviours is seen it is recorded in a table/tally chart. **Creating behaviour categories** Behaviour categories must be created carefully to make sure that the observation is valid and that it is easier to record the data if episodic sampling is being used. The rules for creating behaviour categories 1). Exhaustive -- cover all the categories of interest. 2). Mutually exclusive -- there should be no overlap between the categories. A behaviour should fit into only one category. If the categories are not mutually exclusive (i.e. they overlap) the observer will not know which category to tick off on the tally chart and the observation will lose both reliability and validity. 3). The categories should also be clear and easy to use. **Example Tally Chart** Child Punching Kicking Slapping Pushing Spitting ------- ---------- --------- ---------- --------- ---------- 1 III I IIIII II I 2 I II III I II 3 **Option 2 --** **Interval sampling** -- time intervals are chosen before the observation (e.g. once every two minutes). An alarm or flashing light can be set to remind the observer to write things down. Then whatever is happening when the alarm goes off is written down. When the researcher cannot choose behaviour categories, they use interval sampling instead. **Note: Pilot studies** -- a pilot study can be conducted to make sure that the categories chosen are appropriate and that the observers are able to achieve an elevated level of inter-observer (or inter-rater) reliability. **Checking reliability in an observation** **Inter-observer (or inter-rater) reliability** -- Usually more than one observer will conduct the observation so that the reliability (consistency) between the two observers can be checked. Inter-observer reliability is checked using a **correlational analy**sis where each observer's scores are the covariables. There should be a strong positive correlation between the two observers. The **correlation coefficient** value expected is at least **0.8** (approximately, at least 80% similar). The exam board like asking questions on how to check interobserver reliability as it means they can test observations AND correlations in the same question. The key thing to remember is that the reliability of the observation is tested using correlational analysis. **CONTENT ANALYSIS** **Content analysis** (a form of indirect observation). Is a useful technique that is quite common in some areas of Psychology. The researcher chooses a content analysis when they need to find out the common categories contained in something that has been recorded. It can be used on every type of recorded media (newspapers, magazines, music, films, TV, interview answers, questionnaires). If a researcher were able to gain access to the diaries of people who had become anorexic, it could be possible to look for common categories described in the diaries to give a better understanding of the development of the illness. ***Steps to run a content analysis*** 1. Select the media (e.g. adverts, diaries) you will study 2. Look through the material to identify the most useful categories 3. Carefully create your categories (as you do in a controlled observation). The rules of *cover everything, no overlap,* and *be specific* apply to the categories. 4. Then create a tally chart to record the number of times an example of each category is found 5. Then the results are analysed and compared. If you have quantities and categories, you could analyse the results using a chi square test of association or difference. Reliability can be tested by repeating the content analysis with the same categories and the same material (this is called **test-retest**). **Inter-rater reliability** can also be tested by asking another researcher to conduct the same content analysis with the same categories and the same material. If the second researcher finds the same number of items in each category you can say that the study has reliability. **THEMATIC ANALYSIS** **Thematic analysis**: is where the main themes in written and recorded information are identified and summarised: remarkably similar to identifying the main themes in a novel and authoring a report on the main themes. You listen/read all the material and identify any main/key themes that run through the responses. Examples and quotations are used to support the themes you have identified, to help others, read about your study without needing to listen to/read all the interviews. The findings are written up in a report where each key theme is described and then supported with quotes from the sources. Thematic can be repeated by other researchers quite easily. However, as the process of thematic analysis can be very subjective there is often a lot of bias in the final report. To help deal with this subjective bias, researchers who use thematic analysis often include a short statement at the start about their own beliefs and viewpoints so that the reader can place the report into the context of the researcher. **CASE STUDIES** **Case studies** are rich in detail and can be used to study individuals that it would be unethical to study because you would need to harm them to create the study. For example, HM's brain damage could not be ethically created for a study, but researchers were able to study him after the damage accidently occurred during an operation to cure his epilepsy. Case studies are also useful because they can be used to investigate something that it would be impossible to set up in a laboratory, for the sheer lengths of time involved or resources required. A problem with case studies is that the participant involved is often unique. This means that data collected from them might not be generalisable to other people and we need to be careful with any comparisons we want to make. There are several important case studies in the A-level, and it will be important that you learn the details of the case study as well as how to evaluate it. Broca, Wernicke, Freud and Scoville all wrote case studies that play a significant role in what you need to learn for Psychology A-level. **STUDIES USING CORRELATIONAL ANALYSIS** **Correlational studies** are the only research method to show the strength of the relationship between two covariables. Correlations are also useful when it would be not possible or