Exploratory Data Analysis (EDA) Lecture 4 PDF
Document Details
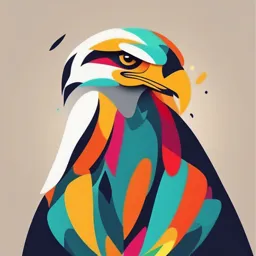
Uploaded by TemptingMorganite
King Saud University
Dr. Asma Abahussin
Tags
Summary
This document is a lecture on Exploratory Data Analysis (EDA). It covers univariate and multivariate methods, both graphical and non-graphical. The content of the lecture details the importance of EDA, variable characteristics, and distribution types. Examples of visualization techniques, including histograms, boxplots, scatterplots, and line charts, are presented.
Full Transcript
BMT 443 Exploratory Data Analysis (EDA) Lecture 4 Dr. Asma Abahussin Department of Biomedical Technology College of Applied Medical Sciences King Saud University Objectives To learn and understand: ▪ What is meant by EDA and why it is important? ▪ What sort of dataset’s characteristic...
BMT 443 Exploratory Data Analysis (EDA) Lecture 4 Dr. Asma Abahussin Department of Biomedical Technology College of Applied Medical Sciences King Saud University Objectives To learn and understand: ▪ What is meant by EDA and why it is important? ▪ What sort of dataset’s characteristics are important to explore? ▪ Methods of conducting EDA and how they are classified. 2 Introduction ❖ After preprocessing and cleaning the data, data scientists need a high level of understanding of the information that is contained in it. ❖They achieve that by asking questions such as: ▪ What are the obvious correlations or trends within the data? ▪ What high-level characteristics does it have? ▪ Are there any of them that are more important than the other? 3 EDA ❖ EDA is a process of examining and understanding data using multiple techniques to abstract core characteristics and facilitate further analysis and decision-making. ❖ Examining data implies: ✓ Assessing the quality of the data ✓ Identifying patterns, relationships, and trends in the data. ✓ Identifying important variables ✓ Testing underlying assumptions 4 EDA methods EDA methods can be cross-classified as: ▪ Univariate or multivariate methods Univariate methods look at one variable at a time, while multivariate methods look at two or more variables at a time to explore relationships - Usually multivariate EDA is bivariate in data science. ▪ Graphical (data visualization) or non-graphical (statistics) methods Non-graphical methods generally involve the calculation of summary statistics to provide insight into the characteristics and the distribution of the variable(s) of interest, while graphical methods obviously summarize the data in a diagrammatic or pictorial way. Why do data scientists care about the variable distribution? 5 Types of data distribution ❖ A distribution of data item values may be symmetrical such as normal distribution or asymmetrical such as skewed distribution. Normal distribution Skewed distribution 6 Univariate graphical EDA (univariate data visualization) ❖ It employs a variety of graphs to gain insight into a single variable’s distribution. ❖ Graphs enable data scientists to gain a quick understanding of shapes, central tendencies, spreads, skewnesses, and outliers of the data they are studying. ❖ The most commonly used univariate graphical EDA techniques are: ▪ Histogram and Boxplots, mainly for quantitative variables ▪ Bar and pie charts, mainly for categorical variables 7 Univariate graphical EDA (univariate data visualization) Boxplot chart Histogram chart Bar chart Pie chart 8 Univariate non-graphical EDA ❖It is a simple method for examining information that utilizes only one variable. ❖ It focuses on figuring out the underlying distribution or pattern in the data and mentions facts about the variables. ❖ For quantitative variables, EDA includes the examination of the attributes of the distribution: ▪ spread (standard deviation and variance) ▪ central tendency (mean, median and mode) ▪ skewness (a measure of the asymmetry of a distribution) ▪ kurtosis (a measure of the tailedness of a distribution). 9 Univariate non-graphical EDA ❖ For categorical variables, EDA includes a simple tabulation of the frequency of each category. ▪ The characteristics of interest for a categorical variable are simply the range of values and the frequency of occurrence for each value. Example of tabulation 10 Multivariate graphical EDA (multivariate data visualization) ❖ It displays relationships between two or more variables using graphics providing a more comprehensive understanding of the data. ❖The most commonly used multivariate graphical techniques: ▪ scatterplots and line charts, mainly for quantitative variables ▪ side-by-side boxplot, mainly for one categorical and one quantitative variables ▪ Stacked bars, mainly for categorical variables 11 Multivariate graphical EDA (multivariate data visualization) Scatterplot Line chart side-by-side Boxplot Stacked bars chart 12 Multivariate non-graphical EDA ❖ It is a technique used to explore the relationship between two or more variables through either: ❖ cross-tabulation for mainly categorical variables ❖ or statistics and computing covariance and correlation which measure the degree of the relationship between two random variables and express how much they change together. Example of cross-tabulation 13 Which of the EDA methods to use? ❖ Data scientists perform whatever EDA methods and steps are necessary to become more familiar with the dataset of interest and learn about variable distributions, and relationships between variables. ❖ Non-graphical and graphical methods complement each other. While the non-graphical methods are quantitative and objective, they do not give a full picture of the data; therefore, graphical methods, which are more qualitative and involve a degree of subjective analysis, are also required. ❖ Univariate methods help to understand one variable’s characteristics, while Multivariate methods help to understand how this variable relates to other variables in the dataset. 14