Correlations Between Variables Lecture Notes PDF
Document Details
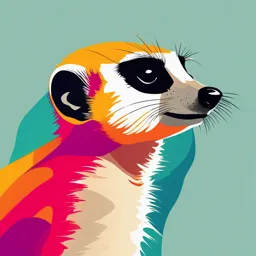
Uploaded by VictoriousElf1785
Bournemouth University
Bryan Leong
Tags
Summary
These lecture notes cover the concept of correlations between variables, including discussions of positive and negative correlations, zero correlation, and the correlation coefficient. They provide real-world examples and explanations of how to interpret correlation plots and use statistical tests for correlation.
Full Transcript
Correlations between variables Learning outcomes 1. What is correlation and when to use it Drawing and interpreting correlation plots Doing correlational tests Understanding correlational results Writing-up discussion (interpret results) 1. What is correlation and when to u...
Correlations between variables Learning outcomes 1. What is correlation and when to use it Drawing and interpreting correlation plots Doing correlational tests Understanding correlational results Writing-up discussion (interpret results) 1. What is correlation and when to use it To find out if there is a meaningful relationship between two variables, which is unlikely to have occurred due to sampling error (if the null hypothesis is true). 1. What is correlation and when to use it Remember, we are looking at an association/relationship here, not differences! There should not be different groups, or different conditions. There should be only two numeric (or ordinal) variables. 1. What is correlation and when to use it When examining differences: “What happens to X in Y1 and Y2?” When examining relationships: “If variable X decreases, what will happen to variable Y?” 1. What is correlation and when to use it In the plot, each point represent the data of one participant. As you can see from the plot, we are looking at the relationship between ‘Spelling test’ and ‘Shoe size’ 1. What is correlation and when to use it As the shoe size of participants increase, the score on the spelling test also increases. Children with bigger feet could spell better (higher on spelling test). 1. What is correlation and when to use it The null (default) hypothesis in a correlation states that there is no relationship between the two variables. In other words, the values of one variable are independent from the values of the other variable. 1. What is correlation and when to use it What do I need to look at? Direction Strength Significance What does correlation actually tell us? Direction of the relationship: Positive: as one variable increases, so does the other E.g., salary and spending allowance- the more money I earn, the greater my monthly spending allowance. What does correlation actually tell us? Direction of the relationship: Positive: as one variable increases, so does the other E.g., study hours and final grades- the longer students spend time studying, the higher the final grades obtained. What does correlation actually tell us? Direction of the relationship: Negative: as one variable increases, the other variable decreases E.g., Isolation and happiness - the more a person isolate themselves from the world, the less happy they felt. What does correlation actually tell us? Direction of the relationship: Negative: as one variable increases, the other variable decreases E.g., number of mistakes on an exam and exam grade- the more mistakes I make on the exam, the lower my exam grade. What does correlation actually tell us? Direction of the relationship: Zero: no relationship between the variables. E.g., Eating ice cream and hair growth has no relation. What does correlation actually tell us? Direction of the relationship: Zero: no relationship between the variables. E.g., How good you are at sports is not related with your exam grades. What does correlation actually tell us? Strength of the relationship(s): Measured with the correlation coefficient (r ) Which can range from -1 to +1 What does correlation actually tell us? Strength of the relationship(s): Measured with the correlation coefficient (r ) Note that the value represents strength, the +/- represents direction What does correlation actually tell us? Strength of the relationship(s): Measured with the correlation coefficient (r ) Perfect positive correlation What does correlation actually tell us? Strength of the relationship(s): Measured with the correlation coefficient (r ) High positive correlation What does correlation actually tell us? Strength of the relationship(s): Measured with the correlation coefficient (r ) Positively correlated but weak? What does correlation actually tell us? Strength of the relationship(s): Measured with the correlation coefficient (r ) Positively correlated but weak? What does correlation actually tell us? Strength of the relationship(s): Measured with the correlation coefficient (r ) Perfect negative correlation What does correlation actually tell us? Strength of the relationship(s): Measured with the correlation coefficient (r ) High negative correlation What does correlation actually tell us? Strength of the relationship(s): Measured with the correlation coefficient (r ) Low/weak negative correlation But we should not blindly believe correlation statistics But we should not blindly believe correlation statistics Source: