Convolutional Neural Networks PDF
Document Details
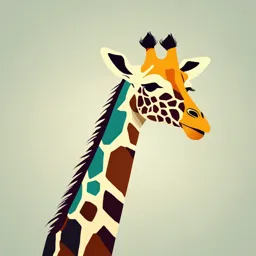
Uploaded by GuiltlessAnemone7230
Amity University Chhattisgarh
Tags
Summary
This document provides an overview of convolutional neural networks (CNNs), covering their historical context, fundamental principles (like convolution), architectures (like ConvNet), and practical applications like image recognition. It details the concepts and methods used in CNNs, which are crucial in various domains.
Full Transcript
History In 1995, Yann LeCun and Yoshua Bengio introduced the concept of convolutional neural networks. Convolution 1D (continuous, discrete) : Input Kernel f * g ( x) f ( ) g ( x )d...
History In 1995, Yann LeCun and Yoshua Bengio introduced the concept of convolutional neural networks. Convolution 1D (continuous, discrete) : Input Kernel f * g ( x) f ( ) g ( x )d N 1 Output is f ( ) g ( x ) sometimes called 0 Feature map 2D (continuous, discrete) : f * g ( x, y ) f ( , ) g ( x , y )dd N 1 N 1 f ( , ) g ( x , y ) 0 0 Convolution Properties Commutative: f*g = g*f Associative: (f*g)*h = f*(g*h) Homogeneous: f*(g)= f*g Additive (Distributive): f*(g+h)= f*g+f*h Shift-Invariant f*g(x-x0,y-yo)= (f*g) (x-x0,y-yo) ConvNet ConvNet architectures for images: – fully-connected structure does not scale to large images – the explicit assumption that the inputs are images – allows us to encode certain properties into the architecture. – These then make the forward function more efficient to implement – Vastly reduce the amount of parameters in the network. 3D volumes: neurons arranged in 3 dimensions: width, height, depth. Convnets Layers used to build ConvNets: a stacked sequence of layers. 3 main types Convolutional Layer, Pooling Layer, and Fully- Connected Layer every layer of a ConvNet transforms one volume of activations to another through a differentiable function. The replicated feature approach Use many different copies of the same feature detector with The red connections all different positions. have the same weight. – Could also replicate across scale and orientation (tricky and expensive) – Replication greatly reduces the number of free parameters to be learned. Use several different feature types, each with its own map of replicated detectors. – Allows each patch of image to be represented in several ways. Example Architecture for CIFAR-10 [INPUT - CONV - RELU - POOL - FC] INPUT [32x32x3] : the raw pixel values of the image CONV will compute the output of neurons that are connected to local regions in the input. With 12 filters, the output volume is [32x32x12] RELU : apply an elementwise activation function, such as the max(0,x) POOL will perform a downsampling operation along the spatial dimensions (width, height), resulting in volume such as [16x16x12]. FC layer will compute the class scores, resulting in volume of size [1x1x10], where each of the 10 numbers correspond to a class score, such as among the 10 categories of CIFAR-10 Convolution Layer The Conv layer is the core building block of a CNN The parameters consist of a set of learnable filters. Every filter is small spatially (width and height), but extends through the full depth of the input volume, eg, 5x5x3 During the forward pass, we slide (convolve) each filter across the width and height of the input volume and compute dot products between the entries of the filter and the input at any position. produce a 2-dimensional activation map that gives the responses of that filter at every spatial position. Intuitively, the network will learn filters that activate when they see some type of visual feature A set of filters in each CONV layer – each of them will produce a separate 2-dimensional activation map – We will stack these activation maps along the depth dimension and produce the output volume. Convolution Convolutions: More detail 32x32x3 image 32 height 32 width 3 depth Andrej Karpathy Convolutions: More detail 32x32x3 image 5x5x3 filter 32 Convolve the filter with the image i.e. “slide over the image spatially, computing dot products” 32 3 Andrej Karpathy Convolutions: More detail Convolution Layer 32x32x3 image 5x5x3 filter 32 1 number: the result of taking a dot product between the filter and a small 5x5x3 chunk of the image 32 (i.e. 5*5*3 = 75-dimensional dot product + bias) 3 Andrej Karpathy Convolutions: More detail Convolution Layer activation map 32x32x3 image 5x5x3 filter 32 28 convolve (slide) over all spatial locations 32 28 3 1 Andrej Karpathy Convolutions: More detail consider a second, green filter