Experimental Research Designs PDF
Document Details
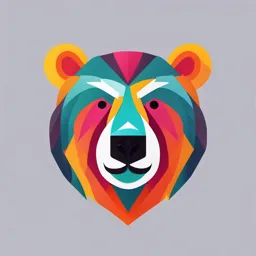
Uploaded by ChasteMannerism
null
Edwards
Tags
Summary
This document provides an overview of experimental research designs, including random sampling and assignment, control groups, and different types of designs. The document also examines the strengths and weaknesses of specific design types and discusses the role of pre-tests and post-tests, and interactions in experimental research. It highlights the importance of controlling for extraneous variables.
Full Transcript
EDWARDS—RESEARCH METHODS LECTURE NOTES PAGE 20 Topic #4 EXPERIMENTAL RESEARCH DESIGNS As a strict technical definition, an experiment is a study or research design in which we MANIPULA...
EDWARDS—RESEARCH METHODS LECTURE NOTES PAGE 20 Topic #4 EXPERIMENTAL RESEARCH DESIGNS As a strict technical definition, an experiment is a study or research design in which we MANIPULATE variables. This also implies that experimental designs are characterized by RANDOM ASSIGNMENT to groups, treatments, etc. That is, the researcher has high levels of control over the WHO, WHAT, WHEN, WHERE and HOW of the study. MANIPULATION and RANDOM ASSIGNMENT are the defining characteristics of experimental designs. RANDOM SAMPLING AND RANDOM ASSIGNMENT Experimental designs call for random sampling (all research designs do) and at the very least random assignment to groups. The use of random sampling and random assignment gives the strongest case for causal inferences. 1. Random Sampling—the process of choosing a "representative" group from an entire population such that every member of the population has an equal and independent chance of being selected into the sample. Achievement of random sampling is rare in applied research. 2. Random Assignment (Randomization)—a control technique that equates groups of participants by ensuring every member (of the sample) an equal chance of being assigned to any group. Randomization is of concern in experimental research where there is some manipulation or treatment imposed. As a systematic procedure for avoiding bias in assignment to conditions or groups, if we can avoid the said bias, then we can assert that any differences between groups (conditions) prior to the introduction of the IV are due solely to chance. The principal concern is whether differences between groups AFTER the introduction of the IV are due solely to chance fluctuations or to the effect of the IV plus chance fluctuations. EDWARDS—RESEARCH METHODS LECTURE NOTES PAGE 21 EXAMPLES OF SOME EXPERIMENTAL RESEARCH DESIGNS 1. Control experiment with control group and experimental group PRETEST TREATMENT POSTTEST GROUP I YES YES YES GROUP II YES NO YES 2. Control experiment with no control group PRETEST TREATMENT POSTTEST GROUP I YES A1 YES GROUP II YES A2 YES 3. Control experiment with control condition within–subjects ALL PARTICIPANTS PRETEST TREATMENT POSTTEST CONDITION I YES YES YES CONDITION II YES NO YES EDWARDS—RESEARCH METHODS LECTURE NOTES PAGE 22 4. Solomon Four–Group design Generally accepted as the best between-subjects design, but requires a large number of participants. PRETEST TREATMENT POSTTEST GROUP I YES YES YES GROUP II NO YES YES GROUP III YES NO YES GROUP IV NO NO YES Some Possible Comparisons A. Effect of treatment (I and II) vs. No treatment (III and IV) B. Effect of pretest (I and III) vs. No pretest (II and IV) C. Effect of pretest on treatment (I vs. II) Why pretest? 1. equivalence of groups 2. baseline 3. effects of testing or practice effects What does one do if there are pretest/baseline differences? 1. Difference scores 2. Analysis of covariance (ANCOVA) 3. Partial/semi–partial correlations EDWARDS—RESEARCH METHODS LECTURE NOTES PAGE 23 EXAMPLES OF SOME RESEARCH DESIGNS TO AVOID 1. One–group posttest only design TREATMENT POSTTEST GROUP I YES YES 2. One–group pretest–posttest design PRETEST TREATMENT POSTTEST GROUP I YES YES YES 3. Posttest only design with nonequivalent control groups ALLOCATION TO TREATMENT POSTTEST GROUPS GROUP I NONEQUIVALENT YES [A1] YES NATURALLY GROUP II OCCURRING GROUPS NO [A2] YES Nonequivalent control group of participants that is not randomly selected from the same population as the experimental group EDWARDS—RESEARCH METHODS LECTURE NOTES PAGE 24 WITHIN AND BETWEENSUBJECTS DESIGNS 