Statistics: Estimation Procedures (Chapter 6)
Document Details
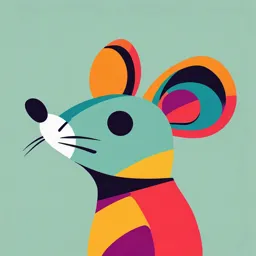
Uploaded by ConscientiousEvergreenForest1127
Toronto Metropolitan University
Tags
Summary
This chapter discusses estimation procedures in statistics, focusing on point estimates and confidence intervals for sample means and proportions. It explains the concepts of bias and efficiency in estimators, drawing on examples like estimating average income or gas prices. The text emphasizes the importance of sample size in determining the precision of estimates.
Full Transcript
Book Title: eTextbook: Statistics: A Tool for Social Research and Data Analysis, Fifth Canadian Edition Chapter 6. Estimation Procedures for Sample Means and Proportions 6.1. Introduction 6.1. Introduction 175 This chapter looks at estimation procedures....
Book Title: eTextbook: Statistics: A Tool for Social Research and Data Analysis, Fifth Canadian Edition Chapter 6. Estimation Procedures for Sample Means and Proportions 6.1. Introduction 6.1. Introduction 175 This chapter looks at estimation procedures. The object of this branch of inferential statistics is to estimate population values or parameters from statistics computed from samples. Although the techniques presented in this chapter may be new to you, you are certainly familiar with their most common applications: public opinion polls and election projections. Polls and surveys on every conceivable issue—from the sublime to the trivial—have become a staple of the mass media and popular culture. The techniques you will learn in this chapter are essentially the same as those used by the most reputable, sophisticated, and scientific pollsters. There are two kinds of estimation procedures. First, a point estimate is a sample statistic that is used to estimate a population value. For example, a newspaper story that reports that 50% of a sample of randomly selected Canadian drivers are driving less than usual due to high gas prices is reporting a point estimate. The second kind of estimation procedure involves confidence intervals, which consist of a range of values (an interval) instead of a single point. Rather than estimating a specific figure as in a point estimate, an interval estimate might be phrased as “between 47% and 53% of Canadian drivers report driving less than usual due to high gas prices.” In this latter estimate, we are estimating that the population value falls between 47% and 53% , but we do not specify its exact value. Half the size (width) of the confidence interval, which in this example is half the percentage-point difference between 47% and 53% , or 3% , is called the radius of the confidence interval, or the margin of error, or simply the sampling error. Book Title: eTextbook: Statistics: A Tool for Social Research and Data Analysis, Fifth Canadian Edition 6.2. Bias and Efficiency 6.2. Bias and Efficiency Both point and interval estimation procedures are based on sample statistics. Which of the many available sample statistics should be used? Estimators can be selected according to two criteria: bias and efficiency. Estimates should be based on sample statistics that are unbiased and relatively efficient. We will cover each of these criteria separately. Book Title: eTextbook: Statistics: A Tool for Social Research and Data Analysis, Fifth Canadian Edition 6.2. Bias and Efficiency Bias Bias An estimator is unbiased if, and only if, the mean of its sampling distribution is equal to the population value of interest. We know from the theorems presented in Chapter 5 that sample means conform to this criterion. The mean of the sampling distribution of sample means (which we note symbolically as μX ) is the same as the population mean (μ). ¯ Sample proportions (Ps) are also unbiased. That is, if we calculate sample proportions from repeated random samples of size n and then array them in 176 a frequency polygon, the sampling distribution of sample proportions has a mean (μp) equal to the population proportion (Pμ). Thus, if we are concerned with coin flips and we sample honest coins 10 at a time (n = 10) , the sampling distribution has a mean equal to 0.50 , which is the probability that an honest coin will be heads (or tails) when flipped. However, other statistics are biased (i.e., have sampling distributions with means not equal to the population value). Knowing that sample means and proportions are unbiased allows us to determine the probability that they lie within a given distance of the population values we are trying to estimate. To illustrate, consider a specific problem. Assume that we wish to estimate the average income of full-time workers in a community. A random sample of 500 full-time workers is taken (n = 500) , and a sample mean of $75,000 is computed. In this example, the population mean is the average income of all full-time workers in the community, and the sample mean is the average income for the 500 full-time workers that happened to be selected for our sample. Note that we do not know the value of the population mean (μ) —if we did, we wouldn’t need the sample—but it is (μ) that we are interested in. The sample mean of $75,000 is important and interesting primarily insofar as it can give us information about the population mean. The two theorems presented in Chapter 5 give us a great deal of information about the sampling distribution of all possible sample means. Because n is large, we know that the sampling distribution is normal and that its mean is equal to the population mean. We also know that all normal curves contain about 68% of the cases (the cases here are sample means) within ±1 Z (i.e., ±1 standard deviation), 95% of the cases within ±2 Z’s ( ±2 standard deviations), and more than 99% of the cases within ±3 Z’s ( ±3 standard deviations) of the mean. Remember that we are discussing the sampling distribution here—the distribution of all possible sample outcomes or, in this instance, sample means. Thus, the probabilities are very good (approximately 68 out of 100 chances) that our sample mean of $75,000 is within ±1 Z , excellent ( 95 out of 100 ) that it is within ±2 Z’s, and overwhelming ( 99 out of 100 ) that it is within ±3 Z’s of the mean of the sampling distribution (which has the same value as the population mean). These relationships are graphically depicted in Figure 6.1. Figure 6.1 Areas under the Sampling Distribution of Sample Means If an estimator is unbiased, it is probably an accurate estimate of the population parameter ( μ) in this case). However, in less than 1% of the cases, a sample mean will be more than ±3 Z’s away from the mean of the sampling distribution (very inaccurate) by random chance alone. We literally 177 have no idea if our particular sample mean of $75,000 is in this small minority. We do know, however, that the odds are high that our sample mean is considerably closer than ±3 Z’s to the mean of the sampling distribution and, thus, to the population mean. Book Title: eTextbook: Statistics: A Tool for Social Research and Data Analysis, Fifth Canadian Edition 6.2. Bias and Efficiency Efficiency Efficiency The second desirable characteristic of an estimator is efficiency, which is the extent to which the sampling distribution is clustered about its mean. Efficiency or clustering is essentially a matter of dispersion, as we saw in Chapter 3 (see Figure 3.1). The smaller the standard deviation of a sampling distribution, the greater the clustering and the higher the efficiency. Remember that the standard deviation of the sampling distribution of sample means, or the standard error of the mean, is equal to the population standard deviation divided by the square root of n. Therefore, the standard deviation of the sampling distribution is an inverse function of n(σX = σ/√n). As the ¯ sample size increases, σX decreases. We can improve the efficiency (or ¯ decrease the standard deviation of the sampling distribution) for any estimator by increasing the sample size. An example should make this clearer. Consider two samples of different sizes: Sample 1 Sample 2 ¯ ¯ X 1 = $75,000 X 2 = $75,000 n1 = 100 n2 = 1,000 Both sample means are unbiased, but which is the more efficient estimator? Consider sample 1, and assume, for the sake of illustration, that the population standard deviation (σ) is $5,000. In this case, the standard deviation of 178 the sampling distribution of all possible sample means with an n of 100 is σ/√n or 5000/√100 or $500.00. For sample 2, the standard deviation of all possible sample means with an n of 1,000 is much smaller. Specifically, it is equal to 5000/√1000 or $158.13 Figures 6.2 and 6.3 illustrate the sampling distribution for each situation. Figure 6.2 A Sampling Distribution with n = 100 and σx = $500.00 ¯ The sampling distribution in Figure 6.3 is much more clustered than the sampling distribution in Figure 6.2. In fact, the former contains 68% of all possible sample means within ±$158.13 of μ , while the latter requires a much broader interval of ±$500.00 to do the same. The estimate based on a sample with 1,000 cases is much more likely to be close in value to the population parameter than is an estimate based on a sample of 100 cases. Figure 6.3 A Sampling Distribution with n = 1,000 and σx = $158.13 ¯ To summarize: The standard deviation of all sampling distributions is an inverse function of n. Therefore, the larger the sample, the greater the 179 clustering and the higher the efficiency. In part, these relationships between the sample size and the standard deviation of the sampling distribution do nothing more than underscore our common-sense notion that much more confidence can be placed in large samples than in small (as long as both have been randomly selected). Book Title: eTextbook: Statistics: A Tool for Social Research and Data Analysis, Fifth Canadian Edition Chapter 6. Estimation Procedures for Sample Means and Proportions 6.3. Estimation Procedures: Introduction 6.3. Estimation Procedures: Introduction The procedure for constructing