Lecture 1 - IT Productivity and the Future of Work.pdf
Document Details
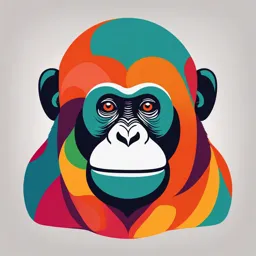
Uploaded by HardWorkingAestheticism
Technical University of Moldova
2024
Tags
Full Transcript
IT and Society Lecture 1: IT Productivity and the Future of Work Prof. Jens Grossklags, Ph.D. Professorship of Cyber Trust Department of Computer Science School of Computation, Information and Technology Technical University of Munich April 15, 2024 Administrative Aspects...
IT and Society Lecture 1: IT Productivity and the Future of Work Prof. Jens Grossklags, Ph.D. Professorship of Cyber Trust Department of Computer Science School of Computation, Information and Technology Technical University of Munich April 15, 2024 Administrative Aspects 2 Objectives Complement technical and business-oriented lectures in the information systems bachelor program Overview regarding the role of IT in society Study complex interactions between modern information and data analytics technologies and individual, organizational and societal concerns 3 Details Lecture (2 SWS) + Practice Session (2 SWS) – Required course in the information systems program Times and Location: – Lecture: 13:00 – 14:30 each Monday → 5-10 min earlier? – Practice Session: 16:15 – 17:45 each Monday – 8120.EG.001 (Lecture Hall in the Galileo Building) Materials: – Uploads & links provided on Moodle; and on slides Classroom etiquette 4 Participation and Grading Practice Session & Materials – Engagement with practice content and exercises is highly recommended to deepen your understanding of course content – Homework and reflection exercises provided on Moodle Exam + Retake Exam: – Exam (July 31, 2024) and Retake exam (not yet scheduled) – Structure: Exam lasts 90 minutes Comprehensive exam across lecture and practice session topics 5 Grade Bonus Grade bonus is applied to the exam grade, provided you passed the exam and collected sufficient bonus points Homework: Submissions will be awarded with points if they are deemed “meaningful“. – Participation in research studies: By participating in research studies you can earn additional points Details on Moodle site; and during practice sessions 6 Teaching Team Lecturers: – Prof. Jens Grossklags, Ph.D. – Guest lecturers and contributors: Dr. Mo Chen, Chiara Ullstein, Dr. Severin Engelmann https://www.cs.cit.tum.de/ct/ Practice sessions – Tutor team – Will be presented in the practice session 7 Interdisciplinary Background Postdoctoral Research Associate, Security, Privacy, and CITP Lecturer, Computer PhD and MS, School of Information Economics Lab Science Information, UC Berkeley MS, Division of Computer (SPIEL) Science, UC Berkeley Samuelson Law and Public Policy Clinic, Berkeley Law School Department of Computer Science Prof. Cyber Trust 8 Security and Privacy Environment Security and Privacy Decision-Making Design of Economic Preferences and Economic & Behaviors Incentives Behavioral Incentives User Interfaces, Information and Data Interdependencies Systems 9 Topic Overview A Balance Between Depth and Breadth Note: Order of lectures may vary to a certain degree 10 IT Productivity and Future of Work Lecture 1: – Course Administrative Aspects – IT Productivity – Automation and Computerization – Impact of Generative AI 11 Privacy Lecture 2: – Individual Privacy, e.g., Value, Perceptions and Behaviors Lecture 3: – Privacy in Organizations, e.g., GDPR Lecture 4: – Societal Concerns, e.g., Privacy, Liberty and National Security 12 Security Lecture 5: – Security on an Individual Level, e.g., Threats and Behaviors Lecture 6: – Security in Organizations, e.g., Role of Security Standards and (Evidence-based) Security Management Lecture 7: – Societal Concerns, e.g., Cyber War, Critical Infrastructures 13 Societal-Scale Mechanisms Lecture 8: – Overview over Mechanisms shaping Behavior of Individuals and Organizations E.g., Nudging/Behavioral Insights Research Units E.g., Chinese Social Credit System 14 Artificial Intelligence Lecture 9: – Artificial Intelligence: Introduction Lecture 10: – Moral Machines & Ethical Dimensions of Artificial Intelligence Lecture 11: – Fairness, Accountability, Transparency of Automated Decision-making 15 Special Topics Lecture 12: – Misleading Information, Disinformation and Fake Advice Lecture 13: (Potentially Changing) – Ethical and Practical Challenges in Conducting Research 16 Wrapping Up Lecture 14: – Summary and Recap – Exam Preparation NOTE: We are missing