https://www.tylervigen.com/spurious-correlations But we should not blindly believe correlation statistics Source: https://www.tylervigen.com/spurious-correlations But we should not blindly believe correlation statistics Other real correlations… Children with bigger feet spell better. Children who watch more media violence are more aggressive. Nations that add fluoride to their water have a higher cancer rate than those that don’t. Shark attacks increase with greater ice-cream sales. Genuine relationships but… Correlation does not mean causation: 1. There may be a third (or fourth, fifth) variable that explains the link Confounding variables E.g., temperature, crowds, number of inexperience swimmers, etc. Genuine relationships but… Correlation does not mean causation: 1. There may be a third (or fourth, fifth) variable that explains the link Confounding variables E.g., temperature, crowds, number of inexperience swimmers, etc. Genuine relationships but… Correlation does not mean causation: 1. There may be a third (or fourth, fifth) variable that explains the link Confounding variables Huff, D. (1954). ‘How to lie with statistics’ Cancer was more common in places where more milk was consumed. A positive correlation between milk consumption and cancer deaths. However, this is because the average life expectancy was higher in countries where more milk was consumed, and cancer tends to affect older people more. Genuine relationships but… Correlation does not mean causation: 2. Bi-directional links The DV may cause the IV or vice versa Playing video games and aggression Aggressive people are more likely to play more video games, or video games increase aggression? Genuine relationships but… Correlation does not mean causation: 3. The takeaway? No statistic can say anything about causation Even if it seems obvious, NEVER use the words: “caused”, “causal”, “causation” Instead, you should use the words: “associated”, “related”, “correlated” etc. 2. Drawing and interpreting correlation plots How to make a scatterplot? 2. Drawing and interpreting correlation plots Child's shoe Participant size (UK Number Measure) Spelling test score (%) How to make a scatterplot? 1 11 81 2 6 40 3 4 27 4 6 36 5 4 21 6 10 77 7 2 5 8 11 83 9 9 63 10 14 96 11 14 96 12 2 7 13 13 92 DV (or criterion variable) goes on IV (or predictor variable) goes on X axis Y-axis 2. Drawing and interpreting correlation plots Child's shoe Participant size (UK Number Measure) Spelling test score (%) How to make a scatterplot? 1 11 81 2 6 40 3 4 27 4 6 36 Each point represents a 5 4 21 participant 6 10 77 7 2 5 It is positioned on the graph depending on the 8 11 83 participant’s score on each 9 9 63 of the two variables. 10 14 96 11 14 96 12 2 7 13 13 92 2. Drawing and interpreting correlation plots Child's shoe Participant size (UK Number Measure) Spelling test score (%) How to make a scatterplot? 1 11 81 2 6 40 3 4 27 4 6 36 5 4 21 6 10 77 7 2 5 8 11 83 9 9 63 10 14 96 11 14 96 12 2 7 13 13 92 Repeat for every participant and you will obtain a scatterplot (i.e., points are scattered) 2. Drawing and interpreting correlation plots Highlight both variables How to make a scatterplot? On Excel Sample data for examining whether memory for faces and cars are associated. This is attached on Brightspace if you want to do it at home. 2. Drawing and interpreting correlation plots Insert and select ‘Scatter’ How to make a scatterplot? On Excel After selecting the two variables, Insert your “Scatter” figure 2. Drawing and interpreting correlation plots How to make a scatterplot? On Excel You have your scatterplot! However… something is missing. 2. Drawing and interpreting correlation plots How to make a scatterplot? On Excel REMEMBER to include the “trendline”. You will now see a dash-line (i.e., line of best fit) across your data points. Note. ‘Line of best fit’ means the line that fits best to ALL your data points 2. Drawing and interpreting correlation plots How to make a scatterplot? On Excel Since all your data point are concentrated on one section of the figure, you can format your axis. Just double click on the y- & x-axis Note. ‘Line of best fit’ means the trendline that fits best to ALL your data points 2. Drawing and interpreting correlation plots How to make a scatterplot? 70 65 On Excel 60 55 Car Memory Now you have a clearer image of how 50 45 your data looks like. 40 Can you tell whether the correlation is 35 30 positive/negative or near-zero? 25 38 43 48 53 58 63 68 Face Memory 3. Statistical tests of correlation 3. Statistical tests of correlation Pearson’s correlation Spearman’s correlation - Pearson’s test/ - Spearman’s Rank test/ Pearson’s r Spearman’s Rho - Parametric - Non-parametric - Normally distributed - Not normally distributed Pearson’s correlation ❑ Correlation was invented by Francis Galton (a cousin of Charles Darwin), but the statistical test and its applications were later developed by Karl Pearson ❑ The statistical test is also sometimes called “Pearson Product-Moment Correlation” ❑ And the correlation coefficient is called… “Pearson’s r ” ❑ Pearson’s correlation test is parametric, meaning it has certain assumptions about the distribution of the data Pearson’s correlation Parametric assumptions: 1. The data should be normal distributed