STAT 105 (Introduction to Statistical Analysis) PDF
Document Details
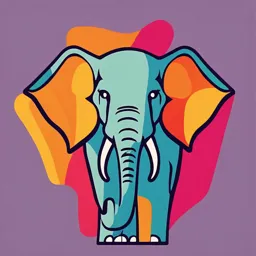
Uploaded by PanoramicLyric
Tags
Summary
This study guide provides an introduction to the world of statistics, including its importance, uses, and misuses. It covers key concepts and descriptive statistical tools. It also outlines a learning plan for the course, including reading materials, videos, activities, and a quiz.
Full Transcript
STAT 105 (Introduction to Statistical Analysis) UNIT 1: PRELIMINARIES STUDY GUIDE Let’s Begin. _______________________________________________________________________________ Our first topics in this course gives you an introduction to the world of Statistics. We are going to tackle...
STAT 105 (Introduction to Statistical Analysis) UNIT 1: PRELIMINARIES STUDY GUIDE Let’s Begin. _______________________________________________________________________________ Our first topics in this course gives you an introduction to the world of Statistics. We are going to tackle the importance of Statistics and its common misuses. We are also going to revisit important basic terms and concepts in Statistics that most of you are already familiar with. These terminologies will be relevant to succeeding sections of the course. Some useful descriptive statistical tools will also be discussed. Bear in mind the following target learning outcomes for this unit: Determine the importance of Statistics Illustrate the different uses and misuses of Statistics Demonstrate knowledge of introductory statistical terms and concepts Classify the different types of variables Differentiate between array and frequency distribution of any data Organize data using a frequency distribution for both numerical and categorical data Determine the appropriate use of the different measures of data description Calculate the different measures of central tendency, location, and dispersion for numerical data. Construct and interpret graphical statistics given data. Read. Watch. Take. _______________________________________________________________________________ 1. The first thing you need to do this week is to watch a video which highlights the importance of Statistics in everyday life. You can watch this video by clicking this link https://www.youtube.com/watch?v=ogeGJS0GEF4. As you watch this video, try to list down the reasons why you need to study Statistics. You can also watch a longer BBC documentary about the “The Joy of Stats” by Hans Rosling made in 2010 but still gives a good history and relevance of Statistics in our world. You can watch it thru this link https://www.gapminder.org/videos/the-joy-of-stats/. You can also watch a more recent BBC documentary in 2016 entitled “The Joy of Data” narrated by Dr. Hannah Fry. This documentary tackles the emergence of data and how to capture, store, share, and analyze these data. You can watch it through this link https://youtu.be/l6oKriR-RjM. 2. Next is to read the handout/ material following this study guide. In reading the module, take note of the different terms presented and try to differentiate these terms from each other. It will be better if you write down these terms together with the main word/s used to define them. Many of these terms will be asked in our first quiz. Also, do some of the exercises and discuss your answers in the designated discussion forum. 3. Read additional examples on the misuse of statistics in research and advertising at https://www.datapine.com/blog/misleading-statistics-and-data/. 1 STAT 105 (Introduction to Statistical Analysis) 4. Additional reading on descriptive and graphical statistics is given by Chapter 2 of Illosky, B., Dean, S. 2013. Introductory Statistics available for free at https://openstax.org/details/books/introductory-statistics. This OER is licensed under the Creative Commons Attribution v4.0 International License. 5. Attend our first synchronous meeting on September 20, 2023, 2:00 – 3:00 pm. Zoom details are as follows: Meeting ID: 931 5101 8107 Passcode: statistics 6. Take the Quiz 1 in our LMS. This quiz will assess your basic understanding of the terms and concepts discussed for this Unit. You can attempt to take the quiz at any time on September 23, 2022. 7. Install and learn how to use jamovi in generating different statistics and plots. Go to your Laboratory section and watch the series of videos to learn to use jamovi. Think. _______________________________________________________________________________ 1. After reading and watching our first set of course materials, answer and submit Problem Set 1 by accessing our Stat-105-Problem-Set-Guide-1 in the LMS. Deadline of submission is on September 23, 2021, 6pm. 2. To apply the tools you learned in this unit, finish and submit your Laboratory Report 1 which can be accessed in your LMS laboratory course. This is accompanied by the Stat-105- Laboratory-Activity-Guide-1 which provides details about this activity. 3. To further optimize your learning, answer the following questions and keep your answers in mind. These questions may also be asked in our discussion forums. a. Are there researches conducted without the help of Statistics? b. What other examples can you think of when statistics was used to influence or sell? c. If most statistics are false, can we do away with statistics? d. Can we use the standard deviation to compare variability of two or more datasets? e. When do we use the median over the mean? Dig Deeper. _______________________________________________________________________________ To gain broader knowledge on the role of Statistics in researches and everyday happenings, you can do the following in your free time: Look at newspapers or online news platform and notice the use of statistics (stocks, GDP, COVID-19, etc) Read on the importance of statistics in stages of vaccine development. Check out (Illosky, B., Dean, S. 2013. Introductory Statistics) practice exercises on descriptive statistics (pp. 125 – 160) with solutions on selected items (pp. 162 – 172). Gapminder is an educational nonprofit organization dedicated to “fight devastating ignorance with a fact-based worldview everyone can understand.