one lecture spot! 17 Questions about Administrative Aspects? Please use forum on Moodle site. 18 Lecture 1 IT Productivity and the Future of Work 19 Let’s Start with Your Prospects 20 Mostly Good News (for You) Statistics for Computer and Information Technology Occupations – Median annual wage: $100,530 in May 2022 – Expected Job Growth: +32% for Information Security Analysts vs. -11% for Computer Programmers (Forecast dates: from 2022 to 2032) Statistics for Business and Financial Occupations – Median annual wage: $76,850 in May 2022 – Expected Job Growth: +20% for Financial Examiners vs. -11% for Purchasing Managers, Buyers, and Purchasing Agents All Occupations: Wage: $46.310 Source: https://www.bls.gov/ooh/home.htm (Data accessed: April 13, 2024) U.S. Department of Labor 21 U.S. Department of Labor (Data Published 2022) OCCUPATION ENTRY-LEVEL EDUCATION 2022 MEDIAN PAY Computer and Information Master's degree $136,620 Research Scientists Computer Network Bachelor's degree $126,900 Architects Computer Programmers Bachelor's degree $97,800 Computer Support - $59,660 Specialists Computer Systems Analysts Bachelor's degree $102,240 Database Administrators Bachelor's degree $112,120 Information Security Analysts Bachelor's degree $112,000 Network and Computer Bachelor's degree $90,520 Systems Administrators Software Developers Bachelor's degree $124,200 Web Developers - $80,730 22 Source: https://www.bls.gov/ooh/computer-and-information-technology/home.htm German IT Labor Market Total employed Year 2020 2021 2022 2023 2024* in Germany: SUM (CE + ICT + CE + TELCO) 1189.1 1262.5 1303.7 1337.7 1367.5 45.7M Change (Year-to-Year) 0% 6% 3% 3% 2% IT Hardware 20.3 20.5 19.5 19.3 19.3 roughly 3% in IT Change (Year-to-Year) -6% 1% -5% -1% 0% Software & IT Services 991.4 1070.8 1116.3 1150.0 1184.5 Change (Year-to-Year) 0% 8% 4% 3% 3% Consumer Electronics 9.0 9.0 8.0 8.0 7.7 Change (Year-to-Year) -1% 0% -11% 0% -4% Telco (hardware/services) 168.3 162.3 160.0 160.4 156.0 Change (Year-to-Year) -3% -4% -1% 0% -3% Employment figures in 1000s; * = Estimates Bitkom (Data Published 2024) Source: https://www.bitkom.org/sites/main/files/2024-01/erwerbstaetigeitkextranetjanuar2024.pdf (Accessed April 13, 2024) 23 Growth in German Internet Industry 2018 – 2025* (employment in 1000s) Compound Annual Growth Rate (CAGR) 2020 – 2025* Source: old report - https://www.adlittle.com/sites/default/files/viewpoints/The_German_Internet_Study_2016-2019.pdf 24 new report - https://norbert-pohlmann.com/wp-content/uploads/2019/08/The-Internet-Industry-in-Germany-2020-to-2025-Prof.-Norbert-Pohlmann.pdf Growth in German Internet Industry 2018 – 2025* (in Billion Euro) Compound Annual Growth Rate (CAGR) 2020 - 2025* Growth GDP Germany -- 2017: 2.7% -- 2018: 1.1% -- 2019: 1.1% -- 2020: -4.6% -- 2021: 2.9% 25 https://www.bundesfinanzministerium.de/Monatsberichte/2022/03/Inhalte/Kapitel-6-Statistiken/6-4-01-wirtschaftswachstum-und-beschaeftigung.html A Look into the Past 26 Historical Anecdotes Ancient and medieval emperors could decide on determinants of the technology race – According to Pliny the Elder, a worker invented unbreakable glass during the reign of Emperor Tiberius (42 BC – 37 AD); instead of rewarding the creativity, Tiberius had the man executed due to fear of rebellion by workers – Emperor Vespasian (9 AD – 79 AD) likewise rejected a technology to transport columns declaring: “How will it be possible for me to feed the populace?” – Many more examples throughout history: Maintain status quo Frey: “The Technology Trap” 27 Change Over Last 100 - 200 Years: An Open Uncontrolled Race Loss of control by political decision-makers over new technologies: “In the West the individual centers of competing political power had a great deal to gain from introducing technological changes that promised commercial or industrial advantage… and much to lose from allowing others to introduce them first. Once it was clear that one or another of these competing centers would always let the genie out of the bottle, the possibility of aligning political power with the economic status quo and against technological change more or less disappeared from the Western mind.” Rosenberg & Birdzell: “How the West Grew Rich” 28 Technology = Progress? Economists’ view during the 20th century: – Technological progress is augmenting existing work or enabling new work – Even if new technologies are replacing or displacing existing jobs/workers, then (more) new and better-paying jobs will emerge due to these new technologies Contrasting (more modern) view: – Tough race between replacing/displacing effects of new technology and augmenting/enabling aspects Frey: “The Technology Trap” & Acemoglu/Restrepo: “Artificial Intelligence, Automation and Work” 29 Did IT Make Businesses More Productive? 