unethical to run an experiment (e.g. the level of stress felt after the tsunami in Japan and how close an individual lived to the Fukushima nuclear reactor). Correlational studies are also used to check inter-observer reliability. There are some key terms that you need to familiarise yourself with in terms of correlations. If you do not make sure that you have learnt these terms off by heart you will struggle as we continue through the course. - Covariables: a covariable is the term used to describe a variable in a correlation. We cannot use the terms IV and DV in a correlation because there is no cause and effect of manipulating the IV on the measurement of the DV. Instead in a correlation the two covariables change at the same time. If the two variables change in the same direction it is called a **positive relationship**, for example the height and weight for a group of people mostly changes in the same direction, the taller you are the more you will weigh. In contrast if the covariables change in opposite directions it is called a **negative relationship,** for example as smoking cigarettes increases average life expectancy decreases. - Correlation coefficient: A correlation coefficient tells you how strong a relationship is. The values can be between -1 and +1. Quite often a correlation coefficient uses the letter "R" to indicate that a relationship strength has been calculated. R=-1 represents a perfect negative correlation where as one covariable increases the other covariable decreases by the same amount. When R=+1 it represents a perfect positive relationship where one covariable increases at the same rate as the other covariable. When R=0 there is no relationship at all. - Scattergraph/plot: A scattergraph is used to display a relationship between two covariables. If the graph slopes up to the right, it is a positive relationship. The closer to a line the dots are the stronger the relationship. The figure below shows a positive relationship (it slopes up to the right). The data points are also close together and the R value is around R=0.7. The figure below is another example of a correlation and this time it is a negative correlation as it slopes down to the right. The data points this time are closer together and this suggests a stronger correlation. It is likely that the R value will be about R=-0.9. The final example of a scattergraph for a correlation shows datapoints that are nearly no correlation. You can see that datapoints are not forming any sort of line and that they are all spread out. The R value would be approximately R=0. The correlations discussed so far have been linear correlations, this means that they form a straight line when there is a correlation. Sometimes the correlation does not form a straight line and instead it forms a curve, we call these curvilinear correlations. These are mentioned on the specification but have not appeared in detail in the exams yet. If we describe a curvilinear relationship, we say that as one covariable increases so does the other, to a peak and then as that covariable decreases so does the other. Strengths: Correlations can be cheaper than experiments because you can often use secondary data. They can also be copied by another research very easily (we call this replication) which makes them easy to check for any mistakes. Weaknesses *-* A weakness is that correlations do not show cause and effect, although they are often misused by people (especially politicians) to show cause and effect. There can also be other 'intervening' variables (additional variables) which have really been the key factor in the relationship. For example, there is a relationship between the amount of day light and the number of ice creams sold at the beach. The intervening variable, and much better predictor of ice cream sold, is temperature. Temperature is the covariable that is actually linked with ice creams sold and not the amount of day light. Researchers must be careful to make sure there is a clear link when they talk about a relationship between two covariables. For example, height and weight can be correlated for a group of people because each person provides a measurement for height and weight and therefore there is a link between the two covariables. **SELF-REPORT METHOD (SURVEYS): QUESTIONNAIRES AND INTERVIEWS** Questionnaires and interviews are often called the **survey** method because you are using questions to "survey" the beliefs and experiences of other people. This approach to gathering information is the best if you would like to collect participants' opinions, ideas and thoughts about themselves and the world around them. When we create a questionnaire, we create a **schedule** (a list) of questions that the participant answers. The questions can all be the same format, or they can be made up of several types of questions, for example, open questions and closed questions. A schedule of questions can be any length, but usually you aim for the questionnaire to be finished within 30 minutes and a maximum of an hour. **Strengths of questionnaires:** they can be given to a lot of people very quickly and they are cheap to produce. They are also easy to repeat which means that they can be checked for replicability. Also, because questionnaires can be filled in anonymously (you do not need to give your name) participants are often more likely to be honest about personal or embarrassing information (in an interview they may be influenced by **social desirability bias**). Questionnaires, compared to interviews, also require less training and experienced researchers. A **weakness of questionnaires** is that response rates (the amount you get back) to questionnaires can be quite low (often about 30%) and those people who do respond can be a biased sample. People who respond are likely to have more free time or have a personal interest in the topic. This means that it can be hard to generalise the results of a questionnaire because it may not represent most of your target population. Lastly the researcher cannot ask any follow up questions because the participants are anonymous. **Note**: Whenever you use questions to gather information you need to make sure that the questions are not leading or ambiguous. Leading questions give away the answer you are looking