Lecture #1.2 - Quick recap.pdf
Document Details
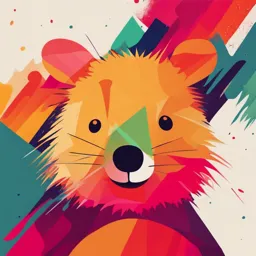
Uploaded by PerfectPanda
Tags
Full Transcript
MODERN DATA ARCHITECTURES FOR BIG DATA II QUICK RECAP AGENDA Big Data The Big Data pipeline 1. BIG DATA BIG DATA IN LAYMAN'S TERMS The 5 V's of Big Data: Volume - big amounts of data handled (batch) Velocity - data produced and processed very quickly (streaming) Variety - structured, semi-structured...
MODERN DATA ARCHITECTURES FOR BIG DATA II QUICK RECAP AGENDA Big Data The Big Data pipeline 1. BIG DATA BIG DATA IN LAYMAN'S TERMS The 5 V's of Big Data: Volume - big amounts of data handled (batch) Velocity - data produced and processed very quickly (streaming) Variety - structured, semi-structured and unstructured data (format) Veracity - data has to be correct, garbage in → garbage out (DQ/DG*) Value - Big Data project aligned to business initiative/strategy (ROI**) Big Data = evolution of Technology & Business Intelligence. Data is an asset → Big Data key to make the most out of it. Loads of potential use cases for companies in every industry. * Data Quality and Data Governance help companies with veracity to ensure valid insights. ** Return Of Investment in terms of revenue increase, cost reduction, operational effiency, customer satisfaction,... THE BEGINNINGS OF BIG DATA The Big Data movement started with Hadoop. Hadoop is inspired by a couple of Google whitepapers. Hadoop is designed to store and process huge amount of data. Hadoop has a rich ecosystem with dozens of technologies to: Ingest data with any format coming from anywhere (ex. Flume, NiFi) Store any kind of data with different requirements ( ex. Kafka, MinIO) Process data independently of nature and use cases ( ex. Spark, Pig) Serve insights as needed by applications or users ( ex. MongoDB, HBase) 2. THE BIG DATA PIPELINE BIG DATA PIPELINE/DATA VALUE CHAIN Stages and common OSS technologies covered so far: DATA INGESTION Capture/collect data and Move it into the Storage Layer. Batch & Stream data should be ingested with different tools. One of the most challenging stages in the pipeline. DATA STORAGE Data needs to be persisted for further processing. Batch & Stream data should be stored with different tools. Data Lake → how data is persisted and organized in this stage. DATA PROCESSING Data transformation to produce curated data or insights. There are two main types of data analysis: Generic - two main categories based upon the nature of data: Batch Processing, batch data is processed and analyzed Stream Processing, streaming data is processed and analyzed Specialized - nature of the scenario, additionally to nature of data: SQL analytics, data preparation and analysis using SQL Graph processing, advanced analytics around relationships of entities Machine learning workloads to identify patterns, build recommendations,... Processing frameworks/tools can combine multiple types. SPARK, UNIFIED PROCESSING Apache Spark is a Unified Procesing engine for Big Data. Advantages over Hadoop MapReduce, the ancient alternative: Faster - leverage as much server memory as possible More flexible - richer data models (ex. DataFrames) and more languages Broader adoption - applicable to different processing paradigms One API (ex. DataFrames API), multiple processing paradigms: Batch processing Stream processing SQL analytics Graph processing Machine Learning workloads CONGRATS, WE'RE DONE!