Meta Marketing Science Professional Exam Study Guide PDF
Document Details
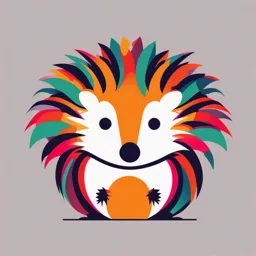
Uploaded by FearlessLoyalty
2023
Meta
Tags
Related
- ecosistema-del-marketing-digital-v81BIR4g.pdf
- El plan de marketing digital PDF.pdf
- 1. Introduction to Digital Marketing.pdf
- Traditional Marketing Versus Digital Marketing.pdf
- Learn Digital Marketing - Class 1_ Introduction to Digital Marketing.pdf
- Learn Digital Marketing - Class 2_ Content Marketing .pdf
Summary
This study guide covers topics for Meta's Marketing Science Professional Exam. It details setting business goals, identifying KPIs, and accessing and analyzing data, making it an excellent resource for professionals preparing for the exam.
Full Transcript
Marketing Science Professional Exam study guide JANUARY 2023 VERSION Table of contents Overview 03 Assess 04 Hypothesize 11 Recommend measurement solutions 20 Perform an analysis 32 Generate insights 47 Make data-driven recommendations 52 Glossary 61 Overview Meta Certification c...
Marketing Science Professional Exam study guide JANUARY 2023 VERSION Table of contents Overview 03 Assess 04 Hypothesize 11 Recommend measurement solutions 20 Perform an analysis 32 Generate insights 47 Make data-driven recommendations 52 Glossary 61 Overview Meta Certification can help you get noticed. Meta Certification recognizes advanced-level proficiency with Facebook, Instagram and Messenger. Certification is the highest level of accreditation that Meta recognizes in digital marketing. Help boost your resume, credibility and career. This document will help prepare you for the Meta Certified Marketing Science Professional Exam, which measures your competency in using data, insights and measurement to make informed marketing recommendations. To learn more about the exam, visit the Marketing Science Professional Certification website. Find answers to frequently asked questions and learn more about Meta Certification. 3 Assess Introduction Clearly defined goals and KPIs are the foundation of any measurement approach. When you create a marketing strategy, your business goals should guide all of your strategic decisions. In addition, at the beginning of the measurement journey, it’s important to assess your data sources, the quality of the data that they enable and the methodologies available to you. Define business goals and KPIs. The best-defined business goals follow a rubric: they are specific, measurable, achievable, relevant and time-bound, or SMART. If you define a business goal that is non-measurable or lacks specificity, strategic decisions will become increasingly complex and less effective. Once you decide on a business goal, you’ll need to determine the KPIs that will measure the success of your campaign. KPIs make clear what outcome will be measured to evaluate your success. But KPIs are more than metrics— they tell a story about what is happening in a business at the time of measurement. To identify your KPIs, ask yourself: ● ● What does success look like? Are my current metrics proxies or do they correlate with business outcomes? Metrics such as likes, comments and followers are proxies and do not necessarily measure incremental impact, or the additional impact that results from running an ad. They can vary by channel. For example, TV metrics include gross rating point (GRP), cost per mille (CPM) and target rating point (TRP), while paid search metrics include impressions and click-through rate (CTR). Instead of using these proxy metrics for KPIs, use metrics such as: ASSESS Incremental reach Website visits Conversions Lift App events Ad recall 5 Examples of goals turned into KPIs Goal Increase sales by 10% in 2021 Increase app installs by 10% in Q4 Increase ad recall by 5 points in Q1 Generate new leads in Q1 KPI Number of sales units Number of mobile app installs Ad recall lift Number of submitted forms Primary and secondary KPIs Primary KPIs Secondary KPIs What is it? The overall business objective that ad spend is being measured against. It might be the primary question that the business is trying to answer. Support primary KPIs. They are important business objectives that ad spend can be measured against to better contextualize the primary KPI. Example Ad recall lift Ad recall lift in a specific target audience caused by video campaigns Evaluate limitations of KPIs. While KPIs are important, they have limitations. To evaluate the limitations of a KPI, identify: Measurable business objectives Which business objectives might not be measurable with available tools, such as non-traceable tender, and therefore cannot be valid KPIs. Primary and secondary KPIs Which KPIs are actually primary vs. secondary. Ensure focus on the primary and don’t switch between KPIs mid-campaign. Accurate measurement Whether the amount of available data for a specific metric is enough to provide an accurate measurement of a proposed KPI. If not, evaluate switching to a different KPI or moving to a different proxy metric. ASSESS 6 Inventory existing data sources. Once you have your business goals and KPIs defined, assess your existing data sources. Data sources can capture and report KPI data with accuracy and timeliness. A data source is a tool, connection, piece of code or other object that collects information that can later be measured and analyzed. A business can receive data from the following sources: Tests and experiments Examples: ● Brand Lift ● Conversion Lift ● A/B Test results Data about marketing performance Examples: ● Ads Reporting ● Marketing mix models Actions people take On websites, mobile apps or in stores using: ● Meta Pixel ● Facebook SDK ● Offline events ASSESS 7 Types of data First-party data refers to data generated from Meta, while third-party data refers to non-Meta data. Within these categories, there are four types of data you’ll use: Actions people take on your website, in your mobile app and in your stores Campaign performance Tests or experiments Marketing performance overall To assess your data source strategy, ask yourself: ● ● What data sources are fueling my success metrics? What data sources are in place and what data do you have access to? Validate data quality. Not all data and data sources are equal. They vary based on dimensions like granularity and scope. Consider all the tools available to you and use those that will generate data aligned with your business goal. Once you have access to a data source, identify abnormalities in data, such as missing data or outliers. ASSESS 8 Evaluate differences in measurement methodology. Understanding the types of data sources and data available is foundational to choosing an appropriate measurement methodology. Consider the different methodologies that can be used to measure impact. Below, we define the measurement methodologies and review the limitations of each. Method Description A/B test A test that allows you to conduct experiments that compare multiple ad sets by splitting audiences into randomized and mutually exclusive groups. ● Does not assess incremental impact. RCTs test a hypothesis by introducing a treatment, studying the effects and determining the impact of your ad. Ultimately, an RCT can help you decide how much to spend on each marketing channel to maximize your results. It can infer causality. ● Tests may not be set up with sufficient statistical power. Randomized control trial (RCT) Limitations ● Reliable only if the confidence level is at