Linear Regression
Document Details
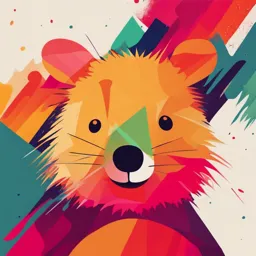
Uploaded by ClearerKoala
2013
Ani Katchova
Tags
Summary
This document provides a lecture or presentation on linear regression, including examples and calculations. It details the linear regression model, estimated regression line, and coefficient interpretations.
Full Transcript
Linear Regression Ani Katchova © 2013 by Ani Katchova. All rights reserved. 2 Linear Regression Overview  Linear regression examples  Linear regression model  Estimated regression line  Single versus multiple regression  Coefficients and marginal effects  Goodness...
Linear Regression Ani Katchova © 2013 by Ani Katchova. All rights reserved. 2 Linear Regression Overview  Linear regression examples  Linear regression model  Estimated regression line  Single versus multiple regression  Coefficients and marginal effects  Goodness of fit (R-squared)  Hypothesis testing for coefficient significance o t-test for a single coefficient significance o F-test for multiple coefficients significance 3 Linear Regression Linear regression examples  Explain student grades using the number of hours studied  Explain the effect of education on income  Explain the effect of the number of bedrooms on house prices  Explain the effect of the recession on stock prices Linear regression set up  Regression analysis does not establish a cause-and-effect relationship, just that there is a relationship.  The cause-and-effect relationship must be determined using a theoretical model or a logical reason.  The dependent variable is a continuous variable.  The independent variables can take any form - continuous or discrete or indicator variables.  The simple linear regression model has one independent variable.  The multiple linear regression model has two or more independent variables. 4 Linear regression model Linear regression model  The linear regression model describes how the dependent variable is related to the independent variable(s) and the error term: U L? 4 E? 5T5 EQ or U LT′? EQ  y is the dependent variable (explained, predicted, or response variable)  x is the independent variables (control variables or regressors)  ? are unknown parameters to be estimated o ? 4 is the intercept o ? 5 is the slope  u is the error term or disturbance 5 Estimated regression equation  The estimated regression equation shows how to calculate predicted values of the dependent variable using the values of the independent variable(s). U ? L> 4 E> 5T5 LT′>  Interpretation of the coefficients: one unit increase in x will increase the dependent variable y by > 5 units.  Note that there is no error term when we predict the value of the depended variable.  Regression residuals are calculated as the difference between the actual and predicted values of the dependent variable: Q LU FU ? LU F> 4 F> 5T5 LU FT′> 6 Simple linear regression examples Regression line x = number of credit cards y = dollars spent For each additional credit card, a person spends $100 more. The equation for the line is U ? L> 4 E> 5T5 L 0 E 100T 5 intercept = b 0 = 0 (when T 5=0, then U ? L> 4) slope = b 1 = 100 (when T 5 increases by 1, then U ? increases by b 1) 0 100 200 300 400 01234 7 Regression line, new example with a positive intercept The equation for the line is U ? L> 4 E> 5T5 L 400 E 100T 5 intercept = b 0 = 400 (when T 5=0, then U ? L> 4) slope = b 1 = 100 (when T 5 increases by 1, then U ? increases by b 1) For each additional credit card, a person spends $100 more. 0 100 200 300 400 500 600 700 800 01234 8 Regression error The error is the difference between the actual values and the predicted values of the dependent variable: Q LU FU ? LU F> 4 F> 5T5 9 Variations: total variation, explained variation and unexplained variation ?:U F U $; 6 L ?:U ? FU $; 6 E ?:U FU ?; 6 Total variation = explained variation due to regression + unexplained variation due to error sum of squares total = sum of squares due to regression + sum of squares due to error SST= SSR+SSE 10 The least squares method (OLS: ordinary least squares)  The least squares method is used to calculate the coefficients so that the errors are as small as possible.  We minimize the sum of squared residuals: ?Q 6 L ?:U FU ?; 6 L ?:U F> 4 F> 5T; 6  In a simple linear regression the coefficients are calculated as: > 5 L?KR: T, U; R=N:T; > 4 LU $ F> 5T̅ 11 OLS regression in matrix form  The regression line is specified as: ': U| T; LT ′? L? 4 E? 5T5 E? 6T6 E⋯ E? ?T?  Marginal effects in the linear regression model are the coefficients. ?': U| T; ?T ? L? ?  In multiple linear regression, the coefficients are calculated as: > L kT ′T o ?5 :T ′U;  Assumptions of the OLS estimator: o Exogeneity of regressors o Homoscedasticity o Uncorrelated observations 12 Goodness of fit R-squared  The coefficient of determination (R-squared or R 2) provides a measure of the goodness of fit for the estimated regression equation.  R 2 = SSR/SST = 1 – SSE/SST  Values of R 2 close to 1 indicate perfect fit, values close to zero indicate poor fit.  R 2 that is greater than 0.25 is considered good in the economics field.  R-squared interpretation: if R-squared=0.8 then 80% of the variation is explained by the regression and the rest is due to error. So, we have a good fit. Adjusted R-squared  Problem: R 2 always increases when a new independent variable is added. This is because the SST is still the same but the SSE declines and SSR increases.  Adjusted R-squared corrects for the number of independent variables and is preferred to R- squared. 4 ?6 L1 F:1 F4 6;J F1 J FL F1  where p is the number of independent variables, and n is the number of observations. 13 t-test for significance of one coefficient  The t-test is used to determine whether the relationship between y and x j is significant. * 4: ? ? L0 * ?: ? ? M0  The null hypothesis is that the coefficient is not significantly different than zero.  The alternative hypothesis is that the coefficient is significantly different from zero.  We use the t-distribution: o The test statistic t = coefficient /standard error o The critical values are from the t distribution o The test is a two-tailed test.  Reject the null hypothesis and conclude that coefficient is significantly different from zero if: o The test statistic t is in the critical rejection zone o The p-value is less than 0.05  The goal is to find coefficients that are significant. -3 -2 -1 0 1 2 3 14 F-test for overall significance of all coefficients  Testing whether the relationship between y and all x variables is significant.  The null hypothesis is that the coefficients are not jointly significantly different from zero.  The alternative hypothesis is that the coefficients are jointly significantly different from zero. * 4: ? 5 L? 6 L⋯ L? ? L0 * ?: ? 5 M0 KN ? 6 M0 KN… ? ? M0  Use the F-distribution o The test statistic F = MSR/MSE o The critical values are from the F distribution o The F-test is an upper one-tail test 15 ANOVA table Total variation = explained variation due to regression + unexplained variation due to error Source Sum of Squares Degrees of Freedom Mean Square F-statistic Regression SSR=∑(U ? FU $; 2 p = number of independent variables MSR=SSR/p F=MSR/MSE Error SSE=∑(U FU ?) 2 n-p-1 MSE=SSE/(n-p-1) To t a l SST=∑(U FU $) 2 n-1 (n=number of observations)  Find critical values in the F table (significance level =0.05) o degrees of freedom in the numerator = number of independent variables = p o degrees of freedom in the denominator = n-p-1  Reject the null hypothesis if the F-test statistic is greater than the F-critical value.  Reject the null hypothesis if the p-value is less than 0.05.  The goal is to find a regression model with coefficients that are jointly significant.