Study Guide for Stats PDF
Document Details
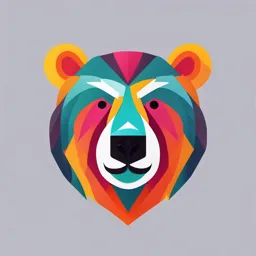
Uploaded by TopCherryTree
Syracuse University
Tags
Summary
This study guide provides an overview of key statistical concepts and techniques. It covers topics such as statistical models, populations and samples, parameters, estimation, and confidence intervals. It also details various statistical methods, including t-tests, ANOVAs, and regression analyses, along with graphing data methods.
Full Transcript
Key Statistical Concepts 1. Statistical Models ○ Definition: Tools used by scientists to test hypotheses. These models predict an outcome variable based on one or more predictor variables. ○ Types: Vary depending on the research question. For example, linear regre...
Key Statistical Concepts 1. Statistical Models ○ Definition: Tools used by scientists to test hypotheses. These models predict an outcome variable based on one or more predictor variables. ○ Types: Vary depending on the research question. For example, linear regression for continuous outcomes or logistic regression for categorical outcomes. 2. Populations and Samples ○ Population: The entire group of interest in a study. ○ Sample: A subset of the population used to draw conclusions. Larger and random samples better represent the population. 3. Parameters ○ Definition: Values that describe characteristics of a population (e.g., population mean or variance). ○ Use: Researchers estimate these using sample data. 4. Estimation ○ Definition: The process of inferring population parameters from sample data. ○ Method: The method of least squares is a common technique, minimizing the difference between observed and predicted values. 5. Standard Error ○ Definition: Reflects how much a sample statistic (like the mean) might differ from the population parameter. ○ Importance: Indicates the reliability of the sample in representing the population. 6. Confidence Intervals ○ Definition: A range of values where the population parameter is likely to fall (e.g., 95% confidence interval). ○ Misconception: It is not an interval where we are 95% confident the parameter lies, but rather where 95% of similar intervals from repeated samples would contain the true parameter. 7. Null Hypothesis Significance Testing (NHST) ○ Null Hypothesis: No effect or difference exists in the population. ○ Alternative Hypothesis: An effect or difference does exist. ○ Key Terms: Alpha Level: Probability of a Type I error (rejecting a true null hypothesis, usually set at 0.05). Beta Level: Probability of a Type II error (failing to reject a false null hypothesis). Statistical Power: Likelihood of correctly rejecting the null hypothesis when false (aim: 0.80 or higher). Statistical Techniques 1. t-Test ○ Purpose: Compares the means of two groups. ○ Types: Independent t-test: For separate groups. Dependent t-test: For repeated measures or paired data. 2. Analysis of Variance (ANOVA) ○ Purpose: Compares means across three or more groups. ○ Extensions: Planned Contrasts: Test specific hypotheses. Post Hoc Tests: Explore all group differences after finding significant results. 3. Analysis of Covariance (ANCOVA) ○ Purpose: Compares group means while controlling for covariates. 4. Factorial Designs ○ Definition: Experiments with two or more independent variables. ○ Key Effects: Main Effects: Impact of one independent variable. Interaction Effects: Combined impact of variables on the outcome. 5. Exploratory Factor Analysis (EFA) ○ Purpose: Identifies clusters of related variables (factors). ○ Applications: Questionnaire design, data reduction. 6. Reliability Analysis ○ Purpose: Measures consistency of a test (e.g., Cronbach’s alpha). 7. Chi-Square Test ○ Purpose: Examines relationships between two categorical variables. 8. Loglinear Analysis ○ Purpose: Explores relationships among more than two categorical variables. 9. Logistic Regression ○ Purpose: Predicts categorical outcomes based on predictors. ○ Types: Binary (two outcomes) and multinomial (multiple outcomes). Graphing Data 1. Common Types of Graphs: ○ Histograms: Show frequency distributions. ○ Boxplots: Highlight data spread, medians, and outliers. ○ Bar Charts: Represent group means, often with error bars. ○ Line Charts: Illustrate trends over time. ○ Scatterplots: Show relationships between two continuous variables, often with a regression line. General Considerations 1. Assumptions ○ Statistical methods often require assumptions (e.g., normality of data). Results may not be valid if assumptions are violated. 2. Effect Sizes ○ Measure the magnitude of an effect, providing insight into practical significance beyond p-values. 