Language Past Paper PDF
Document Details
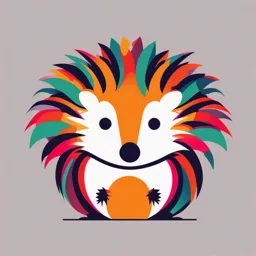
Uploaded by WellBredTurtle345
null
Adam Osth
Tags
Summary
This document is a lecture presentation on language and memory, focusing on topics such as lexical decision tasks, priming effects, spreading activation, word frequency effects, and the interactive activation model. The presentation includes diagrams and figures.
Full Transcript
PSYC20007 Language Adam Osth How is memory for language different from memory for events? Episodic memory Memory for events! Even associations among arbitrary items (A-B, C-D) would be considered episodic memory Example questions: “What did you learn on the study list?” or...
PSYC20007 Language Adam Osth How is memory for language different from memory for events? Episodic memory Memory for events! Even associations among arbitrary items (A-B, C-D) would be considered episodic memory Example questions: “What did you learn on the study list?” or “When did you meet this person?” Semantic memory Memory for general knowledge and meanings of words How is memory for language different from memory for events? Many differences between the two Semantic memory more resistant to forgetting or brain damage than episodic memory Retrieval from episodic memory often described as “mental time travel” – re-experiencing events Retrieval from semantic memory is often automatic and does not have the same experience What can we learn about language from studies on single words? The Lexical Decision Task Participants are presented with letter strings that are either words or nonwords, have to make a decision as to which is which DOG ”word” JKRLS ”non- word” BURGER ”word” SKRONT ”non- word” Lexical decision task Accuracy is often at ceiling (unless participants are pressured to respond very quickly) The dependent variable in lexical decision experiments is the response time (RT) Core component of these RTs assumed to be the latency of lexical access What enhances lexical access? Repetition priming Faster RTs for repeated words than non-repeated words, even if they are separated by other words Semantic priming Faster RTs for words semantically related to the just presented word E.g., faster RTs for “doctor” after being preceded by “nurse” What causes priming effects? Major explanation: spreading activation Reading a word increases its activation Also increases the activation of related words in the lexicon This activation decays over time, which is why priming effects are often short-lived Spreading activation models Even less activation from ROSES to FIRE Less activation from due to being ROSES to RED due to separated by a node their larger distance (RED) Very strong activation from ROSES to FLOWERS due to close Graph from Collins & proximity in the Loftus (1975) network The word frequency effect One of the major findings in lexical decision is the finding that high frequency words have faster RTs than low frequency words What do you mean by frequency? Frequency in the natural language – HF are common words while LF words are uncommon This is often quantified by a corpus analysis – counting the frequencies of each word across a large number of texts Older estimates of word frequency came from books (Kucera & Francis, 1967) Today, there are very large digital databases that are used Subtitles in films (SUBTLEX database) Conversations on Twitter The word frequency effect Word frequency effect implies that HF words are accessed more easily in the mental lexicon Some have even argued that the mental lexicon is searched in a serial fashion by word frequency (Murray & Forster, 2004) Advantage for HF words is eliminated when words are repeated Repetition boosts words to their maximum level of activation No RT difference between HF and LF words when they are repeated Data from Scarborough et al. (1977) Does the word frequency effect really reflect faster reading times for HF words? Research in eyetracking says ”yes”! Eyetrackers measure where people are looking at on a screen and for how long Rayner and Duffy (1986): longer gaze durations to LF words than to HF words while reading sentences Word frequency effects are in memory tasks as well! Recall tasks: advantages for high frequency words In free recall, these only occur in “pure” lists of words Word lists that are composed 100% of one type of frequency In other words, 100% of the study list words are HF words or LF words, but lists do not contain both HF and LF words There is little to no frequency effect when mixed lists of HF and LF words are studied! (Gillund & Shiffrin, 1984) Mixed lists = study lists containing BOTH HF and LF words This is referred to as the mixed-list paradox Largest difference between HF and LF words are in pure lists (100% HF or LF words) Less consistent results with mixed lists of HF and LF words – sometimes even an LF advantage! Word frequency effects are in memory tasks as well! Recognition memory shows an advantage for low frequency (LF) words LF words have a higher hit rate (more “yes” responses to studied words) …and a lower false alarm rate for LF words (fewer “yes” responses to new words) This pattern is referred to as the mirror effect The pattern is called the mirror effect because the hit rate (yes responses to targets) is almost a reflection of the false alarm rate (yes responses to foils, or lures) Data from Hemmer and Criss What is the cause of the word frequency effect? There has yet to be a single unified explanation of word frequency effects across all tasks Lexical decision Stronger ”base level activation” for HF words In other words, HF words are already active from their heavy repetition in language Free recall HF have stronger associations to other HF words, making them easier to learn associations in an experiment This is evident in free association data – HF words tend to elicit other HF words Recognition HF words are more similar to other HF words, both