Model Evaluation Presentation PDF
Document Details
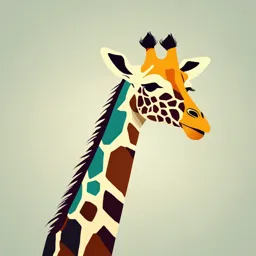
Uploaded by MesmerizingGyrolite5380
Ajou University
So Yeon Kim
Tags
Related
Summary
This presentation covers different methods for evaluating machine learning models, emphasizing model validation techniques like cross-validation, holdout validation, and leave-one-out methods. Evaluation metrics including confusion matrices, and ROC curves are also discussed.
Full Transcript
Model evaluation 의료 인공지능 So Yeon Kim Cross-validation 2 Overfitting and cross-validation Internal validation: validate your model on your current data set (cross- validation) External validation: validate your model on a completely new dataset...
Model evaluation 의료 인공지능 So Yeon Kim Cross-validation 2 Overfitting and cross-validation Internal validation: validate your model on your current data set (cross- validation) External validation: validate your model on a completely new dataset 3 Overfitting and cross-validation Cross-validation To choose the best parameter setting To prove that your model does not over-fit the training data Holdout validation Leave-One-Out Cross Validation (LOOCV) K-fold Cross Validation 4 Tuning Hyperparameters Suppose you want to determine a good value for some hyperparameter Partition data into train and test set (Holdout) In general, 80% of training and 20% test set. 5 Tuning Hyperparameters For each hyperparameter value 𝑖, run learning algorithm on training set and evaluate on test set 6 Holdout validation Advantages: Fast and computationally efficient Simple to implement Scalable for large dataset Disadvantages: High variance: results can vary significantly if different subsets are chosen Waste data: only a portion of the data is used for training, and the other portion is held out for testing, which may lead to less accurate models, especially with smaller datasets. 7 Leave-One-Out Cross Validation (LOOCV) Use only one sample as the test data and the remaining (N-1) samples for model training. Model validation is performed N times, corresponding to the total number of data samples. 8 Leave-One-Out Cross Validation (LOOCV) Model validation is performed N times, resulting in N accuracy values, and the average of these values becomes the final performance score. Accuracy Accuracy Accuracy Average Accuracy 9 Leave-One-Out Cross Validation (LOOCV) Advantages: Maximizes data usage (especially useful for small datasets) Low variance: Since every sample is tested, it provides a more stable and reliable estimate Disadvantages: Computationally expensive May overfit, since the training set is almost the entire dataset, the model might overfit on similar data patterns, giving an optimistic view of performance 10 K-fold Cross Validation After dividing the dataset into k subsets, perform holdout validation k times. In each fold, use one of the k subsets as the test set, and use the remaining (k-1) subsets for model training. 11 K-fold Cross Validation Perform model validation K times, resulting in K accuracy values, and the average of these values becomes the final performance score. Although the K subsets are randomly divided, testing the model on all subsets makes this method more accurate than holdout validation. Advantages: All data points are used for both training and testing, ensuring that no data is wasted Reduces overfitting risk More stable and reliable performance estimates (less variance) 12 K-fold Cross Validation As the value of K increases, the variance in performance decreases, but computation takes longer. N-fold cross-validation = LOOCV (N: number of samples) There is still some sensitivity to how the data is split into folds, especially when K is small. It is important to choose an appropriate value for K based on the dataset size. Typically, K = 5 or 10 is used. 13 Evaluation metrics 14 Confusion Matrix True Positive (TP): Correctly classified as the class of interest False Negative (FN): Incorrectly classified as not the class of interest False Positive (FP): Incorrectly classified as True class the class of interest True Negative (TN): Correctly classified Positive (1) Negative (0) as not the class of interest True Positive False Positive Positive (FP) (TP) (1) Type I error Predicted False Negative True Negative Negative (FN) (TN) (0) Type II error 15 Confusion Matrix False Positive (FP): Predicting a value as 1 when it is actually 0 (Type I error) False Negative (FN): Predicting a value as 0 when it is actually 1 (Type II error) Case 1: A problem to determine whether a drug is effective for a certain disease If the drug is actually ineffective but is judged to be effective (Type I error) If the drug is actually effective but is judged to be ineffective (Type II error) If a drug is falsely judged as effective when it isn’t, a patient may take it and fail to be treated, making it much more dangerous. Therefore, reducing Type I errors is much more important. 16 Confusion Matrix False Positive (FP): Predicting a value as 1 when it is actually 0 (Type I error) False Negative (FN): Predicting a value as 0 when it is actually 1 (Type II error) Case 2: A problem of diagnosing cancer patients When a healthy person is misdiagnosed as having cancer (Type I error). When a cancer patient is misdiagnosed as healthy (Type II error). Misdiagnosing a cancer patient as healthy is more critical, so reducing Type II errors is much more important. 17 How to evaluate the model? 𝑇𝑃 + 𝑇𝑁 Accuracy = 𝑇𝑃+𝐹𝑃+𝐹𝑁+𝑇𝑁 True class Accuracy alone can be misleading Predicted Positive (1) Negative (0) In classification problems where the False Positive True Positive proportion of class 0 is 99%, even if the Positive (TP) (FP) (1) Type I error classifier predicts all instances as class 0, the accuracy will still be 99%. False Negative TP = 0, TN = 99 Negative (FN) True Negative (TN) (0) Type II error 18 How to evaluate the model? 𝑇𝑃 Sensitivity (TP rate, Recall) = 𝑇𝑃+𝐹𝑁 True class The proportion of actual 1s that were correctly predicted as 1 Predicted Positive (1) Negative (0) 𝑇𝑁 Specificity (TN rate) = True Positive False Positive 𝐹𝑃+𝑇𝑁 Positive (FP) (TP) The proportion of actual 0s that were (1) Type I error correctly predicted as 0 False Negative True Negative Negative (FN) (TN) (0) Type II error 19 How to evaluate the model? 𝑇𝑃 Precision = 𝑇𝑃+𝐹𝑃 True class The proportion of predicted 1s that are actually 1 Predicted Positive (1) Negative (0) 𝑃𝑟𝑒𝑐𝑖𝑠𝑖𝑜𝑛×𝑅𝑒𝑐𝑎𝑙𝑙 F1 score = 2 × True Positive False Positive 𝑃𝑟𝑒𝑐𝑖𝑠𝑖𝑜𝑛+𝑅𝑒𝑐𝑎𝑙𝑙 Positive (TP) (FP) The harmonic mean of Precision and Recall (1) Type I error False Negative True Negative Negative (FN) (TN) (0) Type II error 20 Sensitivity & Specificity Tradeoff High Sensitivity: Low false negative rate. High Specificity: Low false positive rate. Case 1: Airport security If the equipment fails to detect a terrorist carrying a weapon (high FN rate), it is extremely dangerous. Increasing sensitivity is much more important than specificity. Case 2: COVID-19 self-test kit Sensitivity: The probability of correctly identifying a truly positive patient as positive. Specificity: The probability of correctly identifying a truly negative patient as negative. It is ideal to have both high sensitivity and high specificity. 21 ROC (Receiver Operating Characteristic) curve ROC curve plots (1-specificity) (or FPR) on the x-axis against sensitivity (or TPR) on the y-axis TPR = TP / (TP+FN) FPR = FP / (FP+TN) AUROC (Area Under the ROC Curve) 22 How to evaluate the model? When we have an unbalanced dataset (more positives than negatives), Precision Recall Curve (PRC) is useful Precision = TP / (TP+FP) Recall = TPR = TP / (TP+FN) AUPRC (Area Under the PRCurve) 23 Evaluation: Regression MAE, RMSE Pearson correlation, Spearman rank correlation Correlation coefficient and significant tests 24 Summary: Evaluation metrics Classification Accuracy, precision, recall, F1 score, AUC Regression Mean absolute error, root mean squared error Pearson, spearman rank correlation, one sided/two sided test, correlation significance test Clustering Adjusted mutual information, adjusted rand score, silhouette index https://scikit-learn.org/stable/modules/model_evaluation.html 25 Thank You! Q&A 26