3. Meta-Analysis ○ Combines results from multiple studies to estimate an overall effect size. 4. Bayesian Statistics ○ Focuses on updating prior beliefs with new data, an alternative to NHST. 5. Open Science ○ Practices like pre-registering studies and sharing data for transparency and reproducibility. Reporting Results Include details like: ○ Type of analysis. ○ Sample size. ○ Assumption checks. ○ Effect sizes and confidence intervals. ○ Statistical software used. Pearson's Chi-Square Test (χ²) Purpose: Tests whether there is a significant association between two categorical variables (e.g., gender and voting preference). When to Use: When you have counts (frequencies) in categories (e.g., Yes/No, Male/Female). How It Works: Compares observed frequencies (actual data) with expected frequencies (what you'd expect if there were no relationship). Reporting: Include: ○ Test statistic (χ²) ○ Degrees of freedom (df) ○ Sample size (N) ○ p-value (indicates significance). Example: "The analysis revealed a significant association between gender and opinion, χ²(1, N = 250) = 12.50, p <.001." Key Point: If p <.05, the variables are significantly associated. 2. Fisher's Exact Test Purpose: Like the chi-square test but used when sample sizes are very small or expected counts in some cells are low (< 5). When to Use: Small samples or contingency tables with low expected frequencies. Reporting: Fisher's test does not calculate a test statistic (like χ²), so report the p-value directly. Example: "Fisher's exact test revealed a significant association between treatment group and outcome, p =.025." 3. t-Tests Purpose: Compare means between groups to determine if differences are statistically significant. Types: 1. One-Sample t-Test ○ Compares the mean of a single sample to a known population mean. ○ Example: Compare your class's average score to the national average. 2. Independent Samples t-Test ○ Compares means between two independent groups. ○ Example: Compare test scores between males and females. 3. Paired Samples t-Test ○ Compares means of the same group at two different times (e.g., pre- and post-test scores). Reporting: Include: ○ Test statistic (t) ○ Degrees of freedom (df) ○ Sample mean (M) and standard deviation (SD) ○ Confidence interval (CI) ○ p-value ○ Effect size (e.g., Cohen's d for independent/paired samples). Example (Independent Samples): "An independent samples t-test revealed a significant difference in anxiety scores between the control group (M = 10.50, SD = 2.30) and the experimental group (M = 8.20, SD = 1.80), t(48) = -3.25, p =.002, 95% CI [-3.80, -0.80], d = 1.20." 4. ANOVA (Analysis of Variance) Purpose: Tests whether means across more than two groups are significantly different. When to Use: When you have one independent variable with more than two levels (e.g., three diets and their effects on weight loss). How It Works: Compares variance between groups (due to the treatment) and within groups (random error). Reporting: Include: ○ F-statistic (F) ○ Degrees of freedom (df) ○ p-value ○ Effect size (e.g., eta-squared, η²). Example: "A one-way ANOVA revealed a significant effect of treatment on depression scores, F(2, 57) = 5.20, p =.008." Key Points: Post hoc tests (e.g., Tukey's HSD) are needed to determine which groups differ if the result is significant. 5. Regression Analysis Purpose: Examines relationships between variables and predicts an outcome (dependent variable) from one or more predictors (independent variables). When to Use: Predict scores (e.g., predicting GPA based on study hours). How It Works: Fits a line through data points to explain how one variable changes based on another. Reporting: Include: ○ R² (proportion of variance explained by predictors) ○ F-statistic and p-value (test significance of the model) ○ Regression coefficients (b) and their p-values. Example: "The regression model significantly predicted academic performance, R² =.25, F(2, 120) = 15.60, p <.001. Study hours (b = 0.50, p <.001) had a significant positive effect." 6. Correlation Coefficients Purpose: Measure the strength and direction of the relationship between two variables. Types: Pearson's r: Linear relationships between continuous variables. Spearman's ρ: Monotonic relationships (continuous/ordinal data). Kendall's τ: Similar to Spearman's but better for small samples or tied ranks. Reporting: Include: ○ Coefficient value (r, ρ, or τ) ○ Confidence interval ○ p-value. Example: "Creativity was significantly related to competition success, τ = −0.30, 95% CI [−0.47, −0.12], p =.001." 7. Reliability Analysis Purpose: Evaluates the consistency of a measure (e.g., survey items). When to Use: When assessing internal consistency of scales (e.g., using Cronbach’s α). Reporting: Report the α value. Example: "The fear of maths subscale had high reliability, Cronbach’s α = 0.82." Key Statistical Symbols and Their Meanings Symbol Meaning M Mean (average) SD Standard Deviation (spread of scores) SE Standard Error (variability of the mean) df Degrees of Freedom (flexibility in data) p p-value (probability of null hypothesis) χ² Chi-Square Statistic t t-statistic (difference of means) F F-statistic (variance ratio) R² Proportion of variance explained b Regression coefficient (slope) β Standardized regression coefficient r Pearson correlation coefficient η² Effect size in ANOVA α Type I error probability