Support_Introduction au Machine Learning.pdf
Document Details
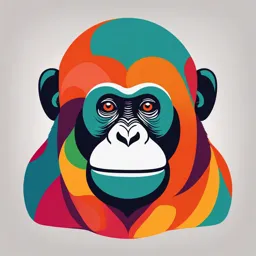
Uploaded by ReasonedFlute
VISIPLUS Academy
Tags
Full Transcript
SI Ac P Page 1 LU ad VI S e au...
SI Ac P Page 1 LU ad VI S e au Learning SI Ac P au Machine Introduction LU ad S em Learning Ac y a Machine Introduction U de VI S m Ac y ad VI em SI y PL U de VI S m SI y PL U VI S SI Ac P LU ad IS S em IP Ac L U ad S em A y SI Ac P ad Page 2 LU VI S e Learning SI Ac P au Machine Introduction LU ad S em Ac y a U de VI S m Ac y ad VI em SI y PL U de VI S Chapitre 1 : m SI y PL U VI S en pleine expansion Le Machine Learning SI Ac P LU ad IS S em IP Ac L U ad S em A y SI Ac P ad Page 3 LU VI S e Learning SI Ac P au Machine Introduction LU ad S em Ac y a U de VI S m Ac y ad VI em SI y PL U de VI S m SI y PL U VI S Tendances SI Ac P LU ad IS S em IP Ac L U ad S em A y e em VI S ad U ad Cas d’usage Ac PL y Ac em SI y em VI S ad Banque et U E-commerce Plateformes de ad secteur Ac PL et marketing contenu financier y Ac m S SI de LU Introduction VI S a LU au Machine P Ac SI Learning y P em S SI A Bâtiment Véhicule Génération de LU intelligent autonome contenu VI S Page 4 ad U P Ac L SI y IP m VI S IS de U SI Ac P ad Page 5 LU VI S e Learning SI Ac P au Machine Introduction LU ad S em Ac y a U de VI S m Ac y ad VI em SI y PL U de VI S m SI y PL U VI S SI Ac P « The sexiest job » LU ad IS S em IP Ac L U ad S em A y e em VI S ad U Les métiers ad Ac PL y Ac em SI y em VI S ad U Machine Learning Deep Learning ad Ac Data Scientist PL Engineer Engineer y Ac m S SI de LU Introduction VI S a LU au Machine P Ac SI Learning y P em S SI A LU VI S Page 6 ad Data Engineer Data Analyst U P Ac L SI y IP m VI S IS de U SI Ac P ad Page 7 LU VI S e Learning SI Ac P au Machine Introduction LU ad S em Ac y a U de VI S m Ac y ad VI em SI y PL U de VI S m SI y PL U VI S Les métiers SI Ac P LU ad IS S em IP Ac L U ad S em A y SI Ac P ad Page 8 LU VI S e Learning SI Ac P au Machine Introduction LU ad S em Ac y a U de VI S m Ac y ad VI em SI y PL U de VI S m SI y PL U VI S Les métiers SI Ac P LU ad IS S em IP Ac L U ad S em A y e em VI S ad U ad Ac Ce qu’il faut retenir PL y Ac em SI y em VI S ad Le Machine Learning est à la mode U ad Ac PL y Beaucoup de demandes sur le marché Ac m S SI de l’emploi de LU Introduction VI S Une grande diversité de métiers a LU au Machine P Ac SI Learning y P em S SI A LU VI S Page 9 ad U P Ac L SI y IP m VI S IS de U SI Ac P LU ad Page 10 VI S e Learning SI Ac P au Machine Introduction LU ad S em Ac y a U de VI S m Ac y ad VI em SI y PL U de VI S m Chapitre 2 : SI y PL U machine learning VI S SI Ac P Découvrir les concepts du LU ad IS S em IP Ac L U ad S em A y e em VI S ad U ad Ac PL y Intelligence artificielle Ac em SI y em VI S ad L'intelligence artificielle (IA) est U un « ensemble de théories et de techniques ad Ac mises en œuvre en vue PL y de réaliser des machines capables de simuler Ac m l'intelligence humaine » S SI de LU Introduction VI S a Wikipédia LU au Machine P Ac SI Learning y P em S SI A LU VI S Page 11 ad U P Ac L SI y IP m VI S IS de U e em VI S ad U ad Ac PL y Machine Learning Ac em SI y em VI S ad « L'apprentissage automatique est un champ U d'étude de l'intelligence artificielle qui se fonde sur ad Ac PL des approches mathématiques et statistiques pour y donner aux ordinateurs la capacité d'apprendre à Ac m S SI partir de données [...] résoudre des tâches sans être de explicitement programmés pour chacune. » LU Introduction VI S Wikipédia a LU au Machine P Ac SI Learning y P em S SI A LU VI S Page 12 ad U P Ac L SI y IP m VI S IS de U SI Ac P ad Page 13 LU VI S e Learning SI Ac P au Machine Introduction LU ad S em Ac y a U de VI S m Ac y ad VI em SI y PL U de VI S m SI y PL U VI S IA > ML > DL SI Ac P LU ad IS S em IP Ac L U ad S em A y SI Ac P ad Page 14 LU VI S e Learning SI Ac P au Machine Introduction LU ad S em Ac y a U de VI S m Ac y ad VI em SI y PL U de VI S m SI y PL U VI S SI Ac P LU ad S em Machine Learning : utilisation IS Ac IP L U ad S em A y e em VI S ad U ad Ac Les familles du Machine Learning PL y Ac em SI y em VI S ad U ad Ac Supervisé Non supervisé PL y Ac Valeur cible connue Pas de valeur cible m S SI de LU Introduction VI S a LU au Machine P Ac Prix d’une maison Clustering de base SI Learning clients y P em Détection de SPAM Réduction de S SI A … dimensions LU VI S Page 15 ad U P Ac L SI y IP m VI S IS de U e em VI S ad U ad Ac PL y Les autres types de Machine Learning Ac em SI y em VI S ad U ad Ac PL y Ac m S SI de LU Introduction VI S a RL : LU au Machine Reinforcement Semi-Supervised Self-Supervised P Ac Learning Learning Learning SI Learning y P em S SI A LU VI S Page 16 ad U P Ac L SI y IP m VI S IS de U e em VI S ad U ad Ac Ce qu’il faut retenir PL y Ac em SI y em VI S ad Le Machine Learning est un type U ad Ac d’intelligence artificielle PL y Ac m S SI Le ML diffère de la programmation de LU traditionnelle Introduction VI S Le programme est généré a LU au Machine P Ac SI Learning y On distingue deux grandes familles : P em S SI A apprentissage supervisé LU apprentissage non supervisé VI S Page 17 ad U P Ac L SI y IP m VI S IS de U SI Ac P ad Page 18 LU VI S e Learning SI Ac P au Machine Introduction LU ad S em Ac y a U de VI S m Ac y ad VI em SI y PL U de VI S m Chapitre 3 : SI Appréhender y PL U VI S SI Ac P l’apprentissage supervisé LU ad IS S em IP Ac L U ad S em A y e em VI S ad U ad Ac PL y Les données Ac em SI y em VI S ad Longueur Largeur Type Pointure du Code postal Prix U propriétaire ad Ac PL y 10 10 Maison 38 31 000 200 000 Ac m S SI 20 Maison 44 31 200 000 de LU Introduction VI S a LU 5 10 Appartement H 31 400 150 000 au Machine P Ac SI Learning y P 8 7 000 T5 31 400 140 k em S SI A LU VI S Page 19 ad U P Ac L SI y IP m VI S IS de U e em VI S ad U ad Ac PL y Les données Ac em SI y em VI S ad Longueur Largeur Type Pointure du Code postal Prix U propriétaire ad Ac PL y 10 10 Maison 38 31 000 200 000 Ac m S SI 20 Maison 44 31 200 000 de LU Introduction VI S a LU 5 10 Appartement H 31 400 150 000 au Machine P Ac SI Learning y P 8 7 000 T5 31 400 140 k em S SI A LU VI S Page 20 ad U Échantillon / Ligne P Ac Variable / Colonne L / Observation Cible / Target SI y / Feature IP / Individu m VI S IS de U e em VI S ad U ad Ac PL y Les étapes Ac em SI y em VI S ad U Training