Lecture 12 - Fairness, Accountability & Transparency PDF
Document Details
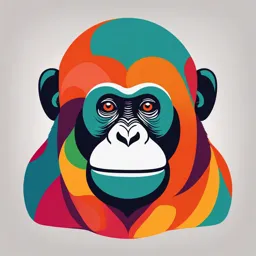
Uploaded by HardWorkingAestheticism
Technical University of Munich
2024
Jens Grossklags, Dr. Severin Engelmann
Tags
Summary
This lecture notes from the Technical University of Munich, discusses fairness, accountability, and transparency in AI and algorithmic systems, specifically focusing on autonomous vehicles, predictive policing, credit scoring, and hiring decisions, as well as the implications for the legal system. It examines issues of trust, transparency, and the question of whether algorithmic systems can be fair in decision-making.
Full Transcript
IT and Society Lecture 12: Fairness, Accountability & Transparency Prof. Jens Grossklags, Ph.D. Dr. Severin Engelmann Professorship of Cyber Trust Department of Computer Science School of Computation, Information, and Technology Technical University of Munich July 8, 2024 Announcements Q...
IT and Society Lecture 12: Fairness, Accountability & Transparency Prof. Jens Grossklags, Ph.D. Dr. Severin Engelmann Professorship of Cyber Trust Department of Computer Science School of Computation, Information, and Technology Technical University of Munich July 8, 2024 Announcements Questions on Moodle regarding exam (until Wednesday noon) Grade bonus tasks released (see Moodle) Announcement about Global AI Dialogue 2 Recap Three major approaches to normative ethics: 1. Deontology (duty, rule-based) 2. Utilitarianism (consequences, outcome) 3. Virtue ethics (moral character) Many digital technologies create morally-charged scenarios. Different cultures may have different ethical preferences (should these be taken into account?) or should experts make decisions? Autonomous vehicles create ethical and social dilemma 3 Today Fairness, Accountability & Transparency (FAT) of AI and algorithmic systems in general Autonomous vehicles: What are the chances for such an ethical dilemma to occur? 4 Today Across multiple other task domains, every single decision made by an algorithm involves an ethical dimensions, e.g.: Predictive policing and jurisdiction (recidivism decisions) Predicting financial worthiness (credit scoring) Predicting employees’ success (hiring decisions) 5 Line of Argumentation In all of these scenarios, one should ask: Is the decision fair? Who made the decision? Who is responsible? Where rests accountability? How was the decision made? Can we understand the decision-process? How transparent is the process? Why bother? 6 Line of Argumentation: Why Bother? Fairness, accountability and transparency can serve as ethical measurements. Products are subjected to legal requirements (based on ethical considerations etc.). Fairness, accountability and transparency are trust-enhancing factors. Ethics Trust-enhancing Factors (FAT) Product Adoption 7 78% of Americans Do Not Trust AVs Ethics Trust-enhancing Factors (FAT) Product Adoption Americans Feel Unsafe Sharing the Road with Fully Self-Driving Vehicles. American Automobile Association, see in Nature, 2017 8 We are Frequent Subjects of Algorithmic Decision-Making Predicting employees’ success (Highhouse 2008) Predicting academic performance (Dawes, 1971) Predictive policing and jurisdiction (Wormith et al., 1984) Predicting driving outcomes (Koo et al., 2015) Predicting sport judgments etc. How fair, accountable and transparent is AI- based algorithmic decision-making? 9 FAT: Trust-enhancing Factors for AI Adoption Without transparency, can we know whether the Transparency decision was fair or who is responsible for it? Is transparency a necessary (and sufficient?) condition to determine accountability and fairness in an algorithmic system? Accountability Fairness 10 Case Study: Assessment Tools to Predict Recidivism Risk How likely is a defendant to commit a felony or misdemeanor once released from prison? 11 What is the Appeal to use Risk Assessment Tools? − The United States locks up far more people than any other country, a disproportionate number of them African-American. − Key decisions in the legal process have been in the hands of human beings guided by their instincts and personal biases. − If computers could accurately predict which defendants were likely to commit new crimes, the criminal justice system could be fairer. 