least 75%. ● The treatment variable may not always be isolated. ● Some tests will have effects beyond initial user interaction. ● They can’t account for the unknown, such as when people use cash or make other untraceable purchases. ● Test and control groups might have outliers. ● Outcomes might be difficult to replicate. Observational method A measurement method in marketing research that observes the effect of ads on people without changing who is exposed to the ads. ● Not experimental, so causal inferences cannot be made. ● Difficult to perform strategy comparisons in a controlled way. ● Might deliver biased outcomes. ● Does not take into consideration contextual variables that may affect the final outcome. ASSESS 9 State assumptions based on situation assessment. Each combination of data type and methodology requires a statement of assumptions in order to correctly assess the situation at hand. Identify whether business objectives are being measured in a way that is compatible with Meta’s measurement tools and whether there are any potential data blind spots. For example, if you are measuring online conversions, make sure you have the correct data source in place (Meta pixel) and it is implemented correctly. If you do not have a Meta pixel on your website, then you will not be able to use Attribution or Lift solutions ASSESS 10 Hypothesize Introduction To run an effective experiment, you should first choose a hypothesis, or question, that you want your experiment to answer. A strong, well-structured hypothesis can be confirmed or rejected through testing and can lead to valuable insights. Formulate a hypothesis. What is a hypothesis? A hypothesis is an idea or explanation that you can test through study and experimentation. A strong hypothesis includes: ● ● ● ● ● Who: audience What: behavior of that audience Where: location When: ad schedule Why: the rationale for the anticipated audience behavior or perspective In order to develop a hypothesis, you need to first determine a variable and then apply research and industry context. Examples of variables ● ● ● Audience Placement Creative: format, message, quantity of ads Delivery optimization HYPOTHESIZE ● ● ● ● Campaign objective Account structure Budget Frequency 12 Example hypothesis 1 An ecommerce clothing company’s goal is to increase online purchases. In May, they launch their summer line and hypothesize that lifestyle-focused creative will achieve more online purchases than value-focused creative among the 18-34 age group. ● ● ● ● Who: people ages 18-34 What: purchase Where: online When: May ● ● ● ● Who: women over the age of 25 What: brand awareness Where: Facebook and Instagram When: June through August Example hypothesis 2 A CPG/FMCG advertiser’s goal is to increase brand awareness for a new hair-care product among a broad global audience of women over the age of 25 between June and August. The advertiser seeks to test the hypothesis that a 10-second video format on Facebook and Instagram would increase brand awareness more effectively than their current 30-second videos. Formulate a test hypothesis. What is a test hypothesis? A test hypothesis is a proposed explanation for an observation you can test, usually in the form of an if/then statement. While a hypothesis could be a subjective assumption, a test hypothesis includes a null and an alternative hypothesis. The objective is to test if the null hypothesis can be rejected and the alternative can be accepted. How to create a test hypothesis ● ● ● ● Identify the independent variables and dependent variables. Ensure variables are specific, measurable and aligned with your business goal. You can test multiple variables at once, but advertisers should test one variable at a time if the goal is to understand and isolate the effect of the strategy being tested. Identify a null and an alternative hypothesis to understand exactly what is being tested. HYPOTHESIZE 13 Example test hypothesis If an advertiser increases the number of ad placements from 1 to 4 or more, their average cost per acquisition (CPA) will decrease. ● ● Independent variable = the number of ad placements Dependent variable = CPA Null hypothesis: ● CPA (1 placement) = CPA (4 placement) Alternative hypothesis: ● CPA (1 placement) < CPA (4 placement) Determine a measurement approach. Once you have a hypothesis, prove or disprove it by gathering existing insights or research or conducting a test. Choose from the following measurement approaches: ● ● ● ● ● Cross-channel reach reporting Attribution Marketing mix modeling Randomized control trial (RCT) A/B test HYPOTHESIZE 14 Cross-channel reach reporting Measures how channels work together to generate business outcomes. Channels include but are not limited to email, TV, direct mail, Facebook and paid search. Limitations ● ● ● ● Metrics vary by channel. Cross-channel reach reporting can be challenging because not all channels share touchpoint/reach data. Limitations vary by specific solution. In general, limitations arise when metrics are not comparable or like-for-like data is not available. Reach does not always correlate with brand and conversion business outcomes. Example of cross-channel reach reporting Context A CPG advertiser runs ads on multiple platforms, including TV and digital channels, with the goal of reaching a broad audience at an efficient cost per person. Hypothesis The advertiser seeks to test the hypothesis that investment in TV delivers more efficient reach relative to their digital channels. Measurement approach The advertiser uses cross-channel reach reporting to test this hypothesis. HYPOTHESIZE 15 Attribution The process of determining credit for each touchpoint on a consumer’s path to conversion. Limitations ● Multi-touch attribution models, or MTAs, allocate value to more than one touchpoint in a consumer’s path to conversion. Data-driven attribution is the process of determining credit for touchpoints on a conversion path often based on historical data and statistical modeling ● ● ● Statistical attribution models can be costly to access in some cases, while others are limited to specific channels. Cross-device limitations on cookie- or web-based systems. Many tools don’t have access to the full path to conversion. For example, in-app impression data is often not shared across publishers. Often only includes digital channels. Example of Attribution Context An advertiser running prospecting and retargeting campaigns on Facebook and Instagram sought to increase return on ad spend (ROAS) from their Facebook and Instagram advertising during their highest sales period. They do not want to have a holdout on their media during this time. Hypothesis They seek to test the hypothesis that their prospecting campaigns deliver less incremental value than their retargeting campaigns but cannot run an experiment at this time. Measurement approach They use our data-driven attribution model to assess the estimated incremental value of these campaigns in the interim. HYPOTHESIZE 16 Marketing mix modeling A data-driven statistical analysis that quantifies the incremental sales impact and return on investment of marketing and nonmarketing activities, measuring both offline and online sales across channels. Limitations ● ● ● ● ● Can struggle to capture incremental sales where an increase is minimal. Requires collaboration between modelers and an econometric model. Doesn’t help with in-channel optimization. Does not infer