Hypothesis Testing PDF
Document Details
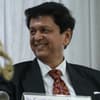
Uploaded by CoolestJackalope
Jiwaji University
Prof.(Dr.) K. S. Thakur
Tags
Summary
This document describes research methodology, focusing on hypothesis testing. It covers concepts like null and alternative hypotheses, types of hypotheses, and the process of hypothesis testing, including decision rules and different test types. The document is a collection of slides or lecture notes on the topic.
Full Transcript
RESEARCH METHODOLOGY Prof.(Dr.) K. S.Thakur Dean, Faculty of Commerce School of Commerce & Business Studies Jiwaji University, Gwalior 474002 (INDIA) HYPOTHESIS Hypothesis is considered as the principal instrument in research. Its main function is to suggest new experiments and o...
RESEARCH METHODOLOGY Prof.(Dr.) K. S.Thakur Dean, Faculty of Commerce School of Commerce & Business Studies Jiwaji University, Gwalior 474002 (INDIA) HYPOTHESIS Hypothesis is considered as the principal instrument in research. Its main function is to suggest new experiments and observation. In Fact many experiments are carried out with the deliberate object of testing Hypothesis. Decision makers often face situations where in they are Interested in testing hypothesis on the basis of available information and then Take decision on the basis of such testing. In social science Where direct knowledge of population parameters is rare. Hypothesis Testing is the often used strategy for deciding whether a sample data often such support for a hypothesis that generalisation can be made. Thus hypothesis testing enables us to make probability statement about Population parameters. The hypothesis may not be proved absolutely, But in practices its accepted if it has with stood a critical testing. Before we explain how hypothesis are tested through different Tests meant for the purpose, it will be appreciation to explain Clearly the meaning of hypothesis and the related concepts. For better understanding of the hypothesis testing techniques. what is hypothesis- Ordinarily, when one talks about hypothesis, one simply means a mere assumption Or some supposition to be proved or disproved. But for the researcher hypothesis Is a formal question that he intends to resolve.Thus a hypothesis may be defined As a proposition or a set of proposition set forth as an explanation for the occurrence of some specified group of phenomena either asserted merely as a provisional Connection to guide some investigation or accepted as highly probably in the lights Of established facts. Quite often a research hypothesis is a predictive statement. Capable of being testing by scientific methods, that relates an independent variable To same depend variable. For example- consider statement like the following ones: Meaning of hypothesis according to Claude bernard“student who receive counselling Will show a greater increase is creativity than students no receiving counselling” or “the automobile A is performing as well as automobile B.” there are hypothesis capable of being objectively verified or tested. Thus we may conclude that hypothesis states what we are looking for and its a proposition which can be put to a that to determine its validity. types of hypothesis- the types of hypothesis need to be explained- 1) Null hypothesis and alternative hypothesis:- in the context of statically analysis. We often talk about null hypothesis and alternative hypothesis if are to compare method A with method B about its superiority and if we proceed on the assumption that both methods are equally good, then this assumption is termed as the null hypothesis, as against this , we may think that the method B is inferior, we are then stating what is termed as alternative hypothesis as Ha. Suppose we want to test hypothesis that the null hypothesis is that the population means is equal to To the hypothesis means 100 and symbolically we can express as: Ho:µ ˭ µ Ho˭ 100 If our sample result do not support this null hypothesis, we should calculate that something else is true. What we conclude rejection the null hypo- thesis is known as alternative hypothesis. In other words, the set of alternative to the null hypothesis is referred to as the alternative hypo- thesis. If we accept Ho, then we are rejecting Ha , Ho:µ ˭ µ Ho ˭ 100, We may consider three possible alternative hypothesis as follow: Alternative hypothesis To be read as follow Ha : µ ≠ µ Ho (the alternative hypo is that the population mean is not equal to 100. if may be more or less than 100) Ha : µ ˃ µ Ho The alternative hypo is that the population means greater than 100 Ha : µ ˂ µHo The alternative hypo is that the population means is lesser than 1oo The null hypothesis and the alternative hypothesis are chosen before the sample is drawn (the researcher must avoid the error of testing the hypothesis from the same data.) the choice of null hypothesis. The following considerations are usually kept in view: A) Alternative hypothesis is usually the which one wishes to prove and null hypothesis is the one which one wishes to disprove. thus a null hypothesis represents all other possibilities. B) if the rejection of a certain hypothesis when its actually true involves great risk , its taken as null hypothesis because then the probability of rejection it when its true is α ( the level of significance) which is chosen very null. C) Null hypothesis should always be specific hypothesis. It should not state about or approximately a certain value. generally hypothesis testing we proceed on the basis of null hypothesis. Keeping the alternative hypothesis. keeping the alternative hypothesis in view. Why so? the answer that on the assumption that null hypothesis is true, one can assign the probability to different possible sample result but this cannot be done if we proceed with the alternative hypothesis. Hence the use of null hypothesis (at times also known as statically hypothesis) is quite frequent. 