Machine-Learning-Guided Peptide Drug Discovery: Development of GLP-1 Receptor Agonists PDF
Document Details
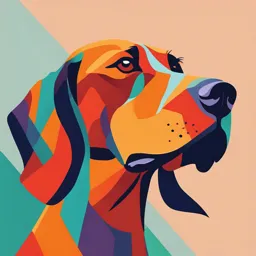
Uploaded by FavoriteBinary
Jens Christian Nielsen, Claudia Hjo̷rringgaard, Mads Mo̷rup Nygaard, Anita Wester, Lisbeth Elster, Trine Porsgaard, Randi Bonke Mikkelsen, Silas Rasmussen, Andreas Nygaard Madsen, Morten Schlein, Niels Vrang, Kristoffer Rigbolt, and Louise S. Dalbo̷ge
Tags
Related
- Machine Learning 1_ classification methods - lectures-1.pdf
- Lecture 6: Machine Learning for Remote Sensing Image Processing - Part I PDF
- Machine learning.pdf
- Machine Learning Overview PDF
- Machine Learning System Design Performance Measurement Lecture
- Lecture12_Machine learning system design.pptx.pdf
Summary
This article details a machine learning-guided approach to peptide drug discovery, specifically focusing on developing improved GLP-1 receptor agonists. The researchers employed a novel platform called streaMLine to synthesize and screen a large peptide library, leveraging quantitative structure-activity relationship (QSAR) models. The study yielded promising results, identifying a candidate agonist with improved properties.
Full Transcript
This article is licensed under CC-BY-NC-ND 4.0...
This article is licensed under CC-BY-NC-ND 4.0 pubs.acs.org/jmc Article Machine-Learning-Guided Peptide Drug Discovery: Development of GLP‑1 Receptor Agonists with Improved Drug Properties Jens Christian Nielsen, Claudia Hjo̷ rringgaard, Mads Mo̷ rup Nygaard, Anita Wester, Lisbeth Elster, Trine Porsgaard, Randi Bonke Mikkelsen, Silas Rasmussen, Andreas Nygaard Madsen, Morten Schlein, Niels Vrang, Kristoffer Rigbolt, and Louise S. Dalbo̷ ge* Cite This: https://doi.org/10.1021/acs.jmedchem.4c00417 Read Online See https://pubs.acs.org/sharingguidelines for options on how to legitimately share published articles. ACCESS Metrics & More Article Recommendations * sı Supporting Information Downloaded via 122.171.17.175 on July 13, 2024 at 04:46:43 (UTC). ABSTRACT: Peptide-based drug discovery has surged with the development of peptide hormone-derived analogs for the treatment of diabetes and obesity. Machine learning (ML)-enabled quantitative structure−activity relationship (QSAR) approaches have shown great promise in small molecule drug discovery but have been less successful in peptide drug discovery due to limited data availability. We have developed a peptide drug discovery platform called streaMLine, enabling rigorous design, synthesis, screening, and ML-driven analysis of large peptide libraries. Using streaMLine, this study systematically explored secretin as a peptide backbone to generate potent, selective, and long-acting GLP-1R agonists with improved physicochemical properties. We synthesized and screened a total of 2688 peptides and applied ML-guided QSAR to identify multiple options for designing stable and potent GLP-1R agonists. One candidate, GUB021794, was profiled in vivo (S.C., 10 nmol/kg QD) and showed potent body weight loss in diet- induced obese mice and a half-life compatible with once-weekly dosing. INTRODUCTION Peptide-based therapeutics are gaining increasing attention in elute chemical motifs necessary for binding.4 For peptide drug discovery, however, data are often sparse, and this has limited the pharmaceutical industry. Peptide hormones have both high the use of machine learning (ML) for QSAR optimization. The receptor potency and selectivity, minimizing off-target effects amount and composition of data are crucial parameters for and generally translating into an excellent drug safety and QSAR methods,5 which is why it is advantageous to generate efficacy profile.1,2 These features make endogenous peptides a data that are specifically designed for modeling purposes. good starting point for the development of novel peptide Glucagon-like peptide-1 (GLP-1) is an endogenous 30- therapeutics. However, native unmodified peptides are rarely amino acid peptide hormone produced by enteroendocrine L- used as drugs because of their inherent limitations due to their cells and secreted into the hepatic portal in response to food very short systemic half-life and unfavorable physicochemical intake. By activating GLP-1 receptors in the pancreas, native properties which must be circumvented to develop peptide GLP-1 serves as an incretin hormone stimulating insulin molecules suitable for therapeutic use.1,2 release and inhibiting glucagon secretion.6 In addition, GLP-1 Early drug discovery phases aim to improve the properties of is an important appetite regulator by activating central GLP-1 candidate molecules by modifying their chemical structure. receptors (GLP-1R).7 In line with this, long-acting GLP-1R Such improvements can be achieved by rational design using an iterative and often laborious approach, where small batches of compounds are screened in multiple rounds of optimization. Received: February 19, 2024 