Statistic Refresher PDF
Document Details
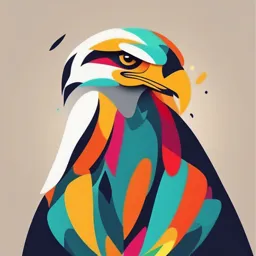
Uploaded by FabulousClematis2571
Engrid Manaloto, RPM
Tags
Summary
This document provides a refresher on statistics, covering topics such as distribution, skewness, measures of variability, quartiles, standard deviation, kurtosis, and Z-scores and T-scores. It discusses different types of correlations, and how to use them including scatterplots. The document also explains different statistical tests and their applications.
Full Transcript
STATISTIC REFRESHER BY; ENGRID MANALOTO, RPM 1. distribution may be defined as a set of test scores arrayed for recording or study. Th 2. a raw score is a straightforward, unmodified accounting of performance that is usually numerical. 3. A raw score may reflect a simple tally, as in numb...
STATISTIC REFRESHER BY; ENGRID MANALOTO, RPM 1. distribution may be defined as a set of test scores arrayed for recording or study. Th 2. a raw score is a straightforward, unmodified accounting of performance that is usually numerical. 3. A raw score may reflect a simple tally, as in number of items responded to correctly on an achievement test. 4. In a frequency distribution, all scores are listed alongside the number of times each score occurred. The scores might be listed in tabular or graphic form In a grouped frequency distribution, test-score intervals, also called class intervals, replace the actual test scores. The number of class intervals used and the size or width of each class interval (or, the range In most instances, a decision about the size of a class interval in a grouped frequency distribution is made on the basis of convenience. Positive skew Data Concentration: In a positively skewed distribution, most values are lower, with fewer high values that stretch the tail to the right. This often indicates that while most participants score lower, there are outliers with significantly higher scores The graph of a positively skewed distribution will show a peak (the mode) on the left side and a tail that extends towards the right. This asymmetry highlights the presence of extreme high values Interpretation of Positive Skew 1. Assessment of Learning: The presence of a positive skew can signal that the learning objectives were not met by most students. 2. Statistical Measures: In interpreting test scores with positive skewness, reliance on the mean can be misleading since it is affected by extreme values. Instead, using the median or mode may provide a better representation of typical performance among students 3. Recommendations for Future Tests: Based on the skewness observed, educators might adjust future assessments to better align with student capabilities. For instance, if a test is consistently yielding positive skewness, it may be beneficial to simplify questions or provide more preparatory resources Interpretation of Negative Skew 1. Assessment of Performance: A negatively skewed distribution often suggests that a test was too easy, as most students score above average. For example, if a majority of students score well on an exam but a few perform poorly, this would create a negative skew 2. Statistical Analysis: Understanding negative skewness is crucial for selecting appropriate statistical tests. Parametric tests assume normality; thus, when data is negatively skewed, non-parametric tests may be more suitable to avoid misleading results 3. Interpretation of Results: The presence of negative skew can lead to misinterpretations if only the mean is considered. Since the mean is pulled down by low scores, it may not accurately reflect the overall performance of the group. Using the median or mode can provide a clearer picture of typical performance levels Measures of Variability Statistics that describe the amount of variation in a distribution are referred to as measures of variability. Some measures of variability include the range, the interquartile range, the semi-interquartile range, the average deviation, the standard deviation, and the variance. The range of a distribution is equal to the difference between the highest and the lowest scores. PURPOSE OF RANGE 1. Indication of Spread: The range provides a basic indication of how spread out the values are in a dataset. 2. Initial Data Assessment: It serves as an initial tool for researchers to assess data dispersion. This can help identify whether further statistical analysis is necessary based on the observed spread of scores Quartiles Quartiles are statistical values that divide a dataset into four equal parts, each containing 25% of the data. They provide a way to understand the distribution and spread of data points in a dataset. Interquartile Range Measure of Variability: The IQR represents the range within which the central 50% of data points lie, providing insight into the dataset's variability while minimizing the influence of outliers. Robustness: Unlike the full range, which can be heavily affected by extreme values, the IQR focuses on the middle portion of the data, making it a more robust measure of spread. Standard Deviation Standard Deviation (SD): It measures how much individual data points deviate from the mean (average) of the dataset. A low standard deviation indicates that the data points are close to the mean, while a high standard deviation signifies that they are