Lectures 1-4 Summary Biostatistics PDF
Document Details
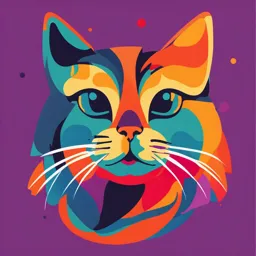
Uploaded by FieryBodhran
European University Cyprus
2024
Dania Yassin
Tags
Summary
This document provides a summary of lectures 1-4 on biostatistics. It covers fundamental concepts like data collection, different variable types, and descriptive measures. The lectures discuss common statistical methods and distributions.
Full Transcript
Lectures 1-4 summary Biostatistics – Dania Yassin Med1 8 November 2024 Biostatistics deals with collection, classification , analysis and interpretation of data from biomedical research. It helps in generating medical knowledge Science is empirical : base...
Lectures 1-4 summary Biostatistics – Dania Yassin Med1 8 November 2024 Biostatistics deals with collection, classification , analysis and interpretation of data from biomedical research. It helps in generating medical knowledge Science is empirical : based on observation and experience natural & experimental observations inductive reasoning generalization Basic Research Clinical Research They are interconnected The clinical research must be randomized , to ensure unbiased results. In biostatistics we study samples , a sample is a subset of a population. BUT THE SAMPLE IS NOT OUR INTEREST ! We study a sample to infer about a population of interest. The Larger our sample is the more likely for our results to be true if our sample was small and biased results may not reflect the population , with a higher chance of random error. Random error (or sampling error) Any difference between sample mean and population mean that is attributable to the sampling Sample quantities are known and are being measured ( e.g. sample mean ) , Population quantities are unknown and are being estimated (e.g. population mean ). Assuming unbiased samples and accurate measurements , we will be able to convert our data into meaningful results. Types Of Variables Categorical Numeric 1. Nominal Variable 1. Continuous without inherent ordering (e.g. blood have units of measurements type, sex, race, occupation…) ( e.g. temperature ) & can convert to other units of 2. Ordinal Variable measurement with inherent ordering (e.g. educational level, satisfaction level…) 2. Discrete count of things , no units of 3. Dichotomous measurements (e.g. nb of children , with just two levels ( diseased/healthy, nb of asthma attacks … ) yes/no , vaccinated/non-vaccinated ). Frequency Tables Present the number of participants (units of observation) in each category Relative frequency table: percentage of participants in each category Appropriate for categorical variables (and grouped numeric - such as “age group”) For ordinal variables it’s the same – we just adhere to the ordering In addition, we can cumulate frequencies We can even tabulate numeric discrete variables, provided the number of categories is small And also cumulate (since numbers have ordering Contingency tables (cross-tabulations) Examine the association between two categorical variables marginal totals and category-specific proportions should be similar if variables NOT associated In medicine we usually deal with contingency tables between exposure and outcome (disease) Plotting Categorical Variables, we use Pie Chart Bar plot 6 5 4 3 2 1 0 1st Qtr 2nd Qtr Series 1 Series 2 Series 3 3rd Qtr 4th Qtr A better way to illustrate categorical Illustrate relative frequencies (up to 100%) variables Require few categories, relatively large Can be stacked, proportional, horizontal differences −→ use sparingly or vertical, etc Color gradient often used to indicate ordering, vs different colors for nominal variables Bars should be same width, with space in- between Plotting numeric variables, we use: Right Skewed Histogram Same bin width, NO space in between (vs. bar plot) Midhinge The median. To show that this is a continuous numeric variable Hinges The 1st and 3rd quartiles Symmetric vs skewed distribution If symmetric, mean = median. Otherwise Whiskers Usually 1.5x IQR (also: range, mean is “pulled” towards the skew 2nd/98th quantile, etc) Outliers Any values further from the whiskers Measures of location Mean = Average The sum of the values divided by the number of values: ∑x / n Sensitive to skewness and outlier values! Median Splits the values in the middle – into a lower and a higher half More robust against skewness and outliers Quantile Splits the values by a certain proportion e.g. 10th percentile is the value that separates the lower 10% from the higher 90% Median is the 50% percentile “Quartiles”: 1st (25%), 2nd (50%), 3rd (75%) Mode The most frequent value in the variable Measures of spread Variance The “expectation” (average) of the squared deviation of a variable from its mean Standard deviation The square root of the variance Range The difference between the highest and lowest value Interquartile range (IQR) The difference between the 3rd (75%) and 1st (25%) quartile e.g. 10th percentile is the value that separates the lower 10% from the higher 90% Distribution as a Concept If we take a single baby... what would be its most likely weight ? Frequency distributions for a set of values (=sample or population) generalize to probability distributions for an individual Density plots – total area under the curve is equal to 1 (100% probability) Population vs sample Every numeric variable in a population (e.g. body weight) has a frequency distribution across the population Therefore is has a population mean and a population variance. These are symbolized µ and σ2 respectively, and are unknown If we take a sample of the population, the same variable will also have a frequency distribution across the sample Therefore is also has a sample _ mean 2 and a sample variance These are symbolized X and S respectively, and are known In fact we’ll be using them to estimate µ and σ 2 Describing Numerical Variables Median and quantiles are more appropriate for skewed / non-normal data (though not exclusively) Distributions Probability distribution A mathematical function f(x) = P(X = x) giving the probabilities of occurrence of different possible values in a “random variable” X Random variable = a variable whose values depend on outcomes of a random phenomenon or experiment “Matches” a certain probability to each possible value of the random variable Some probability distributions are “standard” functions, described by certain parameters e.g. the Normal distribution, the Binomial distribution, the Poisson distribution ALL probability distributions can be empirically described by the same measures that we use for frequency distributions: mean, SD, median & quantiles, cumulative probabilities, etc The Normal distribution: N(µ, σ) Described by just two parameters: µand σ “Standardize” a normally-distributed variable, by subtracting its mean and dividing by its SD: convert to a z-score Show where a value is placed in the Standard Normal distribution __ X−µ σ = Z ~ N(0,1) A symmetric unimodal distribution: mean = median = mode = 0 For any range of values, we can calculate its probability of occurrence (area under the curve) From −∞ to ∞: P = 1 68% of the probability is included between −1σ and 1σ 95% of the probability is included between −1.96σ and 1.96σ 99.7% of the probability is included between −3σ and 3σ Does my variable follow a normal distribution? 