Simulation Ch2 PDF
Document Details
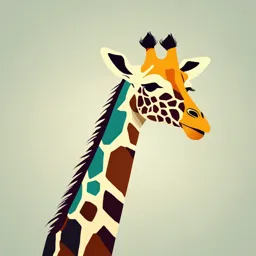
Uploaded by TougherBlackHole
Tags
Summary
This document is a chapter on simulation, probability, and statistics. It covers concepts like discrete and continuous random variables, probability distribution functions, and examples.
Full Transcript
SIMULATION 1 CHAPTER 2 inversal set...
SIMULATION 1 CHAPTER 2 inversal set ↓ (total) subset - S = 6 3 even 6 all 3 common 2 3 2 I I 6 3 - - 11 6 + 1 6 have = if = - we 3 Sets P (A) : All = 6 P(B) : Even : 3 P(C) 93 : : 2 2/6 3/6 - - : (can begreen) total cause :alreadyo a isi um 197 finished finished & finished as ↳ v r &d first d A, B or Comma - 2nd j : ↑ ↳ 11 12 DISCRETE RANDOM VARIABLE 14 WHAT IS DISCRETE DATA? If you have quantitative data, like a number of workers in a company, could you divide every one of the workers into 2 end parts? The answer is absolutely NOT. Because the number 19t B/A before A/B of workers is discrete data. DEFINITION & Discrete data is a count that involves integers. Only a limited number of values is possible. The discrete values cannot be subdivided into parts. 13 DISCRETE RANDOM VARIABLE & Discrete data key characteristics 15 16 EXAMPLES & EXPLANATION 1. You can count the data. It is usually units counted in whole numbers. The number of children in a school is discrete data. You can 2. The values cannot be divided into smaller pieces and count whole individuals. You can’t count 1.5 kids. add additional meaning. So, discrete data can take only certain values. The data 3. You cannot measure the data. By nature, discrete data variables cannot be divided into smaller parts. cannot be measured at all. For example, you can measure your weight with the help of a scale. So, your weight is & How to display graphically discrete data? 1 2 not a discrete data. 4. It has a limited number of possible values e.g. days of We can display discrete data by bar graphs. Stem-and-leaf- the month. plot and pie chart are great for displaying discrete data too. 5. Discrete data is graphically displayed by a bar graph. 3 Y Examples of discrete data: DISCRETE RANDOM VARIABLE 17 18 The number of students in a class. A random variable X and its corresponding distribution are said The number of workers in a company. to be discrete, if X has the following properties. The number of parts damaged during · (1) The number of values for which X has a probability transportation. different from 0, is finite or utmost countable infinite. Shoe sizes. (2) Each finite interval on the real line contains at most Number of languages an individual speaks. finitely many of those values. The number of test questions you answered If an interval a ≤ X ≤ b does not contain such a correctly. value, then P (a < X ≤ b) = 0. Here a and b are the The number of siblings a randomly selected upper and lower limits of the stochastic variable X. individual has Theorems on Expected Value 19 (important) 20 Otherwise the condition, If c is any constant, then I E(cX) = cE(X) o If X and Y are two independent random variables, then sum ↳ (segma) E(X+Y) = E(X) + E(Y) Here f (x) is called the Probability Density Function (PDF) of X and If X and Y are two independent random variables, then determines the distribution of the random variable X. Expected Value and Variance of a Discrete E(XY) = E(X).E(Y) Random Variable Variance The variance is a measure of the relative spread in the values, Expected value the random variable takes on. some of The mean of values of X in the range R Variance of a discrete random variable is: Sum of all the values over the sample space R(x). median Median 3 Measure of Probability Function 1,2 , 4 , 5 , 21 22 Mode of the distribution :Value of x at which Probability Median divides the observations of the variable in two distribution function f (x) is maximum. 1 2 3 4 3 / , , did , , - equal parts. A distribution can have more than one mode. Such a - / distribution is called multimodal. - 3 2 > 1 2 3 Mean – Mode = 3(mean – median). , , , , Discrete distribution Continuous distribution - Some important distribution functions 3 Binomial Distribution Function 23 24 1 Cumulative Distribution function F(x) represents a probability that the random variable X takes on a value less than or equal to x. 4 4thigings Poisson’s Distribution *important ↳ 2 Uniform Distribution function Lambda D - A random variable X which is uniformly distributed in an λ is the expected value. interval [a, b] can be defined as - me should be ③ FB Poisson’s distribution is used in the case where out of large I - number of trials, probability of success of an event is quite low. CONTINUOUS RANDOM VARIABLE CONTINUOUS RANDOM VARIABLE 25 26 As we mentioned above the two types of Example quantitative data (numerical data) are discrete and You can measure your height at very precise scales — continuous data. Continuous data is considered as meters, centimeters, millimeters and etc. You can record continuous data at so many different measurements – width, the opposite of discrete data. temperature, time, and etc. This is where the key difference Definition: with discrete data lies. anything you measured Continuous data is information that could be How to display graphically continuous data? meaningfully divided into finer levels. It can be We can display continuous data by histograms. Line graphs measured on a scale or continuum and can have are also very helpful for displaying trends in continuous almost any numeric value. data. Chapter 2: Parts of a Program Continuous data key characteristics Examples of continuous data: 27 28 In general, continuous variables are not counted. The amount of time required to complete a project. The values can be subdivided into smaller and smaller pieces and they have additional meaning. The height of children. The continuous data is measurable. The amount of time it takes to sell shoes. It has an infinite number of possible values within an interval. The amount of rain, in inches, that falls in a storm. Continuous data is graphically displayed by The weight of a truck. histograms. In comparison to discrete data, continuous data give a The speed of cars. much better sense of the variation that is present. Time to wake up. EXPONENTIAL DISTRIBUTION CONTINUOUS RANDOM VARIABLE 29 30 (1 , 8 , 1. - 2 A random variable X is exponentially distributed with If a random variable X may assume any value in parameter λ > 0 if its probability distribution function some given interval (the interval may be bounded or is unbounded), it is called a continuous random variable. ↳ men If it can assume only a number of separated values, it is called a discrete random variable. Exponential density function Exponentiel distribution function X=1,2,3,4,5,6 :discrete random variable X random choice of a real number from interval [1, 6] :Continuous random variable * Error Probality CIRCULAR PROBABLE ERROR (CEP) AND THE PROBABLE ERROR (PE) Range and Deflection Probable Errors 31 s 32 & radius If all the hits are projected onto a straight line in place D Parameter CEP is the radius of the circle about the true mean of a point, the interval about both sides of the line point with respect to aim point; which includes 50% of the which includes 50% of the shots is called the hits, considering a very large number of rounds fired onto the target ⑫ Range Error Probable (REP). The REP is a one dimensional or univariate measure & of dispersion and is used commonly for range* generally used to describe weapon delivery accuracy in the precision in firing. Circular Error Probable (CEP). REP =0.6745 σ D & This relation is true only for circular distribution Deflection Error Probable (DEP) 33 ③ Deflection probable error is the error in a direction transverse to range and is called Deflection Error Probable (DEP). The value of DEP is also equal to. DEP= 0.6745 σ same as REP ↑ E A N