“ You can visit their website https://www.gapminder.org/ to see and learn world facts backed up with data and statistics. If you find something interesting and you are curious about, feel free to post it as a discussion topic in our LMS. 2 STAT 105 (Introduction to Statistical Analysis) COURSE HANDOUT _______________________________________________________________________________ 1.1. Statistics, its Uses and Misuses Definition of Statistics In its plural sense, statistics is a set of numerical data (e.g., vital statistics in a beauty contest, monthly sales of a company, daily P - $ exchange rate). In its singular sense, Statistics is that branch of science which deals with the collection, presentation, analysis, and interpretation of data. What is the Role of Statistics in any Scientific Investigation? Validity - Will this study help answer the research question? Analysis - What analysis and how should this be interpreted and reported? Efficiency - Is the experiment the correct size making best use of resources? What are Some Uses of Statistics? Some of the reasons why you will be using statistics to analyze your data are the same reasons why you are doing the research. You are likely to be conducting research because you want to: measure things examine relationships make predictions test hypotheses construct concepts and develop theories explore issues explain activities or attitudes describe what is happening present information make comparisons to find similarities and differences draw conclusions about populations based only on sample results Misuses of Statistics A misuse of statistics is a pattern of unsound statistical analysis. They are variously related to data quality, statistical methods, and interpretations. Statistics are occasionally misused to persuade, influence and sell. Misuse can also result from mistakes of analysis that result in poor decisions and failed strategies. The following are common misuses of statistics. 1. False Analogy – A comparison or analogy that is technically valid but that has little or no practical meaning. Implies that a comparison has been designed to be misleading. 2. Biased Labeling – Misleading labels on a graph. 3. Biased Samples – Poor quality samples such as answers to leading questions. 4. Cognitive Biases – Misinterpretations of numbers due to flawed logic. 5. Data Dredging – Looking for patterns in data using brute force methods that try a large number of statistical models until matches are found. 6. Overcomplexity – Graphs and data visualizations that are too complex to be interpreted by your audience. This may prevent data from being challenged and validated. 7. Overfitting – Testing too many theories against data such that random patterns are sure to be found. 3 STAT 105 (Introduction to Statistical Analysis) 8. Prosecutor’s Fallacy – A general term for an invalid interpretation of a valid statistic. 9. Significance – Basing analysis on a statistically insignificant number of samples. 10. Tyranny of Averages – It is a term for overuse of averages in statistical analysis and decision making. It refers to a situation in which an average is relatively meaningless due to the shape of a data distribution. 11. Garbage in - Garbage out (GIGO) – It is the observation that processes, procedures and technologies require meaningful input to produce a meaningful result. 1.2. Basic Terms and Concepts Fields of Statistics 1. Statistical Methods of Applied Statistics - refer to procedures and techniques used in the collection, presentation, analysis, and interpretation of data. There are two branches: Descriptive Statistics and Inferential Statistics. Descriptive Statistics - methods concerned with the collection, description, and analysis of a set of data without drawing conclusions or inferences about a larger set Inferential Statistics - methods concerned with making predictions or inferences about a larger set of data using only the information gathered from a subset of this larger set 2. Statistical Theory of Mathematical Statistics - deals with the development and exposition of theories that serve as bases of statistical methods. Population and Sample Definition. A population is a collection of all the elements under consideration in a statistical study. Definition. A sample is a part or subset of the population from which the information is collected. Example: A manufacturer of kerosene heaters wants to determine if customers are satisfied with the performance of their heaters. Toward this goal, 5,000 of his 200,000 customers are contacted and each is asked, “Are you satisfied with the performance of the kerosene heater you purchased?” Identify the population and sample. In this example, the population of interest consists of all customers who purchased the kerosene heater while the sample consists of those who were contacted to give their level of satisfaction on the product they purchased. There are 200,000 customers in the population and there are 5,000 customers in the sample. Parameter and Statistic Definition. A parameter is a numerical characteristic of the population. Definition. A statistic is a numerical characteristic of the sample. 