Lecture-1.pdf
Document Details
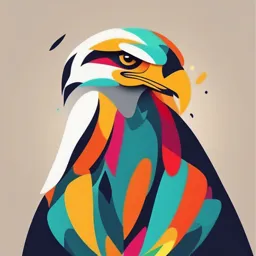
Uploaded by FineRevelation
Isabela State University
2024
Tags
Full Transcript
OVERVIEW OF DATA, DATA SCIENCE...
OVERVIEW OF DATA, DATA SCIENCE ANALYTICS, AND TOOLS DARIOS B. ALADO, DIT DARIOS B. ALADO, DIT | Data Science Analytics | Isabela State University - College of Computing Science, Information and Communication Technology 7/4/2024 HISTORY OF DATA SCIENCE The history of data science and analytics is rich and spans several centuries, involving contributions from various fields such as statistics, mathematics, computer science, and domain-specific knowledge. Here’s a chronological overview highlighting key milestones: Early Foundations (1600s - 1800s) 17th Century: The development of probability theory by mathematicians such as Blaise Pascal and Pierre de Fermat laid the groundwork for statistical analysis. 18th Century: Thomas Bayes formulated Bayes' Theorem, providing a mathematical approach to probability inference. 19th Century: The emergence of statistics as a distinct discipline, with contributions from figures like Carl Friedrich Gauss (normal distribution) and Florence Nightingale (statistical graphics). DARIOS B. ALADO, DIT | Data Science Analytics | Isabela State University - College of Computing Science, Information and Communication Technology 7/4/2024 HISTORY OF DATA SCIENCE 20th Century: The Rise of Statistics and Computing Early 1900s: Karl Pearson and Ronald A. Fisher advanced the field of statistics with the introduction of correlation coefficients, hypothesis testing, and analysis of variance (ANOVA). 1930s: The development of the first mechanical computers by pioneers like Alan Turing and John Atanasoff marked the beginning of the computing era. 1950s: The advent of electronic computers enabled more complex data analysis and the birth of computer science as a field. The term "artificial intelligence" was coined, and the first neural networks were conceptualized. 1960s: The introduction of the term "data processing" as businesses began to use computers for managing data. Edgar F. Codd proposed the relational database model, revolutionizing data storage and retrieval. DARIOS B. ALADO, DIT | Data Science Analytics | Isabela State University - College of Computing Science, Information and Communication Technology 7/4/2024 HISTORY OF DATA SCIENCE Late 20th Century: The Digital Age 1970s: The development of structured query language (SQL) facilitated efficient data management and querying. Statistical software like SAS and SPSS became widely used in academia and industry. 1980s: The rise of personal computers made data analysis tools more accessible. The concept of data warehousing emerged, enabling organizations to consolidate and analyze large datasets. 1990s: The explosion of the internet led to an unprecedented increase in data generation. The term "business intelligence" (BI) became popular, emphasizing data-driven decision-making. DARIOS B. ALADO, DIT | Data Science Analytics | Isabela State University - College of Computing Science, Information and Communication Technology 7/4/2024 HISTORY OF DATA SCIENCE 21st Century: The Era of Big Data and Data Science 2000s: The advent of big data technologies such as Hadoop and NoSQL databases enabled the processing of vast amounts of unstructured data. The term "data science" gained prominence, reflecting the interdisciplinary nature of modern data analysis. 2010s: The proliferation of machine learning and artificial intelligence applications in various industries. Data science became a recognized profession, with dedicated academic programs and roles such as data scientists and data engineers. The rise of cloud computing facilitated scalable data storage and processing. 2020s: The integration of data science with other emerging technologies such as IoT, blockchain, and quantum computing. Emphasis on ethical considerations, data privacy, and interpretability of AI models. The COVID-19 pandemic highlighted the importance of data science in public health and policy-making. DARIOS B. ALADO, DIT | Data Science Analytics | Isabela State University - College of Computing Science, Information and Communication Technology 7/4/2024 KEY MILESTONES AND CONTRIBUTION Development of Machine Learning Algorithms: Algorithms such as decision trees, support vector machines, and neural networks have become fundamental tools in data science. Advances in Statistical Methods: Techniques like bootstrapping, Bayesian inference, and time series analysis have enhanced the ability to draw meaningful insights from data. Growth of Open-Source Tools: The development of open- source languages and libraries such as Python, R, TensorFlow, and Scikit-learn has democratized data science, making powerful tools accessible to a wider audience. Data Visualization: Innovations in data visualization, through tools like Tableau, D3.js, and Matplotlib, have improved the ability to communicate complex data insights effectively. DARIOS B. ALADO, DIT | Data Science Analytics | Isabela State University - College of Computing Science, Information and Communication Technology 7/4/2024 KEY MILESTONES AND