Lesson 4 Part 2.pdf
Document Details
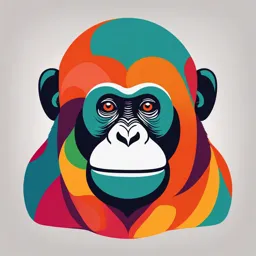
Uploaded by CooperativeJacksonville
Nanyang Technological University
Tags
Related
- Chapter 7 - 04 - Understand Different Types of IDS-IPS and their Role - 05_ocred_fax_ocred.pdf
- Lesson 4 Part 1.pdf
- Lesson 4 Part 1.pdf
- Lesson 4 Part 2.pdf
- Western Governors University_ Certified Ethical Hacker (CEH) Version 12 eBook w_ iLabs (Volumes 1 through 4).pdf
- Psychoanalytic Diagnosis: Understanding Personality Structure
Full Transcript
Lesson 4 Part 2 When you conduct anomaly detection it's important to calculate the average activity for each user and remember that no user's activity will be the same and therefore you need to work out every user's baseline. So next we calculate the standard deviation. So for each month we use the...
Lesson 4 Part 2 When you conduct anomaly detection it's important to calculate the average activity for each user and remember that no user's activity will be the same and therefore you need to work out every user's baseline. So next we calculate the standard deviation. So for each month we use the number of job searches minus the mean and then square it. We then divide by the number of months and square root the value to get the standard deviation. Setting thresholds for anomaly detection can help reduce false positive rates and the upper threshold is usually two times the standard deviation, however this can be adjusted depending on how sensitive the detections need to be. A lower threshold can also be used if activity is below the user's average and you have concerns about that also. We can use other data to correlate with this anomaly to proactively determine if Bob could be a potential insider threat. So for example we can correlate Bob's job search anomalies with other anomalies in emails that Bob has sent externally and a combination of the two indicators can allow us to proactively detect if Bob is going to leave the organization and if he's a potential insider threat and may attempt to exfiltrate business sensitive data before he leaves. Anomaly detection can be very resource intensive as you must calculate per user every user and every user will behave differently and businesses do not always have their log sources centralized into a single sim which can make anomaly detection difficult. Anomaly detection requires having historical user activity data to be able to accurately detect anomalies but there are some tools and products in market that can actually do this for you. Most user behavior analytics or UBA tools available on the market use a form of anomaly detection using standard deviation to detect changes in user behavior and sim systems such as Splunk have apps such as the machine learning toolkit or MLK available as well as built-in standard deviation functions to conduct anomalies or outlier detection. Now anomalies and other indicators alone do not always show the full picture of an insider threat incident and correlating various data sources through sequence detection can help provide a bigger picture of what activity has occurred and the potential threat and sequence detection involves grouping together anomalies or activities that could indicate a certain activity. So for instance a data exfiltration sequence event could involve a user downloading a sensitive file followed by renaming that file and then sending that file to their personal email. Sequence detections can occur over a specified time frame and don't always occur one after another. A user could download a sensitive file then rename it and send out five days later to their personal email account. But what we can do is link the various activities together to create a sequence of events that can alert us to the potential insider threat and this is especially useful when the activities are spread across a longer period and it's harder to detect. So combining anomaly detection and sequence detection and other insider threat indicators can create a full picture of a user's activity and the potential threats that they may pose to an organisation.