causality, only correlation. Can be time-intensive to implement. Example of Marketing mix modeling Context An agency wants to optimize spend across channels for a soda brand. The soda brand client delivers media on TV, email, direct mail, Facebook and other digital channels. Hypothesis The agency hypothesizes that TV achieves a higher ROI than direct mail, and therefore more budget should be allocated to TV next year. Measurement approach Instead of looking at the impact of each channel in a silo, a marketing mix model helps to understand the effect of each channel on sales outcomes in the previous year. HYPOTHESIZE 17 Randomized control trial (RCT) An experiment designed to measure causality. It includes randomization of participants into mutually exclusive groups. One or more of these groups receive the treatment called the test group, while one or more do not receive the treatment, called the control groups. The control provides a standard of comparison and can represent a standard practice, a placebo or no treatment. Limitations ● ● ● ● ● ● ● Tests may not be set up with sufficient statistical power. For example, budget is too low, test duration is too short or not enough conversions. Accurate power calculations can be challenging to carry out where there is a lack of historical data from similar activity on which to base the calculation. Control groups may become contaminated by media running outside the experiment that targets the audience being measured. Treatment variable may not always be isolated. Tests may not run long enough to capture the full purchase cycle or longer-term impact of the strategy being measured. Experiments may not always capture the full impact of a strategy (for example, offline data may not be available to upload). Not all experimental approaches manage outliers and variance equally. Example of Randomized control trial (RCT) Context An advertiser running ads on multiple channels, including Facebook, wants to measure the sales caused by their Facebook advertising in order to assess the accuracy of their current attribution model. Hypothesis The advertiser hypothesizes that their Facebook advertising achieves $500,000 in incremental sales and a $50 cost per incremental purchase in the month of June. Measurement approach They run a randomized control trial experiment to measure the causal impact of their Facebook advertising. HYPOTHESIZE 18 A/B test Testing multiple campaigns against one another to see which tactical approach produces the best results based on your KPIs. Limitations ● ● Some A/B tests don’t include control groups, only randomized test groups. In these cases, they don’t measure causality or the incremental value of a strategy. Therefore, they are not recommended when strategy A has a different baseline conversion rate than strategy B, such as audience. Not all tests are appropriately powered with adequate conversion numbers in each group. Example of A/B test Context An advertiser running ads in Feed on Facebook only wants to reduce their average CPA. Hypothesis They seek to test the hypothesis that incorporating Instagram Feed as an additional placement along with Feed on Facebook would reduce their average CPA. Measurement approach They run an A/B test to measure the cost per result from both strategies. Identify next steps. Once you’ve validated or invalidated your hypothesis, determine the possible actions for the outcomes and recommend appropriate next steps. For example, if your hypothesis was validated, you might test a new hypothesis. You might find that your insights will lead to new questions and new hypotheses that will be the foundation for new tests. It’s always a good idea to think about testing beyond what you have already tested. HYPOTHESIZE 19 Recommend measurement solutions Introduction Meta measurement tools unlock the potential to optimize your ads, understand your audience and grow your business. Measurement solutions like Brand Lift and Conversion Lift tests can help your business find answers to many of the questions you have about ads with the help of rigorous scientific testing. Using randomized control groups, you’ll be able to see how much your Meta ads lead to conversions, which campaign causes the lowest-cost conversions and much more. Design a test. Meta offers a variety of solutions to test your hypothesis. You’re ready to design a test once you have the following: ● ● ● ● A business goal A primary KPI A strong hypothesis A variable to test RECOMMEND MEASUREMENT SOLUTIONS 21 A/B test Use this type of test to test different treatments of one of the following variables: Ad creative Delivery strategy Placement Product sets Target audience After you choose the variable you want to test, we’ll divide your budget to equally and randomly split exposure between two versions. An A/B test can then measure the performance of each strategy on a cost per result basis or cost per conversion lift basis with a holdout. When to use an A/B test To determine best practices To perform day-to-day tactical decision-making To see results based on last ad attribution When you are sure that baseline levels between A and B groups are similar When looking for a test that is easy and quick to set up Example A marketing strategist at Wind & Wool, a fashion retailer, wants to test the impact of a “Learn More” call-to-action button, as compared to a “Shop Now” button. Both buttons direct the audience to the promotions page on their website. With an A/B test, Wind & Wool learns which text is more effective and uses that knowledge to refine their future campaign strategies. Ad A Best performance Group A Group B Ad B RECOMMEND MEASUREMENT SOLUTIONS 22 Lift test Effective measurement starts by understanding incremental business outcomes, such as brand equity and conversions, that your ads can affect. When to use a Lift test To measure incremental outcomes by comparing actions of people who have seen your ad with people who haven’t To see how Meta ads affects the outcome of your ads To learn about cost per lift point, which allows advertisers to optimize their spend in the most efficient way possible Lift tests with statistically significant results can infer causality and accurately measure incrementality, unlike proxy metrics, like clicks and likes, which are indirect approximate measurements that may not be correlated with actual business value and can result in suboptimal business decisions. Test group Exposed to variable Hypothesis Compare conversions between the two groups to determine the true incremental value of the strategy. Control group Not exposed to variable RECOMMEND MEASUREMENT SOLUTIONS 23 Example of a Conversion Lift test An analyst at an ecommerce business develops a test hypothesis that states: Using automatic placements increases incremental sales for business as compared with Facebook placements alone. They run a Conversion Lift test to compare sales from Facebook with sales from automatic placements. Results show with 99.9% confidence that the use of automatic placements resulted in additional conversions, compared with Facebook alone. Example of a Brand Lift test Radiance, an online jewelry business, wants people to get to know their brand and think of them for special occasions. Because they are a new business, it is critical for them to attract and dazzle their prospective customer base, which is identified as men and women currently in relationships. Radiance’s goal is to see