CONTRIBUTION Development of Machine Learning Algorithms: Algorithms such as decision trees, support vector machines, and neural networks have become fundamental tools in data science. Advances in Statistical Methods: Techniques like bootstrapping, Bayesian inference, and time series analysis have enhanced the ability to draw meaningful insights from data. Growth of Open-Source Tools: The development of open- source languages and libraries such as Python, R, TensorFlow, and Scikit-learn has democratized data science, making powerful tools accessible to a wider audience. Data Visualization: Innovations in data visualization, through tools like Tableau, D3.js, and Matplotlib, have improved the ability to communicate complex data insights effectively. DARIOS B. ALADO, DIT | Data Science Analytics | Isabela State University - College of Computing Science, Information and Communication Technology 7/4/2024 KEY MILESTONES AND CONTRIBUTION Current Trends Automation and AutoML: The use of automated machine learning (AutoML) tools to streamline model development and deployment. Explainable AI: Growing focus on making AI models transparent and interpretable. Ethics and Data Privacy: Increasing emphasis on ethical considerations, fairness, and data privacy in data science practices. DARIOS B. ALADO, DIT | Data Science Analytics | Isabela State University - College of Computing Science, Information and Communication Technology 7/4/2024 WHAT IS DATA SCIENCE? Data science is an interdisciplinary field that focuses on extracting knowledge and insights from structured and unstructured data through scientific methods, processes, algorithms, and systems. It combines principles and techniques from mathematics, statistics, computer science, and domain-specific knowledge to analyze and interpret complex data. DARIOS B. ALADO, DIT | Data Science Analytics | Isabela State University - College of Computing Science, Information and Communication Technology 7/4/2024 DATA SCIENCE LIFE CYCLE The Data Science Life Cycle is a systematic approach to solving data-driven problems and extracting actionable insights from data. It encompasses several stages, each involving specific tasks and methodologies. 1. Problem Definition: Objective: Understand the business problem or research question to be addressed. Tasks: Collaborate with stakeholders to define clear goals and objectives, identify key metrics for success, and establish a project plan. 2. Data Collection: Objective: Gather data from various sources that are relevant to the problem. Tasks: Collect data from databases, APIs, web scraping, sensors, surveys, or third-party sources. Ensure data is in a usable format. College of Computing Science, Information and Communication Technology 7/4/2024 DATA SCIENCE LIFE CYCLE 3. Data Cleaning and Preprocessing: Objective: Prepare the data for analysis by addressing quality issues. Tasks: Handle missing values, remove duplicates, correct errors, standardize formats, and normalize data. Perform exploratory data analysis (EDA) to understand data distributions and relationships. 4. Data Exploration and Analysis: Objective: Gain insights and identify patterns in the data. Tasks: Use statistical methods and visualization tools to explore data, summarize key characteristics, and identify trends or anomalies. Develop hypotheses based on initial findings. 5. Feature Engineering: Objective: Create relevant features that improve model performance. Tasks: Transform raw data into meaningful features, perform dimensionality reduction, create interaction terms, and normalize or scale features. 7/4/2024 DARIOS B. ALADO, DIT | Data Science Analytics | Isabela State University - College of Computing Science, Information and Communication Technology DATA SCIENCE LIFE CYCLE 6. Model Building: Objective: Develop predictive or descriptive models based on the data. Tasks: Select appropriate algorithms, train models using training data, tune hyperparameters, and use techniques such as cross- validation to assess model performance. 7. Model Evaluation: Objective: Assess the accuracy and robustness of the model. Tasks: Evaluate model performance using metrics such as accuracy, precision, recall, F1-score, ROC-AUC, or others depending on the problem type (classification, regression, etc.). Validate the model on unseen test data. 8. Model Deployment: Objective: Implement the model in a production environment for real-time or batch processing. Tasks: Integrate the model into business processes or applications, set up APIs or batch processing pipelines, and ensure scalability and reliability. 7/4/2024 DARIOS B. ALADO, DIT | Data Science Analytics | Isabela State University - College of Computing Science, Information and Communication Technology DATA SCIENCE LIFE CYCLE 9. Model Monitoring and Maintenance: Objective: Ensure the model continues to perform well over time. Tasks: Monitor model performance in production, track key metrics, detect and address issues such as data drift or model degradation, and retrain or update the model as needed. 