a point estimate is straightforward. Draw an EPSEM sample, calculate either a proportion or a mean, and estimate that the population parameter is the same as the sample statistic. Remember that the larger the sample, the greater the efficiency and the more likely that the estimator is approximately the same as the population value. Also remember that no matter how rigid the sampling procedure or how large the sample, there is always some chance that the estimator is very inaccurate. Compared to point estimates, interval estimates are more complicated but safer because when we guess a range of values, we are more likely to include the population parameter. The first step in constructing an interval estimate is to decide on the risk you are willing to take of being wrong. An interval estimate is wrong if it does not include the population parameter. This probability of error is called alpha (𝛂). The exact value of alpha depends on the nature of the research situation, but a 0.05 probability is commonly used. Setting alpha equal to 0.05 , also called using the 95% confidence level, means that over the long run the researcher is willing to be wrong only 5% of the time. Or, to put it another way, if an infinite number of intervals were constructed at this alpha level (and with all other things being equal), 95% of them would contain the population value and 5% would not. In reality, of course, only one interval is constructed and, by setting the probability of error very low, we are setting the odds in our favour that the interval will include the population value. The second step is to picture the sampling distribution, divide the probability of error equally into the upper and lower tails of the distribution, and then find the corresponding Z score. For example, if we decide to set alpha equal to 0.05 , we place half (0.025) of this probability in the lower tail and half in the upper tail of the distribution. The sampling distribution is thus divided as illustrated in Figure 6.4. Figure 6.4 The Sampling Distribution with Alpha (α) Equal to 0.05 We need to find the Z score that marks the beginnings of the shaded areas. In Chapter 4, we learned how to calculate Z scores and find areas under the normal curve. Here, we reverse that process. We need to find the Z score beyond which lies a proportion of 0.0250 of the total area. To do this, go 180 down column (c) of Appendix A until you find this proportion (0.0250). The associated Z score is 1.96. Because the curve is symmetrical and we are interested in both the upper and lower tails, we designate the Z score that corresponds to an alpha of 0.05 as ±1.96 (see Figure 6.5). Figure 6.5 Finding the Z Score That Corresponds to an Alpha (α) of 0.05 We now know that 95% of all possible sample outcomes fall within ±1.96 Z- score units of the population value. In reality, of course, there is only one sample outcome, but if we construct an interval estimate based on ±1.96 Z’s, the probabilities are that 95% of all such intervals will include or overlap the population value. Thus, we can be 95% confident that our interval contains the population value. Besides the 95% level, three other confidence levels are commonly used: the 90% level (α = 0.10) , the 99% level (α = 0.01) , and the 99.9% level (α = 0.001). To find the corresponding Z scores for these levels, follow the procedures outlined above for an alpha of 0.05. Table 6.1 summarizes all the information you need. Table 6.1 Z Scores for Various Levels of Alpha (α) Confidence Alpha 𝛂/2 Z Score Level (%) 90 0.10 0.0500 ±1.65 95 0.05 0.0250 ±1.96 99 0.01 0.0050 ±2.58 99.9 0.001 0.0005 ±3.29 You should turn to Appendix A and confirm for yourself that the Z scores in Table 6.1 do indeed correspond to these alpha levels. As you do, note that, in 181 the cases where alpha is set at 0.10 and 0.01 , the precise areas we seek do not appear in the table. For example, with an alpha of 0.10 , we would look in column (c) (“Area beyond”) for the area 0.0500. Instead we find an area of 0.0505 (Z = ±1.64) and an area of 0.0495 (Z = ±1.65). The Z score we are seeking is somewhere between these two other scores. When this condition occurs, take the larger of the two scores as Z. This will make the interval as wide as possible under the circumstances and is thus the most conservative course of action. In the case of an alpha of 0.01 , we encounter the same problem (the exact area 0.0050 is not in the table), so we resolve it the same way by taking the larger score as Z. Finally, note that in the case where alpha is set at 0.001 , we can choose from several Z scores. Although our table is not detailed enough to show it, the closest Z score to the exact area we want is ±3.291 , which we can round off to ±3.29. (For practice in finding Z scores for various levels of confidence, see Problem 6.3.) The third step is to actually construct the confidence interval. In the following two sections, we illustrate how to construct an interval estimate with sample means; in Section 6.7, we show how to construct an interval estimate with sample proportions. Book Title: eTextbook: Statistics: A Tool for Social Research and Data Analysis, Fifth Canadian Edition Chapter 6. Estimation Procedures for Sample Means and Proportions 6.4. Interval Estimation Procedures for Sample Means (σ Known) 6.4. Interval Estimation Procedures for Sample Means ( σ Known) We can construct a confidence interval based on sample means using Formula 6.1: Formula 6.1 ¯ σ c.i. = X ± Z ( ) √N where c.i. = confidence interval ¯ X = the sample mean Z = the Z score as determined by the alpha level σ √N = the standard deviation of the sampling distribution or the stand 182 As an example, suppose you want to estimate the average IQ of a community and randomly select a sample of 200 residents, with a sample mean IQ of 105. Assume that the population standard deviation for IQ scores is 15 , so we can set σ equal to 15. If we are willing to run a 5% chance of being wrong and set alpha at 0.05 , the corresponding Z score is ±1.96. We can directly substitute these values into Formula 6.1 to construct the interval: c.i. = X ± Z ( √σ ) ¯ N = 105 ± 1.96 ( √15 ) 200 15 = 105 ± 1.96 ( 14.14 ) = 105 ± (1.96)(1.06) = 105 ± 2.08 That is, our estimate is that the average IQ for the population in question is somewhere between 102.92(105 − 2.08) and 107.08(105 + 2.08). Since 95% of all possible sample means are within ±1.96 Z’s (or 2.08 IQ units in this case) of the mean of the sampling distribution, the odds are very high that our interval contains the population mean. In fact, even if the sample mean is as far off as ±1.96 Z’s (which is unlikely), our interval still contains μX and, ¯ thus, μ. Only if our sample mean is one of the few that is more than ±1.96 Z’s from the mean of the sampling distribution will we have failed to include the population mean. A word of caution is in order about sample size. Larger samples (i.e., samples with 100 or more cases) are large enough for the Central Limit Theorem to be applied. As discussed in Section 5.3 in the previous chapter, a sample size of 100 or more is considered sufficiently large for the sampling distribution to be approximately normal. This is not necessarily the case for smaller samples (samples with fewer than 100 cases). To use the standardized normal (Z) distribution to construct confidence intervals for means based on small samples, we must assume that the population the sample is taken from is normally distributed. Recall from Chapter 5 that we can assume that the sampling distribution is normal in shape if either the sample size is large—and the Central Limit Theorem is invoked—or the population is normally distributed. (For practice with confidence intervals for sample means using the standard normal distribution, see Problems 6.1a, 6.1b, 6.1c, and 6.1d.) Book Title: eTextbook: Statistics: A Tool for Social Research and Data Analysis, Fifth Canadian Edition Chapter 6. Estimation Procedures for Sample Means and Proportions 6.5. Interval Estimation Procedures for Sample Means (σ Unknown) 6.5. Interval Estimation Procedures for Sample Means ( σ Unknown) In the previous example, the value of the population standard deviation was known. Needless to say, it is unusual to have such information about a 183 population. In the vast majority of cases, we will have no knowledge of σ. In these cases, however, we can estimate σ with s, the sample standard deviation. Because s is only an estimate of σ , the formula for constructing a confidence interval must be slightly revised. The revised formula for cases in which σ is unknown is Formula 6.2 ¯ s c.i. = X ± t ( ) √N − 1 where c.i. = confidence interval ¯ X = the sample mean t = the t score as determined by the alpha level and n − 1 degrees s √n−1 = the estimated standard error of the mean, when σ is unknown Comparing this formula with Formula 6.1, note that there are three changes. First, σ is replaced by s. Second, the denominator of the last term is the square root of n − 1 rather than the square root of n , to correct for the fact that s is a biased estimator of σ (see Section 6.2 for a discussion on bias). Third, to construct confidence intervals from sample means when σ is unknown, we must use a different theoretical distribution, called the Student’s t distribution, to find areas under the sampling distribution. The Student’s t distribution compensates for the fact that we are estimating the unknown σ with s, and so its shape varies as a function of sample size— the larger the sample, the more accurate the estimate—or more specifically as a function of degrees of freedom (symbolized as df ). When we use the t distribution to construct a confidence interval around a sample mean, the degrees of freedom are equal to n − 1. So, there is a “family” of t sampling distributions, and the value of df defines a specific “member” of this family (i.e., it defines the shape of the t distribution). The relative shapes of the Z distribution and two specific t distributions are