In contrast, when larger data sets are available, it is useful to Revised: June 19, 2024 construct mathematical models that capture the quantitative Accepted: June 20, 2024 structure−activity relationship (QSAR) to guide drug design. Published: July 8, 2024 QSAR models have been widely used in the development of small molecule therapeutics e.g., to discover novel binders3 and © 2024 The Authors. Published by American Chemical Society https://doi.org/10.1021/acs.jmedchem.4c00417 A J. Med. Chem. XXXX, XXX, XXX−XXX Journal of Medicinal Chemistry pubs.acs.org/jmc Article Table 1. Alignment of GLP-1, Secretin, Dual-Agonist, and GLP-1R Selective Agonist GUB021794a a Origin of substitutions are highlighted in colors. * denotes attachment of half-life extender: C20DA-gGlu-2xOEG. Figure 1. Overview of the data generation and data analysis workflow of the streaMLine platform. Initially a systematic library of peptides is designed, typically on the order of hundreds to thousands of peptides. The crude library of peptides are prepared using solid-phase-peptide synthesis (SPPS) and cleaved from the resin. Failed peptide samples are identified by high-resolution mass spectrometry and excluded from the analysis. A panel of high-throughput assays for determining receptor potency and physicochemical properties at different pH levels are measured. For each assay end point, a random forest model is trained and used for inferring key amino acids substitutions that determine peptide properties. agonists such as semaglutide have been shown to be important span two key receptor domains. The C-terminal region of the tools in the treatment of diabetes and obesity.8,9 However, peptides binds to the extracellular domain of their respective GLP-1 is known to self-assemble into amyloid fibrils and the receptor whereafter the N-terminal region interacts with the intrinsic physical instability of GLP-1 poses a significant core domain of the receptor enabling receptor activation.20 challenge in synthesis and formulation.10,11 The sequence identity of secretin and GLP-1 is shown in Table Recent drug development efforts have taken advantage of 1. the varying degrees of sequence homology of GLP-1, glucagon, Contrary to other peptides of the glucagon family, such as and glucose-dependent insulinotropic polypeptide (GIP) to GLP-1, secretin is not reported to aggregate.21 Thus, secretin engineer unimolecular dual or triple agonists targeting could serve as the starting backbone with improved receptors of GLP-1, glucagon, and GIP. This approach has physicochemical properties compared to GLP-1. proven to be a successful concept for treatment intervention in The main physiological role of secretin is to regulate water diabetes and obesity.12−17 homeostasis and bicarbonate secretion from the exocrine Alternatively, one could further envision to exploit sequence pancreas and inhibit gastric acid secretion by activating the homology to obtain beneficial peptide properties not only in secretin receptor (SCTR),19 i.e., endogenous activities we terms of receptor pharmacology, but also from a synthesis and/ intended not to activate. or formulation perspective.18 Hence, we aimed to leverage the more favorable Secretin is a 27-amino acid peptide hormone that together physicochemical properties of secretin to develop a selective with GLP-1 belongs to the glucagon superfamily of structurally and physicochemical stable GLP-1R agonist based on the related peptide hormones all targeting family B G-protein secretin backbone. With this aim, we exploited an innovative coupled receptors (GPCRs).19 This family of peptides is linear ML-based peptide drug discovery platform termed streaMLine peptides comprising 25 or more residues allowing them to and demonstrated how streaMLine effectively facilitates B https://doi.org/10.1021/acs.jmedchem.4c00417 J. Med. Chem. XXXX, XXX, XXX−XXX Journal of Medicinal Chemistry pubs.acs.org/jmc Article Figure 2. Schematic representation of the parallelized development process for generating a selective and stable GLP-1R agonist based on the secretin backbone. The two-step process for converting secretin into a preclinical drug candidate. First, a minimal GLP-1R agonist was developed by introducing only necessary GLP-1 residues to provide activation of GLP-1R. Second, a parallelized workflow was initiated where a deep mutational scan, a glutamate scan, and a lipidation scan provided a blueprint for generating various soluble, physically stable, and half-life extended GLP-1R agonist. Figure 3. Overview of substitution-effects from a GLP-1 dial-in scan in the secretin backbone. (A) Effect of introducing GLP-1 residues into the secretin backbone. For each assay end point, a random forest model was trained on 768 peptides and used to compute SHAP values determining the level of contribution of each amino acid substitution. Delta mean SHAP values denote the contribution of substituting