10. Communication and Reporting: Objective: Share insights and results with stakeholders. Tasks: Create reports, dashboards, and visualizations to present findings. Communicate the implications of the results and provide recommendations for decision-making. 11. Iteration and Improvement: Objective: Continuously improve the model and the overall process. Tasks: Use feedback from stakeholders and performance monitoring to refine the model, explore new features or data sources, and enhance the data science pipeline. DARIOS B. ALADO, DIT | Data Science Analytics | Isabela State University - College of Computing Science, Information and Communication Technology 7/4/2024 TYPES OF DATA ANALYTICS DARIOS B. ALADO, DIT | Data Science Analytics | Isabela State University - College of Computing Science, Information and Communication Technology 7/4/2024 TYPES OF DATA ANALYTICS 1. Descriptive Analytics Objective: Understand past and current data to identify trends and patterns. Methods and Tools: Data Aggregation: Summarizing data to extract meaningful information. Data Visualization: Using charts, graphs, dashboards, and reports to represent data visually. Basic Statistical Analysis: Calculating averages, percentages, and other summary statistics. Examples: Generating sales reports to show monthly revenue trends. Visualizing website traffic data to understand user behavior over time. Summarizing customer feedback to identify common themes and sentiments. DARIOS B. ALADO, DIT | Data Science Analytics | Isabela State University - College of Computing Science, Information and Communication Technology 7/4/2024 TYPES OF DATA ANALYTICS 2. Diagnostic Analytics Objective: Determine the reasons behind past outcomes or events. Methods and Tools: Root Cause Analysis: Identifying the underlying causes of specific outcomes. Drill-Down Analysis: Breaking down data into finer details to explore specific aspects. Correlation Analysis: Assessing relationships between different variables. Examples: Analyzing a sudden drop in sales to identify contributing factors. Investigating customer churn rates to determine the reasons for customer loss. Examining production delays to find the root causes in a manufacturing process. DARIOS B. ALADO, DIT | Data Science Analytics | Isabela State University - College of Computing Science, Information and Communication Technology 7/4/2024 TYPES OF DATA ANALYTICS 3. Predictive Analytics Objective: Forecast future outcomes based on historical data. Methods and Tools: Statistical Modeling: Using techniques such as regression analysis to predict future values. Machine Learning: Applying algorithms like decision trees, random forests, and neural networks to make predictions. Time Series Analysis: Forecasting future values based on historical trends and patterns. Examples: Predicting future sales based on past trends and seasonal patterns. Forecasting stock prices or market trends using historical financial data. Anticipating equipment failures in manufacturing using sensor data and historical maintenance records. DARIOS B. ALADO, DIT | Data Science Analytics | Isabela State University - College of Computing Science, Information and Communication Technology 7/4/2024 TYPES OF DATA ANALYTICS 4. Prescriptive Analytics Objective: Recommend actions to achieve desired outcomes. Methods and Tools: Optimization Techniques: Using linear programming, integer programming, and other methods to find the best course of action. Simulation: Modeling different scenarios to assess the impact of various decisions. Decision Analysis: Evaluating different decision options and their potential outcomes. Examples: Recommending inventory levels to minimize costs while meeting demand. Suggesting marketing strategies to maximize customer engagement and conversion rates. Optimizing delivery routes to reduce transportation costs and improve efficiency. DARIOS B. ALADO, DIT | Data Science Analytics | Isabela State University - College of Computing Science, Information and Communication Technology 7/4/2024 WHAT IS DATA? Data refers to raw, unprocessed facts and figures collected from various sources. It can take various forms, such as numbers, text, images, videos, and sounds. Data is the fundamental building block for information and knowledge generation when it is processed, analyzed, and interpreted. DARIOS B. ALADO, DIT | Data Science Analytics | Isabela State University - College of Computing Science, Information and Communication Technology 7/4/2024 TYPES OF DATA Types of Data: 1.Structured Data: 1. Definition: Data that is organized in a predefined format or structure, often in rows and columns, making it easily searchable and analyzable. 2. Examples: Databases, spreadsheets, CSV files. 3. Sources: Relational databases (MySQL, PostgreSQL), spreadsheets (Excel). 2.Unstructured Data: 1. Definition: Data that does not have a predefined format or structure, making it more complex to process and analyze. 2. Examples: Text documents, social media posts, images, videos, emails. 3. Sources: Social media platforms, multimedia files, emails. 3.Semi-Structured Data: 1. Definition: Data that does not fit into a rigid structure like structured data but contains tags or markers to separate elements, making it somewhat easier to organize and analyze. 