spread out over a wider range of values Kurtosis The term testing professionals use to refer to the steepness of a distribution in its center is kurtosis. To the root kurtic is added to one of the prefixes platy-, lepto-, or meso- to describe the peakedness/flatness of three general types of curves Kurtosis Distributions are generally described as platykurtic (relatively flat), leptokurtic (relatively peaked), or—somewhere in the middle—mesokurtic. According to the original definition, the normal bell-shaped curve would have a kurtosis value of 3. In other methods of computing kurtosis, a normal distribution would have kurtosis of 0, with positive values indicating higher kurtosis and negative values indicating lower kurtosis. Normal Curve At the beginning of the nineteenth century, Karl Friedrich Gauss made some substantial contributions. Through the early nineteenth century, scientists referred to it as the “Laplace-Gaussian curve.” Karl Pearson is credited with being the first to refer to the curve as the normal curve, perhaps in an effort to be diplomatic to all of the people who helped develop it is highest at its center. From the center it tapers on both sides approaching the X-axis asymptotically (meaning that it approaches, but never touches, the axis). In theory, the distribution of the normal curve ranges from negative infinity to positive infinity. The curve is perfectly symmetrical, with no skewness. z scoe and T score Raw scores may be converted to standard scores because standard scores are more easily interpretable than raw scores. With a standard score, the position of a testtaker’s performance relative to other testtakers is readily apparent. Different systems for standard scores exist, each unique in terms of its respective mean and standard deviations. A z score results from the conversion of a raw score into a number indicating how many standard deviation units the raw score is below or above the mean of the distribution If the scale used in the computation of z scores is called a zero plus or minus one scale, then the scale used in the computation of T scores can be called a fifty plus or minus ten scale; that is, a scale with a mean set at 50 and a standard deviation set at 10. T score Devised by W. A. McCall (1922, 1939) and named a T score in honor of his professor E. L. Thorndike, this standard score system is composed of a scale that ranges from 5 standard deviations below the mean to 5 standard deviations above the mean T score Purposes 1. Hypothesis Testing: T-scores are primarily used in hypothesis testing to determine whether there is a significant difference between the means of two groups. By comparing the t-score to critical values from the t-distribution, researchers can decide whether to reject or fail to reject the null hypothesis 2. Confidence Intervals: T-scores are also used to construct confidence intervals for estimating population parameters when the sample size is small and the population standard deviation is unknown. 3. Regression Analysis: In regression analysis, t-scores help assess the significance of individual predictors in a model. A high absolute t-score indicates that a predictor significantly contributes to explaining variance in the dependent variable. T score VS Z score Z-Scores vs. T-Scores: While both z-scores and t-scores measure how many standard deviations a point is from the mean, z-scores are used when the population standard deviation is known and typically for larger samples. T-scores are preferred for smaller samples or unknown population parameters. Stanine Researchers during World War II developed a standard score with a mean of 5 and a standard deviation of approximately 2. Divided into nine units, the scale was christened a stanine, a term that was a contraction of the words standard and nine. Stanine Stanine 1: Represents the bottom 4% of scores. Stanine 5: Represents the average performance (50th percentile). Stanine 9: Represents the top 4% of scores. Loss of Precision: While stanines simplify score interpretation, they also result in a loss of information. For instance, multiple individuals can receive the same stanine score despite having different raw scores, which can mask significant differences in performance. Applications 1. Educational Testing: Stanines are commonly used in standardized testing to provide a quick reference for educators and students about relative performance. 2. Performance Assessment: They help categorize students into broad performance bands (below average, average, above average) without getting bogged down in precise numerical differences. Normalised Standard Score One alternative available to the test developer is to normalize the distribution. Conceptually, normalizing a distribution involves “stretching” the skewed curve into the shape of a normal curve and creating a corresponding scale of standard scores, a scale that is technically referred to as a normalized standard score scale. Purposes Standardization of Scores: Normalization allows for the transformation of raw scores into standardized scores (e.g., z-scores or t-scores). This helps in comparing individual scores against a population mean, making it easier to understand where a particular score falls within the overall distribution. Purposes Facilitating Interpretation: By converting raw scores