Quantitative Data Collection PDF
Document Details
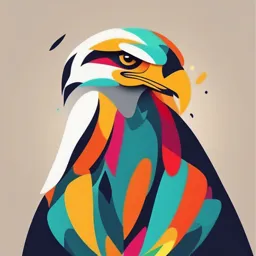
Uploaded by ConciliatoryTangent
University of Limpopo
Mr MI Riba
Tags
Related
- Article_Data Collection Strategies Quantitative Research.pdf
- Characteristics, Strengths, & Weaknesses of Quantitative Research PDF
- Quantitative Research Methods PDF
- Practical Research 2: Fundamentals of Quantitative Research PDF
- Practical Research 2: Kinds of Quantitative Research PDF
- STA 321.1 Unit 5. Quantitative Research Method PDF
Summary
This document is a lecture on quantitative data collection techniques, specifically for the Theory of Communication Research Unit 3 Part 2 at the University of Limpopo. It discusses various methods including surveys, questionnaires, and different types of questions within surveys. Additionally, it covers experimental designs and content analysis.
Full Transcript
Quantitative Data Collection Theory of Communication Research (HCOC032) Unit 3: Part 2 Lecturer: Mr MI Riba University of Limpopo 1 Learning Outcomes Understand the nature of quantitative research. Differentiate the different...
Quantitative Data Collection Theory of Communication Research (HCOC032) Unit 3: Part 2 Lecturer: Mr MI Riba University of Limpopo 1 Learning Outcomes Understand the nature of quantitative research. Differentiate the different forms of quantitative research designs. Understand the usefulness of survey and its limitation. Identify the different types of measurement scales for quantitative research Collect quantitative data Understand the basic statistics used in research analysis. Know how to test hypothesis. Understand how to organise and analysis data set using statistical measurements. Analyse quantitative data. University of Limpopo 2 Textbook Chapter Outline University of Limpopo 3 Questionnaire Surveys: Types of Questions University of Limpopo 4 Questionnaire Surveys: Types of Questions 2. Indirect 3. General 4. Specific 1. Direct questions questions questions questions demand a are asked when are applicable to are similar to response in a we want to be a whole direct questions frank and often more polite and situation. and focus on a confrontational less Example: How particular aspect manner. confrontational. do you feel of a whole Example: Do Example: Do about situation. you plagiarise? you ever plagiarising Example: Do consider you think plagiarising plagiarism is unethical? University of Limpopo 5 Surveys: Types of Questions in a Questionnaire (contd) 7. Paired- 6. Open-ended 8. Contingency 5. Closed-ended comparison questions questions questions questions contain a invite respondents the respondent instruct the fixed number of to answer in their must choose respondent to answers, from own words, in any between two answer another set which the way they wish. The options. of questions, but respondent must question can elicit only if the select one. underlying ideas, respondent gave a feelings, etc that particular response the researcher to a previous might not have answer. considered. University of Limpopo 6 Surveys: Types of Questions in a Questionnaire (contd) 12. Multiple-choice 9. Ranking questions 10. Inventory questions 11. Matrix questions questions ask the respondent to ask respondents to list are scaled questions. certain units are rank several options. options that apply to The respondents are grouped together. them personally. asked to select the They are usually Inventory questions option that best mutually exclusive are not mutually reflects their attitude, (only one answer is exclusive. Thus, more opinion, etc. The same applicable to the than one option can scale is repeated for respondent). The be chosen, and a each question (eg respondent then category called ‘other’ Likert or semantic chooses the category is usually included if differential scale). applicable to him or the respondent wants her. to add to the list. University of Limpopo 7 Problems associated with the wording of questions Double-barrelled questions Questionable assumptions or presumptive questions Questions making use of loaded language Leading questions Negative items Incomplete questions Vague questions Lengthy questions Ambiguous language Abbreviations and acronyms Complex questions (or jargon) University of Limpopo 8 The use of scales in a questionnaire Measurement scales, such as the Likert scale and the semantic differential scale, often form part of questionnaires, but they are also used on their own. It is important to understand the general properties of scales and the types of scales which may be applied under various circumstances. University of Limpopo 9 Measurement Scales In quantitative communication research, these scales are used to: Measure Variables: Assign numerical values to variables, facilitating the quantification of concepts like attitudes, opinions, and preferences. Ensure Precision: Provide a standardized method for measurement, ensuring data collection is precise and consistent. Enable Statistical Analysis: Generate numerical data that can be subjected to statistical analysis, allowing researchers to identify patterns, test hypotheses, and draw conclusions University of Limpopo 10 The use of scales in a questionnaire The measurement scale or level of measurement you decide to use has certain implications for the analysis of data. Choosing the level and scale of measurement are important parts of the research design process because they are necessary for systematized measuring and categorizing of data, and thus for analyzing it and drawing conclusions from it as well that are considered valid. University of Limpopo 11 The use of scales in a questionnaire Certain quantitative analysis techniques require minimum levels of measurement. Levels of measurement, also called scales of measurement, tell you how precisely variables are recorded. In scientific research, a variable is anything that can