2. Examples: XML files, JSON files, HTML documents. 3. Sources: Web pages, APIs. DARIOS B. ALADO, DIT | Data Science Analytics | Isabela State University - College of Computing Science, Information and Communication Technology 7/4/2024 CHARACTERISTICS OF DATA DARIOS B. ALADO, DIT | Data Science Analytics | Isabela State University - College of Computing Science, Information and Communication Technology 7/4/2024 LEVEL OF MEASUREMENT OF DATA Nominal Data Definition: Nominal data is a type of categorical data where the categories do not have any inherent order or ranking. It is used for labeling variables without any quantitative value. Characteristics: Categories: Represents different categories or groups. No Order: No logical order or ranking among the categories. Qualitative: Describes qualities or characteristics. Examples: Gender: Male, Female, Non-binary. Marital Status: Single, Married, Divorced, Widowed. Types of Cuisine: Italian, Chinese, Mexican, Indian. DARIOS B. ALADO, DIT | Data Science Analytics | Isabela State University - College of Computing Science, Information and Communication Technology 7/4/2024 LEVEL OF MEASUREMENT OF DATA Ordinal Data Definition: Ordinal data is a type of categorical data where the categories have a meaningful order or ranking, but the intervals between the categories are not necessarily equal or known. Characteristics: Order: Categories have a logical order or ranking. Unequal Intervals: The difference between categories is not uniform or known. Qualitative or Quantitative: Can describe both qualitative attributes and ranked quantitative measures. Examples: Education Level: High School, Bachelor's, Master's, Doctorate. Customer Satisfaction: Very Unsatisfied, Unsatisfied, Neutral, Satisfied, Very Satisfied. Class Ranks: Freshman, Sophomore, Junior, Senior. DARIOS B. ALADO, DIT | Data Science Analytics | Isabela State University - College of Computing Science, Information and Communication Technology 7/4/2024 LEVEL OF MEASUREMENT OF DATA Interval Data Definition: Interval data is a type of quantitative data where the difference between values is meaningful, but there is no true zero point. Characteristics: Equal Intervals: The difference between values is consistent and measurable. No True Zero: Zero is arbitrary and does not indicate the absence of the attribute. Quantitative: Represents numerical values with equal intervals. Examples: Temperature (Celsius or Fahrenheit): The difference between 20°C and 30°C is the same as between 30°C and 40°C, but 0°C does not mean 'no temperature.' Calendar Dates: The difference between years is consistent, but there is no 'zero year.' DARIOS B. ALADO, DIT | Data Science Analytics | Isabela State University - College of Computing Science, Information and Communication Technology 7/4/2024 LEVEL OF MEASUREMENT OF DATA Ratio Data Definition: Ratio data is a type of quantitative data that has all the properties of interval data, but with a meaningful zero point that indicates the absence of the measured attribute. Characteristics: Equal Intervals: The difference between values is consistent and measurable. True Zero: Zero indicates the absence of the attribute being measured. Quantitative: Represents numerical values with equal intervals and a true zero. Examples: Height: Measured in centimeters or inches, with 0 indicating no height. Weight: Measured in kilograms or pounds, with 0 indicating no weight. Income: Measured in currency units, with 0 indicating no income. DARIOS B. ALADO, DIT | Data Science Analytics | Isabela State University - College of Computing Science, Information and Communication Technology 7/4/2024 SOURCES OF DATA 1. Primary Data Sources Primary data is data collected directly from first-hand sources for a specific research purpose or analysis. It is original and unique to the study at hand. Surveys and Questionnaires: Collect data directly from individuals through structured questions. Example: Customer satisfaction surveys, market research questionnaires. Interviews: Gather in-depth information through one-on-one or group conversations. Example: Employee feedback interviews, qualitative research interviews. Observations: Collect data by observing behaviors, events, or conditions. Example: Observing consumer behavior in a retail store, recording traffic patterns. Experiments: Conduct controlled tests or experiments to gather data on specific variables. Example: A/B testing in marketing, clinical trials in healthcare. DARIOS B. ALADO, DIT | Data Science Analytics | Isabela State University - College of Computing Science, Information and Communication Technology 7/4/2024 SOURCES OF DATA 2. Secondary Data Sources Secondary data is data that has already been collected and published by others for a different purpose. It is readily available and can be used for further analysis. Government Reports and Publications: Official documents and statistics provided by government agencies. Example: Census data, economic reports, public health records. Academic Journals and Research Papers: Published studies and research findings from academic institutions. Example: Articles from scientific journals, conference