Look at the Shapiro-Wilk results for normality test Normality test must have p-value more than.05 This typically comes from interval data 2. Assumption of independence Behaviour between participants should be unrelated E.g., Participant A should not affect results of participant B, and so on… Pearson’s correlation Logic behind Pearson’s r Imagine we are calculating the correlation between sunshine and temperature. Shared variance (covariance) The covariance indicates the amount of shared variance between the two variables. If they are unrelated, the variances will be However, as we know, the two are related; completely separate (non-overlapping) when it’s sunny, it also tends to be warm (so their variances will overlap) Pearson’s correlation Logic behind Pearson’s r Imagine we are calculating the correlation between sunshine and temperature. The covariance indicates the amount of shared variance between the two variables. Remember, for t-tests, the strength (i.e., effect size) is stronger for mean differences when there is LESS overlap… Contrary to t-tests, when there is MORE In short, how much “overlap” there are in overlap, the strength of correlation is the variances of two variables stronger! Pearson’s correlation Logic behind Pearson’s r (1) Deviations from mean (2) Multiply together to create covariance (or “shared” variance) (2) Covariance divided by Individual Variance (the product of the standard deviations of the two variables) Pearson’s correlation Logic behind Pearson’s r Pearson’s correlation test performs null-hypothesis significance testing to tell us: The strength (and direction): shown by the r coefficient, that ranges from -1 (perfect negative correlation) to +1 (perfect positive correlation); or, 0 indicates no correlation. The p-value of the result: how likely we are to see this result in the long run if the null hypothesis is true? So what null hypothesis is being tested? Pearson’s correlation Logic behind Pearson’s r Pearson’s correlation test performs null-hypothesis significance testing to tell us: Null hypothesis: relationship is due to chance Alternative hypothesis: Non-directional (two-tailed): there will be a relationship Directional (one-tailed): there will be a positive/negative relationship Pearson’s correlation Logic behind Pearson’s r Pearson’s correlation test performs null-hypothesis significance testing to tell us: Null hypothesis: relationship is due to chance; statistically non-significant (p >.05) Alternative hypothesis: Non-directional (two-tailed): there will be a relationship Directional (one-tailed): there will be a positive/negative relationship Statistically significant (p <.05) Pearson’s correlation Performing Pearson’s correlation We have a dataset showing how amount of revision (# of hours spent revising for an exam) is related to exam grade. Pearson’s correlation Performing Pearson’s correlation We have a dataset showing how amount of revision (# of hours spent revising for an exam) is related to exam grade. 1st Variable Correlation coefficient 2nd Variable P-value Pearson’s correlation Performing Pearson’s correlation We will learn more running correlation on JASP this during the seminars! 1st Variable Correlation coefficient 2nd Variable P-value Pearson’s correlation Writing up the results There was a strong positive correlation between amount of revision in hours and exam grade, r p(8) =.63, p =.052. However, the correlation did not reach statistical significance, so we fail to reject the null hypothesis 1st Variable Correlation coefficient 2nd Variable P-value Pearson’s correlation Writing up the results There was a strong positive correlation between amount of revision in hours and exam grade, r p(8) =.63, p =.052. However, the correlation did not reach statistical significance, so we fail to reject the null hypothesis r p indicates we are Correlation reporting Pearson’s r coefficient P-value Degrees of freedom (In correlation, it is always N – 2) Pearson’s correlation Writing up the results There was a strong positive correlation between amount of revision in hours and exam grade, r p(8) =.63, p =.052. However, the correlation did not reach statistical significance, so we fail to reject the null hypothesis Degrees of freedom For a correlation, you (In correlation, it is need at the very least, always N – 2) two points on your scatter plot (2 participants) Spearman’s correlation Or what to do when the data does not meet the normality assumption Spearman’s correlation Used with interval data that does not meet the parametric assumptions. Treated as Ordinal data when X and Y values are Ranks Example 1: We want to see the relationship of attractiveness rating (1 – 10) between self and others. We found that most participants rated themselves around highly attractive, while rating for others varied more. The ranks are not normal distributed because self-rating are clumped at the high end. Spearman’s correlation Used with interval data that does not meet the parametric assumptions. Treated as Ordinal data when X and Y values are Ranks Spearman’s correlation Used with interval data that does not meet the parametric assumptions. Treated as Ordinal data when X and Y values are Ranks Example 2: We want to estimate whether exam grades are