take on different values across your data set (e.g., height or test scores). In a measurement scale, numbers should be assigned according to rules, which should correspond to the properties of what is being measured. Rules may be very simple or more complex. University of Limpopo 12 University of Limpopo 13 University of Limpopo 14 The Nominal Scale University of Limpopo 15 The Nominal Scale The nominal scale is a type of measurement scale that assigns numbers or labels to objects or events solely for identification purposes. It does not imply any order or numerical relationship between the values. University of Limpopo 16 The nominal scale The key characteristics of the nominal scale are:Uniqueness: Each value or label is unique and represents a distinct category or group. No inherent order: The values have no specific order or ranking. They are simply used to identify and differentiate between different categories. No numerical meaning: The numbers or labels assigned to the categories do not have any numerical meaning or implication. They are merely used as names or identifiers. Equality and inequality: With the nominal scale, you can only determine if two values are equal (belong to the same category) or not equal (belong to different categories). No other mathematical operations are meaningful. University of Limpopo 17 Example: Nominal Scale Consider, for example, when you need to catch a flight to another city. Each flight is given a number to help passengers understand which route it is travelling on. Here the only property is identity, and any other numerical comparison would be meaningless. The number essentially acts as a name for the flight. Flight A = 1 Flight B = 2 Flight C = 3 In this case, the flight numbers serve as unique identifiers for each route. The numbers 1, 2, and 3 do not represent any numerical relationship between the flights. They are simply used to distinguish between the different routes. University of Limpopo 18 Example: Nominal Scale Some other examples of nominal scales: Gender: Male (1), Female (2), Non-binary (3) Blood type: A, B, AB, O Marital status: Single, Married, Divorced, Widowed Zip codes or postal codes In summary, the nominal scale is a fundamental measurement scale used for identification and classification purposes, where the assigned values have no inherent order or numerical meaning. University of Limpopo 19 The nominal scale Sometimes we use numbers to name or label variables, as in the flight number example previously mentioned. If, for example, you want to distinguish between smokers and non- smokers, you can label smokers (1) and non-smokers (2). You could also label non-smokers (1) and smokers (2), or even (a) and (b). The number is merely a numeral, meaning that it is only used as a symbol and it has no mathematical significance in this context. We therefore cannot use these numbers in any calculations. University of Limpopo 20 The Nominal Scale: Criteria for labelling and assigning variables The categories used must be exhaustive. All the elements of your population or sample must fall into one of the categories and that none of the elements must be excluded. If you have two categories, namely smokers and non-smokers, all the people in your population will fall in either one or the other category because people either smoke or they do not. University of Limpopo 21 The Nominal Scale: Criteria for labelling and assigning variables Categories must be mutually exclusive. What this means is that if an element of your population or sample belongs to one category, it must not be able to belong to any other category. Thus, you either smoke or you do not. Even if you are just a so-called ‘social smoker’, you still smoke and will therefore fall in the ‘smokers’ category. When we label variables or categories on a nominal level of measurement, we use a different number for each variable or category, but the number we assign to each category is of no mathematical significance. There is therefore no order or space implied by the number. University of Limpopo 22 The Nominal Scale Examples of nominally scaled variables include geographic location, gender, income and age. The only arithmetic operation that can be performed in such a scale is a count of each category. In other words, we can only count the number of respondents from Gauteng, or the number of taxis on a given route on a certain day, for example, and express it as a percentage. University of Limpopo 23 The Ordinal Scale University of Limpopo 24 The Ordinal Scale University of Limpopo 25 The Ordinal Scale University of Limpopo 26 The Ordinal Scale An ordinal scale is created through ranking objects or arranging them in order with regard to a common variable. The only question that the use of this scale answers is whether each object has more or less of a particular variable than some other object. The scale does not indicate how much of a difference exists between the objects being observed. University of Limpopo 27 The Ordinal Scale A good example of this scale in application would be the results of a race in which the runners’ times were not recorded — you would be able to discern first place from second and third; however, you would not be able to tell the degree to which the runner in first place beat those in second and third place. Similarly, brands of chocolate could be ranked from most preferred to least preferred. This scale would express which brand rated most highly, but not the differing degrees of preference which exist between the various brands. University of Limpopo 28 The Ordinal Scale An ordinal scale is a type of measurement scale that allows researchers to rank order items based on a specific characteristic, but it does not provide information about the magnitude of differences between the ranks. In communication research, ordinal scales are often used to assess attitudes, perceptions, or