Convolution Layer 32x32x3 image activation maps 5x5x3 filter 32 28 convolve (slide) over all spatial locations 32 28 3 1 Andrej Karpathy Convolutions: More detail For example, if we had 6 5x5 filters, we’ll get 6 separate activation maps: activation maps 32 28 Convolution Layer 32 28 3 6 We stack these up to get a “new image” of size 28x28x6! Andrej Karpathy Convolutions: More detail Preview: ConvNet is a sequence of Convolution Layers, interspersed with activation functions 32 28 CONV, ReLU e.g. 6 5x5x3 32 filters 28 3 6 Andrej Karpathy Convolutions: More detail Preview: ConvNet is a sequence of Convolutional Layers, interspersed with activation functions 32 28 24 …. CONV, CONV, CONV, ReLU ReLU ReLU e.g. 6 e.g. 10 5x5x3 5x5x6 32 filters 28 filters 24 3 6 10 Andrej Karpathy Convolutions: More detail [From recent Yann Preview LeCun slides] Andrej Karpathy Convolutions: More detail one filter => one activation map example 5x5 filters (32 total) We call the layer convolutional because it is related to convolution of two signals: elementwise multiplication and sum of a filter and the signal (image) Adapted from Andrej Karpathy, Kristen Grauman Convolutions: More detail A closer look at spatial dimensions: activation map 32x32x3 image 5x5x3 filter 32 28 convolve (slide) over all spatial locations 32 28 3 1 Andrej Karpathy Convolutions: More detail A closer look at spatial dimensions: 7 7x7 input (spatially) assume 3x3 filter 7 Andrej Karpathy Convolutions: More detail A closer look at spatial dimensions: 7 7x7 input (spatially) assume 3x3 filter 7 Andrej Karpathy Convolutions: More detail A closer look at spatial dimensions: 7 7x7 input (spatially) assume 3x3 filter 7 Andrej Karpathy Convolutions: More detail A closer look at spatial dimensions: 7 7x7 input (spatially) assume 3x3 filter 7 Andrej Karpathy Convolutions: More detail A closer look at spatial dimensions: 7 7x7 input (spatially) assume 3x3 filter 7 => 5x5 output Andrej Karpathy Convolutions: More detail A closer look at spatial dimensions: 7 7x7 input (spatially) assume 3x3 filter applied with stride 2 7 Andrej Karpathy Convolutions: More detail A closer look at spatial dimensions: 7 7x7 input (spatially) assume 3x3 filter applied with stride 2 7 Andrej Karpathy Convolutions: More detail A closer look at spatial dimensions: 7 7x7 input (spatially) assume 3x3 filter applied with stride 2 => 3x3 output! 7 Andrej Karpathy Convolutions: More detail A closer look at spatial dimensions: 7 7x7 input (spatially) assume 3x3 filter applied with stride 3? 7 Andrej Karpathy Convolutions: More detail A closer look at spatial dimensions: 7 7x7 input (spatially) assume 3x3 filter applied with stride 3? 7 doesn’t fit! cannot apply 3x3 filter on 7x7 input with stride 3. Andrej Karpathy Convolutions: More detail N Output size: (N - F) / stride + 1 F e.g. N = 7, F = 3: F N stride 1 => (7 - 3)/1 + 1 = 5 stride 2 => (7 - 3)/2 + 1 = 3 stride 3 => (7 - 3)/3 + 1 = 2.33 :\ Andrej Karpathy Convolutions: More detail In practice: Common to zero pad the border 0 0 0 0 0 0 e.g. input 7x7 0 3x3 filter, applied with stride 1 0 pad with 1 pixel border => what is the output? 0 0 (recall:) (N - F) / stride + 1 Andrej Karpathy Convolutions: More detail In practice: Common to zero pad the border 0 0 0 0 0 0 e.g. input 7x7 0 3x3 filter, applied with stride 1 0 pad with 1 pixel border => what is the output? 0 0 7x7 output! Andrej Karpathy Convolutions: More detail In practice: Common to zero pad the border 0 0 0 0 0 0 e.g. input 7x7 0 3x3 filter, applied with stride 1 0 pad with 1 pixel border => what is the output? 0 0 7x7 output! in general, common to see CONV layers with stride 1, filters of size FxF, and zero-padding with (F-1)/2. (will preserve size spatially) e.g. F = 3 => zero pad with 1 F = 5 => zero pad with 2 F = 7 => zero pad with 3 (N + 2*padding - F) / stride + 1 Andrej Karpathy Convolutions: More detail Examples time: Input volume: 32x32x3 10 5x5 filters with stride 1, pad 2 Output volume size: ? Andrej Karpathy Convolutions: More detail Examples time: Input volume: 32x32x3 10 5x5 filters with stride 1, pad 2 Output volume size: (32+2*2-5)/1+1 = 32 spatially, so 32x32x10 Andrej Karpathy Convolutions: More detail Examples time: Input volume: 32x32x3 10 5x5 filters with stride 1, pad 2 Number of parameters in this layer? Andrej Karpathy Convolutions: More detail Examples time: Input volume: 32x32x3 10 5x5 filters with stride 1, pad 2 Number of parameters in this layer? each filter has 5*5*3 + 1 = 76 params (+1 for bias) => 76*10 = 760 Andrej Karpathy