related to anxiety. While exam grades will be normally distributed (usually!), the anxiety measure we used was not a well validated one, therefore it did not produce data that meet the assumption of normal distribution. Spearman’s correlation Used with interval data that does not meet the parametric assumptions. Treated as Ordinal data when X and Y values are Ranks Spearman’s correlation Used with interval data that does not meet the parametric assumptions. Treated as Ordinal data when X and Y values are Ranks Spearman’s correlation Used with interval data that does not meet the parametric assumptions. Treated as Ordinal data when X and Y values are Ranks Rho = N = Number of participants 4. Writing-up Spearman’s Rho There was a non-significant strong negative correlation between exam grade and anxiety scores, r s(8) = -.60, p =.068. Same way you interpret Pearson’s 4. Writing-up Spearman’s Rho There was a non-significant strong negative correlation between exam grade and anxiety scores, r s(8) = -.60, p =.068. Degrees of freedom (N – 2) Comparing Pearson’s and Spearman’s Same data, different tests Rp(8) = -.64, p =.047 Parametric test are more “powerful” because of the restriction to the data Rs(8) = -.60, p =.68 Comparing Pearson’s and Spearman’s However, when the assumptions of parametric tests are not met, Spearman’s Rho will have more power! Real world applications? Economics Inflation and unemployment rates (+ corr) Medicine Exercise and blood pressure (- corr) Social sciences Stress and Job satisfaction (- corr) Environmental science Global temperature and greenhouse gas (+ corr) Real world applications? Content Engagement on Brightspace and Final Unit Grade (EMSA 22/23) Experimental Methods and Statistics Analysis - Correlation 100 Engagement (% content visited on BS) 90 80 70 More content 60 50 engagement, higher 40 30 20 final EMSA grade 10 0 0 20 40 60 80 100 Final Unit Grade Doing correlation on JASP You will also get more hands-on training in Week 7’s seminars. Doing correlation on JASP Let’s use our ‘face and car memory’ data again. Open it on JASP (remember it has to be csv.) Doing correlation on JASP Click on “Regression” and select “Correlation” Doing correlation on JASP 1. Move over the variables you 4. Pairwise table want to correlate 5. Report significance (p-value) 2. Choose the type of correlation you want 6. Flag significance help identify significant correlation(s) easier 3. Choose your direction 7. You can create scatter (two-tailed or one-tailed) plots on JASP too Doing correlation on JASP Don’t forget to check your assumptions! Pairwise (2 variables) Shapiro-Wilk test was significant! This means normality assumption is violated Therefore, we should run Spearman’s Rho instead of *Included Pearson’s for comparison* Pearson’s r Doing correlation on JASP There is no significant correlation between car and face memory Note. Because normality assumption is violated, you can see Spearman’s Rho has more power than Pearson’s To obtain this figure, you must tick on ‘Display Pairwise’ Doing correlation on JASP You can edit the axis name and values To obtain this figure, you must tick on ‘Display Pairwise’ Doing correlation on JASP Which plot do you prefer? It’s up to you! 5. Writing Discussion 5. Writing Discussion 4. How does it relate 1. What was your to your existing goal? literatures? 2. What did your 5. Limitations and results say? future directions? 3. How does it 6. Conclusion relate to your hypothesis? 5. Writing Discussion 1. What was your goal of the experiment? Restate research question and your hypothesis Provide context and remind readers the purpose of your current study. “The main aim of the current was to examine…. We expected that….” 5. Writing Discussion 1. What was your goal of the experiment? Restate research question and your hypothesis Provide context and remind readers the purpose of your current study. 2. What did your results say? Summarize what you found in the results section (but without statistics). “We found that sleep quality was better for participants when they did not drink coffee compared to when they did.” 5. Writing Discussion 3. How does it relate to your hypothesis? Did your findings support or contradict your initial hypothesis or research question? Be concise, avoid “partially support”. Interpret your results, what are the implications? Explain the meaning of your findings (with the context of your research questions). 5. Writing Discussion 3. How does it relate to your hypothesis? Did your findings support or contradict your initial hypothesis or research question? Be concise, avoid “partially support”. Interpret your results, what are the implications? E.g., if you found that face recognition ability is different between Black-African and White-European. “Our findings suggests that face recognition is modulated by cultural factors.” 5. Writing Discussion 3. How does it relate to your hypothesis? Did your findings support or contradict your initial hypothesis or research question? Be concise, avoid “partially support”. Interpret your results, what are the implications? E.g., if you found that face recognition ability is different between Black-African and White-European. “Our findings suggests that face recognition is modulated by cultural factors.” This is very important for your practical report! 