12 Example: Who gets released? Source: https://www.prisonpolicy.org/ reports/pie2024.html 13 Eric Loomis NY Times, May 2017 Classified by COMPAS software tool as an “individual who is a high risk to the community” Judge sentences Eric Loomis to 6 years in prison COMPAS = Correctional Offender Management Profiling for Alternative Sanctions 14 Is “COMPAS” Fair? How does the algorithm calculate the score? Developed by company Northpointe (now “equivant”) COMPAS in use since the year 2000 (predictions for > 1 million offenders) Scores from 1 – 10 (10 = highest risk score) Algorithm is proprietary and thus a trade secret: − Little transparency over decision-making process 15 Is this is a problem? 16 ProPublica Investigation (May 2016) 17 ProPublica Study Analysis of COMPAS risk score of 7000 people arrested in Florida in 2013 and 2014. Only 20 percent of the people predicted to commit violent crimes actually went on to do so. For misdemeanors, such as driving with an expired license, the algorithm was just above 50 percent correct. Overall: of those deemed likely to re-offend, 61 percent were arrested for any subsequent crimes within two years. 18 ProPublica Investigation (May 2016) Who is more likely to recommit a crime? Name: Vernon Prater (m) Name: Brisha Borden (f) Age: 41 Age: 18 Current charges: Current charges: Shoplifting (80$) Burglary (80$) Previous crimes: Previous crimes (juvenile): Armed robbery Administrative offences Attempted armed robbery Misdemeanours Time served in prison: 5 years Time served in prison: 0 years 19 ProPublica Investigation (May 2016) COMPAS Predictions: 2 years later: 2 years later: Serving 8-year No further prison term for charges. large burglary. 20 Is COMPAS Fair? ProPublica say NO! Northpointe say YES! ProPublica conceptualizes fairness from the perspective of the defendant. Northpointe conceptualizes fairness from the perspective of the sentencer. 21 Is COMPAS Fair? An algorithmic system cannot – in many cases – implement more than one conceptualization of fairness! 22 Northpointe’s Fairness Definition Scores map to equal probability in actual re-offending among both blacks and whites (race does not matter). Prediction: black/white person risk score 7 (medium). Reality: among both black/white with risk score 7 equal rate of re-offending (e.g., 60%). 23 Northpointe’s Fairness Definition Score = 7. Does not matter if black or white same recidivism risk (e.g., 60%) Advantage: Judges do not need to consider race at all! = Northpointe’s definition of fairness. 24 Fair? 25 Fair? Within each risk category, the proportion of defendants who reoffend is approximately the same regardless of race; this is Northpointe’s definition of fairness. From the perspective of the sentencer, system is unbiased (fair). 26 Fair? Within each risk category, the proportion of defendants who reoffend is approximately the same regardless of race. Proportions are similar, but what is different? 27 ProPublica’s Fairness Definition Problem: there are more blacks than whites classified as high or medium risk In the past: blacks twice as likely to be classified as medium or high risk (42% vs 22%). Northpointe is interested in the set of people who reoffended. What about the people who ultimately did not reoffend? 28 ProPublica’s Fairness Definition Assume 200 people, 100 white & 100 black. Test-based predictor is unbiased by race: exactly 60% of blacks classified as “high risk” recidivate and 60% of whites. Also, exactly 30% of blacks classified as “low risk” recidivate and 30% of whites. However the “high risk” group contains 60 blacks and 40 whites, while the “low risk” group contains 40 blacks and 60 whites. 29 ProPublica’s Fairness Definition White Black All Low Risk High Risk Low Risk High Risk Low Risk High Risk Distribution: 60 40 40 60 No 42 16 28 24 70% 40% Recidivism Recidivism 18 24 12 36 30% 60% Note: Distribution across “high” and “low” risk differs across race (real world data). 