Predicting Injury in Athletes Using Machine Learning & Workload Ratios PDF
Document Details
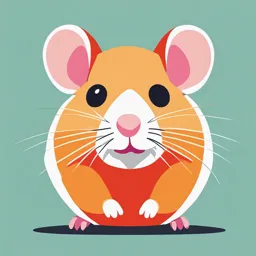
Uploaded by TruthfulRealism2101
Princess Nourah Bint Abdulrahman University
Tags
Summary
This document explores the use of machine learning and wearable technology to predict and prevent sports injuries. It analyzes the concept of the acute-to-chronic workload ratio, which assesses the relationship between short-term and long-term training loads to help identify potential injury risks. The document also discusses the use of sensors to collect data about athletic movements, like boxing punches.
Full Transcript
Welcome back. In this lesson. We will discuss the use of external sensors to try and predict and prevent injury. The idea of reducing injury by preventing over use is certainly not a new one. However, as long as there have been highly competitive athletes. There have been athletes that are likely to...
Welcome back. In this lesson. We will discuss the use of external sensors to try and predict and prevent injury. The idea of reducing injury by preventing over use is certainly not a new one. However, as long as there have been highly competitive athletes. There have been athletes that are likely to do too much regarding their training. I was told as a college cross country athlete. That the mileage that I was running was going to lead to injury. However, I also knew that training considerably less was going to lead to me having poor performances. So how to determine the optimal amount of training for performance while minimizing injury. This is probably the holy grail for coaches and trainers. The incorporation of wearable technology, has made it much easier to more fully characterize the training that is being performed. Next, we're going to talk about machine learning. What is machine learning. And video defines machine learning as the practice of using algorithms, the parse data learn from it. And then make a determination or prediction about something in the world. As we've mentioned, predicting the optimal training load to improve performance while limiting injury, is a major goal of wearable devices used by sport teams. Can machine learning be used to help with this? I believe the answer is yes. And so studies are exploring this topic. And will return to this important question shortly. However, we'll start with a simpler concept discussing the ability of wearable devices to predict what type of punches are being thrown by boxers. In a recently published scientific study. A catapult device was used to collect accelerometer and gyroscope data during boxing. The sensors were placed on the user's boxing gloves and wrist to collect data and in three axial directions. Next, the study was able to use machine learning. And the known signals of the boxing movements. In order to develop predictions of what punches were being thrown. Simply from the information coming from the accelerometers and gyroscopes recorded from the catapult device on the athletes back. Following the machine learning process, the authors demonstrated that machine learning could be used to detect the different striking patterns. And inaccuracy of about 90%. This study demonstrates that simple sensors are effective at discriminating between different striking patterns in boxing. For now, let's look closer at another finding that stem from machine learning the acute to chronic workload ratio. What is the acute chronic workload ratio? It is a simple computation of the ratio of the short term to long term workload. Which could be an internal or external measure, but we're currently focused on external measures. This ratio is thought to help predict the risk of injury. So that teams can avoid high risk training. The acute chronic workload ratio has two components, which need to be defined by the user or the team. Those two components are the acute phase and the chronic face. So what's the relationship between machine learning and the acute chronic workload ratio? Well, for decades, athletes and coaches have been forewarned about doing too much too soon. For example, it's been a common strategy to not increase training volume by more than 10% from week to week. In recent studies, machine learning has been used to evaluate many wearable technology measures while also examining injuries. It was through these studies that the acute chronic workload ratio was initially suggested to predict injury. We will move forward to examine the acute to chronic workload ratio. As it is being used to help guide coaches and trainers. However, there is currently considerable controversy about whether the acute to chronic workload ratio should be called in association with injury, a risk factor for injury or a predictor of injury. In this case, we are going to just evaluate, how much the ratio changes across a soccer season. So let's chat more about how people set up the ratio. And the information that exists about where injury risk is increase for athletes when using this ratio. So we mentioned that there are two components that need to be determined when using the acute chronic workload ratio. As we mentioned, there are two components to the acute to chronic workload ratio. In the acute phase. We're looking at the most recent work of the athlete. Usually this is described by a period of approximately one week. This phase is used as a measurement of the athletes state of fatigue. The chronic phase, on the other hand. Is the detection of the athletes load over a longer period of time. Usually it's a record of the last 3-6 weeks. This phase is used as a measurement of the athletes state of fitness and nowhere long term training load. Putting these together. This is the classic description of the acute to chronic workload ratio. And its relationship to injury. So the acute chronic workload ratio is probably not a radically new variable to be measured and followed. But it does provide the ability to quantify training on a day by day basis that was previously less feasible. The acute chronic workload ratio is being used to identify training load changes that represent an increased risk for overuse injuries. Let's take a look at this figure. So on the x axis we see the acute to chronic workload ratio. And on the y axis we see the likelihood of subsequent injury. The percentage and what we see is a curve. A linear relationship here where the lowest values are right around the value of 1.0 for the acute chronic workload ratio. So if you stop to think about what does this mean? What is suggesting is that the work in the acute window? That is, for example the last 3 to 7 days is consistent with the chronic window. That is the last 3 to 8 weeks. So within each study of the acute to chronic workload ratio. What needs to choose, what is that window that's going to be evaluated for the acute period. So in many cases we use seven days. And then the chronic period where we often use four weeks or 28 days. So a value of 1.0 suggests that the training load over the last week, is the same as the training load over the last month or four weeks. Where the problems exist in the red rectangle that we see here. Is when the acute to chronic workload ratio is above 1.5. And even greater when it's out at 2.0. And so what 2.0 would indicate is that the workload over the last week is twice what the chronic workload has been. And hence the increased likelihood of injury. For our assessment will use a season of soccer to evaluate how the acute chronic workload ratio changes across the competitive and championship portions of a season.