semantically and in terms of their perceptual characteristics (they have more overlap in their letters and phonemes) Why are there so many different explanations of word frequency effects? Word frequency is correlated with many other variables! Word length: high frequency words tend to be shorter Concreteness: low frequency words tend to refer to concrete things while high frequency words tend to be abstract Neighborhood size: high frequency words have more similar words in the lexicon E.g., a common word (e.g., HF) like HOT also has other similar words like TOT, ROT, and POT, but a less common word like COMPUTER doesn’t have as many similar words Does word frequency even matter on its own? Stronger predictor of lexical decision latencies: context variability (Adelman, Brown, & Quesada, 2006) Context variability is defined as the number of documents a word occurs in E.g., words like “where” or “people” are used across many linguistic contexts, words like “dog” or “baseball” are used in particular contexts Isn’t that the same thing as word frequency? No, they can dissociate! A high frequency word that is repeated a lot in one context is a low context variability word Likewise, a word could have low frequency overall but appear in a lot of different contexts Does word frequency even matter on its own? Adelman et al. (2006) found that context variability, not word frequency, predicts performance in lexical decision Word frequency had almost no effect when context variability was controlled High context variability words have shorter RTs than low context variability words Almost no effect of word frequency after contextual diversity is controlled Each point is a correlation between WF and RT for a given level of contextual diversity (CD) Data from Adelman et al. Clear negative relationship between context variability and RT when word frequency is controlled! Each point is a correlation between CD and RT for a given level of WF Data from Adelman et al. Why would there be such strong context variability advantages? Adelman et al. (2006) related these findings to the rational analysis of memory and language by John Anderson Rational analysis states that cognition – and memory in particular - is shaped around need probability in the environment Recency is one example: we tend to need recent things more than non- recent things, which may be why human memory is centered around recency High context variability words are more likely to be needed in future contexts than low context variability words Analogy: high context variability words are like tools that can be used in a lot of different situations (e.g., hammer, swiss army knife) – you’re most likely to use these in future situations Low context variability words have very specific or niche usages Context variability and memory Studies on context variability were directly motivated by findings that memory benefits for presentation of words in different contexts Stronger benefits of repetition when words occur in different contexts (e.g., different backgrounds or font colors) than when presented in the same context Stronger memory when repetitions are separated in time than massed consecutively (the spacing effect) Advantages for low context variability words have been found in episodic memory tasks Free recall and recognition memory show advantages for low CD words over high CD words Easier to be certain that a low CD word was present in the experimental context if it occurred in few other contexts How are words identified? The interactive activation model: combining top-down and bottom- up influences Classical approaches to language and word identification “Classical” (traditional) approaches emphasize rules When reading, we use rules about spelling-sound correspondence When hearing speech, we use rules about how words begin and end to understand where word boundaries are E.g., in English, words tend to end with consonants, so we can use this to infer when a word has ended and another has begun Most languages have exceptions to rules These exceptions are stored in long-term memory Reading: RULE: “X” is pronounced as \eks EXCEPTION: “Bordeaux” where the ”x” is silent Classical approaches to language and word identification Nonetheless, there are a number of problems with the idea that word perception only operates via usage of rules and exceptions Not always clear when to prioritize rules or exceptions Not clear how rules are acquired during linguistic development Brain damage/aging rarely shows the complete loss of rules Brain damage instead suggests “graceful degradation” – loss of some specific words or phrases Not clear how context affects perception Context influences letter perception Same letter in both cases, yet we perceive the left as an “H” and the right as an “A”… why? Context influences letter perception More likely to be an “H” than an “A” because “TAE CAT” doesn’t make sense! Context influences letter perception More likely to be an “A” than an “H” because “THE CHT” doesn’t make sense! Context influences letter perception Letters are perceived more accurately when they are in words than when they are in non-words or random letter strings Faster perception of the letter ”A” in “CATS” than in “ZAZX” Classical approaches did not have insight into this problem Interactive activation model of letters and word perception (McClelland & Rumelhart, 1981) This is a computational model of how we perceive words Computational model: a theory made explicit with computations We don’t know whether this is the truth or not – we can postulate some unobserved mechanisms and evaluate how well they can explain phenomena In this model, the model explains how context affects performance through the interaction of its various mechanisms, namely how top-down and bottom-up perception influence each other Interactive activation model of letters and word perception (McClelland & Rumelhart, 1981) Model