preferences University of Limpopo 29 Ordinal Scale Questions: Examples University of Limpopo 30 Example: Measuring Audience Engagement (Ordinal Scale) Research Objective To evaluate the level of audience engagement during a series of public speaking events. Measurement Tool A survey is distributed to attendees after each event, asking them to rate their level of engagement on a scale from 1 to 5, where: 1 = Not engaged at all 2 = Slightly engaged 3 = Moderately engaged 4 = Very engaged 5 = Extremely engaged Data Collection Attendees fill out the survey immediately following the event. The responses are collected and analyzed to determine the overall engagement levels across different events. University of Limpopo 31 University of Limpopo 32 Interval Scale University of Limpopo 33 Interval scale The interval scale is very similar to the ordinal scale, but the intervals between the numerals are of equal distance, and the numbers can therefore be used in mathematical or statistical calculations. In an interval scale the numbers used to rank the elements are equal increments of the attribute being measured. This means that differences can be compared. For example, the difference between 1 and 2 is the same as the difference between 2 and 3, but is only half the difference between 2 and 4 University of Limpopo 34 University of Limpopo 35 Interval scale When using this scale, it is important to remember that the number zero is actually a value. The scale therefore does not include what is known as an ‘absolute zero’. The measurement of temperature is a good example. When a thermometer reads 0 °C, it does not mean that there is no temperature, just that it is really cold. University of Limpopo 36 Interval Scale In quantitative communication research, an example of an interval scale can be seen in the use of a Likert scale in surveys. The Likert scale often measures attitudes or opinions by asking respondents to rate their agreement with a statement on a numerical scale, typically ranging from 1 to 5 or 1 to 7. Other examples of interval scales in communication research include the Net Promoter Score (NPS), where respondents rate their likelihood of recommending a service on a scale from 0 to 10. University of Limpopo 37 Likert Scale University of Limpopo 38 Ratio Scales Ratio scale is an interval scale which has been taken one step further, in that this scale includes an absolute or true zero. In a ratio scale, zero means that the element or variable is absent. Let’s say you want to measure how many friends you have that believe in aliens or extra-terrestrials. If you have none, then we use zero to show that the element (friends who believe in aliens) is absent. With a ratio scale it is possible to say how many times larger or smaller one object is in relation to another, in terms of variables such as weight, age and money. You can measure how much older one person is than another. University of Limpopo 39 University of Limpopo 40 University of Limpopo 41 University of Limpopo 42 The Likert Scale The Likert scale, named after Renis Likert (1903–1981), is one of the most widely used approaches to scaling responses in survey research. Requires respondents to indicate their degree of agreement or disagreement with a variety of statements related to an attitude or object. Likert scales are also known as summated scales, as the responses to individual items are added to create a total score for the respondent that usually indicates how positive or negative the respondent feels towards a particular issue. The scale is most often composed of two parts, namely the item (which is likely to be a statement) and the evaluation (whether the respondent agrees or disagrees with the statement, and to what extent). The evaluation part usually consists of a five-or seven-point scale. University of Limpopo 43 Example: Five-point Likert Scale University of Limpopo 44 The semantic differential scale The semantic differential scale is also a five-or seven-point rating scale. It uses adjectives that are polar opposites, such as ‘hot’ and ‘cold’. In a similar way to the Likert scale, the scoring of the evaluation changes depending on where the adjective is placed on the scale. University of Limpopo 45 The Semantic Differential Scale The semantic differential scale measures the meaning and connotations we ascribe to verbal and non-verbal symbols. The scoring is also reversed for positive and negative items University of Limpopo 46 Advantages & Disadvantages of Surveys Advantages Disadvantages You can collect a lot of data from an individual The researcher needs to have an extensive respondent at any time. understanding of the nature of the errors that may occur during the process of collecting and interpreting surveys. Versatile and can be conducted in almost any setting They tend to be artificial and, potentially, superficial. Experimental Relatively inexpensive Less time-consuming The questionnaire can be filled in anonymously, which tends to encourage candid responses to sensitive issues A large amount of data can be collected, and the data can be standardised University of Limpopo 47 Experimental Designs University of Limpopo 48 Experimental Designs The basic intent of an experiment is to test the effect of an intervention on an outcome. In the process, the researcher also tries to control other factors which might influence the result. In experimental research (also known as true experiments or randomised experiments), researchers randomly assign individuals to experimental and control groups, as one form of control. University of Limpopo 49 Experimental Designs The experimental group is subjected to an intervention, and the control group is not. The researcher then looks for differences between the two groups. It is assumed that any differences observed in the two groups after the experiment will be the result of the intervention — also known as the treatment effect. University of Limpopo 50 University of Limpopo 51 Experimental Designs Experiments, then, usually involve manipulating a certain condition or set of conditions. This condition or set of conditions is referred to as the independent variable. Once the experiment has been conducted, the researcher measures the effect that the change to the independent variable (for example, class activities) has had on certain outcomes, called the dependent variables (for example, academic results). It is assumed that the manipulation of the independent variable caused the change in the dependent variable University of Limpopo 52 Elements of experimental designs University of Limpopo 53 Elements of an Experimental Design 1. The experimental group (EG) indicates the respondents or test units that will be exposed to the intervention or independent variable (class activities). Multiple EGs may exist in one experiment and should be indicated as per the same method outlines for Os (observations) and Xs (interventions/ independent variables). 2. The control group (CG) indicates a control group of respondents or test units that will not be exposed to the intervention or independent variable (X). Multiple CGs may also exist and should be indicated as per the same method outlines for Os (observations) and Xs (interventions/independent variables). 3. Random assignment (R) indicates that all respondents or test units were randomly assigned to either the experimental group (EG) or the control group (CG). Randomisation is useful because it contributes to experimental validity thanks to increased control over extraneous or intervening variables, as was explained earlier. University of Limpopo 54 Elements of an Experimental Design 4. The independent variable indicates the intervention that the researcher introduced (eg class activities). Essentially, experiments examine the effect. of an independent variable (eg class activities) on a dependent one (academic performance). The independent variable takes the form of an intervention, which is either present (EG) or absent (CG). In such an experiment the researcher then compares what happens if the intervention is present to what happens when it is not present. 5. Pre-testing and post-testing: Respondents, or test units, are measured in terms of a dependent variable in the pre-test (observation 1, or O1), exposed to an intervention representing an independent variable (X1), and then measured ). University of Limpopo 55 Quasi-experimental and true experimental designs Experimental designs are often used to test causal or correlational hypotheses. For example, we want to know if an intervention, such as more activities in class, causes better academic results. Determining the cause of something is often difficult, so several researchers opt for correlational studies instead. In these types of studies you can indicate, for example, if an increase in class activities correlates with better academic results. University of Limpopo 56 Quasi-experimental designs The word quasi comes from Latin and means ‘as if’, or pseudo, which means ‘false’. Quasi therefore refers to something that is almost the same as something else but missing some of its features. In the case of experiments, quasi-experimental designs are similar to true experimental designs, but they lack certain important qualities. They are experiments where the respondents are not randomly assigned to the experimental and control groups. Instead, the similarity of the two groups is based on other variables which can be predetermined. University of Limpopo 57 Quasi-experimental designs These designs are also referred to as correlational research designs. Three popular quasi-experimental designs are propensity score matching (PSM), regression discontinuity (RD) and difference in differences (DID). The biggest advantage of correlational designs is that they are relatively easy to conduct. The disadvantage is that, although they describe the correlation, they are usually silent on the cause of the correlation. University of Limpopo 58 True experimental designs True experimental design is a statistical approach to establishing a cause-and-effect relationship between variables123. To be classed as a true experimental design, an experiment must have the following features: Random assignment of participants into experimental and control groups. A “treatment” (or intervention) provided to the experimental group. A viable control group. Only one variable can be manipulated and tested. University of Limpopo 59 True experimental designs You can have more confidence in the results obtained from a true experimental design than in those obtained from a quasi- experimental design. The reason for this is the degree of control that is incorporated in its implementation. In true experimental designs the variables are carefully isolated from others, so that you can be sure that it is indeed the independent variable that you are testing which is causing the effect, and nothing else. These designs therefore have very high internal validity. University of Limpopo 60 Different factors that can have an influence on internal validity History Maturation Testing Instrumentation Statistical Regression Selection bias Selection maturation Experimental Mortality University of Limpopo 61 Advantages & Disadvantages of Experimental Designs Advantages Disadvantages The experimenter can attempt to eliminate, or control It is not always possible to eliminate extraneous for, all unwanted, extraneous variables. variables Since the experimental design involves the Can lead to a distortion of the results manipulation of the independent variable and the observation of its effect upon the dependent variable, it is possible to determine causal relationships. Since experiments are conducted in strictly controlled Not all experiments can be directly