30 ProPublica’s Fairness Definition White Black All Low Risk High Risk Low Risk High Risk Low Risk High Risk Distribution: 60 40 40 60 No 42 16 28 24 70% 40% Recidivism Recidivism 18 24 12 36 30% 60% But: 60% of blacks classified as “high risk” recidivate (36) and 60% of whites (24). Dataset is biased towards blacks, algorithm is not. 33 Is this is a problem? But: 60% of blacks classified as “high risk” recidivate (36) and 60% of whites (24). Dataset is biased towards blacks, algorithm is not. 34 Is this is a problem? What about the people who ultimately did not reoffend? 35 Possible False/True Positives & False/True Negatives False positive: She must stay in custody even though she poses no threat to society. True positive: She must stay in custody and she is a threat to society. False negative: She can go home even though she poses a risk to society. True negative: She can go home and poses no threat to society. 36 Possible False/True Positives & False/True Negatives False positive: She must stay in custody even though she poses no threat to society. False positive rate for blacks: 44.9% False positive rate for whites: 23.5% Source: https://www.propublica.org/article/machine-bias-risk-assessments-in-criminal-sentencing 37 Possible False/True Positives & False/True Negatives False negative: She can go home even though she poses a risk to society. False negative rate for blacks: 28.0% False negative rate for whites: 47.7% Almost half of the whites classified as “low risk” ended up committing a crime. Source: https://www.propublica.org/article/machine-bias-risk-assessments-in-criminal-sentencing 38 ProPublica‘s conclusion: COMPAS was particularly likely to falsely flag black defendants as future criminals, wrongly labeling them this way at almost twice the rate as white defendants. White defendants were mislabeled as low risk more often than black defendants. This is ProPublica’s conceptualization of fairness: Keep false positive and false negative rate equal between races. 39 Predicament Impossible to simultaneously satisfy both definitions of fairness because black defendants have a higher overall recidivism rate (in the Broward County data set, black defendants recidivate at a rate of 51% as compared with 39% for white defendants, similar to the national averages). 40 The Bias is in the Data: Broken Window Theory (1982) A cycle of crime: Neighborhoods with visible civil disorder o More police forces More arrests 41 ProPublica Study: Bias in the Data A COMPAS questionnaire created a cycle: COMPAS assessment is based on 137 features about an individual and the individual’s past criminal record. Defendants answer 137 questions. Answers are fed into COMPAS software to generate recidivism risk scores. Race is not one of the questions Many proxies for race (e.g., demographic factors) 42 COMPAS Questionnaire (Examples) Possibly creating a cycle of crime https://www.documentcloud.org/documents/2702103-Sample-Risk-Assessment-COMPAS-CORE.html 43 Challenges to Ensure Fairness in AI- Based Decision-Making Garbage in/garbage out: Data can be consistently biased − Data collection: dark zones What are meaningful fairness criteria? How do different criteria relate and create trade-offs? What are their limitations? 44 Consider the Following Example An algorithm is used to allocate a limited amount of loan money to two individuals. What fairness conceptualization should the algorithm use? Which one would you use? 45 Consider the Following Example You’re a loan officer and must allocate a limited amount of loan money to two candidates. There are two candidates – Person A and Person B, they are identical in every way, except that Person A has a loan repayment rate of 100%, while Person B has a repayment rate of 20%. Both of the candidates apply for 50.000 Euro and you have 50.000 Euro available. 46 What’s Fair? There are two candidates – Person A and Person B, they are identical in every way, except that Person A has a loan repayment rate of 100% while Person B has a repayment rate of 20%. Option 1: Give all the money to Person A. Option 2: Give Person A 41.666 Euro, which is proportional to that person’s payback rate of 100% and Person B 8.333 Euro, which is proportional to that person’s payback rate of 20%. Option 3: Split the money 50/50, so that Person A receives 25.000 Euro and Person B receives 25.000 Euro. What would you do? 47 Algorithmic Systems Cannot consider multiple conceptualizations to fairness. Each definition may have its benefits and disadvantages for the data controller and the data subject. Who decides on the specific fairness definition is in a position of power (political decision). 