Wireless Communications I Lecture 3 Notes Fall 2024 PDF
Document Details
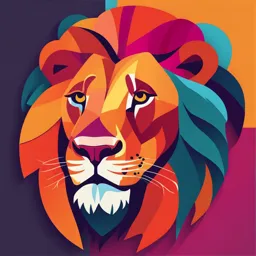
Uploaded by MagicHawk3023
Jacobs University Bremen
2024
Tags
Summary
Wireless Communications I Lecture 3 notes from Fall 2024. This lecture introduces the concept of slotted ALOHA and its limitations. Examines backlogged analysis and stability in detail. Includes key formulas and figures demonstrating stability conditions.
Full Transcript
Wireless Communications I - Fall 2024 - Lecture 3 In the last Lecture Slotted ALOHA: Slotting doubles the potential throughput. Limitations of asymptotic and non-backlogged...
Wireless Communications I - Fall 2024 - Lecture 3 In the last Lecture Slotted ALOHA: Slotting doubles the potential throughput. Limitations of asymptotic and non-backlogged analysis. Backlogged analysis via Queueing Theory. Finite analysis: throughput is still Poisson but not stationary. Stability: not guaranteed. Aggregate Throughput and Drift of Slotted ALOHA 0.4 Aggregate Throughput 0.35 Drif+Throughput 0.3 Throughput: Ps 0.25 0.2 Desirable Stability Point Undesirable Stability Point 0.15 0.1 Unstable Equilibrium Point 0.05 0 0 1 2 3 4 5 6 7 8 9 10 11 12 13 14 15 16 17 18 19 20 21 22 23 24 25 Load: k Figure 1: Plot illustrating the stability conditions for slotted Aloha. The results are calculated with the parameters: N = 25, pe = 0.015 and pb = 0.2. 1 Stabilized Slotted ALOHA: Preliminary Concepts Before we discuss stabilized variations of the Aloha protocol, let us think about what stability really means in the context of a multiple access control (MAC) mechanism. We saw that under the assumption that nodes have buffers to backlog unsuccessful packets, slotted Aloha may drift into situations where either: The arrival of new packets cannot be sustained by the queue, leading to further delays, which in turn causes the queue to grow (positive drift); The queue can (in average) serve more packets than those arriving, leading to a reduction in the queue length (negative drift). Equilibrium: average demand = average throughput. Clearly, equilibrium is a necessary but not sufficient component of sta- bility. What defines a stable system is the property that it tends to equi- librium regardless of any given state. Stability: average demand ←→ average throughput. ∀B Stable Scheme 1: No-buffering A trivial scheme that satisfies the above definition of stability is one in which nodes have no buffer and therefore discard all arrivals until the current packet is successfully transmitted. This “solution” is obviously not acceptable as it suppresses the demand, instead of satisfying it. The extreme opposite of the no-buffer variation is one where nodes have infinite buffers. This is, however, insufficient to ensure stability as seen before. 2 Stable Scheme 2: Exact Rate Adaption Another option is to vary dynamically the transmission rate pb. In particular, from Ps ≈ Rk · e−Rk =⇒ Rk∗ = 1 (1) and from 1 + (k − N ) · pe Rk , (N − k) · pe + k · pb =⇒ pb = (2) k Unfortunately this “solution” is not practical since the state of the queue k is unknown to the users! Motivated by the problems of trivial approaches illustrated above, let us introduce a more tangible definition of stability for slotted Aloha protocols. Stability: S < ∞, ∀ N < ∞. Stabilized Slotted ALOHA: Feasible Solutions Let us now discuss a few feasible alternatives to stabilize the slotted Aloha scheme. Stable Scheme 3: Equal Rate Allocation A simple and practical (if empirical) form of stabilized slotted Aloha could be designed inspired on equation (2), under the assumptions that: a) The number of users in the queue B is a random variable following a Poisson distribution1 ; B̄ k −B̄ P (k; B̄) = e (3) k! b) The expected number of users B̄ = E[B] can be estimated. Specifically, consider the case where the retransmission probability pb is set to be inversely proportional to the average queue length, that is 1 pb = (4) B̄ 1 Recall that in a slotted Aloha system with a fixed transmission probability pb , the distribution of the number of nodes added to the queue, given a state B is given by a geometric distribution ! B Pb (i; B) = (1 − pb )B−i pib. i Recall also that the geometric distribution tends asymptotically to a Poisson model. 