for in the question. Ambiguous questions occur when the question has two meanings and therefore two possible answers and you had only planed for one answer. **Interviews** are like questionnaires, but they occur live and in person. They can be structured, semi structured and unstructured. - Structured interviews are quicker to run and need less training it is like asking a person the questions from a questionnaire instead of the person reading them. They are easier to replicate and check test-rest reliability than unstructured interviews because you can easily hand out the same questionnaire again. However, a **weakness** with structured interviews is that there is a set list of questions in the schedule which means that you cannot ask follow-up questions. - Unstructured are hardest to run of the three types of interviews as you need more training to get them right. It is easy to not have asked the right questions because there is no set list of questions. After a first question the whole interview is made up of follow-up questions. To ask the right follow-up questions takes a lot of expertise and subject knowledge and the interviewer will need to be very experienced. The main weakness of this type of interview is that it takes a long time to analyse the information that has been collected. Often it takes 10 times longer to analyse the interview than the interview itself. This can make the process of interviewing using unstructured interviews time consuming and expensive. A second weakness is that each participant will answer in a unique way which can make comparing results between participants and other interviews difficult. A third weakness is that there is a higher chance that Social Desirability Bias will influence the results as the participant will try to look good (as positive as possible) in front of the interviewer. - Semi-structured interviews (also called clinical interviews) have a schedule of questions and then use unstructured follow up questions. They also require a lot of training to run. However, in contrast to unstructured interviews they tend to be easier to compare the answers between participants because there is a schedule of questions at the start. **Note:** follow up questions are when you add an extra question into your interview to gain more information after the participant has said something of interest. For example, "that is very interesting, can you tell me more about why you think that?" **Types of questions for interviews and questionnaires** The exam board often ask students to create example questions that could be used in an interview or a questionnaire. There are two main types of questions that you need to be familiar with and be able to write in the exams. **Closed questions** A closed question collects quantitative data and is either a simple yes/no answer, a section of different options or a numerical answer. Closed questions often have an instruction to tick, cross out or circle the correct or most suitable answer. *Example of a simple yes/no question* Do you own an Xbox yes/no (circle the correct response) *Example of a different options question* Do you currently own any of the following (please circle each console that you currently own) Xbox Play Station 4 Nintendo Wii Nintendo Switch *Example of a numerical question* How many lessons do you have on a Friday? *Example of a different choices question* How many Xbox games do you currently own? Less than 5 6-10 11-20 21 or more Closed questions can also be statements with a scale, many of these are **Likert** scales where there is a statement that the participant must decide how much they agree with. **Example of a Likert scale:** I prefer the PlayStation over the Xbox 1 2 3 4 5 Strongly agree neither disagree Strongly Disagree agree or disagree Disagree *A strength* of closed questions is that they produce quantitative data which can be easily analysed. However, *a weakness* is that participants who complete the questionnaire may have had to choose an answer they did not really agree with. **Open questions** In this type of question, the participant is asked for their own thoughts, feelings, and beliefs about something. There is no set answer, and the respondent can provide as much information in their answer as they want (this is why they are called "open" questions) *Example of an open question* Please describe how do you feel about coronavirus *A strength* of open questions is that they provide more detail than closed questions AND allow participants to say what they want. They allow the participants to be more flexible in their answers. *A weakness* is that they are harder to analyse because they include a wide range of beliefs, ideas, and opinions between the different participants. Another problem with this approach is that it can take an exceptionally long time to analyse the data for open questions. A final problem with this approach is that there is a risk that the researcher will bias the results through their own interpretation of what the participants have said. When a researcher allows their personal beliefs to influence the results, we say that they have been subjective. **Creating a schedule of questions** The researcher needs to carefully identify the aim of the questions and what type of data they need to have at the end of the study. If they need the data quickly and in a format that can be easily analysed, then it might be best to use a set of questions that are closed. If detailed answers which reflect the participants true opinions and beliefs are needed, and there is not a rush to get the data analysed, then open questions would be more useful. When the researcher has chosen the style of questions, they need to consider how many questions to include. You need to make sure that participants do not get too bored answering the questions because if they do you might start to see the "screw you" effect occur. This is most obvious when participants start writing ridiculous answers or obviously scribbling all over the questionnaire. If the questionnaire is online, they might just keep pressing the same response key rapidly. **Checking the validity and reliability of the data collected through the survey method** **Validity** means that you have collected the data that you intended to collect. In