5. Writing Discussion 4. How does it relate to existing literature? Did your findings support or contradict existing literature? Try to connect this section with your introduction In what way did your study support/contradict? Be clear! “Our findings lends support to previous studies (citations), face recognition was better for own-race faces.” “In contrast to previous study (citation), face recognition was not better for own-race faces.” 5. Writing Discussion 5. Limitations/future directions? Acknowledge the limitations of your study Are there factors that might affect your results? Think about the current design and materials! Avoid saying limited sample size or inattention of participants Now that you know your limitations, what can be done to improve or avoid them in future studies? E.g., how can future research build upon your design to make the study more reliable/valid? 5. Writing Discussion 5. Limitations/future directions? Acknowledge the limitations of your study Are there factors that might affect your results? Think about the current design and materials! SHOW US YOUR ABILITY TO CRITICALLY Avoid saying limited sample size or inattention of participants EVALUATE Now that you know YOURwhat your limitations, FINDINGS! can be done to improve or avoid them in future studies? E.g., how can future research build upon your design to make the study more reliable/valid? 5. Writing Discussion 6. Conclusion Wrap-up everything and summarize the key take-aways from your study (there will be some repetition, don’t worry about it). Keep this short and concise! Should never be more than one paragraph. This is very important for your practical report! Some tips for your report 1. Please remember to follow APA 7th Edition format. 2. This section is 20-30% of your overall marks for the report. Keep it concise, well-structured & accurate (to your results). Avoid overinterpretation (making unsupported claims). Some tips for your report 3. “Statistics in ‘Results’, interpretation in ‘Discussion’!” 4. Don’t only criticize your study! Keep the balance between discussing the implications and limitations of your study. 5. Should not be more than 5 paragraphs in the discussion for this report. Writing your report! Remember the submission deadline for your practical report is on the 17th December, 12:00pm. Use the provided template and refer to the Student Guide. Look at the slides provided in the past few weeks for examples on how to write-up each section and you will be fine! Research experience If you sign-up for a SONA study and failed to attend (without a valid excuse), you will receive a PENALTY, e.g., major deduction of already-obtained credit. You need to let the researcher know beforehand that you are unable to participate to avoid “shooting yourself in the foot”. For your MCQs What does it tell us? Average (Sum divided by number of Summarizing data values) Types? (descriptives) Middle value when data is numerically ordered (use this when Mean (central) Central tendencies data is skewed) (summarize data) Median (distance) Highest frequency Mode (frequency) Range How spread is your data Dispersion based on the median Range IQR (measure of spread) Variance Deviation How spread is your data Standard based on the mean deviation For your MCQs What does it tell us? Type of distribution? Mode = Median = Mean Symmetrical bell-shaped Normal curve Mode < Median < Mean Positive Skewed Negative Mean < Median < Mode For your MCQs How likely will I obtain a Probability using a score within this range? normal distribution 68% of your data will 1 Standard deviation be within 1 SD away from the mean from the mean 1.96 Standard 95% of your data will deviations from the be within 1.96 SD away mean from the mean More than 1.96 5% of your data will Standard deviations fall outside of 1.96SD from the mean from the mean For your MCQs Estimating population What does inferential statistics measure? mean? How much the mean But not always Standard error of Sampling error varies between different mean possible to have samples u … 95% Confidence The range of values that have a Our sample is interval specific % likelihood of 95% of population mean including the population mean (all the sample) For your MCQs What tests to run? We want to look at differences in means. Are your assumptions met? W ’ -test What is your design? No (p <.05) Student/ Equal variance independent t- Between-subject (L ’ test) Yes (p >.05) (compare one measure between test (default) two groups) No (p <.05) Mann- Normality (Shapiro-Wilk) Whitney U Within-subject Wilcoxon (compare one sample between Normality No (p <.05) two conditions) (Shapiro-Wilk) signed-rank Yes (p >.05) Paired sample t-test (default) For your MCQs W ’ -test There is a significant difference, we reject the H0 Student/ and accept H1 independent t- (differences found is due to manipulation) test P-value: Less than.05 (p <.05) Mann- Whitney U Wilcoxon P-value: More than.05 (p >.05) There is no significant signed-rank difference, we accept the H0 and reject H1 Paired sample (any differences found is due to chance) t-test (default) Any questions? Thank you, class. Good luck for the exam and rest of the semester! Extra examples…