3 – Probability of Idleness With such a scheme, the system is idle if either all buffers are empty, that is, the system has no packets (k = 0); or there are packets in buffers but no node decides to transmit. Thus, ∞ 1 k X P∅ = P (0; B̄) + P (k; B̄) · 1 − B̄ k=1 | {z } All k nodes coincidentally and independently decide not to transmit ∞ −B̄ −B̄ X (B̄ − 1)k =e +e k! k=1 ∞ ! X (B̄ − 1)k = e−B̄ 1+ k! k=1 ∞ X (B̄ − 1)k 1 = e−B̄ = ≈ 0.368. k! e |k=0 {z } eB̄−1 – Probability of Success In turn, the probability of success is given by while all the There are k packets others do not, in the system, z }| { ∞ z }| { k−1 X 1 1 k Ps = P (k; B̄) · · 1− · , B̄ B̄ 1 k=1 |{z} | {z } of which a single given all possible is transmitted, choices of 1 out of k. ∞ B̄ k e−B̄ 1 k−1 X 1 = · · 1− · k k! B̄ B̄ k=1 ∞ −B̄ X (B̄ − 1)k−1 1 =e = ≈ 0.368. (k − 1)! e |k=1 {z } eB̄ −1 – Probability of Collision Finally, from the results above it is evident that the probability of collision is 2 Ps̃ = 1 − ≈ 0.264 (5) e Notice that this scheme is implicitly stable since it achieves the maximum throughput Ps = 1e. But unfortunately this is not truly a valid solution, due to the assumption that B̄ (and implicitly N ) are known. 4 Stable Scheme 4: Pseudo-Bayesian Algorithm Despite the limiting impractical assumption that B̄ is known, the latter scheme offers a valuable insight. Namely, it implies that stability can be reached if both new arrivals and backlogged packets be treated the same way. The Pseudo-Bayesian Algorithm exploits this idea, while replacing the assumption of known B̄ by a practical method to estimate the state of the queue. In the Pseudo-Bayesian Algorithm, new arrivals are buffered and treated as backlogged packets, transmitted with the probability p. In other words, pe = 0 and pb = p. In the Pseudo-Bayesian Algorithm, the status of the queue is estimated by all transmitters based on the occurrence or ACKs or lack of the latter. – Attempt Rate If there are k packets in the queue (including new arrivals), then the attempted rate is Rk = k · p (6) – Conditional Probability of Success Consequently, the probability of success conditioned on the as- sumption that k packets exist is while all the others are not, z }| { k Ps|k = p · (1 − p)k−1· = kp(1 − p)k−1 (7) |{z} 1 A single packet | {z } is transmitted, given all possible choices of 1 out of k. Strategy: estimate k and adapt p accordingly. – Adaptation Let us first assume that an estimate k̂i of the queue length at the beginning of the i-th slot is available. In possession of that in- formation the Pseudo-Bayesian Algorithm is such that each node transmits a packet with probability n o p = min 1, 1 (8) k̂i 5 – Posterior Queue Length Distribution We have seen in the analysis of the Equal Rate Allocation scheme that if both new and backlogged packets are transmitted with the same probability, the distribution of the queue length is Poisson. Assume that at the i-th slot, no transmissions occurred (idle) or a single packet was transmitted (success). The corresponding posterior distributions can then be derived as follows. Starting from the idle case first, consider the Bayes rule P∅|k Pk Pk|∅ =. (9) P∅ But k 1 P∅|k = 1− , (10) k̂i and k̂ik −k̂i Pk = P (k; k̂i ) = ·e , (11) k! such that k 1− 1 k̂ik e−k̂i (k̂i − 1)k −(k̂i −1) k̂i Pk|∅ = 1 = ·e , (12) e k! k! which is Poisson with mean (k̂i − 1). Remember from above that P∅ = 1e. Next, consider the case when a successful transmission occurs. Then, referring to equations (7) and (11) we have 1 k k̂ik+1 · e−k̂i 1 (k + 1) · 1− · Ps|(k+1) Pk+1 k̂i k̂i (k + 1)! P(k+1)|s = = −1 Ps e (k̂i − 1)k −(k̂i −1) = ·e , (13) k! which is identical to equation (12). From equations (12) and (13) we conclude that the expected (or most likely) queue length after either the event k −→ k (idle), or k + 1 −→ k (success) is observed, is given by k̂i − 1. Remember from above that Ps = 1e. 6 Adaptation Rule # 1: Reduce the estimate k̂i by one, if idle or success. Think about this result. Why does it make sense? Homework 5: Justify mathematically why in the presence of a collision we must increase the estimate k̂i by (e − 2)−1. Hint: Consider that if the estimation is accurate, the average estimation error must be zero. Adaptation Rule # 2: Increase the estimate k̂i by (e − 2)−1 , if collision. Notice that the first rule is Bayesian, while the second is not. This justifies the name of the algorithm. – Estimation Considering the two rules derived above, and taking into account also new arrivals at the rate λ, the final queue length estimation scheme becomes ( max{λ, k̂i + λ − 1} if no collision occurred, k̂i+1 = (14) k̂i + λ + (e − 2)−1 if