a questionnaire the validity can be assessed in different ways. The first is through **Face Validity**. If the questions "look" like they are testing the right thing "on the face of it" then they can be said to have Face Validity. This is a first quick check of a set of questions. A second check of a new schedule of questions is called **Concurrent Validity** which is where you compare the new questions with a similar questionnaire that already exists. If the new questions are valid, you should get equivalent results to both questionnaires for each participant (if they complete both). Reliability means that the study and the data collected are **consistent** -- this means that the results all test the same thing and that there are no random differences in the responses given. A way to test if a schedule of questions is reliable is to give it to the same participant twice. This is called **test retest**. You test the participant once and then a short while and then test them with the same thing again. If you get comparable results, you can say that the test is reliable. Another way that you can test the reliability of a schedule of questions is to use the "**split half**" method. In this approach to checking reliability the researcher asks a participant to answer the questions. The researcher then compares the score/answers on the first half of the questions to the score/answers on the second half of the questions. If a schedule of questions is reliable (consistent) there should be similar scores/answers in the two halves. For example, if a questionnaire assesses aggression and the first half scores the participant as 'very aggressive' you would expect that the second half would also score the participant as 'very aggressive.' **Meta analyses** Meta analysis = a larger than usual analysis, a beyond normal analysis of data. Meta analyses are a useful way of summarising many similar studies into one single study. The researcher selects as many relevant previous studies as they can find. They take the data from each study and carefully put the data together to build one large (meta) data set. This meta data set is then analysed. By using a meta data set, the researcher can include far more ppts than they would be able to from their own research. Often meta analyses include thousands of ppts and allow the researcher to study any trends or patterns that are not visible in a single study. *Strengths*: excellent at showing patterns and trends on a large scale. Allow large numbers of studies to be joined together giving greater power to the results. Because this technique uses secondary data from other researchers, it is much cheaper than running a single study of the same size with the same number of ppts. *Weaknesses*: Meta analyses can be hard to do because you are fitting data together that was never intended for this purpose. Secondary data can be difficult to use in a valid way because each original researcher had a different aim and so the data might not fit exactly with the intended outcome of the meta analysis. **ADDITIONAL RESEARCH METHODS** **Longitudinal studies**: take place over a long time, usually months or years but sometimes decades. These track changes over time and can help to show the impact of things like a bad childhood on later adult behaviour. Longitudinal studies use the other methods to collect data. For example, you might give the same participants a questionnaire once a year over a decade. **Reviews:** In a review the researcher collects all the previous studies on a topic and summarises them in one large article. They do not analyse the data of the previous studies they just examine the research methods used, and the conclusions drawn by the previous researchers. The purpose of a review is to simplify many previous studies into one more accessible and quicker to read paper. **TOPIC 4: Participant sampling techniques** **Why do we sample?** Sampling is important because there is not enough time to collect all the data that might be needed to truly explain something. For example, if we need to collect data from schizophrenics in the UK we would need to collect data from approximately 700 thousand individuals. It would take too long to collect data from all of them, so we need to find a way to collect data from a smaller number of people. The small number is called a "sample." When we use sampling to collect data, we hope that the sample will represent the target population (the target population is the group of people that we want to study). **Representative** samples are like the target population. If a sample is not representative, then the data we get from the sample is not going to be valid (we will not have measured what we intended to measure). There are five sampling techniques that you need to know for the exams and for each technique, you will need to know the strengths and weaknesses of using that technique. Note: You need three marks of detail for each to make sure you can answer any question. **Random**- The easiest way to get the marks in the exam is to use a random number selector app. You give each member of the target population a number. Then you put the list of names with their numbers into the random number selector and ask the app to choose the number of participants required. An alternative method is to pick names out of a hat. You must explain that names out of a hat must be made completely equal. All the names of the target population should be included. The pieces of paper the names are written on should be the same size, folded in the same way, and the box covered and shaken between each pick of a name. **Opportunit**y -- The participants will be selected from who is present at the time the researcher is looking for ppts. You must consider **when** and **wher**e the sample is going to take place. If you do not select a range of times and places you can have a biased sample. **Volunteer**- You need to fully explain how you are going to advertise to your participants. The adverts can be posters, in newspapers/magazines, emails, or on social media like Facebook. For example, if you need a sample of medical doctors you could advertise in doctors\' magazines and put posters up in hospital staff rooms. This technique was used by Asch, Zimbardo, and Milgram in their