Convolutions: More detail Andrej Karpathy Spatial arrangement Three hyperparameters control the size of the output volume – Depth: no of filters, each learning to look for something different in the input. – the stride with which we slide the filter. – pad the input volume with zeros around the border. Spatial arrangement We compute the spatial size of the output volume as a function of – the input volume size (W) – the receptive field size of the Conv Layer neurons (F) – the stride with which they are applied (S) – the amount of zero padding used (P) on the border. The number of neurons that “fit” is given by (W−F+2P)/(S+1) – For a 7x7 input and a 3x3 filter with stride 1 and pad 0 we would get a 5x5 output. – With stride 2 we would get a 3x3 output. one spatial dimension (x-axis), one neuron with a receptive field size of F = 3, the input size is W = 5, and zero padding of P = 1 Stride = 1, 2 The Krizhevsky et al. architecture that won the ImageNet 2012 images of size [227x227x3]. the first Convolutional Layer, used neurons with receptive field size F=11, stride S=4, no zero padding P=0 Since (227 - 11)/4 + 1 = 55, the Conv layer had a depth of K=96, the Conv layer output volume had size [55x55x96]. Each of the 55*55*96 neurons in this volume was connected to a region of size [11x11x3] in the input volume. Moreover, all 96 neurons in each depth column are connected to the same [11x11x3] region of the input, Parameter Sharing Parameter sharing controls the number of parameters. If there are 55*55*96 = 290,400 neurons in the first Conv Layer, and each has 11*11*3 = 363 weights and 1 bias. Together, this adds up to 290400 * 364 = 105,705,600 parameters on the first layer of the ConvNet alone. Reduce by parameter sharing now have only 96 unique set of weights (one for each depth slice), for a total of 96*11*11*3 = 34,848 unique weights, or 34,944 parameters (+96 biases) During backpropagation, every neuron in the volume will compute the gradient for its weights, but these gradients will be added up across each depth slice and only update a single set of weights per slice. Summary of Conv Layer Accepts a volume of size W1×H1×D1 Requires four hyperparameters: – Number of filters K – their spatial extent F – the stride S – the amount of zero padding P Produces a volume of size W2×H2×D2 – W2=(W1−F+2P)/S+1 – H2=(H1−F+2P)/S+1 – D2=K With parameter sharing, it introduces F⋅F⋅D1 weights per filter, for a total of (F⋅F⋅D1)⋅K weights and K biases. In the output volume, the d-th depth slice (of size W2×H2) is the result of performing a valid convolution of the d-th filter over the input volume with a stride of S, and then offset by d-th bias. Pooling Layer Insertion of pooling layer: – reduce the spatial size of the representation reduce the amount of parameters and computation in the network, and hence also control overfitting. The Pooling Layer operates independently on every depth slice of the input and resizes it spatially, using the MAX operation. The most common form is a pooling layer with filters of size 2x2 applied with a stride of 2 -- downsamples every depth slice in the input by 2 along both width and height, MAX operation would in take a max over 4 numbers (little 2x2 region in some depth slice). The depth dimension remains unchanged. General pooling layer Accepts a volume of size W1×H1×D1 Requires two hyperparameters: – their spatial extent F – the stride S Produces a volume of size W2×H2×D2 where: – W2=(W1−F)/S+1 – H2=(H1−F)/S+1 – D2=D1 Introduces zero parameters Other pooling functions: Average pooling, L2- norm pooling General pooling Backpropagation. the backward pass for a max(x, y) operation routes the gradient to the input that had the highest value in the forward pass. Hence, during the forward pass of a pooling layer you may keep track of the index of the max activation (sometimes also called the switches) so that gradient routing is efficient during backpropagation. Getting rid of pooling 1. Striving for Simplicity: The All Convolutional Net proposes to discard the pooling layer and have an architecture that only consists of repeated CONV layers. To reduce the size of the representation they suggest using larger stride in CONV layer once in a while. Argument: – The purpose of pooling layers is to perform dimensionality reduction to widen subsequent convolutional layers' receptive fields. – The same effect can be achieved by using a convolutional layer: using a stride of 2 also reduces the dimensionality of the output and widens the receptive field of higher layers. The