the secretin residue with the corresponding GLP-1 residue. (B) Detailed overview of SHAP values for selected positions, where substitutions were introduced to obtain analogs with dual GLP-1R and SCTR potency. Small points denote SHAP values per individual peptide and large points denote mean SHAP value. accelerated development of novel peptide-based therapeutics RESULTS The streaMLine Platform. The streamline platform is a by rigorous design and ML-driven analysis of large peptide drug development tool where peptide libraries are designed, synthesized, and screened to provide large data sets suitable for libraries. machine learning (ML)-enabled quantitative structure−activity C https://doi.org/10.1021/acs.jmedchem.4c00417 J. Med. Chem. XXXX, XXX, XXX−XXX Journal of Medicinal Chemistry pubs.acs.org/jmc Article Figure 4. Overview of substitution-effects from a deep mutational scan (DMS) in a secretin derived GLP-1R and SCTR dual agonist. (A) Effect of single mutations in all positions. For GLP-1R and SCTR potency, random forest models were trained on 1152 peptides encoded using z-scales.23 From the models, the effect of single mutations was computed to normalize for assay batch effects. (B) Detailed overview of selected positions highlighting the effect of individual substitutions. relationship (QSAR) approaches. An overview of the learning (HRMS) to determine purity. The average purity per library cycles in the streamline platform is illustrated in Figure 1. ranges between 30 and 50%, and peptide samples with less In the streaMLine platform, peptides are synthesized using than 10% purity are excluded from further analysis. solid-phase peptide synthesis (SPPS) in a plate format. The The peptide libraries are designed in a highly systematic crude peptide libraries are screened directly in functional manner, where each substitution is observed multiple times in potency assays and in preformulation assays for determination combination with other substitutions. Typically, peptide of, for example, fibrillation and solubility. Each peptide library libraries consist of hundreds to thousands of peptides, which is analyzed by using high-resolution mass spectrometry enables robust evaluation of each substitution in multiple D https://doi.org/10.1021/acs.jmedchem.4c00417 J. Med. Chem. XXXX, XXX, XXX−XXX Journal of Medicinal Chemistry pubs.acs.org/jmc Article chemical contexts, i.e., different backbones. The peptide and 22F increased GLP-1R potency. Likewise, 2A was found to sequences together with assay data are used as training data increase the GLP-1R potency. The substitution of alanine for to construct random forest models22 describing the relation- 2-aminoisobutyric acid (Aib) is well-known to prevent DPP-4 ship between peptide sequence and assay end point. In the proteolytic cleavage of the GLP-1 backbone without training data, the systematic peptide library is encoded using compromising GLP-1R potency9,25 hence 2Aib was intro- amino acid descriptors (z-scales23 or one-hot encoding), and duced. The 3E mutation prevented the isomerization of the potential laboratory batch effects are incorporated in the model native aspartic acid residue in secretin without influencing to normalize, e.g., synthesis and assay plate differences. For receptor potency. Next, a comprehensive sequence exploration each assay end point, a new model is trained and used for was performed on the dual GLP-1R-SCTR agonist, i.e., a deep inferring the key amino acid substitutions affecting the end mutational scan. point. Model inference is done either by (1) correcting assay Development of Selective GLP-1R Agonists. A deep data for batch effects and amino acid similarity and thereby mutational scan (DMS) was designed such that all-natural computing normalized assay measurements for individual amino acids (except cysteine and methionine) were introduced peptides, (2) or by computing Shapley Additive explanation in all sequence positions, either as single mutations or as (SHAP) values.24 SHAP values are used to explain the effect of double mutations. The library consisted of 1152 peptides, each amino acid substitution on the end point and can thus be which were screened for GLP-1R and SCTR potency. Based used to infer the key drivers in the data set. After model on these data, we trained random forest models on the inference, the most promising substitutions are assessed in a relationship between all assay end points and the peptide new peptide library design. amino acid sequence. The models were used to normalize for Data points obtained on crude peptides are challenging to batch effects (synthesis and assay plate) and the resulting interpret individually, but in the context of the systematically pEC50 values for each single mutant are shown in Figure 4. designed peptide libraries, the random forest model provides The DMS identified several receptor-selectivity-promoting accurate guidance for