4 STAT 105 (Introduction to Statistical Analysis) Example: In order to estimate the true proportion of students at a certain college who smoke cigarettes, the administration polled a sample of 200 students and determined that the proportion of students from the sample who smoke cigarettes is 0.12. Identify the parameter and the statistic. The population consists of all students at the college of interest while the sample consists of those 200 students who were asked if they smoke cigarette or not. The parameter in this case is the proportion of students in the college who smoke cigarette while the statistic is the proportion of students in the sample who smoke cigarette. The value 0.12 is a realized value of the statistic. Variables and Other Terms Definition. A variable is a characteristic or attribute of persons or objects which can assume different values or labels for different persons or objects under consideration. Definition. A measurement is the process of determining the value or label of a particular variable for a particular experimental or sampling unit. Definition. An experimental unit or sampling unit is the individual or object on which a variable is measured. Definition. An observation is a numerical recording of information on a variable. Definition. Data is a collection of observations. Types of Variables 1. Qualitative vs Quantitative Qualitative variable - a variable that yields categorical responses (e.g. political affiliation, occupation, marital status) Quantitative variable - a variable that takes on numerical values representing an amount or quantity (e.g. weight, height, no. of cars) 2. Discrete vs Continuous Discrete variable - a variable which can assume finite, or, at most, countably infinite number of values; usually measured by counting or enumeration (e.g. no. of cars, no. of defective items in a lot) Continuous variable - a variable which can assume infinitely many values corresponding to a line interval (e.g. weight, height, length) Remark: Only quantitative variables are further classified whether they are discrete or continuous. 5 STAT 105 (Introduction to Statistical Analysis) Levels of Measurement Variables are measured on different measurement scales that goes from “weak” to “strong” depending on the amount or precision of information available in the scale. The four level of measurements are as follows: 1. Nominal Level (or Classificatory Scale) The nominal level is the weakest level of measurement where numbers or symbols are used simply for categorizing subjects into different groups. Examples: Sex M-Male F-Female Marital status 1-Single 2-Married 3-Widowed 4-Separated 2. Ordinal Level (or Ranking Scale) The ordinal level of measurement contains the properties of the nominal level, and in addition, the numbers assigned to categories of any variable may be ranked or ordered in some low-to-high-manner. Examples: Teaching ratings 1-poor 2- fair 3-good 4-excellent Year level st 1-1 yr 2 – 2 yr nd 3 – 3 yr rd 4 – 4th yr 3. Interval Level The interval level is that which has the properties of the nominal and ordinal levels, and in addition, the distances between any two numbers on the scale are of known sizes. An interval scale must have a common and constant unit of measurement. Furthermore, the unit of measurement is arbitrary and there is no “true zero” point. Examples: IQ, Temperature (in Celsius) 4. Ratio Level The ratio level of measurement contains all the properties of the interval level, and in addition, it has a “true zero” point.. Examples: Age (in years), Number of correct answers in an exam Remarks: 1. Some variables can be measured in different scales. For example, actual age is measured in the ratio level, but some uses age groups which are measured in at most the interval scale. Age can also be measured in terms of young or old which is in the ordinal level or in some cases measured in a nominal scale. It is the responsibility of the investigators to determine the appropriate level of measurement they must use to answer the objectives of the study. 2. Quantitative variables are naturally measured in at least interval scale but can be transformed into lower level of measurements. Qualitative variables are measured in either nominal or ordinal scales. 6 STAT 105 (Introduction to Statistical Analysis) 1.3. Data Organization and Management In dealing with one data set involving a single variable, data organization is straightforward. From the raw data, we are going to organize it into an array and eventually into a useful frequency distribution. However, when dealing with multiple variables, we cannot just rearrange these values because we need to maintain one-to-one correspondence of each observation with the variable and the sampling or experimental unit. Definition. The raw data is the set of data in its original form. Example: Final grades of Stat 105 Students 82 82 83 79 72 71 84 59 77 50 87 83 82 63 75 50 85 76 79 68 69 62 79 69 74 53 73 71 50 76 57 81 62 72 88 84 80 68 50 74 84 71 73 68 71 80 72 60 81 89 94 80 84 81 50 84 76 75 82 76 53 91 69 60 89 79 59 62 79 82 72 81 60 84 68 66 94 77 78 87 75 86 82 74 73 72 84 51 50 69 75 70 77 87 86 77 75 96 66 87 73 84 68 85 62 87 92 69 52 65 Definition. An array is an arrangement of observations according to their magnitude, either in increasing or decreasing order. Example: Final grades of Stat 105 Students arranged in an array 50 57 63 69 72 74 77 80 82 84 87 50 59 65 69 72 75 77 80 82 84 87 50 59 66 69 72 75 77 80 82 85 88 50 60 66 69 72 75 77 81 83 85 89 50 60 68 70 73 75 78 81 83 86 89 50 60 68 71 73 75 79 81 84 86 91 51 62 68 71 73 76 79 81 84 87 92 52 62 68 71 73 76 79 82 84 87 94 53 62 68 71 74 76 79 82 84 87 94 53 62 69 72 74 76 79 82 84 87 96 Remark: 1. Arrays help us in easier detection of the smallest and largest value as well as finding the measures of position/location. 2. The data presented above are also called ungrouped data. An ungrouped data set contains information on each member of a sample or population individually. 