depicted in Figure 6.6. For the smaller sample (df = 10) , the t distribution is flatter and has heavier tails (i.e., more area in the tails) than the Z distribution, but, as the sample size increases (df = 100) , the t distribution 184 begins to resemble the Z distribution. The Z and t distributions are essentially identical when the sample size is greater than 100. As n increases, the sample standard deviation becomes a more reliable estimator of the population standard deviation, and so the t distribution becomes more like the Z distribution. Figure 6.6 The Z Distribution and the t Distribution for Selected Degrees of Freedom (df) The t distribution is summarized in Appendix B. The t table differs from the Z table in several ways. First, there is a column on the left side of the table labelled “Degrees of Freedom“ (df). As mentioned above, the exact shape of the t distribution—and thus the exact location of the t score corresponding to our chosen alpha level—varies as a function of sample size (degrees of freedom). Degrees of freedom must first be computed before the t score for any alpha can be located. Second, alpha levels are arrayed across the top of Appendix B in two rows. When we are forming confidence intervals using t, we always use the row labelled “Level of Significance for Two-Tailed Test.” Third, the entries in the table are actual t scores and not areas under the sampling distribution. (For practice in finding t scores for various levels of confidence, see Problem 6.4.) To illustrate the use of this table, let’s find the t score for α =.05 and n = 30. First, we calculate the degrees of freedom (df), which are n − 1 or 29. Next, we scroll down the “Level of Significance for Two-Tailed Test” column at the 0.05 alpha level until we reach the appropriate row, or df = 29. This value, ±2.045 , is the t score. Take a moment to notice an additional feature of the t table. Scan the column for an alpha of 0.05 , and note that, for one degree of freedom, the t score is ±12.706 and that the value of the t score decreases as the degrees of freedom increase. As the sample size increases, the t distribution comes to resemble the Z distribution more and more until, with sample sizes greater than 120 , the two distributions are essentially identical (see Figure 6.6). When the sample 185 size is infinitely large (infinite degrees of freedom), the value of t converges to the value of Z, or ±1.96. This relationship is confirmed in the last row of Appendix B (degrees of freedom = 00) , where the t values correspond to the Z values in Appendix A. Editor, please replace “ 00 ” above with the symbol for infinity. To demonstrate the construction of confidence intervals for sample means using the t distribution, let’s suppose you want to estimate the average IQ of a community using a randomly selected sample of 30 residents. From this sample, we find a mean IQ score of 105 and a standard deviation of 15 , which we will use as an estimate of the population standard deviation. If we are willing to run a 5% chance of being wrong and set alpha at 0.05 , the corresponding t score is 2.045 , as we found previously. We can substitute these values directly into Formula 6.2 to construct an interval: c.i. = X ± t ( √ s ) ¯ n-1 15 = 105 ± 2.045 ( √30−1 ) 15 = 105 ± 2.045 ( 5.39 ) = 105 ± (2.045)(2.78) = 105 ± 5.69 Based on the sample mean of 105 , we estimate the population mean to be located in the range from 99.31 to 110.69 , with only a 5% chance that the actual population mean does not fall in this range. As a final note, caution must be used when n is less than 100 in constructing confidence intervals using the t distribution. As with the standardized normal (Z) distribution, samples with 100 or more cases are large enough for the Central Limit Theorem to be applied. Samples with fewer than 100 cases require us to assume that the population from which the sample is taken is normally distributed. So, in our example, we must assume that IQ scores are normally distributed in the entire population (community) because the sample size is less than 100. Techniques used when this assumption cannot be met are beyond the scope of this text. (For practice with confidence intervals for sample means using the t distribution, see Problems 6.1e–6.1f, 6.5, 6.6, and 6.7, 6.18d, and 6.19a, 6.19b, and 6.19c.) 186 Applying Statistics 6.1. Estimating a Population Mean The Canadian Housing Survey (CHS), conducted in 2018 by Statistics Canada, collected detailed information on how issues related to housing affect the lives of Canadians, including housing costs, first-time homebuyers and affordability, perceptions on neighbourhood safety, and satisfaction and community engagement. Based on a random sample of 16,912 homeowners with mortgages, CHS data show that the average Canadian household owed $213,998 on their mortgage in 2018. Given that the CHS sample reported a mean property mortgage of $213,998 , what is the estimate of the population mean? The information from the sample is ¯ X = 213,998 s = 174,338 N = 16,912 If we set alpha at 0.05 , the corresponding t score is ±1.98 , and the 95% confidence interval is c.i. = X ± t ( √ s ) ¯ n−1 174,338 c.i. = 213,998 ± 1.98 ( √16,912−1 ) 174,338 c.i. = 213,998 ± 1.98 ( 130.04 ) c.i. = 213,998 ± (1.98)(1,340.65) c.i. = 213,998 ± 2,654.49 Based on this result, we estimate the population of homeowners with a mortgage owed on average as $213,998 ± $2,654.49 in 2018. The lower limit of our interval estimate ($213,998 − $2,654.49) is $211,343.51 , and the upper limit ($213,998 + $2,654.49) is $216,652.49. Thus, another way to state the interval is $211, 343.51 ≤ μ ≤ $216, 652.49 The population mean is greater than or equal to $211,343.51 and less than or equal to $216,652.49. Because alpha was set at the 0.05 level, this estimate has a 5% chance of being wrong (i.e., of not containing the population mean). Source: Data from Statistics Canada, 2018 Canadian Housing Survey. Book Title: eTextbook: Statistics: A Tool for Social Research and Data Analysis, Fifth Canadian Edition Chapter 6. Estimation Procedures for Sample Means and Proportions 6.6. Graphing a Confidence Interval of a Sample Mean 6.6. Graphing a Confidence Interval of a Sample Mean A confidence interval of a sample mean can be depicted using a graph called the error bar. The error bar graph is based on the lower and upper limits of the confidence interval of a sample mean. Constructing an error bar takes two steps. First, the sample mean is plotted with a symbol, such as a dot, at the centre of a graph. Second, a vertical line or “error bar” is drawn from the sample mean to the lower limit of its confidence interval, marked by a small horizontal line. The same is done between the sample mean and the upper limit of the confidence interval. The area bounded by the two error bars is equal to the width of the confidence interval of the sample mean. Figure 6.7 displays the error bar graph for the 95% confidence interval of the mean IQ of a community, described in Section 6.5. Recall that the average IQ for 187 the random sample of 30 residents is 105 , with a standard deviation of 15. 188 At the 95% confidence level, the average IQ for the community as a whole is between 99.31 (105 − 5.69) and 110.69 (105 + 5.69). Graphically, the dot in the middle of the error bar graph represents the sample mean, and the vertical lines above and below the sample mean represent the upper and lower limits of the confidence interval. (For practice in constructing and interpreting error bars, see Problem 6.6.) Figure 6.7 Error Bar Graph for the 95% Confidence Interval of the Mean IQ of a Community One Step at a Time Constructing Confidence Intervals for Sample Means Using Formula 6.2 1: Using the table in Appendix B, select an alpha level from the row labelled “Level of Significance for Two-Tailed Test.” Next, find the row corresponding to the degrees of freedom (n − 1) to locate the t score. 2: Substitute the sample values into Formula 6.2 to construct the confidence interval. To Solve Formula 6.2 1: Find the square root of n−1. 2: Divide the value you found in step 1 into s, the sample standard deviation. 3: Multiply the value you found in step 2 by the value of t. 4: The value you found in step 3 is the radius of the confidence interval. To find the lower and upper limits of the interval, subtract this value from and add it to the sample mean. Interpreting the Confidence Interval 1: Express the confidence interval in a sentence or two that identifies each of these elements: a. the sample statistic (a mean in this case) b. the confidence interval c. the sample size (n) d. the population to which you are estimating e. the confidence level (e.g., 95% ) Book Title: eTextbook: Statistics: A Tool for Social Research and Data Analysis, Fifth Canadian Edition Chapter 6. Estimation Procedures for Sample Means and Proportions 6.7. Interval Estimation Procedures for Sample Proportions (Large Samples) 6.7. Interval Estimation Procedures for Sample Proportions (Large Samples) Estimation procedures for sample proportions are essentially the same as those for sample means. The major difference is that, because proportions are different statistics, we must use a different sampling distribution—the distribution of sample proportions. Fortunately, as per the Central Limit Theorem (see Section 5.5 in the previous chapter), if both nPμ and n(1 − Pμ) are greater than or equal to 15 , we can assume that the sampling distribution of proportions is approximately normal in shape, with mean (μp) equal to the population value (Pμ) and standard deviation (σp) equal to √Pμ(1 − Pμ)/n. Because we can assume a normal distribution, we can then use the standardization (Z) formula to construct a confidence interval for a proportion as follows: Formula 6.3 Pμ(1 − Pμ) c.i. = Ps ± Z √ n c.i. = confidence interval Ps = the sample proportion Z = the Z score as determined by the alpha level √ Pμ(1−Pμ) = the standard deviation of the sampling distribution of pr n The values