Training ad Ac New Features PL Features Target y Ac m S SI de LU Introduction VI S Model a LU au Machine Model + P Ac Rules SI Learning y P em S SI A LU VI S Page 21 ad Rules Predicted output U P Ac L SI y IP Entrainement m Inférence VI S IS de U SI Ac P LU ad Page 22 VI S e Learning SI Ac P au Machine Introduction LU ad S em Ac y a U de VI S m Ac y ad VI em SI y PL U de VI S m SI y PL U VI S SI Corrélation ≠ causalité Ac P LU ad Prédire n’est pas expliquer IS S em IP Ac L U ad S em A y SI Ac P LU ad Page 23 VI S e Learning SI Ac P au Machine Introduction LU ad S em Ac y a U de VI S m Ac y ad VI em SI y PL U de VI S m SI y PL U VI S SI Ac P LU ad Prédire n’est pas expliquer IS S em IP Ac L U ad S em A y e em VI S ad U ad Ac Apprentissage supervisé PL y Ac em SI y em VI S ad U ad Ac PL y Ac m S SI Classification Régression de LU Introduction VI S a LU au Machine P Une classe parmi un Ac Un nombre continu SI Learning nombre fini de classes y P em S SI A LU VI S Page 24 ad U P Ac L SI y IP m VI S IS de U e em VI S ad U ad Ac Ce qu’il faut retenir PL y Ac em SI y em VI S ad Les données U Échantillons, colonnes et cible ad Ac PL y Ac Une première phase d’entraînement m S SI Suivie d’une phase d’exploitation de LU Introduction VI S Faire des prédictions n’est pas expliquer a LU au Machine P Ac SI Learning Il y a deux catégories de problèmes y P supervises : em S SI A Classification LU Régression VI S Page 25 ad U P Ac L SI y IP m VI S IS de U e em VI S ad U ad Ac PL y Ac em SI y em VI S ad Chapitre 4 : U ad Ac PL y Visualiser le cycle de vie Ac m S SI de LU Introduction d’un projet VI S a LU au Machine P Ac de Machine Learning SI Learning y P em S SI A LU VI S Page 26 ad U P Ac L SI y IP m VI S IS de U SI Ac P LU ad Page 27 VI S e Learning SI Ac P au Machine Introduction LU ad S em Ac y a U de VI S m Ac y ad VI em SI y PL U de VI S m SI y PL U VI S SI Ac P LU ad Le cycle de vie d’un projet IS S em IP Ac L U ad S em A y e em VI S ad U ad Ac Ce qu’il faut retenir PL y Ac em SI y em VI S ad Un projet de Machine Learning est un U ad Ac processus itératif PL y Ac m S SI Comprendre les enjeux « business » est de LU primordial Introduction VI S a LU au Machine P Ac Analyser pour décider la prochaine SI Learning y étape à traiter est crucial P em S SI A LU VI S Page 28 ad U P Ac L SI y IP m VI S IS de U SI Ac P LU ad Page 29 VI S e Learning SI Ac P au Machine Introduction LU ad S em Ac y a U de VI S m Ac y ad VI em SI y PL U de VI S m Chapitre 5 : SI y PL U S Mettre en place son VI SI Ac P environnement de travail LU ad IS S em IP Ac L U ad S em A y SI Ac P LU ad Page 30 VI S e Learning SI Ac P au Machine Introduction LU ad S em Ac y a U de VI S m Ac y ad VI em SI y PL U de VI S m SI y PL U VI S Démonstration n°01 SI Ac P LU ad IS S em IP Ac L U ad S em A y e em VI S ad U ad Ac Ce qu’il faut retenir PL y Ac em SI y em VI S ad Jupyter est un environnement U ad Ac permettant de coder à travers des PL y notebooks Ac m S SI de LU Un notebook est constitué de cellules Introduction VI S de code et de texte a LU au Machine P Ac SI Learning y P em S SI A LU VI S Page 31 ad U P Ac L SI y IP m VI S IS de U SI Ac P LU ad Page 32 VI S e