collision did occur. The arrival rate λ may be unknown. But setting λ̂ = 1e ensures stability for all λ < 1e. Contention Resolution All variations of the Aloha scheme discussed above have in common the fact that the impact of a collision on the behavior of nodes in only statistical. Specifically, only adjustments on the transmission probabilities is made. In other words, in all schemes studied so far are purely random and in them, packet collisions are resolved. “The second worst enemy of freedom is total efficiency; the worst is total anarchy.” Aldus Huxley (1894 - 1963) A way to mitigate these problems is to introduce a limited amount of de- terminism into the scheme, by inserting policies to resolve collisions. Splitting Tree Algorithms 7 Access Rules: - If no collision all nodes (with packets) attempt transmission; - Blocked access variation: only colliding nodes can transmit; - Free variation: new arrivals act as other nodes. Splitting Rule: In the presence of a collision, each node flips a “Q-ary coin” and transmits surely at q-th subsequent slot. Standard Blocked STA In the standard blocked STA, nodes will transmit immediately when- ever no collision was observed; new arrivals are blocked in the presence of a collision; and nodes involved in a collision split in Q groups. – Contention Resolution Interval (CRI) Analysis CRI: number of slots required for the server (or “sink”) detect that there is no contention. Referring to Figure 2 shown below, let: ∗ The number of nodes to be served (i.e. in collision) be denoted by N ∗ The number of split slots be denoted Q ∗ The number of nodes that go to the q-th split slot be denoted Iq. Figure 2: Illustration of the Q-ary splitting behavior in the standard STA with blocked access. 8 Next, let YN denote the instantaneous CRI associated with N backlogged nodes (or packets). We are interested in the distri- bution of YN , so that we can calculate its average LN , E[YN ]. ∗ If N = 0 (empty queue), we have obviously Y0 = 1 ⇒ L0 = 1, since the server can immediately recognize that no packets are transmitted. ∗ If N = 1 (single client), we also have Y1 = 1 ⇒ L1 = 1, since the server can immediately recognize that the packet is transmitted without collision. ∗ If N > 2, however, the CRI depends on future splits, which is captured by the relation: Time to resolve IQ packets z}|{ YN = YI1 + · · · + YIQ + 1 ← Slot after all contentions are resolved |{z} Time to resolve I1 packets Therefore, the CRI of the standard blocked STA is governed by the recurrence Q X YN −→ 1 + YIq (15) q=1 The problem with the relation (15) is that YIq ’s are correlated random variables, which makes the direct derivation of the distribution of the sum very hard to attain. In order to mitigate this problem consider the following tool. Probability Generating Function: The PGF of a discrete random variable X ∈ N is defined as: ∞ X X GX (z) , E[z ] = p(k)z k (16) k=0 The probability of the outcome X = k, is recovered from G(z) by: 1 dk G(z) p(k) = Pr{X = k} = · , (17) k! dz k z=0 And the expectation (first moment) of X can be computed from: ∞ X dG(z) E[X] = k · p(k) = , (18) dz z=1 k=0 The Probability Generating Function has the following important property, which we shall exploit. 9 PGF of Sum = Product of PGF: Let XN be a sum process, XN = N P i=0 xk. The PGF of XN is: N Y GX (z; N ) = GX (z; k) (19) i=0 Note: This holds exactly for uncorrelated xk ’s, but also approxi- mately (very well) even with correlation amongst xk ’s. Homework 6: Derive expressions for the mean and the variance of the binomial distribution (show below). N k PN (k) , p (1 − p)N −k , k ∈ {0, · · · , N } (20) k Hint: Use the Binomial PGF and its derivatives. From equation (15), it follows that the PGF of the random vari- able YN is given by N X N Y k GY (z; N ) = Pr{YN = k}z = GY (z; k) k=0 i=0 YN =z GY (z; k) (21) i=1 Notice that an immediate consequence of equation (21), under the initial conditions Y0 = Y1 = 1 is that GY (z; 0) = GY (z; 1) = z. (22) If nodes split independently using the same coin, so that each chooses to transmit next on the q − th slot with probability pq , equation (21) yields the following recursive relation N Q Y X N I GY (z; N ) = z pqq GY (z; Iq ) (23) I1 · · · IQ I1 ···IQ q=1 PN where denotes a sum over all possible combinations of I1 ···IQ N P I1 · · · IQ such that Iq = 1, and I1 ···I Q is the multinomial co- efficient. It is hard to obtain a closed-form expression of the Probability Mass