into normalized values, researchers can provide clearer interpretations. For instance, a t-score might indicate how many standard deviations a score is from the mean, allowing for quick assessments of performance relative to a normative group. Improving Data Comparability: Normalization helps in comparing scores from different tests or measures that may have different scales or distributions. This is particularly useful in psychological assessments where various instruments may be used to measure similar constructs. Methods of Normalizations Z-Scores: This method transforms raw scores into a scale where the mean is 0 and the standard deviation is 1. T-Scores: Similar to z-scores but adjusted so that the mean is 50 and the standard deviation is 10. This method is often used in educational and psychological testing to simplify interpretation. Percentiles indicate the relative standing of a score within a distribution. For example, a score in the 75th percentile means that the individual performed better than 75% of the population. Correaltion and Inferences A coefficient of correlation (or correlation coefficient) is a number that provides us with an index of the strength of the relationship between two things correlation is an expression of the degree and direction of correspondence between two things. A coefficient of correlation (r) expresses a linear relationship between two (and only two) variables, usually continuous in nature The coefficient of correlation is the numerical index that expresses this relationship: It tells us the extent to which X and Y are “co-related.” Positive Correlation A positive correlation occurs when two variables move in the same direction. As one variable increases, the other variable also increases, and vice versa. This relationship is quantified by a correlation coefficient (denoted as r) that ranges from 0 to +1. A coefficient closer to +1 indicates a stronger positive relationship. EXAMPLES Height and Weight: Generally, taller individuals tend to weigh more. Study Time and Test Scores: Increased study time is often associated with higher test scores. Negative Correlation A negative correlation, on the other hand, occurs when two variables move in opposite directions. As one variable increases, the other decreases. This relationship is represented by a correlation coefficient that ranges from 0 to -1, where values closer to -1 indicate a stronger negative relationship. EXAMPLES Sleep and Tiredness: More hours of sleep are generally associated with less tiredness. Exercise and Body Fat: Increased physical activity tends to correlate with lower body fat percentages. Pearson's correlation coefficient, often denoted as r, is a statistical measure that quantifies the strength and direction of the linear relationship between two continuous variables. Spearman Rho alternative statistic is variously called a rank-order correlation coefficient, a rank-difference correlation coefficient, or simply Spearman’s rho. Developed by Charles Spearman, a British psychologist this coefficient of correlation is frequently used when the sample size is small (fewer than 30 pairs of measurements) and especially when both sets of measurements are in ordinal (or rank-order) form. Does not assume normal distribution, linearity, or homoscedasticity. It is robust to outliers since it relies on ranks. Calculated based on the ranks of the data. If there are tied ranks, average ranks are used. Values range from -1 to +1, where +1 indicates a perfect positive monotonic relationship, -1 indicates a perfect negative monotonic relationship, and 0 indicates no monotonic relationship. Scatterplot Values range from -1 to +1, where +1 indicates a perfect positive monotonic relationship, -1 indicates a perfect negative monotonic relationship, and 0 indicates no monotonic relationship. Scatterplot Purposes Display Relationships: Scatterplots effectively illustrate the relationship between two quantitative variables by plotting data points on a two-dimensional graph. Correlation Analysis: They help identify the type and strength of correlation (positive, negative, or none) between variables. Outlier Identification: Scatterplots can reveal outliers—data points that deviate significantly from the overall pattern. Theory Testing: They are useful for testing hypotheses about relationships between variables Scatterplot Limitations Clutter and Confusion: When there are too many data points, they can overlap, making it difficult to discern patterns or relationships. Dimensionality: Scatterplots typically visualize the relationship between only two variables at a time Assumption of Linearity: Scatterplots primarily highlight linear relationships. If the relationship is non-linear (e.g., exponential or quadratic), it may not be adequately represented, leading to potential misinterpretation of the data Misinterpretation: A common misconception is that correlation implies causation. Just because two variables appear related in a scatterplot does not mean one causes changes in the other; other confounding factors may be at play Sensitive to Outliers Subjective Analysis: The interpretation of scatterplots can be subjective, as different observers may perceive relationships differently based on visual cues, potentially leading to inconsistent conclusions Question & Answer THANK YOU