for Ps , the sample proportion, and n, the sample size, come directly from the sample, and the value of Z is determined by the confidence level, as was the case with sample means. This leaves one unknown in the formula, Pμ —the same value we are trying to estimate! As with the population standard deviation, σ , when constructing a confidence interval 189 for sample means, the population proportion Pμ is rarely known, so we estimate it with our best guess, the sample proportion Ps , as follows: Formula 6.4 Ps(1 − Ps) c.i. = Ps ± Z √ N To illustrate, assume that you wish to estimate the proportion of students at your university who missed at least one day of classes because of illness last semester. Out of a random sample of 200 students, 60 reported that they had been sick enough to miss classes at least one day during the previous semester. The sample proportion, Ps , upon which we will base our estimate is thus 60/200 , or 0.30. Before constructing the confidence interval for the proportion, we must check the assumption that both nPμ ≥ 15 and n(1 − Pμ) ≥ 15. We can assume that the sampling distribution is approximately normal if we meet this assumption. In the current example, and using Ps as a substitute for Pμ , we find that nPs = 200(0.30) n(1 − Ps) = 200(0.70) = 60 = 140 Both nPs , or 60 , and n(1 − Ps) , or 140 , are well above the minimal value of 15. In other words, the sample is sufficiently large and Ps is far enough from zero or one to safely assume a normal distribution and use the standardization (Z) formula in Formula 6.4. At the 95% level of confidence, the interval estimate is Ps(1−Ps) c.i. = Ps ± Z √ n 0.30(0.70) = 0.30 ± 1.96√ 200 = 0.30 ± 1.96√ 0.21 200 = 0.30 ± 1.96(0.032) = 0.30 ± 0.06 Based on this sample proportion of 0.30 , you would estimate that the proportion of students who missed at least one day of classes because of illness was between 0.24 and 0.36. The estimate could, of course, also be phrased in percentages by reporting that between 24% and 36% of the student body was affected by illness at least once during the past semester, at the 95% level of confidence. As with the sample mean, the error bar can also be used to graph the confidence interval of a sample proportion. It is important to point out that in this example we are constructing a confidence interval for the proportion of a binary variable—a variable with only two categories (missed class this semester, yes or no?). When dealing with a variable with more than two categories, it is often convenient to estimate the value of Ps at a constant 0.50 for each category. Furthermore, this approach 190 is the most conservative solution to the dilemma posed by having to assign a value to Ps in the estimation equation—because the second term in the numerator under the radical (1 − Ps) in Formula 6.4 is the reciprocal of Ps , the entire expression always has a value of 0.50 × 0.50 , or 0.25 , which is the maximum value this expression can attain. If we set Ps at 0.40 , for example, the second term (1 − Ps) is 0.60 , and the value of the entire expression decreases to 0.24. Setting Ps at 0.50 ensures that the expression Ps(1 − Ps) is at its maximum possible value (0.25) and, consequently, the interval is at maximum width. See Table 6.6 for an example of setting Ps at 0.50 for each category of the variable “voting intention in a federal election.” (For practice with confidence intervals for sample proportions, see Problems 6.2, 6.8, 6.9, 6.10, 6.11, and 6.12, 6.15, 6.16, and 6.17, and 6.19d, 6.19e, 6.19f, and 6.19g.) Prostock-studio/Shutterstock.com To estimate a population proportion, such as the proportion of students who missed a class because of illness, use the confidence interval to estimate a range of values. Book Title: eTextbook: Statistics: A Tool for Social Research and Data Analysis, Fifth Canadian Edition Chapter 6. Estimation Procedures for Sample Means and Proportions 6.8. A Summary of the Computation of Confidence Intervals 6.8. A Summary of the Computation of Confidence Intervals To this point, we have covered the construction of confidence intervals using four different formulas. Table 6.2 presents these formulas organized by the situations in which they are used. For sample means with large samples (n ≥ 100) or normally distributed populations, when the population standard deviation is known, use Formula 6.1. When the population standard deviation is unknown, use Formula 6.2. For sample proportions, when both nPμ ≥ 15 and n(1 − Pμ) ≥ 15 , use Formula 6.3 if Pμ is known and Formula 6.4 if Pμ is unknown. Because the population standard deviation and proportion are almost never known, Formulas 6.2 and 6.4 are typically used to construct confidence intervals for means and proportions, respectively. 191 192 Table 6.2 Choosing Formulas for Confidence Intervals If the Sample and Use Formula Statistic Is a mean ¯ population 6.1 c.i. = X ± Z ( σ ) standard √ N deviation is known and n ≥ 100 or population is normally distributed mean ¯ population 6.2 c.i. = X ± t ( s ) standard √ n − 1 deviation is unknown and n ≥ 100 or population is normally distributed proportion population 6.3 proportion is Pμ(1 − Pμ) c.i. = Ps ± Z √ known and n nPμ and n(1 − Pμ) are ≥ 15 If the Sample and Use Formula Statistic Is a proportion population 6.4 proportion is Ps(1 − Ps) c.i. = Ps ± Z √ unknown and n nPμ and n(1 − Pμ) are ≥ 15 Applying Statistics 6.2. Estimating Population Proportions To assess how the COVID-19 pandemic has shaped the personal lives of Canadians, a Leger Marketing survey asked a random sample of 1,542 people to compare their lives in general before and after the pandemic started. One of the survey questions asked if, “Overall, when you compare to how things like health, finances, and lifestyle were for you (and, if applicable, your family) before the pandemic, are you worse off or about the same or better off since the start of the pandemic?” The survey found that 29% of Canadians reported that they were worse off than before the pandemic (this percentage is stated as a proportion below). Based on this finding, what is the estimate of the population value? The sample information is Ps = 0.29 n = 1,542 If we set alpha at 0.05 , the corresponding Z score is ±1.96 , and the interval estimate of the population proportion is Ps(1−Ps) c.i. = Ps ± Z √ n 0.29(0.71) = 0.29 ± 1.96√ 1,542 0.21 = 0.29 ± 1.96√ 1,542 = 0.29 ± 1.96(0.012) = 0.29 ± 0.02 We can now estimate that the proportion of the population that believes their lifestyle and habits have changed for the worse during the pandemic is between 0.27 and 0.31. That is, the lower limit of the interval estimate is 0.29 − 0.02 or 0.27 , and the upper limit is 0.29 + 0.02 or 0.31. We may also express this result in percentages and say that between 27% and 31% of the population is of the opinion that they are worse off than before the pandemic. This interval has a 5% chance of not containing the population value. Source: Leger Marketing. (2021). https://leger360.com/surveys/legers-north- american-tracker-june-22-2021/. One Step at a Time Constructing Confidence Intervals for Sample Proportions Using Formula 6.4 Begin by selecting an alpha level and finding the associated Z score in Table 6.1. If you use the conventional alpha level of 0.05 , the Z score is ±1.96. To Solve Formula 6.4 1: Multiply Ps by 1 − Ps. 2: Divide n into the value you found in step 1. 3: Find the square root of the value you found in step 2. 4: Multiply the value you found in step 3 by the value of Z. 5: The value you found in step 4 is the radius of the confidence interval. To find the lower and upper limits of the interval, subtract this value from and add it to the sample proportion. Interpreting the Confidence Interval 1: Express the confidence interval in a sentence or two that identifies each of these elements: a. the sample statistic (a proportion in this case) b. the confidence interval c. the sample size (n) d. the population to which you are estimating e. the confidence level (e.g., 95% ) Book Title: eTextbook: Statistics: A Tool for Social Research and Data Analysis, Fifth Canadian Edition Chapter 6. Estimation Procedures for Sample Means and Proportions 6.9. Controling the Width of Interval Estimates 6.9. Controling the Width of Interval Estimates The radius of a confidence interval for either sample means or sample proportions can be partly controlled by manipulating two terms in the equation. First, the confidence level can be raised or lowered, and, second, the interval can be widened or narrowed by gathering samples of different sizes. The researcher alone determines the risk they are willing to take of being wrong (i.e., of not including the population value in the interval estimate). The exact confidence level (or alpha level) depends, in part, on the purpose of the research. For example, if potentially harmful drugs are being tested, the researcher will naturally demand very high levels of confidence ( 99.99% or even 99.999% ). On the other hand, if intervals are being constructed only for loose “guesstimates,” then much lower confidence levels can be tolerated (such as 90% ). The relationship between interval size and confidence level is that intervals widen as confidence levels increase. This should make intuitive sense. Wider intervals are more likely to include or overlap the population value; hence, more confidence can be placed in them. To illustrate this relationship, let us work through a hypothetical problem. The average salary for a random sample of 500 assistant professors at Canadian universities is $115,000 , with a known population standard deviation of $11,000. In constructing a 95% confidence interval, we find that it extends 193 $964.22 above and below the sample mean (i.e., the interval is $115,000 ± $964.22. If we had constructed the 90% confidence interval for these sample data (a lower confidence level), the Z score in the formula would have decreased to ±1.65 , and the interval would have been narrower: ¯ σ c.i. = X ± Z ( √n ) 