Learning SI Ac P au Machine Introduction LU ad S em Ac y a U de VI S m Ac y ad VI em SI y PL U de VI S m Chapitre 6 : SI y PL U Prendre en main Python et Numpy VI S SI Ac P LU ad IS S em IP Ac L U ad S em A y SI Ac P LU ad Page 33 VI S e Learning SI Ac P au Machine Introduction LU ad S em Ac y a U de VI S m Ac y ad VI em SI y PL U de VI S m SI y PL U VI S Démonstration n°02 SI Ac P LU ad IS S em IP Ac L U ad S em A y e em VI S ad U ad Ac Ce qu’il faut retenir PL y Ac em SI y em VI S ad Numpy est une bibliothèque de calculs U ad Ac matriciels extrêmement puissante PL y Ac m S SI Privilégier Numpy et délaisser un de LU maximum les boucles « for » Introduction VI S traditionnelles a LU au Machine P Ac SI Learning y L’élément de base est le ndarray P em S SI A LU VI S Page 34 ad U P Ac L SI y IP m VI S IS de U SI Ac P LU ad Page 35 VI S e Learning SI Ac P au Machine Introduction LU ad S em Ac y a U de VI S m Ac y ad VI em SI y PL U S Pandas de VI m Chapitre 7 : SI y PL U VI S SI Ac P LU ad IS S em IP Ac L U ad S em A y SI Ac P LU ad Page 36 VI S e Learning SI Ac P au Machine Introduction LU ad S em Ac y a U de VI S m Ac y ad VI em SI y PL U de VI S m SI y PL U VI S Démonstration n°03 SI Ac P LU ad IS S em IP Ac L U ad S em A y e em VI S ad U ad Ac Ce qu’il faut retenir PL y Ac em SI y em VI S ad Pandas est une bibliothèque U ad Ac s’appuyant sur Numpy PL y Ac m S SI Elle permet de traiter des données de LU tabulaires de manière pratique et Introduction VI S efficace a LU au Machine P Ac SI Learning y L’élément de base est le DataFrame P em S SI A LU VI S Page 37 ad U P Ac L SI y IP m VI S IS de U SI Ac P LU ad Page 38 VI S e Learning SI Ac P au Machine Introduction LU ad S em Ac y a U de VI S m Ac y ad VI em SI y PL U de VI S m Scikit-learn Chapitre 8 : SI y PL U VI S SI Ac P LU ad IS S em IP Ac L U ad S em A y SI Ac P LU ad Page 39 VI S e Learning SI Ac P au Machine Introduction LU ad S em Ac y a U de VI S m Ac y ad VI em SI y PL U de VI S m SI y PL U VI S Scikit-learn SI Ac P LU ad IS S em IP Ac L U ad S em A y SI Ac P LU ad Page 40 VI S e Learning SI Ac P au Machine Introduction LU ad S em Ac y a U de VI S m Ac y ad VI em SI y PL U de VI S m SI y PL U VI S SI Ac P Scikit-learn – v1.2 LU ad IS S em IP Ac L U ad S em A y e em VI S ad U Les autres bibliothèques ad Ac PL y Ac em SI y Deep Learning em Préparation VI S ad U ad Ac PL y Ac m S SI de LU Introduction VI S a LU au Machine P Ac SI Learning y P Typologie em S SI A Modèles LU VI S Page 41 ad U P Ac L SI y IP m VI S IS de U e em VI S ad U ad Ac PL y Ac em SI y em VI S ad U ad Ac Démonstration n°04 : PL y Ac m S SI de https://scikit- LU Introduction VI S a LU au Machine learn.org/stable/index.html P Ac SI Learning y P em S SI A LU VI S Page 42 ad U P Ac L SI y IP m VI S IS de U e em VI S ad U ad Ac Ce qu’il faut retenir PL y Ac em SI y em VI S ad Scikit-Learn est la bibliothèque U ad Ac incontournable de Machine Learning PL y Ac m S SI Pour des tâches plus spécialisées, de LU l’écosystème Python dispose Introduction VI S (forcément) d’une bibliothèque dédiée a LU au Machine P Ac SI Learning y P em S SI A LU VI S Page 43 ad U P Ac L SI y IP m VI S IS de U SI Ac P LU