1. Withinsubjects design—a research design in which each participant experiences every condition of the experiment. A. Advantages 1. do not need as many participants 2. equivalence is certain B. Disadvantages 1. effects of repeated testing 2. dependability of treatment effects 3. irreversibility of treatment 2. Betweensubjects design—a research design in which each participant experiences only one of the conditions in the experiment. A. Advantages 1. effects of testing are minimized B. Disadvantages 1. equivalency is less assured 2. greater number of participants needed EDWARDS—RESEARCH METHODS LECTURE NOTES PAGE 25 SUMMARY OF KEY CONCEPTSEXPERIMENTAL DESIGNS 1. Control Any means used to rule out possible threats to a piece of research Techniques used to eliminate or hold constant the effects of extraneous variables 2. Control Group Participants in a control condition Participants not exposed to the experimental manipulation 3. Experimental Group Participants in an experimental condition 4. Control Condition A condition used to determine the value of the dependent variable without the experimental manipulation. Data from the control condition provide a baseline or standard to compare behavior under changing levels of the independent variable. 5. Experimental Condition Treatment condition in which participants are exposed to a non–zero value of the independent variable; a set of antecedent conditions created by the experimenter to test the impact of various levels of the independent variable. 6. Withinsubjects Design Research design in which each participant serves in each treatment condition. 7. Betweensubjects Design Research design in which different participants take part in each condition of the experiment. EDWARDS—RESEARCH METHODS LECTURE NOTES PAGE 26 CAUSAL INFERENCES An advantage that experimental designs have over other research designs is that they permit us to make causal inferences. Causation implies the ability to make statements about the absence or presence of cause–effect relationships. While there are several methods—some of which are discussed in the text—to experimentally identify causality, there are three conditions that must be met to infer cause. A. Contiguity—between the presumed cause and effect. B. Temporal precedence—the cause has to precede the effect in time. C. Constant conjunction—the cause has to be present whenever the effect is obtained. The ability to make causal inferences is dependent on how well or the extent to which alternative causes or explanations are ruled out. Cause—is a necessary and sufficient condition. An event that only causes an effect sometimes is NOT a cause. The assessment of causation technically demands the use of manipulation. Caveats to determining causality A. Concerning cause–effect relationships, it cannot be said that they are true. We can only say that they have NOT been falsified. B. The use of correlational methods to infer casual relationships should be avoided. C. Although one might find that r =/ 0 or that the regression equation is significant, this does NOT prove a causal relationship (i.e., that X caused Y to change). At best we can only say that there is a linear relationship between X and Y. EDWARDS—RESEARCH METHODS LECTURE NOTES PAGE 27 ANALYSIS OF VARIANCE (ANOVA), FACTORIAL DESIGNS, MAIN EFFECTS, AND INTERACTIONS 1. Analysis of Variance (ANOVA)—is a statistical procedure used to compare two or more means simultaneously; it is used to study the joint effect of two or more IVs. The ANOVA is based on the F–statistic and can be thought of as an extension of the t– test. t2=F t test = 2 means ANOVA = 2 or more means One–factor or simple ANOVA—employs only one IV. We might use two or more levels of the variable but there is only ONE IV. EXAMPLE OF (SIMPLE) ANOVA SUMMARY TABLE SOURCE df SS MS F Factor A a–1 SSa SSa/dfa MSa/MSe Error dftot–dfa SSe SSe/dfe Total N–1 where a = number of levels The assumption is that: individual's score = base level + treatment effect + effects of error where treatment effect = Factor A or IV The above design is also referred to or described as a one–factor (experimental) design, primarily because only one IV is manipulated. "Factor" is merely another term for "IV". EDWARDS—RESEARCH METHODS LECTURE NOTES PAGE 28 2. Factorial Design—a design in which 2 or more variables, or factors are employed in such a way that all of the possible combinations of selected values of each variable are used. Examples of Factorial Designs 2x2 factorial design 2 levels IVA 2 levels IVB 2x2x2 factorial design 2 levels IVA 2 levels IVB 2 levels IVC 2x3 factorial design 2 levels IVA 3 levels IVB An issue that arises when we use factorial designs is that of main effects and interactions. 