Object Recognition & Cognition PDF
Document Details
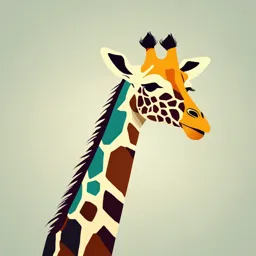
Uploaded by BallerGiraffe0118
Concordia University
Tags
Summary
This document discusses object recognition, exploring processes such as matching representations to stored memories and categorization. It examines theories like template matching and feature detection, along with experimental findings on speed and accuracy of recognition. Topics covered include the role of features, attention, and the impact of visual noise.
Full Transcript
Object Recognition Recognition: process of matching the representation of a stimulus to a representation stored in long-term memory about 100ms Occurs under noisy conditions (partial occlusion, low luminance, different shapes) Viewpoint invariance: ability to recognize an object from any view...
Object Recognition Recognition: process of matching the representation of a stimulus to a representation stored in long-term memory about 100ms Occurs under noisy conditions (partial occlusion, low luminance, different shapes) Viewpoint invariance: ability to recognize an object from any view point Categorization: deciding whether or not an object belongs to a given category (e.g., cake, food, etc.) about 150ms Different object recognition processes that depend on the category level Entry-level category: label that first comes to mind Subordinate-level category: more specific term Superordinate-level category: more general term for the object Natural Object Categorization Natural Object Categorization (experiment) Subjects (n=16) perform a categorization tasks (y/n) ERPs are recorded (32 electrodes) 480 images for 20ms presentation No mask presented, after-image effects Results Early peak at 75ms (recognition) Max peak at 100ms Late peak at 150ms (categorization) Speed of Scene Recognition (experiment) Categorization of scenes Fixation of masks 300-900ms Images presented for 26ms Masks for 1000ms Results: Scene categorization is fast and accurate under short presentations Natural scenes are categorized faster than “artificial” scenes Speed of visual processes: other estimates (experiment) Can accurate detect a picture within 167ms Basic-level categorization takes about 120ms Depends on kind of objects (natural kind is faster) Detection of shapes “Artificial” objects take longer to label Object Recognition Theories A Theory to Discard: Template matching Templates require dedicated mechanisms that are specific to a given input Naïve template theory: visual system recognizes objects by matching the neural representation of the image with a store representation of the same "shape" in the brain Problem: We would need a different template for every size, orientation, and style of the same “thing” i.e. infinite amount of templates We do not have the recognition capacity nor the memory capacity for templates Feature Detection Theories Goal: To build 3-D representations from 2-D retinotopic representation: Detecting edges and surface discontinuities Perception relies on feature detection/discrimination Texture, colour, patterns, Attention grabbers features: processed and combined automatically from the input representation Visual Search Tasks Subjects are given sets of displays, with variations in each display Target hidden within distractors Respond Yes if a given target element is present & No if absent Measure RT to detect target in relation to the amount of distractors Results: If RT is the same = pop out effect Search is parallel: Odd element pops out We can detect about 4 objects in parallel If RT increases as the amount of distractors = no pop out effect Search is serial: search time depends on display size Singletons: single feature easy to detect Conjunction features take longer to find **Treisman’s Feature Integration Model Emphasis on features in early vision Specialized modules compute different types of information (colour, orientation, size, etc.) Attention spotlight integrates features Features maps activate object properties, relations, even names (what & where) Also activate recognition network: stored descriptions of objects Object files (& concepts) are access by the activation and integration of features Conscious perception depends on object files Step 1: Pre-attentive stage objects are analyzed into separate features (colour, shape, movement) Occurs very early (even before we are conscious of the objects) Evidence: illusory conjunctions (combinations of features from different stimuli) because at the beginning of the perceptual process each feature exists independently of the others Step 2: Focused-attention stage Combination of features Perception of the objects Evidence: patient R.M., a patient who had parietal lobe damage= Balint’s syndrome (inability to focus attention on individual objects) lack of focused attention makes it difficult to combine features correctly Treisman’s model: co-activation of features to account for objects Connectivistic architecture Shape Detection Theories Objects are matched to sets of 3-D components that represent object parts Objects are recognized by stored (“memory”) representations / parameters for transformation Knowledge of objects implies knowledge of their parts Part of objects are shape We may have a catalog of shapes According to Marr: cylinder is the basic shape Concave creases identify boundaries between parts Transversality regularity: when any 2 surfaces “penetrate” each other at random, they always meet at a concave discontinuity **Biederman’s Recognition by Component Theory Recognition by component (RBC): recognition of objects by the identities and relationships of their component parts Bottom up processing Finite set of geometric icons (geons) create infinite possibilities of objects Representations: the geometrical elements (Biederman) Processes: the combination of objects Geons are defined by non-accidental properties: if something is curved in your view point, then it is curved in real 3D space Perceive objects with viewpoint invariance 5 invariant properties of edges 1. Curvature: points on a curve 2. Parallelism: sets on points in parallel 3. Cotermination: edges terminating at a common point 4. Symmetry: contrast with asymmetry 5. Collinearity: points sharing a common line BIEDERMAN’S STAGES OF OBJECT PROCESSING Edge extraction: Basic perceptual mechanism for detection of regions of contrast where there are sharp changes in luminance or texture Detection of Non-accidental Properties & Parsing at Concave Regions: Mechanism for determining properties of lines that mark the regions (and surface discontinuities) their orientation in 3-D space and geometric properties (e.g., curvilinearity, parallelism) Also, concave crests are marked for determination of parts Determination of components: Each segmented region (based on non-accidental properties and concave crests) is matched to a geon Components are arranged and matched to an object representation: Mechanism in which sets of activated geons are arranged to match object representations Object is identified: The activated representations allow for the object to be recognized Empirical evidence for Biederman’s theory: Concavity: objects recognized faster when concave creases are preserved ADVANTAGES: Good evidence for geons being important in object recognition Evidence that the identification of concavities and edges is also of major importance Many principles have stood the test of time LIMITATIONS/QUESTIONS: De-emphasises importance of top-down influences from context, expectations, and previous knowledge Fails to account for most within-category discriminations Much recognition is actually viewpoint-dependent But some view points have no viewable geons Some classes of objects do not have invariant geons yet are still recognizable as members of a category (e.g., clouds, ocean) Some objects dont have a shape, rather are a mass Biederman’s model: geons are symbolic of parts of objects Symbolic architecture