a 20-point lift in ad recall by mid-February, after Valentine’s Day. They run a Brand Lift test to quantify the value of their advertising on ad recall. RECOMMEND MEASUREMENT SOLUTIONS 24 Design a Lift test. There are two ways to design your lift test: Single-cell test Multi-cell test This option is best used to get a baseline understanding of incremental brand or conversion outcomes your campaign is currently driving. Compare two competing strategies to understand which leads to greater incrementality. In general, keep Lift tests as simple as possible. The more cells you add, the more complex the test becomes. You can also perform multiple tests. For example, you can use an A/B test to choose between two creatives, and in addition, a Conversion Lift test to understand the incremental conversions that result from this new creative strategy. Single-cell Was your campaign a success? Multi-cell How can you optimize? Target B Target A Ads on Facebook No ads on Facebook vs. Broad vs. narrow targeting vs. Creative 2 Creative 1 Influencer vs. brand Feed vs. Stories vs. Freq. A Freq. B vs. RECOMMEND MEASUREMENT SOLUTIONS Freq. C vs. Was the buy frequency sufficient to deliver creative impact? 25 Measurement solutions You can choose from the following solutions to measure your ad performance. Attribution Strengths ● ● ● ● Limitations ● ● ● Outputs ● ● ● Example Measure the performance of your ads across channels (paid vs. organic), publishers and devices. Learn about your consumer’s journey to purchase. Choose from two types of models: rule-based and statistical-based. Can be a useful complement to lift tests. Since there are a variety of attribution models, it will take time and experimentation to find the one that best fits your business. The length of the attribution window may limit results. Results may not reflect performance from all marketing efforts. Metrics for variables related to the media channels used in marketing, such as paid, organic and direct Metrics for variables that relate to the desired action, such as visits, conversions and sources Return on ad spend (ROAS) An advertiser who uses a mix of prospecting and retargeting campaigns can track their consumer journey and attribute incremental value to all of their media touchpoints. This allows them to optimize budgets across publishers and tactics. RECOMMEND MEASUREMENT SOLUTIONS 26 Brand Lift Strengths ● ● ● ● Limitations Outputs Example Measure the incremental impact your ad has on people’s perception of your brand. Can be single-cell or multi-cell. See how your campaign performs against norms for campaigns in your industry and region. See lift by demographic breakdown (for example, age, gender). Can be performed with third-party measurement partners. ● You need to get at least 250 responses to one poll question in order for Meta to show results. A holdout is required for measurement. ● ● ● ● ● Poll results Brand lift percent for all responses Brand lift percent by demographic Cost per Brand Lift Test details: a summary of your test setup Confidence levels ● An advertiser uses Brand Lift to understand which tactics result in the greatest awareness of its new line extension. For managed Brand Lift studies, work with an account representative to set up the tests. RECOMMEND MEASUREMENT SOLUTIONS 27 Conversion Lift Strengths ● ● ● Measure the incremental impact your ad has on people’s perception of your brand. Use intent to treat (ITT) to manage the effect of potential error in the test results and more accurately ensure comparable audiences. Can be performed with third-party measurement partners. Limitations ● ● A holdout is required for measurement. Must ensure that the test has at least 80% statistical power in order to allow for statistically significant outcomes. Outputs ● ● ● ● ● ● ● Conversion lift Sales lift Cost per conversion lift ROAS lift Conversion lift percent Breakdowns by demographic and attribution window Confidence levels Example An advertiser uses Conversion Lift to understand which of its targeting audiences generates the greatest incremental ROAS. RECOMMEND MEASUREMENT SOLUTIONS 28 Marketing mix models (MMM) Strengths ● ● ● Limitations ● ● ● ● ● Outputs ● ● ● Example Quantify the impact of a large set of variables on sales. Understand what influenced past sales and predict what may happen as a result of future marketing. Understand how your marketing activity impacts sales. Requires high-quality data. Requires collaboration between modelers and an econometric model. Doesn’t help with in-channel optimization. Doesn’t infer causality, only correlation. Can take up to six months to fully implement. Marginal return associated with each marketing channel A report that details how much influence each of your marketing activities had on sales An overview of how your spending in different channels contributed to success An advertiser wants to cut its marketing budget by 10% and uses MMM to decide where to direct the cuts. RECOMMEND MEASUREMENT SOLUTIONS 29 A/B test Strengths ● ● ● ● Limitations ● ● Outputs Example ● ● ● Assess the correlation between different versions of your ads. Create multiple ad sets and test them against each other to see which tactical approach produces the best results. Understand which specific images, videos, placement, text and/or call to action performs best. Know which combination of variables (creative, audience, delivery optimization, product sets or placement) performs better at meeting your business goal. Understand the best allocation between full funnel stages. While split testing creates random, non-overlapping groups, it does not create a corresponding control group, like Lift studies do. As a result, A/B tests do not show incremental impact. If your split groups have different baseline levels, then results will be difficult to interpret, because differences in metrics are to be expected. Winning ad set Cost per result of each ad set Confidence level An advertiser hypothesizes that their ads get more engagement with Instagram Stories as compared to Instagram News Feed. They use an A/B test to understand which placement is more effective for their ads. RECOMMEND MEASUREMENT SOLUTIONS 30 Determine test feasibility. Now that you’ve chosen an approach, there are several factors to consider when assessing the likelihood of success of your chosen measurement solution, including: Potential reach A larger holdout increases statistical power because it increases the size of the control group that you are comparing against. Look for a big difference between the test group and the control group. It’s harder to detect lift with a small control group. If you’d like to increase the statistical power, you can either increase the reach or the control group. Both actions will give you more chances to detect lift. Conversely, with smaller reach, you’ll have a smaller holdout and less statistical power. Budget A higher budget can make for a more powerful test. Budget affects the media pressure to actually cause an effect, also called media weight. Although budget doesn’t technically affect statistical power, it does affect lift. If you spend more money, you’ll be able to expect a larger effect. If your test has a larger effect, it’s easier to detect lift. Because statistical power is the ability to detect lift, a greater budget will lead to greater statistical power. Data coverage and availability Does the platform have the ability to tie orders from all