7 STAT 105 (Introduction to Statistical Analysis) Definition. A frequency distribution for quantitative data lists all the classes and the number of values that belong to each class. Data presented in the form of a frequency distribution are called grouped data. Definition of terms 1. Class frequency - the number of observations falling in the class 2. Class interval - the numbers defining the class 3. Class limits - the end numbers of the class 4. Class boundaries - the true class limits; the lower class boundary (LCB) is usually defined as halfway between the lower class limit of the class and the upper class limit of the preceding class while the upper class boundary (UCB) is usually defined as halfway between the upper class limit of the class and the lower class limit of the next class 5. Class size - the difference between the upper class boundaries of the class and the preceding class; can also be computed as the difference between the lower class boundaries of the current class and the next class; can also be computed by using the respective class limits instead of the class boundaries 6. Class mark (CM) - midpoint of a class interval 7. Open-end class - a class that has no lower limit or upper limit Examples: Using the Stat 105 Final Grade Data Class Freq. LCB UCB CM 50 – 55 10 49.5 55.5 52.5 56 – 61 6 55.5 61.5 58.5 62 – 67 8 61.5 67.5 64.5 68 – 73 24 67.5 73.5 70.5 74 – 79 22 73.5 79.5 76.5 80 – 85 25 79.5 85.5 82.5 86 – 91 11 85.5 91.5 88.5 92 – 97 4 91.5 97.5 94.5 or Class Freq. LCB UCB CM 50 – 54 10 49.5 54.5 52 55 – 59 3 54.5 59.5 57 60 – 64 8 59.5 64.5 62 65 – 69 13 64.5 69.5 67 70 – 74 17 69.5 74.5 72 75 – 79 19 74.5 79.5 77 80 – 84 22 79.5 84.5 82 85 – 89 13 84.5 89.5 87 90 – 94 4 89.5 94.5 92 95 – 99 1 94.5 99.5 97 Note: As we can see in the above example, we can arrive at different frequency distributions depending on the class size, the smallest LCL, etc., even though we used the same data set. Of course, these distributions yield different interpretations, thus, constructions and comparisons of these distributions should be made with caution. 8 STAT 105 (Introduction to Statistical Analysis) Steps in Constructing a Frequency Distribution Table 1. Determine the number of classes. There must be an adequate number of classes to show the essential characteristics of the data; at the same time, there should not be too many classes that it is already difficult to grasp the picture of the distribution as a whole. There are no precise rules concerning the optimal number of classes but Sturges’ formula can be used as a first approximation. Sturges’ formula: K = 1 + 3.322 log n where K is the approximate number of classes n is the number of observations 2. Determine the approximate class size. Whenever possible, all classes should be of the same size. The following steps can be used to determine the class size. Solve for the range, R = max – min. Compute for C’ = R K. Round-off C’ to a convenient number to work with, say C, and use C as the class size. 3. Determine the lowest class limit. The first class must include the smallest value in the data set. 4. Determine all class limits by adding the class size, C, to the limit of the previous class. 5. Tally the frequencies for each class. Sum the frequencies and check against the total number of observations. Remark: There are cases that standards are in placed in terms of coming up with ranges or intervals such as those published by government agencies. If this is the case, we use these standards for comparability, and we need not to compute for the number of classes as described in Step 1 above. Variations of the Frequency Distribution There are variations in presenting frequency distributions such as those which include percentages and cumulative frequencies. 1. Relative Frequency (RF) Distribution and Relative Frequency Percentage (RFP) RF = class frequency no. of observations RFP = RF * 100% 2. Cumulative Frequency Distribution (CFD) - shows the accumulated frequencies of successive classes, beginning at either end of the distribution Greater than CFD – shows the no. of observations greater than the LCB Less than CFD – shows the no. of observations less than the UCB 9 STAT 105 (Introduction to Statistical Analysis) Example: Construct and interpret a frequency distribution for the following dataset about task completion times (in seconds) of participants aged 25 to 29 in a hackathon. Use Sturges’ formula to approximate the number of classes. 1595 1472 1820 1580 1804 1635 1959 2020 1480 1250 2083 1522 1306 1572 2296 1445 1716 1618 1824 1778 Solution: In this example, n=20 and using the Sturges’ formula yields K = 1 + 3.322 ∗ log(20) = 5.32 ≈ 5 classes. From the given data, the maximum value is 2296 seconds and the minimum value is 1250 seconds. Thus, R = max − min = 2296 − 1250 = 1046 R C ′ = = 209.2 K It is convenient to take C as the next integer larger than C’, in this case, C = 210. The lowest class limit is usually the lowest observation in the data, in this case 1250. Adding the class size of 210 from 1250 results to the following class intervals. Tallying the observations and determining several additional features such as the class boundaries, class marks, relative frequencies and cumulative frequencies, the frequency distribution table is as follows: Class Freq. LCB UCB CM RF RFP CF 1250 - 1459 3 1249.5 1459.5 1354.5 0.15 15.0 3 20 1460 - 1669 8 1459.5 1669.5 1564.5 0.40 40.0 11 17 1670 - 1879 5 1669.5 1879.5 1774.5 0.25 25.0 16 9 1880 - 2089 3 1879.5 2089.5 1984.5 0.15 15.0 19 4 2090 - 2299 1 2089.5 2299.5 2194.5 0.05 5.0 20 1 Majority of the participants (40.0%) finished the task in 1460 to 1669 seconds or 24.3 to 27.8 minutes. Three participants or 15% of them have the fastest completion time (1250 to 1459 seconds) while only 1 participant (5.0%) of them took the longest time of 2090 to 2299 seconds to complete the task. Out of 20 participants, 16 or 80% of the them finished the task in at most 1879 seconds while 17 out of 20 participants or 85% finished it in at least 1460 seconds. Remark: Notice that the above components of a frequency distribution are only possible for quantitative variables. Constructing frequency distributions for qualitative variables involves enumerating categories of the variable and counting the number of observations under those categories. A relative frequency and its corresponding percentage can also be added. 