11,000 = 115,000 ± 1.65 ( √500 ) = 115,000 ± (1.65)(491.95) = 115,000 ± 811.72 On the other hand, if we had constructed the 99% confidence interval, the Z score would have increased to ±2.58 , and the interval would have been wider: ¯ σ c.i. = X ± Z ( √n ) 11,000 = 115,000 ± 2.58 ( √500 ) = 115,000 ± (2.58)(491.95) = 115,000 ± 1,269.23 At the 99.9% confidence level, the Z score would be ±3.29 , and the interval would be wider still: ¯ σ c.i. = X ± Z ( √n ) = 115,000 ± (3.29) ( 11,000 √ ) 500 = 115,000 ± (3.29)(491.95) = 115,000 ± 1,618.51 These four intervals are grouped together in Table 6.3, and the increase in interval size can be readily observed. Although sample means have been used to illustrate the relationship between interval width and confidence level, exactly the same relationships apply to sample proportions. (To further explore the relationship between alpha and interval width, see Problem 6.13.) Table 6.3 Interval Estimates for Four Confidence Levels ¯ (X = $115,000, σ = $11,000, n = 500 throughout) Sample size bears the opposite relationship to interval width. As sample size increases, interval width decreases. Larger samples give more precise (narrower) estimates. Again, an example should make this clearer. In Table 6.4, confidence intervals for four samples of various sizes are constructed and then grouped together for the purposes of comparison. The sample data are the 194 same as in Table 6.3, and the confidence level is 95% throughout. The relationships illustrated in Table 6.4 also hold true, of course, for sample proportions. (To further explore the relationship between sample size and interval width, see Problem 6.14.) Table 6.4 Interval Estimates for Four Different Samples ( X = $115,000, σ = $11,000, σ = 0.05 throughout) ¯ Notice that the decrease in interval width (or increase in precision) does not bear a constant or linear relationship with sample size. Comparing sample 2 to sample 1, the sample size increased by a factor of five, but the interval is not one fifth the width. This is an important relationship because it means that n might have to be increased many times to appreciably improve the accuracy of an estimate. Because the cost of a research project is a direct function of sample size, this relationship implies a point of diminishing returns in estimation procedures. A sample of 10,000 costs about twice as much as a sample of 5,000 , but estimates based on the larger sample are not twice as precise. That having been said, Table 6.4 also shows that the largest relative benefit for narrowing a confidence interval can be obtained by increasing the 195 size of a small sample even by a fairly modest (and therefore more affordable) amount. Book Title: eTextbook: Statistics: A Tool for Social Research and Data Analysis, Fifth Canadian Edition Chapter 6. Estimation Procedures for Sample Means and Proportions 6.10. Determining Sample Size 6.10. Determining Sample Size Have you ever wondered how researchers or pollsters decide how many people to include in a random sample when conducting a survey or poll? Well, they use a process similar to “reverse engineering.” That is, they determine how many people are needed in a random sample to obtain a desired confidence interval. For instance, a gerontologist wants to estimate the mean income of older adults in Canada. How many would they have to sample to be 99% confident that the sample mean is within plus or minus $1,000 (margin of error, ME) of the population mean, where previous research showed that the population standard deviation is $5,000 (lacking knowledge of σ , the gerontologist can replace it with s, the sample standard deviation, from a similar study done in the past if available). Or, what sample size would a public opinion pollster need if they wanted to know what proportion of Canadians support reinstating the death penalty for murder within plus or minus 3% with a 95% certainty? We can solve these problems by simply rearranging the formulas for constructing a confidence interval. For the mean, Formula 6.5 finds the sample size needed in a simple random sample to get results with the desired level of precision: Formula 6.5 Z 2 × σ2 n= ME 2 where n = required sample size Z = Z score as determined by the alpha level σ = population standard deviation ME = margin of error Using this formula, the gerontologist can calculate the n needed for a 99% confidence interval, where Z equals 2.58 and the margin of error is 1,000 : Z 2×σ2 n = ME 2 2.582×5,0002 = 1,0002 6.6564×25,000,000 = 1,000,000 166,410,000 = 1,000,000 = 166.41 196 The gerontologist needs to randomly sample, rounding up, at least 167 older adults to be 99% confident that the sample mean is within plus or minus $1,000 of the population mean, that is, the mean income of all older adults in Canada. For the proportion, Formula 6.6 finds the sample size required to get the desired results in a simple random sample: Formula 6.6 Z 2 × Pμ(1 − Pμ) n= ME 2 where n = required sample size Z = Z score as determined by the alpha level Pμ = population proportion ME = margin of error With this formula, the public opinion pollster can calculate the n needed for a 95% confidence interval, where Z equals 1.96 , the margin of error (expressed as a proportion) is 0.03 , and Pμ is 0.50 (because prior knowledge of Pμ does not exist, the pollster uses the most conservative guess of 0.50 , or 50% , which produces the largest possible n): Z 2×Pμ(1−Pμ) n = ME 2 1.962×(0.50)(0.50) = 0.032 3.8416×0.25 = 0.0009 0.9604 = 0.0009 = 1,067.11 So, at least 1,068 Canadians need to be randomly sampled for the pollster to be 95% confident that the sample proportion will be within plus or minus 0.03 of the population proportion. The information in this example might sound familiar. Pollsters typically report their findings to the media with this information in a footnote, telling us that their estimate is “accurate within ±3% , 19 times out of 20 ” or that the “margin of error for a sample of 1,068 is ±3% , 19 times out of 20.” This example also reveals why political polls tend to randomly sample only 1,000 or so people—it is not necessary to have a sample much larger than around 1,000 because we are already 95% confident that our sample results will have a high level of precision, with a margin of error of just plus or minus 3%. Furthermore, and as noted in the previous section, the relationship between sample size and level of precision is not linear. That is, that doubling the sample size, for instance, does not cut the margin of error in half. Doubling 197 the sample size from 1,068 to 2,136 cuts the margin of error from ±3% to just ±2.1%. A relatively small increase in precision may not justify the cost associated with doubling the sample size. As a final note, Formulas 6.5 and 6.6 are used to determine the sample size of a simple random sample when the population of interest is very large, typically a population size of 100,000 or more. Otherwise these formulas need to be slightly modified to correct for sampling design (when a simple random sample is not used) and/or population size (when the population size is less than 100,000 ). Book Title: eTextbook: Statistics: A Tool for Social Research and Data Analysis, Fifth Canadian Edition 6.11. Interpreting Statistics: Predicting the Election of the Government of Canada 6.11. Interpreting Statistics: Predicting the Election of the Government of Canada The statistical techniques covered in this chapter have become a part of everyday life in Canada and elsewhere. In politics, for example, estimation techniques are used to track public sentiment, measure how citizens perceive the performance of political leaders, and project the likely winners of upcoming elections. We should acknowledge, nonetheless, that these applications of estimation techniques are also controversial. Many wonder if the easy availability of polls makes politicians overly sensitive to the whims of public sentiment. Others are concerned that election projections might work against people’s readiness to participate fully in the democratic process and, in particular, cast their votes on election day. These are serious concerns, but, in this textbook, we can do little more than acknowledge them and hope that you have the opportunity to pursue them in other, more appropriate settings. In this installment of Interpreting Statistics, we will examine the role of statistical estimation of voting intentions in the 2021 campaign for the federal election (election projections) that culminated in the re-election of Justin Trudeau and the Liberal Party. We could not examine trends in post-election polls on party support after the 2021 election as this data was not available at the time of writing. However, both kinds of polls use the same formulas introduced in this chapter to construct confidence intervals (although the random samples were assembled according to a more complex technique that is beyond the scope of this textbook). Book Title: eTextbook: Statistics: A Tool for Social Research and Data Analysis, Fifth Canadian Edition 6.11. Interpreting Statistics: Predicting the Election of the Government of Canada Election Projections Election Projections Table 6.5 presents the results of five surveys conducted in the weeks leading up to the September 20, 2021, federal election by EKOS Research Associates Inc., a Canadian public opinion research firm. The two left-hand columns show the dates of the polls and the sample size (n) for each poll. The six right-hand columns list the percentage of the sample that said, at the time the poll was conducted, that they intended to vote for each party. These are point estimates of the population parameters, which would be the actual percentage of the 198 entire national electorate that would vote for the Liberal Party of Canada (LPC), Conservative Party of Canada (CPC), New Democratic Party (NDP), Bloc Québécois (BQ), Green Party of Canada (GPC), or People’s Party of Canada (PPC), at that specific time. Results are shown in