48 FAT: Trust-enhancing Factors for AI Adoption Without transparency, can we know whether the decision was fair or who is responsible Transparency for it? Is transparency a necessary (and sufficient?) condition to determine accountability and fairness in an algorithmic system? Accountability Fairness 49 GDPR: “A Right to Explanation” of Automated Decision Making A ‘right to explanation’ of all decisions made by automated or artificially intelligent algorithmic systems is legally mandated since May 2018. Article 13,15, 22 (including Recital 71) Regulation (EU) 2016/679 of the European Parliament and of the Council of 27 April 2016 on the protection of natural persons with regard to the processing of personal data and on the free movement of such data (General Data Protection Regulation) Official Journal of the European Union, Vol. L119 (4 May 2016), pp. 1-88 50 GDPR: “A Right to Explanation” of Automated Decision Making 1) Create transparency about automated decision-making (right of data subject). 2) Create accountability (duty of data controller). Create trust: the comfort in making oneself vulnerable to another entity in the pursuit of some benefit. 51 Example: Credit Scoring and the “Right to Explanation” Scenario: your application for a loan is rejected based on your digital footprint “right to explanation”. Data controller has two options: 1) Ex ante explanation 2) Ex post explanation 52 Ex Ante versus Ex Post Decision Explanation 1) Ex ante explanation; explanation before the decision was made: “ex ante explanation can logically address only system functionality, as the rationale of a specific decision cannot be known before the decision is made“ 53 Ex Ante versus Ex Post Decision Explanation 2) Ex post explanation; explanation after the decision was made: „Ex post explanation occurs after an automated decision has taken place. Ex post explanation can address both system functionality and the rationale of a specific decision“ 54 What Does “Explanation” Mean? GDPR: Data controllers are obliged to provide the following: Meaningful information about the logic involved, as well as the significance and the envisaged consequences of automated-decision making. Test different degrees of transparency 55 Facebook: personal data classification targeted advertisements How is that process made transparent? 56 >4000 attributes to make classification 57 Explanation: ≥ 3 Attributes Is this transparency? 58 Interacting with a non-transparent algorithmic system is hardly possible. …and the stakes can be high as you saw in this lecture... Recidivism risk. Go to jail or not. Credit score. Get a loan or not. Employment. Get a job or not. … 59 Final two case studies: The ethics of facial analysis AI 60 Studies on the Ethics of Facial Analysis AI FAccT’22 (see next slides) EAAMO’22 FAccT’24 (see next slides) What do laypeople think AI Do individuals with AI How do people in Japan, should infer from human faces? competence and laypeople Argentina, Kenya, and the USA https://dl.acm.org/doi/pdf/10.114 ethically evaluate AI inference- ethically evaluate facial 5/3531146.3533080 making from faces differently? analysis AI? https://dl.acm.org/doi/10.1145/3 https://dl.acm.org/doi/pdf/10.11 551624.3555294 45/3630106.3659038 Facial Analysis AI in a Visual Data Culture thispersondoesnotexist.com RESEARCH QUESTIONS: Study 1: What do non-experts in AI think AI should infer from human faces? Study 2: How do people in Japan, Argentina, Kenya, and the USA ethically evaluate facial analysis AI? How do they justify what differentiates permissible from. impermissible facial AI.inferences? STUDY 1: DESIGN What people think AI should infer from faces Experimental Vignette Study (similar experimental set-up in study 2, slightly different inferences) 8 AI facial inferences (gender, emotion expression, likability, assertiveness, intelligence, skin color, wearing glasses, trustworthiness) 1) a low-stake advertising facial inferences to show more suitable product ads 2 contexts 2) a high-stake decision context facial inferences to inform hiring decision What do laypeople think AI should