proceedings. Books and Reference Materials: Information compiled in books, encyclopedias, and other reference sources. Example: Textbooks, industry handbooks. Commercial Data: Data collected and sold by commercial entities. Example: Market research reports, syndicated data services. DARIOS B. ALADO, DIT | Data Science Analytics | Isabela State University - College of Computing Science, Information and Communication Technology 7/4/2024 SOURCES OF DATA 3. Digital and Online Sources With the rise of the internet and digital technologies, a vast amount of data is generated and available online. Websites and Online Databases: Information available on the internet through various websites and online repositories. Example: Company websites, online encyclopedias, databases like PubMed and Google Scholar. Social Media Platforms: Data generated by users on social media platforms. Example: Tweets, Facebook posts, Instagram photos. E-Commerce Platforms: Data from online transactions and user interactions. Example: Purchase history, product reviews, browsing behavior. DARIOS B. ALADO, DIT | Data Science Analytics | Isabela State University - College of Computing Science, Information and Communication Technology 7/4/2024 SOURCES OF DATA 4. Machine-Generated Data Data generated automatically by machines and sensors, often in large volumes and at high velocity. IoT Devices and Sensors: Data from interconnected devices and sensors in various environments. Example: Smart home devices, industrial sensors, environmental monitoring systems. Log Files: Records of events and transactions automatically logged by software applications and systems. Example: Server logs, application logs, security logs. DARIOS B. ALADO, DIT | Data Science Analytics | Isabela State University - College of Computing Science, Information and Communication Technology 7/4/2024 SOURCES OF DATA 5. Internal Organizational Data Data generated and stored within an organization, often used for operational and strategic purposes. Customer Databases: Information about customers collected through interactions and transactions. Example: Customer profiles, purchase history, customer support tickets. Financial Records: Data related to financial transactions and performance. Example: Sales records, expense reports, profit and loss statements. Human Resources Data: Information about employees and workforce management. Example: Employee records, payroll data, performance evaluations. DARIOS B. ALADO, DIT | Data Science Analytics | Isabela State University - College of Computing Science, Information and Communication Technology 7/4/2024 SOURCES OF DATA 6. Open Data Sources Data that is freely available for anyone to use, often provided by governments, organizations, or communities. Open Government Data: Publicly accessible data released by government entities. Example: Open data portals, public datasets on health, education, and transportation. Community-Contributed Data: Data shared by communities or collaborative projects. Example: Wikipedia, open-source software repositories, community science projects. DARIOS B. ALADO, DIT | Data Science Analytics | Isabela State University - College of Computing Science, Information and Communication Technology 7/4/2024 DATA ANALYTICS TOOL DARIOS B. ALADO, DIT | Data Science Analytics | Isabela State University - College of Computing Science, Information and Communication Technology 7/4/2024 DATA ANALYTICS TOOL Data analytics tools are software applications and platforms designed to process, analyze, visualize, and interpret data. These tools enable organizations and individuals to derive meaningful insights from large and complex datasets. Here are some popular data analytics tools categorized based on their functionalities: DARIOS B. ALADO, DIT | Data Science Analytics | Isabela State University - College of Computing Science, Information and Communication Technology 7/4/2024 DATA ANALYTICS TOOL Data Collection and Integration Tools 1.Apache Kafka: A distributed streaming platform for collecting and processing real-time data streams. 2.Apache NiFi: An open-source data integration tool that allows for the automation of data flow between systems. 3.Talend: Provides data integration and data quality services through a unified platform for ETL (Extract, Transform, Load) processes. Data Storage and Management Tools 1.Apache Hadoop: An open-source framework for distributed storage and processing of large datasets across clusters of computers. 2.Apache Spark: A unified analytics engine for large-scale data processing, offering both batch processing and real-time data streaming capabilities. 3.Amazon S3 (Simple Storage Service): A scalable object storage service offered by Amazon Web Services (AWS) for storing and retrieving data. DARIOS B. ALADO, DIT | Data Science Analytics | Isabela State University - College of Computing Science, Information and Communication Technology 7/4/2024 DATA ANALYTICS TOOL Data Analysis and Exploration Tools 1.Tableau: A powerful data visualization tool that allows users to create interactive and shareable dashboards and reports. 