percentages for ease of communication, but actual estimates would be computed using sample proportions. Table 6.5 EKOS Research Polling Results for the 2021 Canadian Federal Election Poll Date Percentage of Sample for Sample LPC CPC NDP BQ GPC PPC Size (n) August 16, 840 33.7 29.4 20.5 5.4 5.7 4.2 2021 August 23, 1,192 32.0 32.8 19.6 6.2 3.5 5.0 2021 August 30, 942 31.3 35.9 18.0 5.8 3.2 5.0 2021 September 1,062 30.8 33.7 19.2 3.8 3.8 7.9 6, 2021 September 1,241 31.2 32.2 19.4 6.2 3.1 7.3 13, 2021 Source: EKOS Research Associates Inc., https://www.ekospolitics.com. Over the last few weeks of the campaign, Table 6.5 shows that the lead went back and forth between the Liberals and the Conservatives, but the difference in support for either party was very small. This made it difficult to discern which of these two parties was probably going to win the election. Let us assume that we wanted to predict the election results based on the September 13, 2021, poll, when the Conservatives had a lead of 1 percentage point over the Liberals. Does this mean that the Conservatives were actually ahead of the Liberals in the popular vote? The percentages in Table 6.5 are point estimates, and these types of estimates are unlikely to match their respective parameters exactly. In other words, it is unlikely that the sample values in the table were exactly equal to the percentage of the electorate who were going to vote for each of the parties on that particular date. For this reason, confidence intervals would be safer because they use ranges of values, not single points, to estimate population values. Table 6.6 displays the 95% confidence intervals for each party for these five polls, with the results again expressed as percentages rather than proportions. The date and sample size of each poll are displayed in the left-most columns, followed by the 95% confidence intervals for each estimate and the width of the interval estimates. Table 6.6 Sample Size, Confidence Intervals, and Likely Winners for the 2021 Canadian Federal Election Source: EKOS Research Associates Inc., https://www.ekospolitics.com. Before we look at the confidence intervals, we should point out that Table 6.6 underscores a very important point about estimation: as the sample size increases, the width of the interval narrows. For example, the first poll, taken on August 16, 2021, was based on a smaller sample (n = 840) and was 6.8 percentage points wide, wider than the other four polls, which were based on larger samples. 199 The most important information in Table 6.6 is, of course, the intervals themselves, and it is an overlap in some intervals that can cause pollsters to declare a race too close to call. So, for example, the poll on September 13, 2021, showed that support for the Liberals was between 28.4% (31.2% − 2.8%) and 34.0% (31.2% + 2.8%) , a width of 5.6 percentage points. Support for the Conservatives in this poll was between 29.4% and 35.0% , again a width of 5.6 percentage points. It is also worth noting that the confidence intervals for each polling date have the same width because they were computed using the same sample size, n, confidence level, 95% , and estimate of Pμ , 0.50 , for each political party (see Section 6.7). Remember that while we can be 95% sure that the parameter is in the interval, it may be anywhere between the upper and lower limits. This poll tells us that it was just as likely that the Liberals would win (e.g., support for the Liberals could have been as high as 34.0% , and support for Conservatives the could have been as low as 29.4% ) as it was that the Conservatives would win (e.g., support for the Conservatives could have been as high as 35.0% , and support for the Liberals could have been as low as 28.4% ). When the confidence intervals overlap like this, it is possible that the apparent losing party is actually ahead. In the end, when the intervals overlap—when the race is so close—the polls cannot identify a winner. Of course, we know that the Liberals went on to win the election and form a single-party minority government, while the Conservatives formed the official opposition, even though the Conservatives won the popular vote with 33.7% of all votes compared to 32.6% for the Liberals. 200 201 202 Marc Bruxelle/Shutterstock.com Polls conducted leading up to an election are used to try to gauge support for each party and predict which political party will win. Reading Statistics 4. Polls You are most likely to encounter the estimation techniques covered in this chapter in the mass media in the form of public opinion polls, election projections, and the like. Professional polling firms use interval estimates, and responsible reporting by the media usually emphasizes the estimate itself (e.g., “In a survey of the Canadian public, 57% of the respondents said that they approve of the prime minister’s performance”) but will also report the width of the interval (“This estimate is accurate to within ±3% ,” or “Figures from this poll are subject to a margin of error of ±3% ”), the alpha level (usually as the confidence level of 95% ), and the size of the sample (“ 1,458 households were surveyed”). Election projections and voter analyses have been common since the middle of the 20th century and are discussed further in Section 6.11. More recently, public opinion polling has increasingly been used to gauge reactions to everything from the newest movies to the hottest gossip to the prime minister’s conduct during the latest crisis. News websites routinely report poll results as an adjunct to news stories. We would include an example or two of these applications here, but polls have become so pervasive that you can choose your own example. Just find a news website, such as CBC, CTV, or Global news, casually browse it, and we bet that you’ll find at least one poll. Read the story and try to identify the population, the confidence interval width, the sample size, and the confidence level. Bring the news item to the attention of your class and dazzle your instructor. As a citizen, as well as a social scientist, you should be extremely suspicious of polls that do not include such vital information as sample size or interval width. You should also find out how the sample was assembled. Samples selected in a non-random fashion cannot be regarded as representative of the Canadian public or, for that matter, of any population larger than the sample itself. Such non-scientific polls can be found when local television news or sports programs ask viewers to call in and register their opinions about some current controversy. These polls are for entertainment only and must not be taken seriously. You should, of course, read all polls and surveys critically and analytically, but you should place confidence only in polls that are based on samples selected according to the rule of EPSEM (see Chapter 5) from some defined population. In addition, advertisements and reports published by partisan groups sometimes report statistics that seem to be estimates of the population. Often, such estimates are based on woefully inadequate sampling sizes and biased sampling procedures, and the data are collected under circumstances that evoke a desired response. “Person in the street” (or shopper in the grocery store) interviews have a certain folksy appeal but must not be accorded the same credibility as surveys conducted by reputable polling firms. The social research industry in Canada, however, is regulated to some extent. By and large, the industry is self-regulated. The Marketing Research and Intelligence Association, representing most of the industry, has adopted codes of ethics and established standards for reporting and interpreting survey research results. Further, the reporting of election survey results during federal election campaigns is formally regulated by the Canada Elections Act. For instance, federal electoral legislation requires that published election period poll results contain basic methodological information such as margin of error and the date on which the poll was conducted. Public Opinion Surveys in the Professional Literature Thousands of political, social, and market research polls are conducted each year in Canada. For the social sciences, probably the single most important consequence of the growth in opinion polling is that many nationally representative databases are now available for research purposes. These high-quality databases are often available for free or for a nominal fee, and they make it possible to conduct state-of-the-art research without the expense and difficulty of collecting data ourselves. This is an important development because we can now easily test our theories against very high-quality data, and our conclusions will thus have a stronger empirical basis. Our research efforts will have greater credibility with our colleagues, with policymakers, and with the public at large. One of the more important and widely used databases of this sort is the General Social Survey (GSS). Since 1985, Statistics Canada has annually questioned a nationally representative sample of Canadians about a wide variety of issues and concerns. Because many of the questions are asked every five years or so, the GSS offers a longitudinal record of Canadian sentiment and opinion about a large variety of topics (e.g., see Reading Statistics 5). Each year, new topics of current concern are added and explored, expanding the variety of information available. Like other nationally representative samples, the GSS sample is chosen by a probability design based on the principle of EPSEM (see Chapter 5). With a sample size in the thousands, GSS estimates are highly precise (see Table 6.4 and Section 6.9 for a discussion on the relationship between sample size and precision of estimates). The computer exercises in this textbook are based on the 2018 GSS. This database is described more fully in Appendix G. Reading Statistics 5. Using Representative Samples to Track National Trends Among other uses, the