channels, such as an app, a website or offline, to impressions? Time constraints Consider whether your test duration aligns with best practices. For example, the ideal time frame for an A/B test is at least three days but no longer than 30 days. Technological constraints For example, does the platform in question have the capability to run a Randomized control trial (RCT) test, such as a lift tool? Maximize measurement validity Ensuring that your test is setup for success is a crucial step in any lift test. You may need to adjust the test and campaign parameters to maximize measurement validity. For example: ● Perform power calculations: This can maximize your chances of detecting the effect. Statistical power is a vital indicator of whether there will be enough data to report reliable results. ● Perform a preliminary analysis: Build a rudimentary MMM model with key variables. RECOMMEND MEASUREMENT SOLUTIONS 31 Perform an analysis Introduction When performing an analysis, it’s important to use the right data, matched with the right methodology. To conduct statistical and data analyses, you need to interpret statistical outputs, consider validation metrics, create data visualizations and write a simple script or query to extract or manipulate data. Identify and interpret statistical outputs. Why are these important? Statistical outputs help you to interpret the results of your analysis, while validation metrics allow you to measure the quality of the analysis. Together, they indicate how robust your data is. Consider the value of each statistical output across different media channels and platforms. Almost every output has a validation metric, so it’s important to consider both when conducting an analysis. You can distinguish the value of statistical outputs across media channels and platforms through the validation metrics. PERFORM AN ANALYSIS 33 Examples of outputs and validation metrics Review some examples of outputs and validation metrics in the graphic below. Both Statistical outputs Validation metrics Regression coefficients R-squared Slope and intercept Correlation coefficient (R) Mean, median, mode Standard errors (SE) Confidence Intervals Mean error Log-likelihood Bias and variance P-values Standard deviation (STDEV) Adjusted R-squared T-static F-static Durbin-Watson Variance inflation factor Sample size Choose an attribution model. Given the validation metrics, recommend an attribution model. Single-touch attribution models Multi-touch attribution models First click or visit Even credit Last touch Positional Data-driven attribution model Time decay PERFORM AN ANALYSIS 34 Single-touch attribution models Single-touch attribution models give credit to only one touchpoint. First click or visit What it is This model gives 100% of the credit for a conversion to the first click on the conversion path. How it’s used Use this model to better understand how to value the first click or visit in a conversion path, especially when success is defined within a longer attribution window or a longer consideration period. It gives no credit to impressions or later touchpoints that could have incremental effects on your conversion rate, and may oversimplify conversion paths that rely on middle- and lower-funnel activity. If you want to understand and credit the full conversion path, consider even-credit, positional or time-decay attribution models. How it’s calculated This model is a rules-based single-touch attribution model. It gives 100% of the credit for a conversion to the first click or visit in a conversion path. If a click and visit happen within 60 seconds of each other, then only the click is counted. Example If a conversion path contained an impression first, then a click, and then a visit, the click would receive 100% of the credit for the conversion. If a conversion path contained an impression, then a click, and then a visit 30 seconds later, the click and the visit would count as the same touchpoint and get 100% of the credit for the conversion. First click Visit PERFORM AN ANALYSIS 35 Last touch (including last click) What it is This model gives 100% of the credit for a conversion to the last click, visit, impression or view that happened in a conversion path. If there is no click or visit, then this model credits the last impression. How it’s used Use the last-touch model when you want to consider only the last touchpoints in a conversion path. For example, in a last-click attribution model, the last touch is a click. This model can help understand how to value the last touchpoint in a conversion path, especially when success is defined within a shorter attribution window or you have low-consideration conversions. This model does not give credit to earlier touchpoints that could have incremental impact, and may oversimplify conversion paths that rely on upper and middle-funnel activity like awareness and consideration. If your goal is to understand and credit the full conversion path, consider even credit, positional or time-decay attribution models. How it’s calculated This model is a rules-based single-touch attribution model. It gives 100% of the credit for a conversion to the last click or visit that happened in a conversion path. If there is no click or visit, then it will credit the last impression. If a click and a visit happen within 60 seconds of each other, only the click is credited. Example If a conversion path contained an impression first, then a click, and then a visit, the visit would receive 100% of the credit for the conversion. If the path didn’t contain a click or a visit, then the impression would receive 100% of the credit for the conversion. If a conversion path contained an impression first, then a click, and then a visit 30 seconds later, the click and the visit would count as the same touchpoint and get 100% of the credit for the conversion. Last touch Including last click PERFORM AN ANALYSIS 36 Multi-touch attribution models Multi-touch attribution models take into account more than one interaction with a given media channel. They are inclusive of other models, including even credit, positional and time decay, which are explained below. Even credit What it is This model considers the full conversion path and gives each touchpoint equal credit for a conversion, regardless of where it appeared on a conversion path or if it was an impression, click or visit. How it’s used This model helps you understand how to value the first touchpoint that introduced the product, the middle touchpoints that build consideration and the last touchpoint that helped people get to the point of conversion. Typically, even-credit models are more illustrative than actionable, since it’s unlikely that all touchpoints are equally effective. Compared to a last-touch or last-click model, even credit better reflects how all touchpoints can lead to a conversion, and can inform your business decisions. If your goal is to understand and credit the full conversion path, consider positional and time-decay attribution models. How it’s calculated This model is a rules-based multi-touch attribution model. It gives an equal credit percentage to each click, visit and impression on a conversion path. If there was one impression, three clicks and one visit on the conversion path, each one would share 20% of the credit for the conversion. Example If a click and visit happen within 60 seconds of each other, then only the click is credited. If an impression