Non-experts rated each of the 8 inferences and provided a written infer from human faces? (FAccT’22) justification for their rating. Data Collection N = 3745 of non-experts in AI 29,760 written justifications analyzed with RoBERTa STUDY 1: RESULTS Perception of Inferences as Two Distinct Constructs Method: Exploratory factor analysis Result: Laypeople distinguish two types of inferences, i.e., they perceive them as two different constructs. Factor 1: emotion expression, gender, wearing glasses, skin color Factor 2: assertive, likeable, intelligent, trustworthy STUDY 1: RESULTS The consequentiality of the scenario influences non-experts’ ethical evaluations of AI facial inferences. Laypeople show more agreement towards the inferences gender, emotion, wearing glasses, and skin color in the advertisement context. Laypeople show disagreement towards the inferences intelligent, trustworthy, assertive, and likeable despite the decision making context. Disagree Agree STUDY 1: RESULTS Evaluations of AI inferences gender, skin color, emotion expression, and wearing glasses. Hiring context: Majority: rejection due to AI can tell no relevance of inference STUDY 1: RESULTS Evaluations of AI inferences intelligence, trustworthiness, likability, and assertiveness Minority: Majority: Reference to relevance AI cannot and AI’s ability to infer tell STUDY 2: RESULTS Types of qualitative justifications 5 THEMATIC CLUSTERS 3 JUSTIFICATION TYPES inference from portrait no inference from portrait EPISTEMIC (IN)VALIDITY OF dynamic concept THE INFERENCE subjectivity the medium image AI ability BELIEF/NON-BELIEF AI inability IN AI CAPABILITIES inference relevant PRAGMATIC (DIS)ADVANTAGE inference not relevant OF INFERENCE positive attitude negative attitude Study 2: Paper: Attitudes indecisive Toward Facial Analysis AI (FAccT’24) STUDY 2: RESULTS Inference differences Highlighting 4 different patters of inference perception: emotion wearing Inference: beautiful age gender expression skin color glasses trustworthy intelligent AI ability positive/ inf. from portrait affirmative inference relevant P2 connotation positive attitude AI inability no inf. from portrait negative/ inference not relevant P1 rejecting negative attitude P4 connotation dynamic concept subjectivity the medium image P3 neutral indecisive connotation PATTERNS beautiful is perceived wearing glasses, skin skin color is perceived trustworthy and as “subjective”, with color, and emotion as “irrelevant” or intelligent cannot be high variation in expression can be “discriminatory”, with inferred from a inference ratings [P3] inferred from a portrait highest variation in portrait or by an AI or by an AI [P2] inference ratings [P4] system [P1] 12 STUDY 2: RESULTS Country and context differences Positive justifications: 🔝🔝 participants from Kenya (53.1%) – inference from portrait. Negative justifications: 🔝🔝 participants from Japan (58.4%) – no inference from portrait or AI. 🔝🔝 participants from Argentina (55.3%) – not relevant. Context matters: sig. differences in ratings between AD and HR in all countries but Kenya. 13 Key Results from Both Studies Rationalizing AI visual data inferences represents a negotiation between epistemic considerations, pragmatic considerations, and believes on whether AI can or cannot perform certain inferences. Different (groups of) inferences are subject to a particular justification profile/patterns. Perceptual differences are specific to inference and context. Takeaways 1. Fairness, accountability and transparency can serve as ethical measurements. 2. Fairness, accountability and transparency are trust-enhancing factors product adoption. 3. While algorithms outperform humans on a variety of tasks, they may systematically and consistently discriminate if the dataset contains (human) biases. 4. Raw data is an oxymoron! 73 Takeaways (2) 1. Algorithmic systems can only implement one conceptualization of fairness. 2. The GDPR contains a “Right to Explanation” but only grants data subjects ex ante explanations. 3. Potentially all ML-based systems face FAT challenges if they make prediction on individuals. 4. Visual data inferences are particularly challenging because visual data are semantically ambiguous. 74 See you next week! 75