General Social Survey (GSS) can be used to track national trends and shifts in public opinion over time because many of the same questions are asked every few years. We demonstrate here how to assess trends in criminal victimization using the 1993, 1999, 2004, 2009, and 2014 GSS. We considered two questions that measure perceptions of crime. The two questions, asked in the 1993, 1999, 2004, 2009, and 2014 GSS, are as follows: Q1. Compared to other areas in Canada, do you think your neighbourhood has a higher amount of crime, about the same, or a lower amount of crime? Q2. Do you feel very safe, reasonably safe, somewhat unsafe, or very unsafe from crime walking alone in your area after dark? Over this period, respondents were less likely to say that their neighbourhood had above-average crime and that they felt unsafe from crime after dark. Figure 1 shows that the point estimate for the proportion of those who thought their neighbourhood has higher than average crime fell from 0.116 (or 11.6% ) in 1993 to 0.039 (3.9%) in 2014, as did the proportion who felt unsafe from crime while walking after dark: 0.273 in 1993 to 0.062 in 2014. Figure 1 Proportion of Respondents Who Said Their Neighbourhood Has Higher Crime and They Feel Unsafe Walking Alone after Dark, 1993 to 2014 To estimate the population parameters or the proportions for all Canadians, interval estimates were calculated using Formula 6.4. The results, expressed this time in percentages, are shown in Table 1. For example, we can conclude that at the 95% confidence level, between 5.66% and 6.74% (6.2 ± 0.54) of Canadians felt unsafe walking alone after dark in 2014. In conclusion, the trends in these data suggest that Canadians’ perception of crime generally declined over those 21 years. Table 1 95% Confidence Interval of Percentage Who Said Neighbourhood Has Higher Crime and They Feel Unsafe Walking Alone after Dark, 1993, 1999, 2004, 2009, and 2014 Year Sample Neighbourhood Unsafe Walking Size Has Higher after Dark Crime 1993 11,960 11.6 ± 0.90 27.3 ± 0.90 1999 25,876 8.5 ± 0.61 17.1 ± 0.61 2004 23,766 8.5 ± 0.63 16.0 ± 0.63 2009 19,422 7.6 ± 0.70 12.9 ± 0.70 2014 33,089 3.9 ± 0.54 6.2 ± 0.54 Book Title: eTextbook: Statistics: A Tool for Social Research and Data Analysis, Fifth Canadian Edition Chapter 6. Estimation Procedures for Sample Means and Proportions Summary Summary 1. Population values can be estimated with sample values. With point estimates, a single sample statistic is used to estimate the corresponding population value. With confidence intervals, we estimate that the population value falls within a certain range of values. 2. Estimates based on sample statistics must be unbiased and relatively efficient. Of all the sample statistics, only means and proportions are 203 unbiased. The means of the sampling distributions of these statistics are equal to the respective population values. Efficiency is largely a matter of sample size. The greater the sample size, the lower the value of the standard deviation of the sampling distribution, the more tightly clustered the sample outcomes will be around the mean of the sampling distribution, and the more efficient the estimate. 3. With point estimates, we estimate that the population value is the same as the sample statistic (either a mean or a proportion). With interval estimates, we construct a confidence interval, a range of values into which we estimate that the population value falls. The width of the interval is a function of the risk we are willing to take of being wrong (the alpha level) and the sample size. The interval widens as our probability of being wrong decreases and as the sample size decreases. 4. A confidence interval can be depicted using an error bar graph. The middle of an error bar graph represents the sample statistic, and vertical lines above and below the sample statistic represent the upper and lower limits of the confidence interval. 5. The sample size needed to obtain a desired confidence interval can be determined before conducting a study. Sample size is determined by “rearranging” the formulas for confidence intervals. 6. To construct confidence intervals for sample means, use the standard normal distribution (Z) when the population standard deviation is known (and the sample size is large or the population normally distributed); otherwise, use the Student’s t distribution. For sample proportions with large samples, use the standard normal distribution (Z). Summary of Formulas Confidence interval for a sample σ ¯ c.i. = X ± Z ( ) mean, population standard √n deviation known and large sample or population normally distributed Confidence interval for a sample ¯ c.i. = X ± t ( s ) mean, population standard √ n − 1 deviation unknown and large sample or population normally distributed Confidence interval for a sample Pμ(1 − Pμ) c.i. = P s ± Z √ proportion, population proportion n known and large sample Confidence interval for a sample Ps(1 − Ps) c.i. = P s ± Z √ proportion, population proportion n unknown and large sample Required sample size, for a mean n= Z 2 × σ2 ME 2 Required sample size, for a Z 2 × Pμ × (1 − Pμ) n= proportion ME 2 Glossary 204 Alpha (α) Bias Confidence intervals Confidence level Efficiency Error bar Margin of error Point estimate Student’s t distribution Multimedia Resources Visit the companion website for the fifth Canadian edition of Statistics: A Tool for Social Research and Data Analysis to access a wide range of student resources: www.cengage.com/healey5ce. Problems 6.1 For each sample mean below, construct the 95% confidence interval for estimating μ , the population mean. a. X = 5.2 ¯ σ = 0.7 n = 157 SHOW ANSWER b. X = 100 ¯ σ=9 n = 620 SHOW ANSWER c. X = 20 ¯ σ=3 n = 220 SHOW ANSWER d. X = 1,020 ¯ σ = 50 n = 329 SHOW ANSWER e. X = 7.3 ¯ s = 1.2 n = 105 SHOW ANSWER f. X = 33 ¯ s=6 n = 220 SHOW ANSWER 6.2 For each set of sample outcomes below, construct the 99% confidence interval for estimating Pμ. a. Ps = 0.14 n = 100 b. Ps = 0.37 n = 522 c. Ps = 0.79 n = 121 d. Ps = 0.43 n = 1049 e. Ps = 0.40 n = 548 f. Ps = 0.63 n = 300 6.3 For each confidence level below, determine the corresponding Z score. Confidence Level Alpha Area Z Score (%) beyond Z 95% 0.05 0.0250 ±1.96 94% 92% 97% 98% 99.9% 205 SHOW ANSWER 6.4 For each sample size and confidence level below, determine the corresponding t score. Sample Size Confidence Alpha t Score Level (%) 5 95 0.05 2.776 6 99 15 95 15 99 30 99 31 95 35 95 39 95 61 90 6.5 SOC A researcher has gathered information from a random sample of 178 households. For each variable below, construct confidence intervals to estimate the population mean. Use the 90% level. a. An average of 2.3 people reside in each household. The standard deviation is 0.35. SHOW ANSWER b. There was an average of 2.1 televisions (s = 0.10) and 0.78 cars (s = 0.55) per household. SHOW ANSWER c. The households averaged 6.0 hours of television viewing per day (s = 3.0). SHOW ANSWER 6.6 SOC A random sample of 100 television programs contained an average of 2.37 acts of physical violence per program. At the 99% level, what is your estimate of the population value? Construct an error bar graph to display your results. ¯ X = 2.37 s = 0.30 n = 100 6.7 SOC A random sample of 429 university students were interviewed about a number of matters. a. They reported that they had spent an average of $178.23 per textbook during the previous semester. If the sample standard deviation for these data is $15.78 , construct an estimate of the population mean at the 99% level. SHOW ANSWER b. They also reported that they had visited the health services clinic an average of 1.5 times a semester. If the sample standard deviation is 0.30 , construct an estimate of the population mean at the 99% level. SHOW ANSWER c. On average, the sample had missed 2.8 days of classes per semester because of illness. If the sample standard deviation is 1.0 , construct an estimate of the population mean at the 99% level. SHOW ANSWER d. On average, the sample had missed 3.5 days of classes per semester for reasons other than illness. If the sample standard deviation is 1.5 , construct an estimate of the population mean at the 99% level. SHOW ANSWER 6.8 CJ A random sample of 500 residents of a city shows that exactly 50 of the respondents had been the victims of violent crime over the past year. Estimate the proportion of victims for the population as a whole, using the 90% confidence level. (HINT: Calculate the sample proportion Ps before using Formula 6.4. Remember that proportions are equal to frequency divided by n.) 6.9 SOC The survey mentioned in Problem 6.5 found that 25 of the 178 households consisted of unmarried couples who were living together. What is your estimate of the population proportion? Use the 95% level. SHOW ANSWER 6.10 PA A random sample of 324 residents of a community revealed that 30% were very satisfied with the quality of garbage collection. At the 99% level, what is your estimate of the population value? 6.11 SOC A random sample of 1,496 respondents of a major metropolitan area were questioned about a number of issues. Construct estimates for the population at the 90% level for each of the results reported below. Express the final confidence interval in percentages (e.g., “between 40% and 45% agreed that premarital sex was always wrong”). a. When asked to agree or disagree with the statement, “Explicit sexual books and magazines lead to rape and other sex crimes,” 823 agreed. SHOW ANSWER b. When asked to agree or disagree with the statement, “Guns should be outlawed,” 650 agreed. SHOW ANSWER c. 375 of the sample agreed that marijuana should be legalized. SHOW ANSWER d. 1,023 of the sample said that they had attended a religious service at least once within the past month. SHOW ANSWER e. 800 agreed that public elementary schools should have sex education programs starting in Grade 5. SHOW ANSWER 6.12 SW A random sample of 100 patients treated in a program for alcoholism and drug dependency over the past 10 years was selected. It was determined that 53 of the patients had been re-admitted to the program at least once. At the 95% level, construct an estimate for the population proportion. 