2) The level of significance :- this is a way important concept in the context of hypothesis testing. Its always some percentile (usually 5%) which should be chosen with great care, through and reason. In case we take the significance level at 5% than this implies that Ho will be rejected. Reason in case we take the significance level at 5% when the sampling result (i.e. Observed evidence) has a less than 0.05 probability of occurring if Ho is true. In other words , the 5% level of significance means that researcher is willing to take as much as a 5% risk of rejecting a null hypothesis when it (Ho) happens to be true and is usually determined in advance before testing the hypothesis. 3)- Decision rules and test of hypothesis- Given a hypothesis Ho an alternative hypothesis Ha, we make a rule which is known as decision rule according to which we accepted Ho(i.e. Reject Ha) or reject Ho(i.e. Accept Ha). For instance if (Ho is that a certain lot is good). There are to many defective items in it. Then we must decide the numbers of items to be tested and the criterion for accepting or rejecting the hypothesis. We may test 10 items in the lot and plan our decision saying that if there are none or only 1 defective items among the 10. we will accept Ho otherwise we will reject Ho (or accept Ha). This sort of basis is known as decision rule. 4) Type 1 and type 2 errors- in the context of testing of hypothesis , there are basically two types of errors we can made. We may reject Ho when Ho is true and we may accept Ho when in fact Ho is not true. the former is known as type 1 error and the letter as type 2 error. in other words, type 1 error means rejection of hypothesis which should have been accepted and type 2 error means rejection of hypothesis which should have been rejected. type 1 error is denoted by α (alpha) known as α error, also called the level of significances of tests and type 2 errors are denoted by β (beta) known as β errors. In the tabular form they said two errors can be presented as follow:- Decision Accept Ho Reject Ho Ho (true) Correct decision Type 1 error(α error) Ho (false) Type 2 error (error) Correct decision The probability of type 1 error is usually determined in advance and is understood as the level of significance of testing. the hypothesis if type 1 is fixed at 5%, it means that there are about 5 chances in 100 that we will reject Ho when Ho is true. We can control type 1 errors just by fixing it at a lower level. For instance if we fix it at 1% we will say that the maximum probability of committing type 1 error would only be 0.01. but with a fixed sample size, n when we try to reduce type 1 errors the probability of committing type 2 error increase. Both type of errors increase cannot be reduced simultaneously. There is trade of between two types of error can only be reduced if we are willing to increase the probability of making the other type of error. to deal with this trade off in business situation, decision makers decide the appropriate level of type 1 error by examining the cost of penalties. attached to both type of errors. If type 1 errors involves the time and trouble of reworking a batch of chemical that should have been accepted Whereas type 2 error means taking a chance that an entire group of users of the chemical compound will be poisoned, then in a situation one should prefer a type 1 error to a type 2 error. As a result one must set very high level for type 1 error in ones testing technique of a given hypothesis? Hence in the testing hypothesis, one must make all possible efforts to strike an adequate balance between type 1 and type 2 errors. 5) Two tailed and one tailed tests :- in the context of hypothesis testing there two terms are quite important and must be clearly understood. A two tailed test reject the null hypothesis if say, the sample means is significantly higher and lower than the hypothesised value of the mean of the population, such a test is appropriate when the null hypothesis is some specified value and the alternative hypothesis is a value not equal to the specified value of the null hypothesis. symbolically the two tailed test is appropriate when we have Ho = µ = µ Ho and Ha : µ ≠ µ Ho which we mean µ ˃ µ Ho or µ ˂ µ Ho. Thus in the two tailed test , there are are two rejection region. HYPOTHESIS TESTING :- Two test of hypothesis means to tell ( on the basis of data the researcher Has collected) whether or not the hypothesis testing the main question is : whether to accept the null hypothesis or not to accept the null hypo Thesis ? Procedure for hypothesis testing refer to all those step that we undertake for making a choice between the two action i.e. rejection or acceptance of a null hypothesis. The various steps involved in hypothesis testing are stated below : 1) Making a formal statement- Two steps consists in making a formal statement of the null hypothesis (Ho) and also of the alternative hypothesis (Ha). This means that hypothesis should means that hypothesis should be clearly stated considering the nature of research problem. For instance, Mr. Mohan of civil engineering Department want to test the load bearing capacity of an old bridge which must be more than tons, in that case he can state his hypo- thesis as under- Null hypothesis Ho : µ = 10 Tons Alternative hypothesis Ha : µ ˃ 10 Tons Take another example the score in an aptitude test administered at the national level is 80. to evaluate a state education system, the average score and national score. In such a situation the hypothesis may be states as under : Null hypothesis is Ho : µ = 80 Alterative hypothesis Ha : µ ≠ 80 The formulation of hypothesis is an important step which must be accomplished with due care in accordance with the object and nature of the problem under consideration. It also indicates whether we should use a one tailed test or a two tailed test. If Ha is of the type greater than (or of the type lesser than) , we use a one tailed test but when Ha is of the type whether greater or smaller than we use a two tailed test. 2) Selecting a significance level- The hypothesis are tested on a predetermined level of significance and as such the same should be specified. Generally in practice, either 5% level or 1% level is adopted for the purpose. The factor that affect the level of significance are- (a) The magnitude of the difference between sample means. (b) The size of the sample: and (c) The validity of managements within samples (d) Whether the hypothesis is directional or non – directional of the difference between say, means. In brief, the level of significance must be adequate in the context of the purpose and nature of enquiry. 3) Deciding the distribution to use- After distribution the level of significance the next step in hypothesis testing is to determine the appropriate sampling distribution. The choice generally remain between normal distribution and t- distribution. The rules for selecting the correct distribution are similarly to those which we have stated earlier in the context of estimation. 4) Selecting a random sampling and computing an appropriate value- Another step is to select a random samples and compute an appropriate value from the sample data concerning the test statically utilizing the relevant distribution. in other words, Draw a sample to furnish empirical data. 5) Calculating the probability - One has then to calculate the probability that the sample result would diverge as widely as it has from expectations, If the null hypothesis were in fact true. 6) Computing the probability- Yet another step consists in comparing the probability thus calculated with the specified value for α , the significance level. If the calculated probability is equal to or smaller than the α value in case of tailed test ( and α/2 in case of two tailed test), then reject the null hypothesis ( i.e. Accept the alternative hypothesis), but if the calculated probability is greeter then accept the null hypothesis, in case we reject Ho , we run a risk of (At most the level of significance) committing an error of type 1 , but if we accept Ho , then we run some risk ( the size of which cannot be specified as long as the Ho happen to be vague rather than specific) of committing an error of type 2. State Ho as well as Ha Specify the level of significance ( or the α value) Decide the correct sampling distribution Sample a random samples (s) and worked out an appropriate value from sample data Calculating the probability that sample result would diverge as widely as it has from expectations, if Ho were true Is this probability equal to or smaller than α value in case of one tailed test α Value in case of one tailed test and α/2 in case of two tailed test. Yes No reject Ho Accept Ho Thereby run the risk of Thereby run some risk of FOMULATION OF HYPOTHESIS- 1- MAKING A FOMAL STATEMENT- The researcher Undertake research start with defining the problem clearly. Once that has been achieved the researcher is in a position to Define the null hypothesis and alternative hypothesis. 2- select relevant test and probability distribution- In the next step, its necessary to select a statistical technique and Probability distribution. The choice of probability distribution Depend on the purpose of hypothesis test. The researcher should Carefully se how the test statistic is computed and which sampling Distribution is it following normal or chi distribution. 3- choosing the critical value- the criteria for accepting Or rejecting the null hypothesis is depend on - A) Level of significance B) Degree of freedom C) One or two tailed test. 4) Collect data and test statistic- the next stage involves drawing a sample and collecting data using a data collection data using a data collection strategy that suit the purpose of study. 5) Compare the test statistics and critical value- this is a crucial stage where the test statistics computed earlier is compared with the critical value specified. 6) Taking a final decision- in the last step the researcher has to now make a decision of accepting or not accepting null hypothesis. SOURCES OF HYPOTHESIS- 1) CULTURE- Culture is a first and main sources of hypothesis. the area and the tradition of this place describe the one way of hypothesis. 2)SCIENTIFIC THEORY- Scientific theories are another sources of hypothesis because the culture is affected by scientific theories. 3)ANALOGIES- analogies are another third sources of hypothesis they provide the idea about the scientific theories and the culture of area. Test of hypothesis- As has been stated above that hypothesis testing determines the validity of the assumption (Technically described as null hypothesis) with a view to chosen between two conflicting hypothesis about the population parameters. Hypothesis testing help to decide on the basis of samples data, whether a hypothesis about the population is likely to be true or false. Statiscians have developed several tests of hypothesis ( also known as the tests of significance) for the purpose of testing of hypothesis which can be classified as: a) Parametric tests or standard test of hypothesis. And b) Non parametric test or distribution – free test of hypothesis. HYPOTHESIS TESTING PARAMETRIC TEST NON PARAMETRIC TEST ONE SAMPLE TWO SAMPLES ONE SAMLE TWO SAMPLE 1)T-TEST 1)CHI-SQURE TEST 2)Z-TEST 2)RUN TEST 3)CHI- SQURE IDEPENDENT TEST PAIRED SAMPLE INDEPNDENT PAIRED 1)TWO GROUP T-TEST 