Function of YN from the later equation, but plots can be obtained and shown to agree with simulations 10 STA-based Relay Selection Strategy 50 N=2, Analysis N=2, Simulation N=3, Analysis N=3, Simulation 40 N=4, Analysis N=4, Simulation PMF of CRI (%) 30 20 10 0 3 5 7 9 11 13 15 17 19 21 CRI (slots) Figure 3: Distributions of YN , for a few values of N. The expected CRI is given by " N # dGY (z; N ) d Y LN = = z GY (z; k) (24) dz z=1 dz k=1 z=1 which for GY (z; N ) as in equation (23) reduces to Q X N X N k LN =1+ p (1 − pq )N −k Lk (25) k q q=1 k=0 The later recursive relation can be shown to yield the following closed-form solutions N X N (k − 1) LN = 1+Q (−1)k Q (26) k k=2 pkq P 1− q=1 ∞ X = 1+Q Qn [1−(1−Q−n )N −N Q−n (1−Q−n )N −1 ] (27) n=0 We will omit the details, but using these results it can be shown that the throughput of a network with multiple access based on the (standard) Splitting Tree Algorithm is N 1 ρ=E = 0.366204 < ≈ 0.3679 (28) LN Q=3 e The maximum average throughput of the Standard STA is inferior to that of stabilized slotted Aloha. 11 – Variations of the STA Variations of the standard splitting tree algorithm arise in the two following ways: a) Modified: If all nodes choose to transmit on the Q-th slot, they will discover that fact by observing that the next Q−1 slots are idle. In this case, they may skip the sure collision that would result if they transmitted on the Q slot and in- stead split again; b) Unblocked: New arrivals may not be blocked, but rather incorporated into the stack by flipping the same coin used by nodes under contention. (a) Modified, blocked (b) Unmodified, unblocked Figure 4: Illustration of splitting behavior of variations of Q-ary splitting tree algorithms with different access rules. Modified Blocked STA In the modified case, the behavior of the queue is such that the system may essentially reset to the state N , after Q − 1 slots have been idle, except that one slot is saved by the modification. Taking into account that this event occurs when all the N nodes choose to transmit at the Q-th slot, which happens with probability pN Q , the following recurrence relation results PGF: z Q−1 PGF: (z−1)GY (z;N ) z }| { z }| { YN −→ 1 + (Q − 1)Y0 + (YN − 1) (29) | {z } with probability pN Q This leads to the following CRI PGF N Q Y X N I GY (z; N ) = z pqq GY (z; Iq ) − pN Qz Q−1 (z − 1)GY (z; N ) I1 · · · IQ I1 ···IQ q=1 (30) 12 and correspondingly, the following closed-form average CRI ∞ X LN = 1+Q Qn [1−(1−Q−n )N −N Q−n (1−Q−n )N −1 ] (31) n=0 ∞ X Qn [1− Q−n (1−Q−1 )]N −(1−Q−n )N − N Q−n−1 (1 − Q−n )N −1 − n=0 From this result one finally obtains N 1 ρ=E = 0.3753 > ≈ 0.3679 (32) LN Q=3 e The maximum average throughput of the Modified STA is superior to that of stabilized slotted Aloha. Homework 7: Write a Matlab program to implement both the standard and modified splitting tree algorithms. What values of the average CRI you find for N = 2, 3, · · · ? Unblocked STA: Standard and Modified In the unblocked case, new arrivals are added to the stack under the same splitting probabilities. Referring to figure 4(b) the following recursion results XQ YN −→ 1 + YIq +Xq , (33) q=1 where Xq are Poisson random variables representing the new packets added to the q-th slot of the stack. For details see , but from equation (33) it can be shown that the maximum throughput of a network with multiple access via the stan- dard Splitting Tree Algorithm with free-access (unblocked) is N 1 ρ=E = 0.4016 > ≈ 0.3679 (34) LN Q=3 e Likewise the modified STA algorithm with free access, represented by the splitting behavior depicted in figure 5, is governed by a recursive relation obtained via the combination of equations (29) and (33), which in turn yields the maximum throughput N 1 ρ=E = 0.4069 > ≈ 0.3679 (35) LN Q=3 e Unblocked STA, both standard and modified outperforms both stabilized slotted Aloha and blocked forms of STA. 13 Figure 5: Illustration of the Q-ary splitting behavior in the modified STA with free access. Recommended Reading - Bertsekas and Gallager : Chapter 4 (Section 4.2 ∼ 4.4.3) - Article by Mathys and Flajolet - Online: http://www.youtube.com/watch?v=T1Q1v5wLJNo http://www.youtube.com/watch?v=-nGfQ9ewNSc References P. Mathys and P. Flanjolet, “Q-ary collision resolution algorithms in random-access systems with free or blocked channel access,” IEEE Trans. Inform. Theory, vol. 31, no. 2, 1985. D. Bertsekas and R. Gallager, Data Networks. Prentice Hall, 1992. 14