2.Power BI: Microsoft's business analytics service for creating interactive visualizations and business intelligence reports. 3.QlikView / Qlik Sense: Business intelligence and data visualization tools for exploring and analyzing data from multiple sources. Statistical Analysis and Modeling Tools 1.R: A programming language and software environment for statistical computing and graphics, widely used for data analysis and machine learning. 2.Python (with libraries like NumPy, Pandas, SciPy, scikit-learn): A versatile programming language with extensive libraries for data manipulation, analysis, and machine learning. 3.IBM SPSS Statistics: Statistical software used for data analysis, including descriptive statistics, regression analysis, and predictive modeling. DARIOS B. ALADO, DIT | Data Science Analytics | Isabela State University - College of Computing Science, Information and Communication Technology 7/4/2024 DATA ANALYTICS TOOL Machine Learning and AI Tools 1.TensorFlow / Keras: Open-source frameworks for deep learning and machine learning applications, developed by Google. 2.PyTorch: A machine learning library for Python, developed by Facebook's AI Research lab, known for its flexibility and ease of use. 3.Azure Machine Learning: Microsoft's cloud-based service for building, training, and deploying machine learning models. Big Data Processing and Querying Tools 1.Apache Hive: A data warehouse infrastructure built on top of Hadoop for querying and managing large datasets stored in Hadoop's HDFS. 2.Apache Drill: A schema-free SQL query engine for big data exploration, supporting a wide range of data sources and formats. 3.Google BigQuery: A serverless, highly scalable, and cost-effective cloud data warehouse for running SQL queries on large datasets. DARIOS B. ALADO, DIT | Data Science Analytics | Isabela State University - College of Computing Science, Information and Communication Technology 7/4/2024 DATA ANALYTICS TOOL Data Governance and Security Tools 1.Collibra: A data governance platform that provides tools for data cataloging, data lineage, and data stewardship. 2.IBM InfoSphere Information Governance Catalog: Offers capabilities for metadata management, data lineage, and governance policies enforcement. 3.Varonis Data Security Platform: Provides tools for data access governance, data security, and threat detection across on-premises and cloud environments. Data Visualization and Reporting Tools 1.D3.js: A JavaScript library for producing dynamic, interactive data visualizations in web browsers. 2.Plotly: A graphing library for creating interactive plots and charts in Python, R, and JavaScript. 3.Microsoft Excel: Widely used spreadsheet software that includes features for data analysis, visualization, and reporting. DARIOS B. ALADO, DIT | Data Science Analytics | Isabela State University - College of Computing Science, Information and Communication Technology 7/4/2024 DATA ANALYTICS TOOL Business Intelligence (BI) Platforms 1.Sisense: BI software that allows users to prepare, analyze, and visualize complex datasets using AI-driven analytics. 2.Looker: A data exploration and business intelligence platform that offers data modeling, exploration, and real-time analytics capabilities. 3.Yellowfin BI: Provides tools for data visualization, dashboards, and reporting, with embedded analytics and collaboration features. Workflow and Automation Tools 1.Alteryx: A platform for data blending and advanced data analytics, offering workflow automation and predictive analytics capabilities. 2.Apache Airflow: A platform to programmatically author, schedule, and monitor workflows, with support for task dependencies and data pipelines. 3.Knime: An open-source platform for creating data science workflows, including data integration, preprocessing, analysis, and visualization. DARIOS B. ALADO, DIT | Data Science Analytics | Isabela State University - College of Computing Science, Information and Communication Technology 7/4/2024 CHAPTER ACTIVITIES Activity 1 Instructions: 1. Briefly explain the concept of data types in data science: nominal, ordinal, interval, and ratio. 2. Provide examples for each type to ensure understanding. Activity 2 1. Distribute a list of examples (see examples below) or display them on a screen. 2. Classify each example into one of the four data types. Example list: 1. Temperature readings in Celsius 2. Types of animals in a zoo 3. Educational attainment (e.g., high school diploma, bachelor's degree) 4. Customer satisfaction ratings (e.g., very satisfied, satisfied, neutral, unsatisfied, very unsatisfied) 5. Heights of students in a class 6. Gender (e.g., male, female, non-binary) 7. Years of experience in a job 8. Scores on a 1-10 happiness scale Activity 3 1. After classifying the examples, discuss as a group why each example belongs to its respective data type. 2. Explore the implications of each type for data analysis and decision-making. 3. Ask participants to brainstorm real-world scenarios where understanding data types is crucial (e.g., healthcare, marketing, finance). DARIOS B. ALADO, DIT | Data Science Analytics | Isabela State University - College of Computing Science, Information and Communication Technology 7/4/2024