and a click appear to be associated with the same ad and happen within 24 hours of each other, then they are counted as a single touchpoint when receiving credit for a conversion. Even credit PERFORM AN ANALYSIS 37 Positional What it is This model gives a specific percentage of credit for a conversion to the first and last touchpoints in a conversion path, with the remaining credit distributed evenly across all intermediate touchpoints. This model considers the full conversion path, but gives weighted credit to the first and last touchpoints. How it’s used This model helps you understand how to value the touchpoints that occurred first and last in a conversion path. This model typically values any middle touchpoints with less credit than the first and last. Compared with a last-touch or last-click model, positional better reflects how all touchpoints helped lead to a conversion while considering the important roles that the first and last touchpoints may have played. If your goal is to understand and credit the full conversion path, you should also consider even-credit and time-decay attribution models. How it’s calculated This model is a rules-based multi-touch attribution model in which the first and last touchpoints are given a specific percentage of credit and the remaining credit is distributed evenly across all other touchpoints. The positional model is offered in two configurations, 30% and 40%, where either 30 or 40 percent of the credit is given to both the first and last touchpoints, with the remaining 40 or 20 percent of the credit distributed evenly among the remaining touchpoints Example If you choose Positional 30%, and there are five touchpoints in your conversion path, the first touchpoint will receive 30% of the credit, the last touchpoint will receive 30% of the credit and the remaining three touchpoints will each get 13% of the credit. If a click and a visit happen within 60 seconds of each other, then only the click is credited. If an impression and a click appear to be associated with the same ad and happen within 24 hours of each other, then they are counted as a single touchpoint when receiving credit for a conversion. Positional PERFORM AN ANALYSIS 38 Time decay What it is This model gives an increasing percentage of credit for a conversion to touchpoints as they get closer in time to the conversion. This model considers the full conversion path, but gives weighted credit to touchpoints as they get closer in time to a conversion. How it’s used This model helps you understand how to value the multiple touchpoints that helped lead to a conversion, but gives the most recent touchpoints more credit. Compared with a last-touch or last-click model, time decay better reflects how all touchpoints lead to a conversion in a way that may more realistically represent how customers interact with and consider ads as they get closer to converting. To understand and credit the full conversion path, consider even credit and positional attribution models. How it’s calculated This model is a rules-based multi-touch attribution model. It decreases the amount of credit given to each touchpoint by half after a set amount of time, with more credit given to the most recent touchpoints. This model is offered in two configurations, 1 day and 7 day half-life. A longer half-life leads to a more even distribution of credit over time, whereas a shorter half-life distributes a majority of credit to the most recent touchpoints. Example Choosing a one day half-life means that touchpoints that happened one day before the conversion get 50 percent of the credit, and touchpoints that happened two days before get 25 percent of the credit. Time decay PERFORM AN ANALYSIS 39 Data-driven attribution model The data-driven attribution model assigns fractional credit for a conversion to Meta touchpoints based on their estimated incremental impact. This is a statistical-based model developed by Meta and updated periodically. It has coefficients that can vary from advertiser to advertiser, industry to industry and analyst to analyst. All other models, last click, last touch, even credit, positional and time decay, are rule-based models, which follow a set of finite and transparent rules and a predetermined formula. Because this model uses learnings from actual data observations and is trained on randomized control experiments, it can more accurately measure the incremental value of your marketing efforts. This model is available on Facebook, Instagram, Audience Network and Messenger only. Conduct a statistical analysis. Based on your hypothesis, determine which statistical analyses are required: Simple linear regression Multiple linear regression Logistic regression T-Test Chi-Square Test F-Test ANOVA Correlation Given a set of coefficients, the functional form of a model and a data table, calculate the output column. See an example of how it could be calculated below. Note that this sample data is independently compiled and not the output delivered from an actual test. PERFORM AN ANALYSIS 40 Reconcile differences across different measurement solutions. Because not all measurement tools use the same methodology, you may have to reconcile results across different measurement solutions. To do that, ask yourself: ● ● ● ● ● ● ● What data input is used? Identify which events are included in the data, whether they are accurately monitored and how data is collected across devices. What time frames are used? What conversion window is applied? What attribution window is used? What measurement methodology is used? Does it use an observational method, like attribution or marketing mix modeling? Or an experimental method, like lift studies? Does it include the use of historical data or data from similar campaigns or industries? Examples of reconciling differences across measurement solutions Platforms such as YouTube or Twitter may define a video view, or thruplay, differently, so it’s important to consider what counts as a video view. Conduct a data analysis. To kick off a data analysis, make a table that breaks down summary statistics. Summary statistics give a quick and simple description of the data. They can include mean, median, mode, minimum value, maximum value, range and standard deviation. For example: The standard Ads Manager attribution window is set to 1-day view and 28-day click, but in Google Ads conversion reporting is 30 days, so attribution windows need to be reconciled before analysis. Data Data 34 56 Mean 65 112 Standard error 7.63201278 78 Median 56 65 Mode 55 44 Standard deviation 29.5586584 55 Sample variance 873.714286 75 Kurtosis -0.9432981 23 Skewness 0.18550307 110 Range 92 98 Minimum 20 97 Maximum 112 53 Sum 975 55 Count 15 20 PERFORM AN ANALYSIS 41 Calculate lift based on experiment data. Breaking down a summary statistic is the first step of most analyses. After, you can perform additional analyses, such as lift calculations. Lift results include information about the results your ads caused, including the metrics: Lift % Indicates how much your ads increased the rate at which people converted (as defined by the conversion events you chose when you created the test). For example, if your ads increased the conversion rate by 50%, that could mean that you got 100 conversions during the test without ads and 150 with them. That would mean that they got you 50 additional conversions. Lift % calculations Divide the number of additional conversions