identifying the effect of substitutions. substitutions. For each position, substitution maps were The performances of all models generated in this study are obtained allowing us to navigate toward desired properties, given in Figure S1. including GLP-1R potency and/or improved receptor Development of Dual GLP-1R-SCTR Agonists. We selectivity. Rather than identifying a single compound with applied the streaMLine platform to generate selective GLP-1R desired properties through an iterative design process, the agonists with suitable physicochemical parameters starting DMS generated a solution space of possible amino acid from the secretin backbone. substitutions from which peptide candidates could be designed The native secretin peptide has no GLP-1R potency, hence and synthesized. A selection of substitutions is described to identify both desired substitutions a starting point with below. some GLP-1R potency was needed. We therefore aimed at We identified amino acid positions 9, 12, and 25 where generating first a dual GLP-1R-SCTR agonist that could be substitutions could significantly improve GLP-1R selectivity by used as an intermediate for further optimization (Figure 2). increasing GLP-1R potency and decreasing SCTR potency A peptide library was designed to evaluate the effect of (Figures 4A, B and S2). At position 12, several substitutions introducing GLP-1 residues into the native secretin. Non- improved GLP-1R selectivity. 12Y most effectively improved conserved residues (position 2−3, 9−10, 12−14, and 17−25), GLP-1R potency and reduced SCTR potency, whereas 12E were changed into the corresponding GLP-1 residue, one at a only reduced SCTR potency. Aromatic residues 25H, 25F, time or in combinations (Table 1). The library (768 peptides) 25Y, and 25W improved GLP-1R potency while also reducing was screened by determining GLP-1R and SCTR potency SCTR potency, with 25H being the most effective. At position (EC50), fibril formation (ThT assay), and solubility 9, only the native GLP-1 residue 9D improved potency and (turbidity), and random forest models were trained to selectivity. determine the relationship between measured end points and In addition, amino acid positions 10, 14, and 19 were the amino acid sequence of peptides. From these models, we identified to improve selectivity by decreasing SCTR potency computed SHAP values to determine the level of contribution with a neglectable effect on GLP-1R potency (Figures 4A, B of each substitution.22 Substitutions with positive SHAP values and S2). 10I and 10V reduced SCTR potency without increase the end point, while substitutions with negative SHAP significantly compromising GLP-1R potency. A similar effect values decrease the end point. was seen by substituting position 14 to F, Y, or L. Amino acid positions 2, 9, 18, and 22 had the highest Positions 16, 18, and 22 could be substituted to enhance positive SHAP values for GLP-1R EC50, thus being critical for GLP-1R selectivity. For position 16, all mutations, except P, improving GLP-1R potency. Conversely, positions 3, 9, 10, 14, increased GLP-1R potency (Figure S2). For positions 18 and and 19 exhibited the most negative SHAP values for SCTR 22, 18A, 18Aib, 18L, 18, 22F, 22W, and 22Y considerably EC50, hence being critical for abolishing SCTR potency or improved GLP-1R potency while only marginally affecting enhancing GLP-1R selectivity (Figure 3A). SCTR potency. In addition to potency determination, the propensity for Improving Solubility and Conjugation of Fatty Acid. fibril formation and a reduction in solubility was most In parallel with DMS, we systematically investigated the effect pronounced when mutating amino acid positions 12, 14, 18, and tolerability of glutamate substitution and derivatization 19, 21, 23, and 25. The introduction of GLP-1 residues at these with half-life extenders (HLEs) in our dual GLP-1R-SCTR positions could thus negatively affect the physicochemical agonist. Glutamate substitutions can be used to modulate the properties of a peptide (Figure 3B). isoelectric point of a peptide, thereby improving solubility at Based on these learnings, five substitutions were introduced the desired formulation pH.26 Fatty acid conjugation is a well- in the secretin backbone to achieve an agonist with dual described technology broadly applied to extend the half-life of activity on GLP-1R and SCTR (Table 1). Mutations 9D, 18A, peptides from minutes to hours. Native secretin and GLP-1 E https://doi.org/10.1021/acs.jmedchem.4c00417 J. Med. Chem. XXXX, XXX, XXX−XXX Journal of Medicinal Chemistry pubs.acs.org/jmc Article Figure 5. Overview of substitution-effects from glutamate scan and half-life extender (HLE) scan in a secretin-derived GLP-1R and SCTR dual agonist. (A) Effect of introducing HLEs or glutamate. For each assay end point, a random forest model was trained on 576 peptides and used to compute SHAP values determining the level of contribution