research. **Systematic sampling** -- sometimes random sampling would take too long to run and is not possible, so the researcher decides to pick every 10^th^ person instead (or every 5^th^, 100^th^ whatever fits the population best). This is no longer random sampling as you are using a **system,** and everyone therefore no longer has an equal chance of being selected. **Stratified sampling** -- is especially useful if you need to obtain a sample with similar proportions of groups within the target population, for example, the correct proportion of males and females. You calculate the ratio of each group and then select through random sampling the correct number of participants. For example, if a workplace had 100 people (60 men and 40 women) and a stratified sample was needed for a sample of 20 participants. You need to calculate the correct number of males and females needed for the sample. One way is to divide the total population by the sample size 100/20 =5. You then divide the number of males by 5, 60/5=12, and the number of females by 5, 40/5=8. This means the stratified sample needs 12 males and 8 females. Then you select the number needed for each group using random sampling. It is important in the exam to make it clear that you know that there will need to be a separate list of participants for each group and that you will use random sampling to select the participants from the list. **Snowball sampling -- (not on the specification but useful to know)** this is used when it is difficult to find participants because what you are studying has strong social issues. For example, studying heroin addiction can be difficult because addicts tend not to respond to adverts and doctors must respect their patient\'s confidentiality -- which means a GP cannot give you a list of drug addicts they see. Add to this the fact that drug addicts often do not have a fixed address and may not have anyway to be contacted by researchers. In this sort of situation, a snowball sample is especially useful. The researcher meets one drug addict and gains their trust and asks them to take part in the research project. This first drug addict then introduces the researcher to other drug addicts. Then these new drug addicts introduce the researcher to more addicts. This process continues and the number of participants grows like snow sticking to a snowball when it is rolled across the ground. **Generalising from a study** The generalisability of a study is important because researchers (and people using the results of a study, like NHS policy makers) often want to generalise the results of the study from the target population to another group of people. Sometimes a study is generalisable, this means that it is possible to use the results to explain and predict the behaviour of a wider group of people. For example, a study may have used participants from a target population of 18- to 21-year-olds. The study could be generalised (used to also explain) the behaviour of 22-25 years old without a loss of validity. However, it would be problematic to generalise a study on 18--21-year-olds to the residents of elderly care homes across the UK. The larger the sample and the wider variety of people included in the study the more generalisable the results of the study will be. Many studies in psychology have used degree students and this is a problem for the generalisability of the information that has been gained within the discipline. **TOPIC 5: descriptive statistics** Descriptive statistics describe data. They are used to help us understand any patterns in the data. They are a way of simplifying the total data set, so that our brains can understand what we are looking at. Data can be 'described' in terms of what the middle of the data set looks like. It can be 'described' in terms of how spread out the data is. Data can also be turned into diagrams, tables, and charts to make the patterns more visible. **Quantitative data**: is numerical data. Often in the form of frequencies or the use of a scale **Qualitative data**: is written information, it is usually thoughts, feelings, and opinions. It is richer in detail than quantitative data. **Primary data**: data the researcher has collected themselves for their own purposes. It has high internal validity because you have measured what you intended to measure. However, primary data is expensive and time consuming to collect. **Secondary data**: data that already exists because someone else has collected it. The advantage is that it is quicker and cheaper to use secondary data. The problem is that the data may not be valid for what you want to use it for. **Measures of central tendency: Mean, Median, and Mode** The measures of central tendency are used to describe what the centre/middle of the data looks like. The *mean* tells us what the average of the data set is. The *median* is the number in the middle of the data set, if you put the data values into order from smallest to largest. The *mode* is the most common number in the data set. *Mean* - add all the values up and then divide by the total number of values *Median* -- put the numbers into size order and find the middle value. If you have an equal number of values (e.g. 2, 2, 4, 6, 6, 6) then it is the average of the two middle numbers. In the example, in the brackets, the median would be '5'. *Mode* -- count the frequency of every value and find the most common one. **Measures of dispersion: Standard deviation and Range** The measures of dispersion are used to describe how spread out the data set is. The more spread out the data set the higher the measure of dispersion values will be. *Standard deviation (SD)* -- is far each data value is from the mean value for the data set (OK, I have simplified this a lot, but that is the basics. If you would like to know all the steps for calculating the SD, ask me or google it.) *Range* -- this is the highest value minus the lowest value. The difference between the highest and lowest values is quite easy to calculate **NORMAL DISTRIBUTIONS** One of the most important concepts in psychology is the normal distribution. These are quite common in psychology and when we plot the frequency of things like height, weight, and IQ we can see that the data forms a normal distribution (ND). The ND is also called a Bell curve because of the shape of the line. The figure below shows the ND for male and female heights. Note that the frequency is on the y axis. The properties of the ND are always the same: the mean median and mode are all at the peak; The two sides of the ND are symmetrical around the mean; there is a tail on either side. The ND can be measured using the standard deviation. One standard deviation either side of the mean covers approx. 