Statistics and Epidemiology Lecture 13 PDF
Document Details
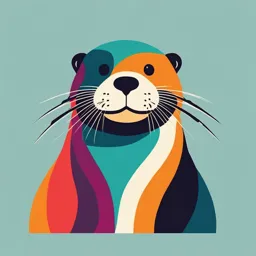
Uploaded by ForemostTheme2043
Rutgers University
Tags
Summary
This lecture covers fundamental concepts in statistics and epidemiology, focusing on the detection of infectious agents, different types of tests, and various study designs. It explains concepts like surrogate markers, prevalence, test sensitivity and specificity, and the difference between screening and confirmatory tests.
Full Transcript
Statistics and Epidemiology Applying statistics and epidemiology to evaluate published literature General Approaches to the Detection of Infectious Agents Surrogate markers Ex. Immune response to the agent or a clinical consequence of the infection. Presence of antibodies indicates: a....
Statistics and Epidemiology Applying statistics and epidemiology to evaluate published literature General Approaches to the Detection of Infectious Agents Surrogate markers Ex. Immune response to the agent or a clinical consequence of the infection. Presence of antibodies indicates: a. there was exposure to antigen from the infectious agent, but no live infection existed. b. there was active infection in the past that has resolved c. there is a latent infection; or d. there is an active infection. Persistence- the presence of antibodies does not indicate resolution of infection. Ex. Presence of anti-HIV antibodies = persistent infection; no resolution of past infection. Prevalence: This refers to how common a disease is in a specific population. High prevalence means many people have the disease, while low prevalence means few people do. Epidemologic assessment of tests: Definitions Sensitivity —The probability that a test result will be positive if infection is present. The ratio of (true positives)/(all those with infection). Testing how sensitive the assay is. The ability of a test to correctly identify individuals who have the disease (true positives) Specificity —The probability that a test result will be negative if infection is not present The ratio of (true negatives)/(all those without infection). The ability of a test to correctly identify individuals who do not have the disease (true negatives) Positive Predictive Value —The probability of infection being present given a positive test result, The ratio of (true positives)/(true positives + false positives) (equivalently: (true positives)/(all those with a positive t est result)). This is the probability that individuals who receive a positive test result actually have the disease. A low PPV means that many people who test positive might not actually have the disease. Low Prevalence and PPV: Conversely, in a low prevalence setting (where few individuals have the disease), even a test that is reasonably accurate might have a low PPV. This happens because if most people in the population do not have the disease, many of those who test positive may be false positives. Negative Predictive Value —The probability of infection not being present given a negative test result The ratio of (true negatives)/(true negatives + false negatives) (equivalently: (true negatives)/(all those with a negatives test result)). This is the probability that individuals who receive a negative test result truly do not have the disease. A high NPV indicates that the test is reliable for ruling out the disease. High Prevalence and NPV: The text suggests that in situations of high prevalence (when many individuals have the disease), the NPV of the test might not be as high. This is because even if a test is good at identifying negatives, if a lot of people actually have the disease, there could still be a significant number of false negatives. Epidemologic assessment of tests: Definitions Gold Standard (Reference Standard) —A definitive means of categorization, widely accepted by experts in the field, for absolutely defining the presence or absence of a condition (such as HIV infection). Silver Standard (Criterion Standard) —The best currently available (or the accepted) standard, which is expected to be superseded as technology advances; used as an interim reference standard when a gold standard either does not exist or is otherwise unavailable. Supplementary Test —An additional test that further clarifies the result of a screening assay. Does not necessarily have truly independent qualities. Usually used as part of a sequence of tests, which may be referred to as a “testing algorithm.” Confirmatory Test —A supplementary test that is maximally independent from any other tests that have been utilized. A well-performing confirmatory test will be part of a “confirmatory algorithm,” the results of which would serve as the basis for optimally definitive test result categorization. Screening vs. Confirmatory testing Screening tests and confirmation tests serve different purposes in the diagnostic process, especially in the context of infectious diseases. Screening Tests Purpose: Screening tests are designed to identify individuals who may have a disease but are asymptomatic or have not yet been diagnosed. The goal is to detect cases early, allowing for timely intervention. To identify potential cases of disease in a large population. Characteristics High Sensitivity: Screening tests aim to maximize sensitivity to catch as many true positive cases as possible. This reduces the chances of missing someone who has the disease. Cost-Effective and Quick: They are often easier, faster, and cheaper to administer, making them suitable for widespread use. Example: A rapid HIV antigen test is often used as a screening tool. It quickly identifies individuals who may be infected with HIV, prompting further testing. Confirmation tests Purpose: Confirmation tests are used to verify the results of a screening test. If a screening test indicates that a person may have a disease, a confirmation test provides a definitive diagnosis. To provide a definitive diagnosis after a positive screening result. Characteristics High Specificity: Confirmation tests prioritize specificity, aiming to correctly identify individuals who do not have the disease, thus minimizing false positives. More Complex: These tests are usually more complex, time-consuming, and may be more expensive than screening tests. Example: After a positive result from a rapid HIV test, a confirmatory test like a Western blot or an HIV RNA test is performed to ensure the diagnosis is accurate. Descriptive Analysis Think of any factor as a variable. What are the variables? What is the shape of their distribution? examine each of your outcome variable(s) and see how they relate to (depend on) the “independent” variables (“predictors”). Two Major Variable types 1. Continuous numeric Sometimes you will break a continuous variable, such as age, into categories. Examples:- Adults: Young, middle-aged and old persons. Each group can differ greatly. 