10 STAT 105 (Introduction to Statistical Analysis) Data Management We now focus on some important data management procedures we can use in dealing with data sets having multiple variables which are measured in different scales. In practice, preparing our data to be suited for the intended analysis may consume 75% of the total effort in data analysis. This involves encoding the data following some coding manuals/scheme, cleaning the data, transforming variables, merging datasets, and in some cases analyzing and imputing missing observations. Procedures in Processing Data: 1. Source of Data - Take note of the sources of your data. Who collected the observations? How many sampling units, variables, and observations should you expect? Skim through these raw files and try to determine if values make sense. This will help you pinpoint some errors on the spot and correct them immediately. 2. Encode the Data - For most studies, we need to encode the data in a spreadsheet. A coding guide (coding manual) is often used to facilitate uniform encoding. As a rule-of-thumb, columns in a spreadsheet are reserved for the variables while rows identify the sampling/experimental units. Thus, the number of rows corresponds to the number of units/respondent/cases included in the study while the number of columns should be related to the number of variables involved in the study. Carefully check all number and characters encoded. Missing observations should be properly encoded to differentiate it with encoder-induced errors. Any problems that arise in this process must be resolved before proceeding further. 3. Clean the Encoded Data - Having hundreds of observations to encode and check can be tiresome and prone to errors and miscoding. Logic checks should be done depending on the structure of the data. For each variable/column, check whether values under it conforms to possible values indicated in the coding manual. If there are errors or miscoded values were encountered, check the original files and correct these observations accordingly. 4. Save and Keep the Original - After cleaning the data, save the final version of your raw data. Keep a copy of the original cleaned data and create multiple copies for further processing. 5. Compute, Recode, or Transform Variables - There are some variables that need to be transformed such as from actual age to some age groups, or using height and weight to compute for the BMI. This can be done manually or by the use of command or formulas in the spreadsheet application or statistical software. Retaining the original variables and creating new columns for these new variables is always a good idea than overwriting it. 6. Ready for Analysis - Your data is now ready for analysis. Remark: For this course, we are going to use Excel or any spreadsheet in managing our data. A free and open-sourced statistical software called jamovi will also be used to further prepare our data and to come up with the actual analysis. The first laboratory activity of the course will focus on managing data using Jamovi and come up with some descriptive measures that we are going to discuss in the next sections. 11 STAT 105 (Introduction to Statistical Analysis) jamovi jamovi is a free, open-source application that is enough to perform basic statistical analysis and at the same time can be powerful because it is based on the powerful statistical programming language R. According to their website, jamovi.org, jamovi aims to be community driven, meaning anyone can contribute to its development, giving way to new statistical methodologies to have a platform to be implemented and made readily available to users. When first starting jamovi, you will be presented with a user interface which looks something like the figure below. It has a spreadsheet window at the left side, an output window at the right side, and tabs section at the top to perform statistical analysis. A User’s Manual is available at https://www.jamovi.org/user-manual.html. Exercises Given the following subset data from the Family Income and Expenditure Survey (FIES) in the next page and provided in the LMS, do the following: 1. Identify the variables included and for each of these variables, determine whether it is quantitative or qualitative, whether it is discrete or continuous, and the level of measurement used. Post your answers in the designated discussion forum. 2. For those with laptops, install jamovi and try to encode the data. You may start with 2 to 5 variables only or even the whole data set since the excel file is also provided. Make sure to properly indicate the level of measurements and data labels. Take a screenshot of your work and post it in the designated discussion forum. 3. With or without laptops, select one or two variables and create frequency distributions of the observations and let your classmates come up with an interpretation of your work. 12 13 STAT 105 (Introduction to Statistical Analysis) 1.4. Descriptive Statistics Suppose that a variable X is the variable of interest and n observations are taken denoted by X1, X 2, … , X n. For example, to evaluate effectiveness of a processor for a certain type of tasks, we recorded the CPU time for n = 30 randomly chosen jobs (in seconds), 34 8 80 49 56 9 84 50 37 31 59 41 51 101 32 52 45 55 76 33 62 58 34 27 37 52 58 59 54 42 In this section, we are going to discuss common descriptive statistics that measures the central tendency, other locations, spread/variability, the skewness, and heaviness of the tails of any data. As a working example, we are going to use the CPU data to illustrate the use of these statistics. In order to fully understand how these statistics work, we first discuss summation notation. Summation Notation Definition. The summation notation provides a compact way of writing statistical formulas. The capital Greek letter sigma, Σ, is the mathematical symbol used to denote the sum of numerical values. Suppose that a variable X is the variable of interest and n observations are taken denoted by X1, X 2, … , X n. Then, we can write the sum of n observations as n ∑ X i = X1 + X 2 + ⋯ + X n i=1 where X i= value of the variable for the ith observation i = index of the summation 1 = lower limit of the summation n = upper limit of the summation. Example: Using the CPU Time data, we have the following results: n ∑ X i = X1 + X 2 + ⋯ + X 30 = 34 + 8 + ⋯ + 42 = 𝟏, 𝟒𝟔𝟔 i=1 n ∑ X i2 = X12 + X 22 + ⋯ + X 30 2 = (34)2 + (8)2 + ⋯ + (42)2 = 𝟖𝟑, 𝟒𝟐𝟐 i=1 n ∑(X i − 50)2 = (X1 − 50)2 + (X 2 − 50)2 + ⋯ + (X 30 − 50)2 i=1 = 256 + 1764 + ⋯ + 64 = 𝟏𝟏, 𝟖𝟐𝟐 Some Results on Summation 1. The summation of the sum of variables is the sum of their summations. n n n n ∑(a i + bi + ⋯ + zi ) = ∑ a i + ∑ bi + ⋯ + ∑ zi i=1 i=1 i=1 i=1 2. If c is a constant, then n n ∑ cXi = c ∑ Xi i=1 i=1 3. If c is a constant then n ∑ c = nc i=1 14 STAT 105 (Introduction to Statistical Analysis) Measures of Central Tendency Definition. A measure of central tendency is any single value that is used to identify the “center” or the typical value of a data set. It provides a summary of the data which facilitates comparison of two or more data sets. It is often referred to as the average. The Arithmetic Mean Definition. The arithmetic mean of a data set or simply the mean is the sum of all values of the observations divided by the number of observations. To find the mean of a data set, use one of the following formulas: N n Xi X i Population Mean: i 1 Sample Mean: X i 1 N n Characteristics of the Mean - Employs all available information - Strongly influenced by extreme values (outliers) - May not be an actual number in the data set - Possesses two mathematical properties that will prove to be important in the subsequent analyses: (a) the sum of the deviations of the values from the mean is zero (b) the sum of the squared deviations is minimum when the deviations are taken from the mean - Always exists and is unique - If a constant c is added (subtracted) to all observations, the mean of the new observations will increase (decrease) by the same amount c - If all observations are multiplied or divided by a constant, the new observations will have a mean that is the same constant multiple of the original mean. The Median Definition. The median, Md, is the value that divides the array into two equal parts. If the number of observations, n, is odd, then the median is given by Md = X (n+1), the 2 n+1 ( 2 )th observation in the array. If n is even, then the median is the average of the 1 two middle values in the array, i.e., Md = 2 (X (n) + X (n+1) ). 2 2 Characteristics of the Median - The median is a positional measure. - The median is affected by the position of each item in the series but not by the value of each item. This means that extreme values affect the median less than the arithmetic mean. 15 STAT 105 (Introduction to Statistical Analysis) The Mode Definition. The mode, Mo, is the observed value that occurs most frequently in a data set. It locates the point where the observation values occur with the greatest density which is determined by counting the frequency of each value and finding the value with the highest frequency of occurrence. Characteristics of the Mode - It does not always exist; and if it does, it may not be unique. A data set is said to be unimodal if there is only one mode, bimodal if there are two modes, trimodal if there are three modes, and so on. - It is not affected by extreme values. - The mode can be used for qualitative as well as quantitative data. Example: Using the CPU Time data, the mean, median, and mode are as follows: ∑ni=1 X i 1 Mo = 34,37,52,58,59 ̅ X= Md = (X n + X (n+1) ) n 2 (2 ) 2 1,466 1 = = (X 30 + X 30 ) 30 2 (2) ( +1) 2 = 48.87 1 = (X (15) + X (16) ) 2 1 = (50 + 51) 2 = 50.50 On the average, the CPU time for these jobs is 48.87 seconds. Half of the 30 jobs were finished in at least 50.50 seconds while half is at most 50.50 seconds. Multiple modes exist. In this case, the mode is not a good measure of central location. Both the mean and median can be used to describe the center of the distribution of CPU time. Other Measures of Location Definition. Measures of location (or fractiles/quantiles) are values below which a specified fraction or percentage of the observations in a given set must fall. It provides the relative position of an observation in an array. The Percentiles Definition. Percentiles are values that divide a set of observations in an array into 100 equal parts. Thus, P1, read as first percentile, is the value below which 1% of the values fall. P2, read as second percentile, is the value below which 2% of the values fall. ⋮ P99, read as ninety-ninth percentile, is the value below which 99% of the values fall. To compute for the ith percentile: i(n+1) Pi = the value of the [ 100 ]th observation in the array 16 STAT 105 (Introduction to Statistical Analysis) The Deciles Definition. Deciles are values that divide the array into 10 equal parts. Thus, D1, read as first decile, is the value below which 10% of the values fall. D2, read as second decile, is the value below which 20% of the values fall. ⋮ D9, read as ninth decile, is the value below which 90% of the values fall. The Quartiles Definition. Quartiles are values that divide the array into 4 equal parts. Thus, Q1, read as first quartile, is the value below which 25% of the values fall. Q2, read as second quartile, is the value below which 50% of the values fall. Q3, read as third quartile, is the value below which 75% of the values fall. Example: Using the CPU Time Data, the 35th percentile, 8th decile, and the 3rd quartile are as follows: P35 = X 35(30+1) D8 = X 80(30+1) Q3 = X 75(30+1) ( ) ( ) ( ) 100 100 100 = X (10.85) = X (24.8) = X (23.25) = X (10) + 0.85 ∗ (X (11) − X (10) ) = X (24) + 0.8 ∗ (X (25) − X (24) ) = X (23) + 0.25 ∗ (X (24) − X (23) ) = 37 + 0.85 ∗ (41 − 37) = 59 + 0.8 ∗ (59 − 59) = 58 + 0.25 ∗ (59 − 58) = 40.4 = 59.0 = 58.25 This means that 35% of the jobs were finished in at most 40.4 seconds, 75% were done in at most 58.25 seconds and 80% of the jobs fall below 59.0 seconds. Measures of Dispersion Definition. Measures of dispersion indicate the extent to which individual items in a series are scattered about an average. Some Uses for Measuring Dispersion - to determine the extent of the scatter so that steps may be taken to control the existing variation - used as a measure of reliability of the average