related back to the environments, they can be repeated again and again, real world; experimental research’s greatest weakness and the results can be compared. therefore lies in its artificiality. People do not live in laboratories under controlled circumstances. It may also be unethical to assign people to groups on a random basis. University of Limpopo 62 Content Analysis Content analysis is a data collection technique as well as a data analysis technique. It helps us to understand information as symbolic phenomena. It is used to investigate symbolic content such as words that appear in, for example, newspaper articles, comments on a blog, political speeches, and so on. Content analysis, then, is a quantitative technique in which the researcher attempts to describe the denotative meaning of content in an objective way. There are two levels of meaning, namely denotative and connotative meaning. University of Limpopo 63 Content Analysis The denotative meaning of a word refers to the literal meaning you will find in the dictionary when you look up that word. This meaning is free from any form of interpretation. The connotative meaning of a word refers to the connotation we ascribe to a particular word, based on the feeling or idea the word evokes in us, which is often based on our experiences. In content analysis, we only work with the denotative meaning of words to make valid and reliable assumptions of data within context. University of Limpopo 64 Content Analysis When conducting a quantitative content analysis, you can only work with what is reported and you are not allowed to make any assumptions about the author’s intended meaning. The researcher can make certain observations about a communicator, such as a politician, without influencing the communicator. Because of the symbolic nature of words, we have to be context specific in our analysis, since the meaning of words changes depending on the context. University of Limpopo 65 Content Analysis We can also only make context-specific assumptions when we do a content analysis. The researcher has no control over the situation in which a response is given, and the sender of the message is also unaware that the message will be analysed. The researcher does not run the risk of collecting information that then becomes invalid because the data was not correctly gathered. With content analysis, data does not have to be gathered in a structured way. University of Limpopo 66 Content Analysis Without the establishment of reliability, content analysis measures are useless’. Inter-coder reliability not only makes coding more efficient but it also helps to dismiss sceptical reviewers and critics. You must report inter-coder reliability carefully, clearly and in a detailed manner in your research report. Intercoder reliability is a measure of agreement between different coders on how to code the same data. It is used in content analysis when accuracy and consistency are key research objectives. Intercoder reliability ensures that multiple researchers arrive at the same findings when coding the same content. University of Limpopo 67 Quantification of the data in content analysis Since content analysis is considered a quantitative technique, you must be able to express the results numerically. Quantification is a prerequisite for those who see content analysis as a scientific method. Some researchers feel that there is no purpose in content analysis unless the results are measurable; in other words, unless it can be quantified. Proponents of quantitative research are also of the opinion that quantitative methods describe the quality of communication better. University of Limpopo 68 Quantification of the data in content analysis Researchers who employ quantitative research use frequency to determine, for example, how often certain concepts and themes are used. Use of statistical methods University of Limpopo 69 A research design for content analysis It needs to be appropriate to the context The design is also used to answer a research question and to test hypotheses related to the research question. Data does not have to be gathered in a structured way. A major advantage of content analysis is that it allows you to collect and analyse large amounts of data because you can use more than one researcher to analyse the data, as long as you can establish high inter- coder reliability. Inter-coder reliability is imperative in quantitative content analysis because we are supposed to work with only the exact denotative meanings of words. University of Limpopo 70 Criteria for evaluating categories in content analysis All categories must fulfil the following criteria: l Mutually exclusive Exhaustive Equivalent University of Limpopo 71 Steps in content analysis 1. Read through the text several times and make brief notes in the margin when you come across interesting or relevant information. 2. Go through the notes made in the margins and list the different types of themes that emerge — what are the things that reoccur in speeches/newspapers/texts? 3. Read through the list and categorise each item in a way that describes what it is about. These are your operational definitions. 4. Use your operational definitions to code your data and place the information in a particular category. 5. Once you have all your categories, identify whether the categories can be linked in any way or grouped together. 6. If you are working with more than one transcript (in this case speeches), repeat the first few steps again for each transcript. University of Limpopo 72 Steps in content analysis 1. Collect all the categories. Examine each category in detail and consider its relevance. 2. Verify and check if you have collected all the relevant data from the transcripts. Verify the codes 3. Apply an appropriate statistical test to analyse your data. University of Limpopo 73 End University of Limpopo 74