by the number of conversions you would’ve gotten without ads and multiply that by 100 to calculate Lift%. In this case, that would be: ● 50 / 100 = 0.5 ● 0.5 x 100 =50% Conversion lift The number of conversions that wouldn’t have happened without your ads. Confidence A percentage that represents how confident Meta is that your ads caused conversion lift. Results that Meta is at least 90% confident in are considered reliable. Meta’s testing methodology includes thousands of simulations based on your test. If your ads caused conversion lift in 80% of Meta’s simulations, Meta would be 80% confident that your ads caused conversion lift during the test. PERFORM AN ANALYSIS 42 Write simple script or query to extract and manipulate data. Raw data for analysis often exists in databases and other sources and needs to be retrieved. Data that exists in various databases or data tables needs to be combined and filtered to extract what is suitable to enter into an analysis. A JOIN clause combines rows from two or more tables, based on a related column between them. Inner join Returns records that have matching values in both tables. Left join Returns all records from the left table and the matched records from the right table. Right join Returns all records from the right table and the matched records from the left table Full join Returns all records when there is a match in either left or right table. Read SQL Joins for examples about how to apply the different JOINs. Inner join table 1 table 2 Left join table 1 table 2 Right join table 1 table 2 Full outer join table 1 table 2 Imagine a retail analyst has a database that contains comprehensive data for stores of all sizes from the last 5 years, but they only want to analyze data from stores in California that are larger than 500 square feet and from the last 12 months. The analyst queries a database to pull such data using SQL and then conducts some of the analysis, including visualizing the data, creating descriptive statistics and running a form of statistical analysis such as regression. An appropriate JOIN based on data structure would combine data from multiple tables. PERFORM AN ANALYSIS 43 Create data visualizations. Data visualization is a graphic representation of data. It involves producing images that communicate relationships among the represented data to viewers. This communication is achieved through the use of a systematic mapping between graphic marks and data values to create a visualization. While this is not required, it’s recommended as a good practice for analysts. Heat map Jan Feb Mar Jul Aug All Placements Feed In-stream video Explore Stories Bubble chart PERFORM AN ANALYSIS 44 Line graph Scatter plot PERFORM AN ANALYSIS 45 Bar chart PERFORM AN ANALYSIS 46 Generate insights Introduction Data-driven insights can inform the next steps in your marketing strategy. Through your analysis, you may have learned which marketing actions, campaigns and campaign strategies had a positive effect. You can use these insights to make media planning and buying decisions or decide on your future campaign strategies. Synthesize results from statistical and data analyses. After completing an experiment and analyzing marketing performance across multiple tools, it’s time to draw insights to develop media recommendations. Contextualize the results by taking the following actions: Combine campaign insights with research. Leverage all insights and research available to inform your marketing recommendations. GENERATE INSIGHTS Identify a common thread across studies, platforms and/or channels. For example, an advertiser ran a series of Conversion Lift tests against different strategies. Results showed that including video creative in addition to static creative drove incremental results across all strategies. Because of this, the advertiser considers prioritizing the development of video assets when new creative is developed. 48 Generate insights. Insights can be captured from the following sources. From clients First-party data Insights derived from a client’s efforts to learn and gather information, including campaign data in Ads Manager or results from a lift test or actual sales data and qualitative customer data. For example, an analytics team identified customers with the highest lifetime value. Testing the incrementality of a lookalike audience based on this segment showed a significant increase in incremental conversions compared with their current strategy in a multi-cell Conversion Lift test. From Meta Foresight A robust offering of studies designed to help leaders navigate cultural shifts, challenge convention and prepare for what’s next. Through meta-analysis, Meta Foresight-commissioned surveys and industry research, Meta Foresight is able to formulate three types of insights: ● People insights: trends among consumer groups across demographic interests and behaviors ● Advertising insights: information about behaviors in different digital spaces, such as messaging, video views. These insights are derived from an analysis of aggregated campaign and test results within a specific period of time, across advertisers. ● Industry insights: include vertical and market-level insights and support broader stories and pitches. From third-party resources There are a variety of free and paid online hubs where you can gather more insights and research to flesh out your story. Insights should be based on data and can incorporate a variety of dimensions, including but not limited to: Dimension Example Budget Doubling the campaign budget made ROAS less efficient. Inter-channel allocation A brand sees that overall incremental return on ad spend increases by 10% when running with a media allocation of 60% on channel A and 40% on channel B, vs. 50% on channel A and 50% on channel B. GENERATE INSIGHTS 49 Dimension Example Intra-channel allocation A brand sees that overall incremental return on ad spend increases by 10% when allocating 30% of their budget to upper-funnel campaigns, versus allocating 50% of their budget to upper-funnel campaigns. Reach Reach across both TV and Meta technologies has more impact on in-store purchases compared to reach on each platform alone. Bid strategy Utilizing cost cap drove business outcomes with the most efficient returns compared to target cost. Buying strategy ROAS was more efficient when using reach and frequency buying instead of auction buying. Audiences Targeting women ages 18-24 brought more value than targeting women ages 18-55. Note: ● Core audiences: uses information that people provide to Meta, such as interests. ● Custom audiences: uses information that the advertiser provides to Meta, such as activity from a pixel or a list of email addresses from a customer database. Placement Selling products is more effective on Instagram and Facebook combined, as compared to Facebook alone. Creative Using Creative A outperformed Creative B. Test duration Running a four-week campaign resulted in better outcomes than a two-week campaign. GENERATE INSIGHTS 50 Provide or disprove a hypothesis. Approach your research with your proven or disproven hypothesis in hand, and let that hypothesis focus your research. Interpret the significance of your test results using relevant metrics, such as: P-value The probability of obtaining test results at least as extreme as the results actually observed during the test, assuming that the null hypothesis is correct. ● Null hypothesis rejected when