value The Standard Deviation and the Variance Definition. The variance is the average squared difference of each observation from the mean while the standard deviation is just the positive square root of the variance. For a finite population of size N, the population variance and standard deviation are as follows: N N X i X 2 2 i Variance: SD: 2 i 1 i 1. N N 17 STAT 105 (Introduction to Statistical Analysis) For a sample of size n, the sample variance and standard deviation are X X n n 2 2 i X i X Variance: s 2 i 1 SD: s i 1. n 1 n 1 Characteristics of the Variance and Standard Deviation - The standard deviation is the most frequently used measure of dispersion. - The variance is not a measure of absolute dispersion. It is not expressed in the same units as the original observations. - It is affected by the value of every observation. It may be distorted by few extreme values. - If each observation of a set of data is transformed to a new set by the addition (or subtraction) of a constant c, the standard deviation of the new set of data is the same as the standard deviation of the original data set. - If a set of data is transformed to a new set by multiplying (or dividing) each observation by a constant c, the standard deviation of the new data set is equal to the standard deviation of the original data set multiplied (or divided) by c. The Coefficient of Variation Definition. The coefficient of variation, CV, is the ratio of the standard deviation to the mean and is usually expressed in percentage. It is computed as s Population: CV 100% Sample: CV 100% X Characteristics of the Coefficient of Variation - It can be used to compare the variability of two or more data sets even if they have different means or different units of measurement. - It expresses the standard deviation as a percentage of the mean. - Large value of CV indicates that the data set is highly variable. - Cannot be computed when the mean is zero and is meaningless when the mean is negative. Example: Using the CPU Time data, the sample variance, standard deviation, and coefficient of variation are as follows: n 1 1 2 s = ∑(X i − ̅ X)2 = (11,783.47) = 406.33 n−1 30 − 1 i=1 s = √s 2 = √406.33 = 20.16 s 20.16 CV = ∗ 100% = ∗ 100% = 41.25% ̅ X 48.87 Thus, the average squared difference about the mean is 20.16 and this variability is 41.25% of the mean. 18 STAT 105 (Introduction to Statistical Analysis) 1.5. Graphical Statistics Before coming up with any formal statistical methods and approaches, it is a good practice to first explore the data by coming up with some graphical statistics. Through these graphical statistics, we can come up with obvious trends and patterns in the data, insights on outliers and other perturbations, the heterogeneity that will give us confidence in the accuracy of the results, and even apparent relationships among variables. In this section, we are going to construct and discuss histograms, stem-and-leaf display, boxplots, and parallel boxplots. Frequency and Relative Histograms Definition. The frequency histogram is a graph that displays the classes on the horizontal axis and the frequencies of the classes on the vertical axis; the vertical lines of the bars are erected at the class boundaries and the height of the bars correspond to the class frequency. Relative frequency histogram, on the other hand, is a graph that displays the classes on the horizontal axis and the relative frequencies on the vertical axis. Note: The relative frequency histogram has the same shape as the frequency histogram but has a different vertical axis. Example: Using the Stat 105 Final Grades data, we have the following frequency histogram corresponding to the given frequency distribution in page 6. The frequency histogram contains the same information as the relative frequency histogram in terms of the shape and variability in the data. Both will give us where most of the data lies, whether there are outliers and other peculiar behaviors in the data. 19 STAT 105 (Introduction to Statistical Analysis) The Stem-and-Leaf Display Definition. The stem-and-leaf display (SALD) is an alternative method for describing a set of data. It presents a histogram-like picture of the data, while allowing the experimenter to retain the actual observed values of each data point. Hence, the stem-and-leaf display is partly tabular and partly graphical in nature. Steps in Constructing the Stem-and-Leaf Display: In creating a stem-and-leaf display, we divide each observation into two parts, the stem and the leaf. For example, we could divide the observation 244 as follows: Stem Leaf 2 | 44 Alternatively, we could choose the point of division between the units and tens, whereby Stem Leaf 24 | 4 The choice of the stem and leaf coding depends on the nature of the data set. We now proceed with constructing the whole plot as follows: 1. List the stem values, in order, in a vertical column. 2. Draw a vertical line to the right of the stem value. 3. For each observation, record the leaf portion of that observation in the row corresponding to the appropriate stem. 4. Reorder the leaves from lowest to highest within each stem row. Maintain uniform spacing for the leaves so that the stem with the most number of observations has the longest line. 5. If the number of leaves appearing in each row is too large, divide the stem into two groups, the first corresponding to leaves beginning with digits 0 through 4 and the second corresponding to leaves beginning with digits 5 through 9. this subdivision can be increased to five groups if necessary. 6. Provide a key to your stem-and-leaf coding so that the reader can recreate the actual measurements from your display. Example: Given the CPU time for n = 30 randomly chosen jobs (in seconds) in array below, construct and interpret the stem-and-leaf display. 