68% of the distribution and 2 SD either side of the mean covers 95% of the distribution. **POSITIVE AND NEGATIVE SKEWED DISTRIBUTIONS** In addition to NDs you also need to be familiar with skewed distributions. In a skewed distribution one of the tails is longer because there are extreme values on that side of the distribution. The two sides of the distribution are no longer equal which means that they are not symmetrical. A negative skew is also called a left distribution because the distribution is stretched to the negative or left side of the axis. A positive skew is also called a right skewed distribution because it is stretched to the positive or right side. The mean median and mode are not in the same place in a skewed distribution. In both positive and negative skewed distributions, the mode is still at the peak, but the median has been pulled a little way towards the direction of skew and the mean has been pulled a longer way towards the direction of skew. **CHOOSING AND DRAWING GRAPHS** The different graphs usually display means, medians, and modes. This means that they are part of descriptive statistics because they help to describe the data in a way that our brains can understand. *Bar charts* -- are used when we want to depict differences or similarities between groups. *Scatter graphs* -- are used to show relationships between covariables -- both covariables should be linked to the same thing. *Contingency tables* -- are used in chi square to work out the totals for each category. *Histograms* - a histogram is a graphical display of tabulated frequencies. That is, a histogram is the graphical version of a table which shows what proportion of cases fall into each of several or many specified categories. The categories are usually non-overlapping intervals of some variable. The categories (bars) must be adjacent. For example, if we wanted to know the number of bilingual students in each year group of a school, the frequency of each year group could be plotted using a bar to represent the frequency in each year group. **When can I use each type of graph?** Bar chart -- can be used when you have frequencies from separate groups or categories and the data is not continuous. For example, male and female IQ scores would be plotted using a bar chart, because the groups *male* and *female* are not continuous data. Scatter graph -- is only used for plotting relationship between two related co-variables in a correlational analysis. Histogram -- should only be used when you have frequency data, and the data is continuous (there are no gaps). For an IQ score the bars might represent the frequencies for 50-69, 70-99, 100-119, and 120-139. There would be no gaps, and the data is continuous from 50 all the way to 139. **Remember when you are drawing a chart** 1. Choose the correct type of chart for the data and the research design 2. Plot the data correctly 3. Label the axes -- you lose marks if do not label them 4. Give the chart a title -- the title should mention each of the operationalised variables in the graph (e.g. gender, men, and women; reaction time in seconds) **When you draw a table remember you should give the table a title --** the title should mention each of the operationalised variables in the table and how they have been summarised, e.g. as means and SDs **INTERPRETING GRAPHS AND FIGURES** The research methods questions can include a task where you must interpret a table or a chart -- you may even have had to draw the chart yourselves. The general rule here is to identify as much as you can in the chart. Bar charts -- how similar/different are the bars? If there is a difference can you tell exactly how big that difference is? **Scatter plots** -- do the dots show a negative or a positive relationship? How close to a line are the points? The closer the points are to a line the stronger the relationship between the two covariables is. Are there any outliers (anomalies)? You should mention there are outliers, whenever they are present. For example, in the above scatter plot there is a negative correlation between mathematical ability score and musical ability score. As the maths ability increases the musical ability decreases. There are two outliers who did not fit this relationship pattern. One with both high musical and maths ability and one with both low musical and maths ability. **Tables** -- If the table contains the means for the separate groups, how similar are the means? How large are the range or standard deviation values (if they are included). Remember that a broad range or SD value indicates that the data are more spread out. **TOPIC 6: Reliability and validity** **THE DIFFERENT TYPES OF RELIABILITY: HOW TO TEST RELIABILITY AND HOW TO IMPROVE RELIABILITY** **Internal reliability** is the consistency of the data collection within the research. For example, in a questionnaire all of the questions should be measuring the same thing. In an experiment measuring reaction times, all of the responses from participants should be similar. If the data is all over the place (inconsistent) then the study does not have internal reliability. **External reliability** is where the research is repeated, and the same results are found. This means that between the two attempts at the research you have consistency. **Replicability** is when another researcher copies your study exactly and finds the same results. There is consistency between researchers running the same research **Reproducibility** is when a new, but similar, piece of research is conducted, and the same results have been found. This means that you have not replicated the research (as you did not copy it exactly) but you have reproduced the results. Reproducibility helps to show that a result is very robust and trustworthy. **Test-retest reliability** -- You test the same test on the same