1)PAIRED T-TEST 1)CHI-SQURE 1)SIGN T 2)Z-TEST 2)MANN-WHITNEY 2)wilcoxon t 3)F-TEST 3)KURSKALL WALLIS 3)mc nemer t 4) chi-squre t HYPOTHESIS TEST OF DIFFERENCE Parametric test:- Parametric test usually assume certain properties of the parent population from which we draw samples. Assumption like observation come from a normal population. Sample size is large assumption about the population parameters like mean, variance etc. Must hold good before parametric test can be used but there are situation when the researcher cannot or does not want to make such assumptions. Important parametric test- 1) Z- Test 2) T- Test 3) Chi-square Test, and 4) F- Test All these test are based on the assumption of normality i.e. the source of data is considered to be normally distributed. Yet the test will be applicable on account of the fact that we mostly deal with samples and the sampling distribution closely approach distribution. a) Z- test :- Z- test is based on the normal probability distribution. and its used for judging the significances of several statically measures. particularly the mean. The relevant test statistics. Z is working out and compared with its probable value ( to be read from table showing area under normal curve ) at a specified level of significance for judging the significance of the measure concerned. This is most frequently used test in research studies. This test is used even when binominal distribution or t- distribution is applicable on the presumption that such a distribution tends to approximate normal distribution as “n” became larger. Z- test is generally used for comparing the mean of the sample to same hypothesised mean for the population in case of larger sample, or when population variance is known. Z- test is also used for judging the significances of difference between mean of the two independent sample in case of large sample or when population variance is known. Z- test is also used for comparing the sample Proportion to a theoretical value of population proportion or for judging the difference in proportions of two independent samples when n happen to be large. Besides , this test may be used for judging the Significance of median, mode coefficient of correlation and several other measurement. 2) T- test-. T test is based on t distribution and its considered an appropriate test for judging the significances of a sample mean or for judging the significance of difference between the mean of two sample in case of small samples when population variance is not known (in which case two samples are related , we use paired T- test ( or what is known as difference test ) for judging the significance of the mean of difference between the two related samples. It can also be used for judging the significances of the coefficient of simple and partial co-rreletion. The relevant test statistics, T is calculated from the sample data and than compared with its probable value based on t- distribution ( to be read from the table that gives probable value of t- for different levels of significance for concerning degree of freedom for accepting or rejecting the null hypothesis. It may noted that T- test applies only in case of small samples (s) when population variance is unknown. 3) chi-square Test:- This test based on chi- square distribution and as a parametric test is used for comparing a sample variance to a theoretical population variance. 4) F- test:- F- Test is based o chi- square distribution and its used to compare the variance of the two independent samples. This test is also used in the context of analysis of variance (ANOVA) for judging the significance of more than two samples mean at one and the same time. Its also used for judging the significances of multiple co- relation coefficient test statistics. F- test is calculated and compared with its probable value ( To be seen in the F ratio table) for different degree of freedom for greater and smaller variance at specified level Of significance for accepting or rejecting the null hypothesis. Non- Parametric Test:- In such situation we use statistical methods for testing hypothesis which are called Non- parametric test because such test do not depend on any assumption about the parameters of the parent population. besides, most no parametric test assume only nominal or ordinal data, whereas parametric test require measurement equivalent to at test on interval scale. Types of non parametric test:- 1) Sign test 2) Wilcoxon matched pair test ( or signed rank test) 3) Wilcoxon mann whitney test ( or U- test ) 4) Kruskal- wallis H- test 5) Rank co- rrelation 6) Kendell´s coefficient of concordance 7) Fisher – Irwin test 8) One sample run test 1) Sign test:- sign test uses direction of difference to test if population mean is equal to hypothesized mean. The sign test is of two types: a) One sample sign test b) Two sample sign test 2) Wilcoxon matched pairs test:- this test is suitable for matched pairs of before and after experiment type. The direction as well as magnitude of difference is considered. 3) Wilcoxon- mann- whitney test (U- test)- U- test measures the degree of separation or the amount of overlap between two independent samples. 4) Kruskal- wallis H-test:- H- test is a non- parametric analysis of variance that does not require the assumption of normality of population. 5) Rank Correlation method:- This method is actually a measure of correlation or association. 6) Kendall´s coefficient of concordance:- this method is used for determining the degree of association among several k sets of ranked data of n objects. 7) Mc nemer test:- This test is used for two related samples in situations where the attitude of peoples are noted before and after treatment to test the significance of change in opinion if any. THANK- YOU.........