30 IT Productivity Growth Paradox Productivity: – How efficient is production? – Ratio of (units of) output to (units of) inputs in production process assessment over time: growth or decline, e.g., labor productivity growth Hypothesis: – Increase in productivity growth with rapid development in information technology (first questioned in 70s, 80s in the U.S.) Observation: – Opposite finding for parts of 20th century Slowdown in productivity growth 31 About Computers… “… what everyone feels to have been a technological revolution, a drastic change in our productive lives, has been accompanied everywhere, including Japan, by a slowing-down of productivity growth, not by a step up. You can see the computer age everywhere but in the productivity statistics.” (Robert Solow, 1987 [†2023]) 32 Empirical Studies about 1970 – 2010 Several research studies have been challenging the IT productivity growth paradox (arguing that there is positive growth) Sources of error in studies could be: – Measurement of outputs and inputs is difficult – Profits may be subject to redistribution and dissipation – Lagged impact of IT due to learning and adjustment – Inadequate management of IT 33 Ongoing “Messy” Discussion Brynjolfsson and McAfee (2011) and others: – First, all sectors—but particularly IT-intensive sectors— are experiencing major increases in productivity. – Second, IT-powered machines will increasingly replace workers, ultimately leading to a substantially smaller role for labor in the workplace of the future. Fleck, Glaser, Sprague (2011) – Third, we observe a “decoupling” between robust U.S. productivity growth and sclerotic or negligible growth rates of median U.S. worker compensation. Source: Acemoglu et al. (2014) 34 Ongoing Discussion (2) Acemoglu et al. (2014) – Earlier “resolutions” of the Solow paradox may have neglected certain paradoxical features of IT-associated productivity increases, at least in U.S. manufacturing 1) Find little evidence of faster productivity growth in IT-intensive industries after the late 1990s 2) To the extent that there is more rapid growth of labor productivity in IT-intensive industries, this is associated with declining output and even more rapidly declining employment 35 OECD Countries Manufacturing Non-Manufacturing Percentage change in total employment across industry sectors for selected OECD countries 1995 - 2015 https://read.oecd-ilibrary.org/employment/oecd-employment-outlook-2019_9ee00155-en#page65 36 Source: OECD Employment Outlook 2017 What about Germany? Reinhold and Thomsen “The changing situation of labor market entrants in Germany”, Zeitschrift für Arbeitsmarktforschung. Data from 1975 – 2010; Not IT-specific https://doi.org/10.1007/s12651-017-0227-3 37 Findings of Reinhold and Thomsen General decline of wages for young workers – In particular, for medium-skilled and low-skilled workers – Implies growing wage premia for higher education and rising inequality in wages Highly-educated workers have faced declining employment opportunities in top-paying jobs Analytical jobs have more positive history overall 38 What about the Future? 39 40 NY Times (1928) 41 42 1940s 1980s 43 Many Pessimistic Predictions Justified? 1964 1978 2016 Automation in Germany Computer-Revolution: You are Fired: How Computers Progress Yields Unemployment and Robots Take Away Jobs 44 Trends Changing Future World of Work What jobs will be created? How, where and by whom are they carried out? Ageing What jobs are Societies lost? Globalization New Business Models Technology/ See: Practice Session TODAY Digitalization Paolo Falco, OECD 45 Is There Enough New Work? E.g., Platform Economy/Gig Economy? Greater income, efficiency and flexibility? Less social protection and greater precarity? Greater risk for individuals who manage their jobs, protection and training? Paolo Falco, OECD Non-Standard Employment is Not New 16,5 16,1% 16,0 15,6% 15,5 OECD Average 15,0 (not dated) 14,5 14,0 13,5% 13,5 13,0 12,5 12,0 Self-employment Part-time employment Temporary employment (as % of total employment)(as % of total employment) (as a % all employees) Paolo Falco, OECD 47 Research Study Projecting Fate of Different Jobs (Analysis from 2015/Published 2017) 48 How Susceptible are Different Professions to Computerization? Frey and Osborne “The Future of Employment: How Susceptible are Jobs to Computerisation?” Technological Forecasting and Social Change Used ML methodology to estimate the probability of computerization for 702 occupations – 17-page appendix detailing the results – Types of jobs at risk – Relationship between an occupation’s probability of computerization, wages and educational attainment 49 Discussing Different Trends Labor market polarization: – Growing employment in high income cognitive jobs Problem-solving skills making use of computing power – Growing employment in low-income manual service occupations Hard to automate – Loss of employment in middle-income routine jobs See earlier slide on OECD countries 50 Model of Tasks Routine vs. Non-routine tasks Cognitive vs. Manual tasks Social Intelligence, Creativity, Perception and Manipulation 51 Findings: 47% of Employment at Risk Employment Probability of Computerization 52 Examples: Prob. of Computerization Position Probability Occupation 32 0.0065 Computer Systems Analysts 69 0.015 Computer and Information Research Scientists 109 0.03 Network and Computer Systems Administrators 110 0.03 Database Administrators 118 0.035 Computer and Information Systems Managers 130 0.042 Software Developers, Applications 181 0.13 Software Developers, Systems Software Information Security Analysts, Web Developers, and 208 0.21 Computer Network Architects 214 0.22 Computer Hardware Engineers 428 0.78 Computer Operators 442 0.81 Word Processors and Typists … … 702 0.99 Telemarketers 53 An Even Bigger Problem? Controversial Point of View “The crucial problem isn’t creating new jobs. The crucial problem is creating new jobs that humans perform better than algorithms. Consequently, by 2050 a new class of people might emerge – the useless class. People who are not just unemployed, but unemployable.” Yuval Noah Harari 54 Do workers have the skills for the new jobs? % Problem-Solving Skills in Technology-Rich Level 4/5 omitted Environments Percentage of the working-age population (aged 15/16-64) Definition: Problem solving = Facing complex problems (at least 30 minutes of thinking to find a solution) Close to 70% of the wage Few high-skilled differential between temporary workers and permanent workers can be explained by differences in the use of problem-solving skills at work. Pol Irl Svk Est Kor USA Aut Cze Avg. Bel JP UK DE Can Aus Dnk Nor NL Fin Swe Source: See Figure 6.1 in OECD Skills Outlook 2013: First Results from the Survey of Adult Skills. (466 pages) 55 http://www.oecd.org/skills/piaac/Skills%20volume%201%20(eng)--full%20v12--eBook%20(04%2011%202013).pdf AI Skills: Developer Type of skill Examples General knowledge of AI (such as Machine Learning) Specific knowledge of AI models (“decision Specialized AI skills trees”, “deep learning”, “neural network”, “random forest”, etc.) AI tools (“tensorflow”, “pytorch”, etc.) and AI software (“java”, “gradle”, “galaxy cluster”, etc). Skills to develop and Data analysis maintain AI systems Software Programming languages, in particular, Python for Data science skills Big data analysis Data visualization Cloud computing Other cognitive skills Creative problem solving Social skills Transversal skills Management skills 56 AI Skills: Adopter, User Type of skill Examples Elementary AI Principles of machine learning knowledge Skills to adopt, use and Digital skills Ability to use a computer or a smartphone interact with AI Analytical skills, Problem-solving, Critical Other cognitive skills applications thinking, Judgment Creativity, Communication, Teamwork, Transversal skills Multitasking Realistic for individuals with few problem-solving skills? Already outdated given the OECD Employment Outlook 2023 emergence of generative AI in Artificial Intelligence and the Labour Market https://www.oecd.org/employment-outlook/2023/#report the marketplace? 