participants twice (with a period of time in-between). For example, a researcher could give a new IQ test to a group of students to test their IQ. To test the reliability of the test the researcher gives the same participants the same test two months later. If the test is reliable the results should be similar. If the researcher finds completely different results, they will know that the test is not reliable (if a test is not reliable it cannot be valid -- as it clearly is not testing what it was intended to test). **Split-half method** -- this is particularly useful for assessing the reliability of a questionnaire or exam. If the exam is reliable then all the questions should measure the same thing. We can test this by comparing half of the exam with the other half for each participant. To do this you can take the first half and last half, OR you could compare all the odd questions with all the even questions. If the test shows different results for each half, we know that the test is not consistent throughout: it lacks internal reliability. **Inter-observer reliability (inter-rater reliability)** -- Two or more people observe, rate, or mark the same thing. Then the relationship between each observer can be tested. Ideally, we would like to reach a correlation coefficient level of 0.8 (approximately 80% the same). If the correlation between the researchers is below 0.8, we do not have inter-observer/rater reliability. **Inter-interviewer reliability** is the same thing as inter observer reliability but for interviews. **Improving reliability** One way that researchers have improved reliability is to use the scientific method. Part of this approach to data collection has included the use of standardised instructions. Standardised instructions are written by the researcher for the participants to read. They are written clearly and explain what it is the participant must do. At the end of the instructions the participant is asked if they have any questions. Because all the participants in study read the same instructions this increases the likelihood that the results will be reliable (consistent). **INTERNAL AND EXTERNAL VALIDITY AND HOW TO IMPROVE VALIDITY** **Types of validity** **Internal validity** is whether you have measured/tested what you intended to measure/test. If you tried to measure a variable but by accident or mistake you measured a different variable, your research would lack validity. The same is true of an observation -- if you attempt to measure one behaviour (responses to anger) but in fact measure another behaviour (responses to frustration) you research would lack validity. **External validity** is whether your research can be compared (generalised) to the real world (**Ecological validity**), or, to other populations. For example, one of the biggest problems with psychological research is that much of the time it uses students. Students cannot be compared to non-students, so these studies often lack external validity, in terms of generalizability. **Checking validity** **Face validity --** do the results look like what would be expected from the hypothesis and method? This is the easiest way to check the validity of the research. **Historical validity** -- being able to generalise over time (towards the past) -- similar to predictive validity **Predictive validity --** like historical validity, but technically it is aimed towards the future. **Concurrent validity** -- is useful for checking the validity of questionnaires. You compare the results of your questionnaire to the results of another questionnaire. For example, if a child completes a questionnaire on their beliefs, you can give their parent a different version of the questionnaire. Then you can compare if the questionnaire is valid. You can also compare a new questionnaire with existing (made by other researchers) questionnaires on the same topic. If you get comparable results, you have concurrent validity. **Improving validity** **Controlling variables -** such as demand characteristics, experimenter/investigator bias, and social desirability bias. **Sampling of participants** -- needs to be conducted without investigator bias. It should also represent the target population as much as possible so that it has external validity (it can be generalised) **Random allocation into groups** -- this is used when independent groups design has been chosen. If the participants are randomly allocated to the groups there should be no influence of experimenter bias over who is put into which group. For example, a researcher could be biased and put all the intelligent people in one group. This would bias the results and decrease the validity of the experiment. **TOPIC 7: Problems that occur when running research** There can be many problems with the collection of data during research. If any of the problems listed below are present in the research, there may well be a loss of validity. The exam board will often include an issue in the description of a study and expect students to be able to identify what the problem is and identify a way in which it could be improved. **Social desirability bias (SDB)** -- participants change their behaviour so that they do not look bad in front of others. This is because humans have a desire to look good in front of others. This can cause a problem in any type of research as we usually try to look good in front of others. However, social research and interviews might be the most at risk of this type of bias. Additionally, overt observations can also be influenced by this bias. SDB can be reduced by using questionnaires instead of interviews and covert instead of overt observation. **Demand characteristic** -- The participant changes their behaviour in line with what they think the researcher is trying to find. If the research has been constructed in a way that allows the participants to guess what is happening (rightly or wrongly), then the participants might try to change their behaviour to do the correct thing. **Experimenter/researcher bias** -- The researcher is biased in the way they set up, analyse, or record the research so that they will get the result they hope for. This may not be intentional and may have happened unconsciously. **The "good participant" effect** -- The participant figures out