p < alpha ● Not rejected when p > alpha where alpha is determined by the analyst ● ● R2 ● Known as the coefficient of determination The proportion of the variance in the dependent variable that is predictable from independent variables Typically used when evaluating goodness of fit of multiple models to determine most accurate model Construct a narrative. Explain why the hypothesis was true or false by contextualizing the data, determining the caveats and acknowledging that the consumer path is complex. Stay clear on the how and why of the data, as well as the tools and research that yielded it. Evaluate the success of a measurement approach by determining whether it adequately measured your KPIs. There are issues that could come up that would prevent you from adequately measuring your KPIs, such as: ● ● ● ● Dilution during a test Not accounting for offline transactions during a Conversion Lift test Insufficient statistical power Contamination between the test and control cells of an experiment Adjust the measurement approach if the original plan failed to meet the intended measurement goals by considering either testing a new hypothesis or retesting a hypothesis. Retest a new hypothesis if the test results were contaminated, the test results were inconclusive or you want to validate the results. Example The p-value of a statistical test showed that there was insufficient evidence to reject the null hypothesis, but the analyst uncovered a data issue with the pixel that led to conversion volume being drastically lower than it should have been. During a re-test, the team needs to ensure that the proper data is flowing into the experiment so that the results are as accurate as possible. GENERATE INSIGHTS 51 Make data-driven recommendations Introduction Measurement is an iterative process. As your marketing strategy evolves, it’s important to continue to test different variables to determine which strategy is most effective at driving results. How ads appear across Meta technologies It’s important to know how the ad delivery system works so you can make effective recommendations, such as adjusting your bid strategy or targeting. Meta gives you many opportunities to show ads. The delivery system determines which ad is shown using the following three components: Ad auction ● ● ● Determines the best ad to show to a person at a given time The winning ad maximizes value for both people and businesses. Can help you understand your ad performance Performance optimization ● ● Spends your budget evenly over the schedule of your ad set. Budget and bid strategy determine pacing Advertiser controls Strategic levers to consider before launching a campaign including bid, budget, audience, creative, placement and optimization MAKE DATA-DRIVEN RECOMMENDATIONS 53 Total value To ensure that all ads are evaluated in a consistent way and the winning ad maximizes value for both people and businesses, Meta assigns a total value to every ad that competes in the auction. The total value is based on the amount you bid, how likely it is that showing your ad to a person will lead to your desired outcome and ad quality, along with how relevant your ad is to the targeted individual. The ad with the highest total value wins the auction for the targeted individual. Maximize advertiser value Advertiser bid x estimated action rates Optimize the customer experience + Ad quality = Total value Advertiser bid The amount the advertiser is willing to pay to achieve their desired outcome, such as a conversion. A bid can be the same as or less than a budget, which is the total amount of money an advertiser is willing to spend through the life of a campaign. Estimated action rates The probability that showing an ad to a person leads to the advertiser’s desired outcome. The desired outcome is aligned with the advertiser’s campaign objectives. A measure of the quality of an ad and how interesting a person will find it. Ad quality Buying types There are two main buying types for Meta ads: Auction Reach and frequency Auction buying offers more choice, efficiency and flexibility, with less predictable results. Ads can be placed across Facebook, Instagram, Messenger and Audience Network. Reach and frequency buying lets you plan and buy your campaigns in advance, with predictable ad delivery and more control over your frequency settings. MAKE DATA-DRIVEN RECOMMENDATIONS 54 Performance optimization system The performance optimization system uses machine learning to predict which ad auctions give you the best value for your money. Pacing is a budget optimization process that ensure your budget is spent as evenly as possible over the lifetime of your ad set. It helps prevent you from spending your budget too quickly on inefficient results. Pacing enables flexibility to help get you the best available results for your goals by enabling adjustments. Budget pacing Bid pacing The aspect of pacing where we may increase budget if there’s an opportunity to get many optimization events with costs aligned with your bid strategy The aspect of pacing where we adjust your bid or which auctions we enter based on how much budget and time are left for your ad set. MAKE DATA-DRIVEN RECOMMENDATIONS 55 Bid strategies There are five bid strategies to choose from. You can also choose not to enter a cost control. Highest volume Select the highest volume bid strategy if you want to maximize delivery and conversions you can get from your budget. This bid strategy is best for spending your budget as efficiently as possible. However, the cost of your ad results will fluctuate more, as changes are made to get the highest volume. For example, if auction competition decreases, the cost of your ads may go down and if auction competition increases, the costs of your ads may go up. Highest volume optimized for value Select the highest volume optimized for value bid strategy if you want to spend the entire budget by the end of the schedule of an ad set while maximizing the amount of value you get from purchases. This automated bid strategy is available for ad sets optimizing for purchase value. Value optimization uses machine learning to predict how much ROAS you may generate for a business over a one- or 7-day window. This prediction is then used to bid for your highest value customers. By bidding more for people who are likely to spend more, you can maximize the ROAS for your campaigns. Cost per result goal Select the cost per result goal bid strategy if you want to maximize cost-efficiency and need to keep cost within a specific threshold. This bid strategy enables you to provide a benchmark cost for the results you care about. This helps limit your cost per conversion while maximizing the number of conversions. The cost per result goal reflects how much you are paying on average for results. Not all optimization goals are available for cost per result goal. Learn more about best practices for using cost per result goal. ROAS goal Select the ROAS goal bid strategy if you want to keep return on ad spend around an average amount of the course of your campaign, providing you with more command over the value a campaign brings to your business. When you set a ROAS goal, we’ll try to deliver against that over the campaign’s lifetime, dynamically bidding as high as needed to maximize results. To use this bid strategy, you'll need to optimize your ad set for purchase value. Bid cap Select the bid-cap bid strategy if you want to set