resulting operation differs from a max-pooling layer in that – it cannot perform a true max operation – it allows pooling across input channels. Springenberg, Jost Tobias, et al. "Striving for simplicity: The all convolutional net." arXiv preprint arXiv:1412.6806 (2014). Getting Rod of Pooling 2 2. Very Deep Convolutional Networks for Large-Scale Image Recognition. The core idea here is that hand-tuning layer kernel sizes to achieve optimal receptive fields (say, 5×5 or 7×7) can be replaced by simply stacking homogenous 3×3 layers. The same effect of widening the receptive field is then achieved by layer composition rather than increasing the kernel size – three stacked 3×3 have a 7×7 receptive field. – At the same time, the number of parameters is reduced: – a 7×7 layer has 81% more parameters than three stacked 3×3 layers. Simonyan, Karen, and Andrew Zisserman. "Very deep convolutional networks for large-scale image recognition." arXiv preprint arXiv:1409.1556 (2014). Fully-connected layer Neurons in a fully connected layer have full connections to all activations in the previous layer Their activations can hence be computed with a matrix multiplication followed by a bias offset. Converting FC layers to CONV layers the only difference between FC and CONV layers is that the neurons in the CONV layer are connected only to a local region in the input, and that many of the neurons in a CONV volume share parameters. However, the neurons in both layers still compute dot products, so their functional form is identical. Converting FC layers to CONV layers For any CONV layer there is an FC layer that implements the same forward function. The weight matrix would be a large matrix that is mostly zero except for at certain blocks (due to local connectivity) where the weights in many of the blocks are equal (due to parameter sharing). Conversely, any FC layer can be converted to a CONV layer. For example, an FC layer with K=4096 that is looking at some input volume of size 7×7×512 can be equivalently expressed as a CONV layer with F=7,P=0,S=1,K=4096. In other words, we are setting the filter size to be exactly the size of the input volume, and hence the output will simply be 1×1×4096 since only a single depth column “fits” across the input volume, giving identical result as the initial FC layer. ConvNet Architectures Layer Patterns The most common architecture stacks a few CONV-RELU layers, follows them with POOL layers, and repeats this pattern until the image has been merged spatially to a small size. At some point, it is common to transition to fully-connected layers. The last fully-connected layer holds the output, such as the class scores. In other words, the most common ConvNet architecture follows the pattern: INPUT -> [[CONV -> RELU]*N -> POOL?]*M ->[FC -> RELU]*K -> FC N >= 0 (and usually N = 0, K >= 0 Prefer a stack of small filter CONV to one large receptive field CONV layer. three layers of 3x3 CONV vs a single CONV layer with 7x7 receptive fields. The receptive field size is identical in spatial extent (7x7), but with several disadvantages. 1. The neurons would be computing a linear function over the input, while the three stacks of CONV layers contain non-linearities that make their features more expressive. 2. If we suppose that all the volumes have C channels, the single 7x7 CONV layer would contain C×(7×7×C)=49C2 parameters, while the three 3x3 CONV layers would contain 3×(C×(3×3×C))=27C2 parameters. Intuitively, stacking CONV layers with tiny filters as opposed to having one CONV layer with big filters allows us to express more powerful features of the input, and with fewer parameters. Case Studies LeNet. The first successful applications of Convolutional Networks were developed by Yann LeCun in 1990’s. was used to read zip codes, digits, etc. AlexNet. popularized Convolutional Networks in Computer Vision, developed by Alex Krizhevsky, Ilya Sutskever and Geoff Hinton. The AlexNet was submitted to the ImageNet ILSVRC challenge in 2012 and significantly outperformed the second runner-up (top 5 error of 16% compared to runner-up with 26% error). The Network had a very similar architecture to LeNet, but was deeper, bigger, and featured Convolutional Layers stacked on top of each other ZF Net. The ILSVRC 2013 winner was a Convolutional Network from Matthew Zeiler and Rob Fergus. An improvement on AlexNet by tweaking the architecture hyperparameters, -- expanding the size of the middle convolutional layers and making the stride and filter size on the first layer smaller.