57 Generative AI: Possible Impact Eroding last bastions of human advantage? – Creativity – High level of expertise – Cognitive skills –… 58 Standardized Testing Rapid progress with new models GPT-4 Technical Report https://arxiv.org/abs/2303.08774 59 Impact on Professions We investigate the potential implications of large language models (LLMs), such as Generative Pretrained Transformers (GPTs), on the U.S. labor market, focusing on the increased capabilities arising from LLM- powered software compared to LLMs on their own. Using a new rubric, we assess occupations based on their alignment with LLM capabilities, integrating both human expertise and GPT-4 classifications. Our findings reveal that around 80% of the U.S. workforce could have at least 10% of their work tasks affected by the introduction of LLMs, while approximately 19% of workers may see at least 50% of their tasks impacted. We do not make predictions about the development or adoption timeline of such LLMs. The projected effects span all wage levels, with higher-income jobs potentially facing greater exposure to LLM capabilities and LLM-powered software. Significantly, these impacts are not restricted to industries with higher recent productivity growth. Our analysis suggests that, with access to an LLM, about 15% of all worker tasks in the US could be completed significantly faster at the same level of quality. When incorporating software and tooling built on top of LLMs, this share increases to between 47 and 56% of all tasks. This finding implies that LLM-powered software will have a substantial effect on scaling the economic impacts of the underlying models. We conclude that LLMs such as GPTs exhibit traits of general-purpose technologies, indicating that they could have considerable economic, social, and policy implications. An Early Look at the Labor Market Impact Potential of Large Language Models 60 https://arxiv.org/abs/2303.10130 Software Engineering E.g., CoPilot increases speed https://arxiv.org/pdf/2302.06590.pdf Other factors less well studied https://arxiv.org/abs/2403.07506 Higher IT Productivity? 61 Example: GitHub CoPilot GitClear Data and Report: Commit Line Operations Churned: Must have been authored, pushed to the Git repo, and then reverted or substantially revised within the subsequent two weeks https://gitclear-public.s3.us-west-2.amazonaws.com/Coding-on-Copilot-2024-Developer-Research.pdf 62 Generative AI: Possible Impact (2) Reducing inequality? The last round of automation, affecting manufacturing jobs, increased income inequality by depriving workers without college educations of high-paying jobs, research has shown. Some scholars say large language models could do the opposite — decreasing inequality between the highest-paid workers and everyone else. “My hope is it will actually allow people with less formal education to do more things,” said David Autor, a labor economist at M.I.T., “by lowering barriers to entry for more elite jobs that are well paid.” “Reversal Because of A.I., Office Jobs Are Now More at Risk” (New York Times) 63 Generative AI at Work New AI tools have the potential to change the way workers perform and learn, but little is known about their impacts on the job. In this paper, we study the staggered introduction of a generative AI-based conversational assistant using data from 5,179 customer support agents. Access to the tool increases productivity, as measured by issues resolved per hour, by 14% on average, including a 34% improvement for novice and low-skilled workers but with minimal impact on experienced and highly skilled workers. We provide suggestive evidence that the AI model disseminates the best practices of more able workers and helps newer workers move down the experience curve. In addition, we find that AI assistance improves customer sentiment, increases employee retention, and may lead to worker learning. Our results suggest that access to generative AI can increase productivity, with large heterogeneity in effects across workers. What effects do you foresee? Will low-skilled individuals earn more, or high-skilled individuals less? Generative AI at Work https://www.nber.org/papers/w31161 64 Daron Acemoglu Lecture https://caiml.org/dighum/dighum-lectures/daron-acemoglu-can-we-have-pro-human-ai-2024-02-13/acemoglu-slides.pdf 65 Takeaways Minefield of different (strongly expressed) opinions over the last 100 years regarding impact of technology IT productivity is generally difficult to measure (see productivity growth paradox) Labor market polarization denotes a loss of employment in middle-income routine jobs; breeding inequality New business models may provide new employment opportunities but also potential societal tensions Need to avoid “unemployability“ Generative AI introduces different effects from automation 66 The End. For Today. See you next week. 67