of each amino acid substitution. Delta mean SHAP values denote the contribution of substituting the backbone residue with either a glutamate or HLE. (B) Detailed overview of SHAP values for selected positions that tolerate HLE derivatization or glutamate substitution for improving half-life and solubility, respectively. Small points denote SHAP values per individual peptide and large points denote mean SHAP value. have reported half-lives in the range of 2−4 min.27,28 27. Position 12, 16, and 24 were found to have a positive Conjugation with fatty diacids facilitates strong binding to impact on GLP-1R potency compared to the backbone serum albumin, thus reducing renal clearance and enzymatic residue. Among these positions, we found the largest reduction degradation.28,29 in turbidity from introducing 16E and 24E, indicating Fatty acid conjugation was investigated by attachment to the improved solubility (Figure 5B). epsilon nitrogen of lysines via linker moieties. The combined For the HLEs, we found no major difference in potency fatty acid and linker is here referred to as the half-life extender across the different fatty acids and/or linker combinations and (HLE). Six different HLEs were evaluated in each position therefore analyzed the different HLEs as a single substitution. representing different lengths of fatty diacids, octadecanedioic Evaluating the effect of attaching HLEs on the GLP-1R acid (C18DA) and eicosanedioic acid (C20DA), and varying potency revealed several positions where HLEs were tolerated, combinations of linker moieties, L-γ-glutamyl (gGlu) and 3,8- i.e., positions 10, 12, 14, 16, 17, 20, 21, 24, 25, and 27. For dioxa-aminooctanoic acid (OEG). positions 12, 14, and 16, the attachment of HLEs was found to A library of 576 peptides was designed where each HLE at have positive effects on GLP-1R potency compared to the each position was examined in backbones comprising 0, 1, or 2 backbone residue, with positions 12 and 14 also inducing GLP- glutamate mutations. All positions were screened, except a few 1R selectivity. This selectivity effect of positions 12 and 14 was positions in the pharmacophore essential for receptor consistent with the observations from the DMS, where GLP- activation. For the glutamate substitution screening, positions 1R selectivity could also be improved by mutating these 1−5, 7, and 8 were excluded, with position 15 already being a positions (Figure 4). Importantly, no effect on turbidity was glutamate. For the HLE screening, positions 1−5, 7−9, 18, and observed when HLEs were conjugated to positions 12 (Figure 22 were excluded. The library was screened for GLP-1R and 5A) and 14 (Figure 5A, B). SCTR potency and turbidity. For each end point, we Fine-Tuning Potency, Selectivity, and Physicochem- computed SHAP values to determine the contribution of ical Properties. Previous sections described our parallel each mutation relative to the backbone residue (Figure 5). peptide development process. The conjugation of HLEs could Evaluating the effect of glutamate mutations on GLP-1R dramatically alter the properties of a peptide.26 We therefore potency revealed several amino acid positions where glutamate set out to investigate if an HLE was compatible with positions was tolerated, i.e., positions 12, 16, 17, 19, 20, 21, 24, 25, and and substitutions found to be selectivity-inducing in the deep F https://doi.org/10.1021/acs.jmedchem.4c00417 J. Med. Chem. XXXX, XXX, XXX−XXX Journal of Medicinal Chemistry pubs.acs.org/jmc Article Figure 6. Overview of substitution-effects from selected substitutions in secretin derived selective GLP-1R agonist. Effect of combining selected substitutions identified in the deep mutational scan, HLE scan, and glutamate scan. For each assay end point a random forest model was trained on 192 peptides and used to determine SHAP values determining the level of contribution of each amino acid substitution. Mean SHAP values denote the contribution of each substitution relative to the data set mean. Table 2. Profiling of Optimized Secretin-Derived GLP-1R Agonist hGLP1R hSCTR EC50 selectivity solubility pH 7.0 and 8.0 fibrillation pH 7.0 chemical stability pH 7.0 and 8.0 rat half-life, compound EC50 (nM) (nM) ratioa (mg/mL) and 8.0 (% degradation) i.v. (h) secretin 2300 0.0023 10−6 NA no 8.6/9 NA GLP-1 0.002 800 400,000 NA no 3.8/8.1 NA GUB021794 0.018 190 10,556 >10 no 1.4/0.55 22 a Selectivity ratio was calculated as hSCTR EC50 divided by hGLP1R EC50. mutational scan. Furthermore, this library would enable us to Design and Characterization of Final Candidate. rank the mutations relative to each other and according to Based on all of the substitution options identified, we designed desired end points to identify an optimal combination of GUB021794. The peptide sequence is shown in Table 1. We substitutions providing a selective GLP-1R agonist with aimed for a peptide candidate with minimal (