what the researcher is looking for and tries to make sure that the researcher gets the results that they want. This is a form of SDB. **"Screw you" effect --** the participant figures out what the researcher is looking for and goes out of their way to sabotage the research because they have decided, for some reason, that they do not want the research to work. **TOPIC 8: Maths in Psychology** **Fractions --** you should be able to do these, for example, ½ = 0.5 and ¼ = ⅛+ ⅛ **Percentages --** you should be able to do these but let me know if you have any problems. **Ratios --** these can be questioned as part of stratified sampling AND as a question on the relationship between two or more groups. A ratio is often reduced to its simplest form, for example, 32:54 would be 16:27. Simply, find a number that both parts can be divided by, in this example the number is 2. Remember, a prime number cannot be divided any further. **Estimates** -- the exam board may ask you to calculate using estimates. This means that you offer a rough approximation of what the answer might be. It is useful when precise calculations are not important, and you just need a rough estimate that is easy to use for things like planning resources. For example, if a researcher wanted to know how many sheets of paper, they might need for a piece of research they would multiply the number of pages in the questionnaire by the number of participants. You would not want to order too few sheets of paper so you would round up to make sure there was extra. If there were 96 participants and 18 pages you would estimate 100 participants and 20 pages. So, the estimate would be 100x20 which is 2000 sheets of paper. The real number would be 1728. **Standard form and order of magnitude:** when a researcher is dealing with exceptionally large numbers (e.g. 5,000 000 000 000) it can be easier to use order of magnitude instead. In this example 5 000 000 000 would be written as 5x10^9^**.** The superscript "9" represents the number of decimal places we need to move. The superscript number is the order of magnitude -- which is a way of saying the number of decimal places we need to move a number to make it a number between 1 and 10. **TOPIC 9: Writing Psychological reports** **TAIMpedpRDR:** acronym for remembering the order of the sections listed below Title, Abstract, Introduction, Method, Participants, Equipment/apparatus, Design, Procedure, Results, Discussion, References **Title**: 10-15 words long. A title should be descriptive about the key variables or results of the study. **Abstract**: a summary of the whole paper that appears at the beginning of the article. It is written by including one to two sentences on each of the major sections. **Introduction**: this is often the longest part of a psychological report. It includes all the background research for the study. It is important as the introduction sets the scene and establishes the scientific context for 'why' the current study is being run. It should explain the key theory or theories the research is testing as well as describing the results of previous studies. The introduction should identify an issue in the theory or previous research that the current study will attempt to fix, solve, or learn more about. At the end of the introduction, you write your **aims** and **hypotheses**. **Method**: the method section describes exactly how the research was conducted and should be written in enough detail that a different researcher could read the method and replicate the study perfectly. The method section includes the Participant, Equipment, Design, and Procedure sections. **Participants**: a clear description of the participants who took part in the research, how they were sampled, the number of participants, and any key variable information, such as the age range, numbers of males and females, level of education, first language status. **Equipment (apparatus, stimuli)**: a description of all the equipment used (e.g. a computer and monitor, or an iPad), apparatus (e.g. measuring tape), or the stimuli (e.g. pictures in a slide show or how the questions on a questionnaire were created). **Design**: whether the research is repeated measures, matched pairs, or independent groups AND why you chose that design. What the IV and DV is. Which variables had to be controlled. In a correlation study what the co-variables were. **Procedure**: the step-by-step instructions of how the research was completed. For example, you would normally start with things like the location, how participants were given the instructions, what the participants had to do. There must be enough detail to allow another researcher to replicate the procedure perfectly. **Results**: in this section the author includes a summary of the raw data. Never put raw data into a results section. However, if you are instructed in the exam to create a table with raw data in that is what you should do. The summary of the data most often includes an appropriate measure of central tendency and an appropriate measure of dispersion. The results section will also include either a chart (scatter or bar) or a table. This section will also include the results from one of the four statistical tests (Mann-Whitney, Wilcoxon, Spearman's, or Chi Square). **Discussion**: In the discussion section you review the findings of the paper in the context of the research and theories introduced in the introduction section. You would state whether the research was successful, what problems occurred, and how the research could have been improved. You might then suggest how the results could be used to change the theories and what future directions should be taken. **References**: a list of all the articles used in the writing of the paper. **Writing a reference**: In psychology, students must be able to write a reference in APA format. This is the standard format for most of the articles published each year. An example for Zimbardo's famous study is below: Haney, C., Banks, W., & Zimbardo, P. (1973). Interpersonal dynamics in a simulated prison. *International Journal of Criminology and Penology, 1,* 69-97 The format: surname, initial., surname, initial., ampersand, surname, initial. (Year of publication). Title of article. *Title of Journal, volume number,* page numbers.