2. Categorical [which can either be ordered or not] For some categorical variables, there are “standard” constructed scales you’ll tend to use as a continuous variable: Example: the APACHE II scale is used in many clinical studies P-Values P-value The probability that a test statistic would be as extreme as, or more extreme, than observed. Unimodel Curve representing entire test if the null hypothesis (= NO relationship) is TRUE. P< 0.5 = usual standard Typically using two-tailed hypothesis (uni- model curve; tail graph shape at the start and.95 beginning). Each extreme of the “tails” represent 0.25 p- value Acceptance region is in the center. Null hypothesis rejection region is at the tails. (100% = 5% p-value (0.25 +0.25) + 95% Confidence Interval) 95% Confidence Intervals These surround, and are computed from, the observed RR (Relative Risk) or OR (Odds Ratio). CI’s are “random” estimates because the observed RR or OR is from a random sample. The (random) 95% CI has a 95% chance of including the “true” (unknown) RR or OR. Does the CI include the value 1? An odds ratio < 1.0 is “protective” (decreased risk). An odds ratio > 1.0 is “increased risk.” IF the CI includes 1.0 > then it includes NO EFFECT > equivalent to the NULL hypothesis being true > then there is NOT statistical significance! You expect to see an agreement between the P-value and CI with respect to statistical significance. 2x2 Contingency Tables A contingency table, also known as a cross-tabulation or crosstab, is a statistical tool used to summarize and analyze the relationship between two or more categorical variables. It organizes data into rows and columns, allowing for easy comparison of the frequencies or counts of different categories. Chi-Square Test: One common analysis performed using contingency tables is the chi-square test, which evaluates whether there is a significant association between the two categorical variables Ex. Two categorical variables would be comparing smoker status (smokers vs non-smokers) and disease presence (disease vs. no disease) in a given population. Major Types of Study Designs These differ in size, cost, and appropriate interpretations and inferences that can be legitimately made. 1. Retrospective a research approach that examines existing data from past events or outcomes. This type of study is particularly useful in si tuations where researchers want to investigate associations or effects that have already occurred. Biases: Recall bias in studies relying on participant memory (e.g., surveys), there may be inaccuracies in self-reported data. Selective loss of some subjects (e.g., those that have died) Ex. women who died quickly after receiving “bone-marrow transplants” for breast cancer would no longer be alive for study. Limitation: can only use whatever data had been collected and can be found. 2. Prospective a research approach where participants are followed over time to observe outcomes that occur after the exposure or intervention has been identified. This design is commonly used in clinical and epidemiological research to establish relationships between exposures (such as risk factors or treatments) and outcomes (such as disease incidence). Biases: selective loss of some subjects (e.g., those that are lost to follow up, due to illness/death or relocation) 3. Case Report Single case A case report is a detailed account of a single patient’s medical history, symptoms, diagnosis, treatment, and outcomes. It provides an in-depth narrative of a unique or interesting clinical situation. 4. Case Series More than one case A case series is a collection of case reports involving multiple patients with similar characteristics or conditions. It pres ents data on a group of patients rather than focusing on an individual Major Types of Study Designs 5. Cross-sectional a type of observational research that examines a population at a single point in time. This design is often used in public health and social sciences to assess the prevalence of an outcome or to explore relationships between variables. 6. Cohort a type of observational study where researchers follow a group of individuals (a cohort) over time to assess the relationship between exposures (such as risk factors or interventions) and outcomes (such as disease development or health status). This design can be either prospective or retrospective. 7. Case Control an observational study that compares individuals with a specific outcome or disease (cases) to individuals without that outcome or disease (controls) to identify and assess the relationship between exposures or risk factors and the outcome. 8. Randomized Clinical Trial (RCT) an experimental study design in which participants are randomly assigned to receive either the intervention being tested or a control (such as a placebo) to evaluate the efficacy and safety of the intervention. Double blinded: neither the patient nor the physician know who is getting what. Causality causality refers to the determination that a specific exposure or risk factor is responsible for or significantly contributes to the occurrence of a disease or health outcome. Factors such as latency now make causation complex. Cohort and RCT = gold standard Confounding Variable An “extra” variable that was not accounted for. This variable can ruin an experiment and yield useless results. A confounding variable can have a hidden effect on experimental outcome. Intention to Treat A principle in clinical trials that involves including all participants in the groups to which they were randomly assigned, regardless of whether they completed the study, adhered to the treatment, or were lost to follow-up. This approach helps to preserve the benefits of randomization, reduces bias, and provides a more realistic estimate of the treatment effect in the general population. Ex: Drug Trials: In a study evaluating a new hypertension medication, participants are randomly assigned to either the drug or placebo. If a participant in the drug group drops out due to side effects but is still analyzed as part of the drug group, this preserves the randomization and reflects real- world scenarios. Statistics and Epidemiology Evaluating literature in Covid-19 and SARS CoV2 Vaccination and masking Stats- Summary Data and science are continually evolving. Thus, it is critical for you to be able to judge the state of the science, its limitations, and be willing to alter your opinions when warranted. MANY studies have limitations or methodological flaws. Once you find a single major error: BEWARE – there may well be others, and that study may be biased. Just because an article is in a prominent peer-reviewed journal or makes the headlines – that is NOT assurance that it is “right.” BE ATTENTIVE. LEARN more biostatistics and design methodology. APPLY what you know. Do your own calculations when warranted! ALWAYS assess the potential direction(s) of bias. Incorrect Assumptions made by the CDC & Their Corrections 1. The aerosol transmission ONLY by drops – not droplet nuclei [there are well established examples for the latter – such as Mycobacteria tuberculosis] Correction: Droplet nuclei are VERY much involved – perhaps more so than droplets 2. Asymptomatic persons not infectious [assumes viral titers do not rise until symptoms exist; NOT true for HIV!] Correction: Asymptomatic persons 3. Much of the transmission risk was due to contact with surfaces Correction: Perhaps 1% of all COVID-19 spread is by contact