1. Contextual knowledge Variables such as height, weight, blood pressure, most physiological measurements, course grades, etc (Note disease may skew the distribution; although it may itself be defined in terms of the normal distribution) Shapiro-Wilk test A statistic is calculated that has an associated p-value; if p < 0.05, this indicates a deviation from normality Q-Q plots (Q as in Quantile) If variable follows normal distribution, the points should lie on the diagonal line y = x ( straight line ) Why is the Normal distribution important? 1. Many variables follow a normal distribution 2. The “central limit theorem” Central limit theorem: The means of repeated random samples from any distribution, will follow a normal distribution — even if the underlying variable is NOT normally distributed in the population The standard deviation (SD) of this distribution of sample means, is called the standard error (SE) The smaller our SE the closer our sample mean to the population mean The Three Distributions 1. Distribution of a characteristic in the population Mean µ, standard deviation σ Unknown, we are interested in estimating them (particularly µ) 2. Distribution of a variable in the sample Mean ¯x, standard deviation s Known quantities from the sample 3. Distribution of the sample mean of the variable Mean µ, standard error (SE) σ/√ n We estimate this by: mean ¯x, standard error (SE) s/ √ n 95% Confidence Interval If we take one random sample from a population, It will have a mean X However If we randomly draw more than one sample from the population , _ _ _ We will obtain different means : X1,X2,X3… & X=X=X We will notice that these means lie in a specific range Now it is very useful X2 to know the range in This range is X1 ________ _________ which the true value called X3 _________ confidence of µ will lie with high probability interval 95% Confidence Interval __ CI = X +- Z*SE WHERE: __ X : mean value Z-value : for confidence level of 95% SE : standard error The z-value is 1.96 Z : z-value for CI This applies to large samples The confidence interval can of course be however... calculated for other parameters not only mean , it only says in which range the For smaller samples there is some parameter lies with certain probability uncertainty (imprecision) about the (e.g. 95% ) sample Standard Deviation s, and thus the Standard Error s/ √ n. Therefore we use the t-distribution, with n − 1 “degrees of freedom” The “one-sample t-test”, to determine the 95% CI for a mean Assumptions of the t-test Both samples are from a normally distributed population If in doubt, you may test normality (Q-Q plots, Shapiro-Wilk test) Both samples are independent Both have the same Standard Deviation in the population If these assumptions do not hold:... then we can perform a non-parametric test called the Wilcoxon-Mann-Whitney (or Mann- Whitney) test Assesses whether the two samples come from the same distribution Non-parametric = it does not assume normality of the samples The Mann-Whitney test is the non-parametric “brother” of the two-sample t-test Comparing two (sub-)sample means Sample means will be different, even by little Two possible cases: Both population means are the same, µ1 = µ2, and any difference in sample means is due to random error This is called the null hypothesis (H0) Population means are actually different µ1 ̸= µ2, and that is the cause of the difference in sample means This is called the alternative hypothesis (H1) We need to decide between the two... The difference between two population means: d = µ1 − µ2 d = 0 means NO difference. WHAT IS THE P-VALUE ? Represents the probability of observing the obtained results ( or more extreme ) if the null hypothesis is true. P0.05 ( high probability of observing the obtained results ) then, The results are likely to be seen There is no statistically significant difference between population means Hence , we failed to reject the null hypothesis ( we never accept the null hypothesis , instead we can say that we failed to reject it or we can say that there is statistically significant difference but our data cannot confirm it ) CI does include the null value of zero What is a proportion ? Ratio = X/Y comparing two unrelated but similar quantities (same units). Numerator not included in the denominator! Proportion = A/(A + B) Number of individuals (or other things) meeting a criterion / total number of individuals (or other things) A proportion includes the numerator into the denominator A proportion ranges from (is bounded between) 0 to 1 We cannot use the Normal or t-distributions to analyze proportions We often use proportions to represent frequency of diseases, especially existing cases of disease (=prevalence) The prevalence of type 2 diabetes (T2DM) in the US is 11.3% The prevalence of MS in Italy is 140 cases per 100,000 population The Binomial Distribution A discrete probability distribution, that gives us the probability of getting a certain number of successes (x) out of n independent trials, each having probability of success p Two parameters: n and p If I take a random sample of 50 Americans, what is the chance that no more than 10 of them will have TD2M? (p = 0.113, n = 50, x ≤ 10) Probability distribution f(x) = P(X = x) A function matching a probability of occurrence to each possible value x of a random variable X The binomial distribution assigns a probability to each But note: “success” in our value x (number of “successes” in a binary experiment) if context often means “disease”. we know p (and n) The Binomial distribution is a skewed distribution