8 9 27 31 32 33 34 34 37 37 41 42 45 49 50 51 52 52 54 55 56 58 58 59 59 62 76 80 84 101 20 STAT 105 (Introduction to Statistical Analysis) Solution: The corresponding SALD is as follows: Stem Leaf (unit = 1) 0 89 1 2 7 3 1234477 4 1259 5 01224568899 6 2 7 6 8 04 9 10 1 The distribution of CPU time seems to be symmetrical around 50 seconds, i.e. almost half of the tasks were executed in at most 50 seconds. Fastest task to be executed is in 8 seconds while the slowest is 101 seconds. There are gaps, i.e. there are stems with no leaves, which means that some tasks may have been irregularly performed, either faster or slower than usual. Note: The stem-and-leaf display should include a reminder indicating the units of the data value. Examples of different unit representations: Unit = 0.1 1 | 2 represents 1.2 Unit = 1 1 | 2 represents 12 Unit = 10 1 | 2 represents 120 The Boxplot Definition. A boxplot or the box-and-whisker plot is an exploratory data analysis tool that is very useful for displaying the features of the data such as location, spread, symmetry, extremes, and outliers. Remarks: Some key features that can be observed in a boxplot are: Location – as indicated by the median. Spread – as indicated by the distance between the first and third quartiles Skewness – as indicated by the location of the median in the box. Tail length – as indicated by the length of the whiskers. Possible outliers – as indicated by the observations outside the whiskers. Steps in Constructing a Boxplot: 1. Construct a rectangle with one end at the first quartile and the other end at the third quartile. 2. Put a vertical/horizontal line across the interior of the rectangle at the median. 3. Compute for the interquartile range (IQR), lower fence (FL) and upper fence (FU) given by: 21 STAT 105 (Introduction to Statistical Analysis) IQR = Q3 - Q1 FL = Q1 - 1.5 IQR FU = Q3 + 1.5 IQR 4. Locate the smallest value contained in the interval [FL , Q1]. Draw a line from this value to Q1. 5. Locate the largest value contained in the interval [Q3,FU]. Draw a line from this value to Q3. 6. Values falling outside the fences are considered outliers and are usually denoted by “x” or a dot. Example: Using the CPU Time data, we have the following statistics: Md = 50.50 Q1 = 34.00 Q 3 = 58.25 IQR = Q 3 − Q1 = 58.25 − 34 = 24.25 FL = Q1 − 1.5 ∗ IQR = 34 − 1.5 ∗ 24.25 = −2.375 FU = Q 3 + 1.5 ∗ IQR = 58.25 + 1.5 ∗ 24.25 = 94.625 It can be observed that the CPU can finish this type of tasks in 50.50 seconds, on average. One task is a possible outlier with more than 100 seconds to perform. Further investigation should be made for this observation. Remarks: 1. The width of the rectangle is usually arbitrary and has no specific meaning. If several boxplots appear together, however, the height is sometimes made proportional to the different sample sizes. 2. If the outlying observation is less than Q1 - 3 IQR or greater than Q3 + 3 IQR it is identified with a circle at their actual location. Such an observation is called a far outlier. 3. Other variation of the boxplot includes a plus sign (+) at the location of the mean. 22 STAT 105 (Introduction to Statistical Analysis) The Parallel Boxplots Boxplots are often used to compare different populations or parts of the same population. For such a comparison, samples of data are collected from each part, and their boxplots are drawn on the same scale next to each other. Example: Suppose that there are four types of tasks performed and the performance of the new CPU was tested on these tasks. Given below are the efficiencies (in seconds) of the CPU on 20 tasks for each type. Construct and interpret parallel boxplots of the four types. Type 1 Type 2 Type 3 Type 4 58 47 34 38 52 55 91 156 61 36 65 78 65 52 130 146 47 51 81 75 69 65 98 59 41 27 68 62 51 55 134 69 27 69 95 62 53 54 66 130 46 82 94 52 62 60 73 145 75 29 88 62 62 48 107 96 46 64 42 92 51 56 82 112 60 24 97 48 57 55 102 107 49 30 93 89 53 97 91 50 Solution: You can verify the following statistics computed from the given data. Statistics Type 1 Type 2 Type 3 Type 4 Md 47.00 71.50 55.00 100.00 Q1 31.50 54.50 52.25 75.25 Q3 60.75 91.25 62.00 130.00 IQR 29.25 36.75 9.75 54.75 FL -12.38 -0.63 37.63 -6.88 FU 104.63 146.38 76.63 212.13 Constructing the parallel boxplots, we have the following: Using the boxplots above, we can see that the CPU is slowest is performing Type 4 tasks, on average, followed Type 2, Type 3, and Type 1, respectively. Type 4 tasks also have the highest variability as suggested by the length of the box, while Type 3 has the most consistent CPU time. One possible outlier was observed in performing a Type 3 task. 23 STAT 105 (Introduction to Statistical Analysis) Exercises: Using the FIES subset data in page 13 of this material, do the following: 1. Choose one or more variables and compute for the mean, median, mode, 25th percentile, 60th percentile, standard deviation, and coefficient of variation. Post a picture/ table/ screenshot of your work in the designated discussion forum in our LMS and let your classmates interpret these statistics. 2. Using the same variables in (1), construct any graphical statistics that will help you interpret or visualize the distributions of your variables. Try to do your work manually or using jamovi. Post your work in the designated discussion forum and discuss with your classmate what can you see in your work. 3. Construct parallel boxplots of some variables across sexes of the household heads or across types of households. Post your work in the designated discussion forum and discuss with your classmates the interpretation of these boxplots. COPYRIGHT NOTICE This material has been reproduced and communicated to you by or on behalf of University of the Philippines pursuant to PART IV: The Law on Copyright of Republic Act (RA) 8293 or the “Intellectual Property Code of the Philippines”. The University does not authorize you to reproduce or communicate this material. The Material may contain works that are subject to copyright protection under RA 8293. Any reproduction and/or communication of the material by you may be subject to copyright infringement and the copyright owners have the right to take legal action against such infringement. 24