206 6.13 For the sample data below, construct four different interval estimates of the population mean, one each for the 90% , 95% , 99% , and 99.9% level. What happens to the interval width as the confidence level increases? Why? ¯ X = 100 s = 100 n = 500 SHOW ANSWER 6.14 For each of the three sample sizes below, construct the 95% confidence interval. Use a sample proportion of 0.40 throughout. What happens to the interval width as the sample size increases? Why? Ps = 0.40 Sample A: n = 100 Sample B: n = 1,000 Sample C: n = 10,000 6.15 PS Two individuals are running for mayor of your community. You conduct an election survey a week before the election and find that 51% of the respondents prefer candidate A. Can you predict a winner? Use the 99% level. (HINT: In a two-candidate race, what percentage of the vote does the winner need? Does the confidence interval indicate that candidate A has a sure margin of victory? Remember that while the population parameter is probably in the confidence interval ( α = 0.01 ), it may be anywhere in the interval.) Ps = 0.51 n = 578 SHOW ANSWER 6.16 SOC The World Values Survey is administered periodically to random samples from societies around the globe. Below are listed the number of respondents in each nation who said that they are “very happy.” Compute sample proportions and construct confidence interval estimates for each nation at the 95% level. Nation Number Sample Size Confidence “Very Interval Happy” United States 805 2,232 Japan 788 2,443 Brazil 523 1,486 Nigeria 977 1,759 China 361 2,300 Source: Data from World Values Survey Association. World Values Survey, Wave 5. 6.17 SOC The student clubs at Algebra University have been plagued by declining membership over the past several years and want to know if the incoming first-year students will be a fertile recruiting ground. Not having enough money to survey all 1,600 first-year students, they commission you to survey the interests of a random sample. You find that 35 of your 150 respondents are “extremely” interested in clubs. At the 95% level, what is your estimate of the number of first-year students who would be extremely interested? (HINT: The high and low values of your final confidence interval are proportions. How can proportions also be expressed as numbers?) SHOW ANSWER 6.18 Construct a confidence interval for each of the sample outcomes below at the 0.05 level. a. X = 34.60 ¯ σ = 12.30 n = 187 b. X = 68 ¯ σ = 25 n = 540 c. X = 164.80 ¯ σ = 83.20 n = 150 d. X = 76 ¯ s = 9.60 n = 100 e. Ps = 0.35 n = 112 f. Ps = 0.56 n = 456 6.19 SOC The results listed below are from a survey given to a random sample of the Canadian public. For each sample statistic, construct a confidence interval estimate of the population parameter at the 95% confidence level. The sample size (n) is 2,987 throughout. a. The average occupational prestige score was 43.87 , with a standard deviation of 13.52. SHOW ANSWER b. The respondents reported watching an average of 2.86 hours of television per day, with a standard deviation of 2.20. SHOW ANSWER c. The average number of children was 1.81 , with a standard deviation of 1.67. SHOW ANSWER d. Of the 2,987 respondents, 876 identified as Catholic. SHOW ANSWER e. Five hundred thirty-five of the respondents said that they had never married. SHOW ANSWER f. The proportion of respondents who said they voted for the Liberal Party of Canada in the 2019 federal election was 0.36. SHOW ANSWER g. When asked about capital punishment, 2,425 of the respondents said they opposed the death penalty for murder. 207 SHOW ANSWER You Are the Researcher Using SPSS to Produce Confidence Intervals with the 2018 CCHS The demonstrations and exercises below use the shortened version of the 2018 CCHS data. Start SPSS and open the CCHS_2018_Shortened.sav file. SPSS DEMONSTRATION 6.1 Using the Explore Command to Construct Confidence Intervals for Sample Means The Explore procedure can be used to construct confidence intervals for the sample mean. The Explore command produces many of the same summary statistics and graphical displays as the Frequencies and Descriptives procedures but offers additional features. Here we will use Explore to compute the confidence interval for the mean of smk_045 (number of cigarettes smoked per day for daily smokers). From the main menu, click Analyze, Descriptive Statistics, and Explore. The Explore dialog box will open. Use the cursor to find smk_045 in the list on the left, and click the right-arrow button to transfer the variable to the Dependent List box. SPSS by default uses the 95% confidence level, which in fact we want to use for this demonstration. To request another level, you can click on the Statistics button at the top of the Explore dialog box. The Explore: Statistics dialog box will open. Type the desired level (e.g., 90%, 95%, or 99%) in the textbox next to “Confidence Interval for Mean,” and click Continue. You will return to the Explore dialog box, where you might want to click the Statistics radio button within the Display section. Otherwise, SPSS will produce both summary statistics and plots (i.e., graphical displays). Finally, click OK, and the output below will be produced: The “Descriptives” output table contains a variety of statistics used to measure central tendency and dispersion. Some of these statistics are not covered in this textbook. If you wish to explore them, please use SPSS’s online Help facility. In this section, we focus on the parts of the table related to confidence intervals for the sample mean: the values for the “Lower Bound” and “Upper Bound” of the “ 95% Confidence Interval for Mean.” The lower limit of our interval estimate is 13.76 and the upper limit is 16.19. Another way to state the interval is 13.76 ≤ μ ≤ 16.19 208 We estimate that daily smokers, on average, smoke between 13.76 and 16.19 cigarettes per day. Because alpha was set at the 0.05 level, this estimate has a 5% chance of being wrong (i.e., of not containing the population mean for number of cigarettes smoked). SPSS DEMONSTRATION 6.2 The Error Bar Graph Here we’ll produce an error bar graph for the confidence interval for the mean number of cigarettes smoked per day for daily smokers (smk_045). Click 209 Graphs, Legacy Dialogs, and then Error Bar. The Error Bars dialog box will appear, with two choices for the type of graph we want. The Simple option is already highlighted, and this is the one we want. At the bottom of the Error Bars dialog box, click the “Summaries of separate variables” radio button within the Data in Chart Are box, and then click Define. The Define Simple Error Bar dialog box appears with variable names listed on the left. Use the cursor to find smk_045 in the list on the left, and click the right-arrow button to transfer the variable to the Error Bars box. In the Bars Represent box, you have the option to select whether the error bar represents the confidence interval of the mean, the standard error of the mean, or the standard deviation. Let’s choose “Confidence interval for mean,” the option that is already selected. You can also enter the desired confidence level in the Level box. We’ll use the 95% level, which is the default setting. Click OK, and the following error bar graph will be produced: The error bar illustrates the exact information given in the “Descriptives” output in Demonstration 6.1. The mean number of cigarettes smoked per day for daily smokers is 14.98 (marked by the small circle on the bar), and we estimate, at the 95% level of confidence (indicated by the length of the bar), that the mean number of cigarettes smoked for all daily smokers in Canada is somewhere between 13.76 (the small horizontal line at the bottom of the bar) and 16.19 (the small horizontal line at the top of the bar). SPSS DEMONSTRATION 6.3 Generating Statistical Information for Use in Constructing Confidence Intervals for Sample Proportions Not all statistical techniques covered in this text are performed directly by SPSS. Unlike the Explore procedure for constructing confidence intervals for the sample mean, SPSS does not provide a program specifically for constructing intervals for the sample proportion. However, we can use SPSS to calculate the sample statistics, namely proportions, on which the interval estimates are based. Once you know these sample statistics, you can easily compute the confidence interval for the sample proportion. To illustrate this process, we’ll construct a confidence interval for flu_005, “Have you ever had a seasonal flu shot, excluding the H1N1 flu shot, in your lifetime, yes or no?” Note that if we had selected a variable with more than two categories, such as marital status, we could estimate the population parameter for any or all of the categories of the variable. First, calculate the sample proportion using the Frequencies command. From the menu bar, click Analyze, Descriptive Statistics, and Frequencies. Find flu_005 in the left-hand box and move it to the Variable(s) box. Click OK. The output for flu_005 will look like this: Had a Seasonal Flu Shot (Excluding H1N1)—Lifetime Frequency Percent Valid Cumulative Percent Percent Valid Yes 1046 60.5 60.5 60.5 No 684 39.5 39.5 100.0 Total 1730 100.0 100.0 Second, substitute the values into Formula 6.4, remembering to change the percentages to proportions. Thus, using the 95% confidence level, we have Pu(1−Pu) c.i. = Ps ± Z √ n 0.605(0.395) = 0.605 ± 1.96√ 1,730 0.24 = 0.605 ± 1.96√ 1,730 = 0.605 ± (1.96)(0.012) = 0.605 ± 0.02 Changing back to percentages, we can estimate that between 58.5% (60.5% − 2%) and 62.5% (60.5% + 2%) of Canadians have had the seasonal flu shot (excluding H1N1) at least once in their lifetime. 210 Exercises (using CCHS_2018_Shortened.sav) 6.1 Use the Explore command to get the 95% confidence interval for hwtdghtm and hwtdgwtk. Express the confidence intervals in words,