3. Main Effect—the effect of one IV averaged over all levels of the other IV That is, the effect of IVA independent of IVB or holding IVB constant; can also be described as the mean of A1 and A2 across levels of B A main effect is really no different from a t–test for differences between means. 4. Interaction—when the effect of one IV depends upon the level of the other IV. Two or more variables are said to interact when they act upon each other. Thus, an interaction of IVs is their joint effect upon the DV, which cannot be predicted simply by knowing the main effect of each IV separately. Main effects are qualified by interactions (interpreted within the context of interactions). Thus, the occurrence of an interaction is analyzed by comparing differences among cell means rather than among main effect means. Graphical plots are used merely to illustrate the results of the ANOVA test. We plot graphs to aid us in interpreting the ANOVA results after we have run the test. EDWARDS—RESEARCH METHODS LECTURE NOTES PAGE 29 Number of job leads by social media and whether or not your resume is posted. A experimental design investigating this issue would be a basic 2x2 factorial design. IVA = 2 types of social media (Facebook and LinkedIn) IVB = 2 levels of resume posted (yes and no) Which conditions would lead to the most job leads? What will the data look like? Main effects or an interaction? Plot the data. What would an interaction look like? How would you interpret a resume/social media interaction? On the next 2 pages are examples of data plots that illustrate main effects and interactions of variables (a) and (b). Can you look at a data plot (AKA Figure) and interpret the main effects and interactions? EDWARDS—RESEARCH METHODS LECTURE NOTES PAGE 30 50 50 40 40 B1 B2 30 30 B2 B1 20 20 10 10 0 0 A1 A2 A3 A1 A2 A3 A main effect significant (a) B main effect significant (b) 60 60 B2 B1 50 50 40 40 B2 B1 30 30 20 20 10 10 0 0 A1 A2 A3 A1 A2 A3 Interaction effect significant (c) A and B main effect significant (d) 50 50 B2 40 40 B2 30 30 B1 20 20 B2 10 10 B1 0 0 A1 A2 A3 A1 A2 A3 A main effect and interaction significant (e) B main effect and interaction significant (f) EDWARDS—RESEARCH METHODS LECTURE NOTES PAGE 31 70 B1 60 50 40 B2 B1 30 B2 20 10 0 A1 A2 A3 A and B main effect and interaction significant (g) EDWARDS—RESEARCH METHODS LECTURE NOTES PAGE 32 RESEARCH SETTING Behavior research usually takes place in one of two settings—lab or field. A. The major distinction between lab and field has to do with the "naturalness" or "artificiality" of the setting. Field research typically employs a real–life setting (e.g., in a company) B. Another important concept associated with naturalness and artificiality is that of control. Lab settings tend to permit higher degrees of control than field settings 1. Lab Experiment A. Advantages: 1. Is the best method for inferring causality. Permits the elimination of, or control for other explanations of observed behavior. 2. Measurement of behavior is very precise. 3. Experiments can be replicated by other researchers because experimental conditions are measured and recorded. B. Disadvantages: 1. There is a lack of realism—i.e., the degree of similarity between experimental conditions and the natural environment is limited. 2. Some phenomena cannot be studied in the lab. 3. Some variables may have a weaker impact in the lab than they do in the natural environment. EDWARDS—RESEARCH METHODS LECTURE NOTES PAGE 33 2. Field Experiment A. Advantages: 1. Very realistic 2. Results are highly generalizable 3. Suggestions of causal inference are possible 4. Broader research issues dealing with complex behavior in real–life contexts can be addressed. B. Disadvantages: 1. Variables are less precisely measured 2. Individuals or groups may refuse to participate 3. Often cannot gain access to "natural" (business, organization, home or other) environment.