consists of three layers: Feature layer: basic perceptual features like lines in text or handwriting Letter layer: abstract letters which may look like the features but may not Word layer: word representations in the mental lexicon Activation flows back and forth between these layers Higher activation = stronger perception Lateral inhibition In the word layer, the activations inhibit each other so only one word can be strongly activate This is why we tend to only perceive a single word rather than multiple words Interactive activation model of letters and word perception (McClelland & Rumelhart, 1981) Context influences perception because of a combination of top down and bottom up influences Bottom up: sensory perception from the environment Features from the stimulus – these become activated when a letter string is perceived The activations of the features are used to activate the letters that contain them The activations of the letters activate the words that contain them (e.g., the letters C, A, and T activate CAT but also CART) Top down: knowledge and expectations shaping our perception Word layer in the model ”feeds back” to influence the letters When words become activated, they add activation to their own letters, but inhibit letters that are not present in them E.g., for the word “cats” strengthens the letters “c”, “a”, “t”, and “s”, but will inhibit other letters like “x” and “z” that are not present in the word Words and letters influence each other Arrows indicate how Words are composed of letters (bottom up) activation flows in the But our knowledge of network the words influences how we perceive the letters (top down) From McClelland and Rumelhart (1981) Word layer: words inhibit each other, strengthen and inhibit the letter layer Letter layer: the letters that compose the words Feature layer: lines that compose the You probably know that this is the word “WORK” But how is the word identified? The final letter here is ambiguous. Based on the FEATURES (lines), it could be the letter R or K In the interactive activation model, the letters “W”, “O”, and “R” activate “WORK” in the WORD layer due to the strong match between these letters (bottom-up perception) The word layer feeds back to the letter layer – the word “WORK” activates its corresponding letter units (W, O, R, K) leading to the perception of the letter “K” (top-down perception) In the coming slides, we will walk through some hypothetical simulations of the interactive activation model to show how the different layers interact to produce perception Word Layer First three letters Final letter is less clearly are clearly identified from the features – multiple identified by the possibilities here! features in the previous display! Letter W1 O2 R3 K4 D4 R4 Layer “K” and “R” match the features of the final letter better than “D”, so they have stronger activation Word WORK WORD WEAK WEAR Layer Letter W1 O2 R3 K4 D4 R4 Layer “WORK” supported by activations of each of its letters in the letter layer Word WORK WORD WEAK WEAR Layer Letter W1 O2 R3 K4 D4 R4 Layer “WORD” is also supported by activations of each of its letters in the letter layer, but receives less activation because “D” has Word WORK WORD WEAK WEAR Layer Letter W1 O2 R3 K4 D4 R4 Layer “WEAK” has less activation because it is only supported by two letters Word WORK WORD WEAK WEAR Layer Letter W1 O2 R3 K4 D4 R4 Layer “WEAK” has less Likewise for “WEAR”! activation because it is only supported by two letters Word WORK WORD WEAK WEAR Layer Letter W1 O2 R3 K4 D4 R4 Layer Lateral inhibition – inhibition BETWEEN the word activations on the word layer. Word WORK WORD WEAK WEAR Layer Letter W1 O2 R3 K4 D4 R4 Layer Lateral inhibition – inhibition BETWEEN the word activations on the word layer. Word WORK WORD WEAK WEAR Layer Letter W1 O2 R3 K4 D4 R4 Layer Lateral inhibition – inhibition BETWEEN the word activations on the word layer. Word WORK WORD WEAK WEAR Layer Letter W1 O2 R3 K4 D4 R4 Layer Lateral inhibition – inhibition BETWEEN the word activations on the word layer. Word WORK WORD WEAK WEAR Layer Letter W1 O2 R3 K4 D4 R4 Layer Words with weak activations are heavily punished by lateral inhibition! This makes it such that there are only a small number of Word WORK WORD Layer Letter W1 O2 R3 K4 D4 R4 Layer Feedback from the word layer! “WORK” ACTIVATES letters contained within it, but INHIBITS other letters not in it Word WORK WORD Layer Letter W1 O2 R3 K4 D4 R4 Layer “WORD” does the same, but sends less activation and inhibition because of its lower activation Word WORK WORD Layer Letter W1 O2 R3 K4 D4 R4 Layer With many repeated iterations, “WORK” will inhibit “WORD”, and will continue to activate the letter “K” in the fourth position, causing even more activation back to “WORK” “WORK” and “WORD” are both perceived These are early in simulated reading, but activation “WORK” patterns from dominates as the time continues computational model! Higher activation indicates a stronger perception From McClelland and Rumelhart (1981) These are simulated activation patterns from the Final letter is computational equally likely to model! Higher be “K” or “R”, activation but “K” indicates a dominates as stronger time progresses perception From McClelland and Rumelhart (1981) The Interactive Activation Model The interactive activation model has become the cornerstone of theories of reading They are all centered around interactions between the bottom- up influences of perception and the top-down expectations from our understanding of words …and they can also be used to understand speech perception Speech Perception For a long time, a question in speech perception has been: how do we segment speech? Audio is completely continuous – there are no actual breaks between words when we speak, even though it sounds like there are! How do we mentally divide up continuous audio into discrete words? The TRACE model (McClelland & Elman, 1986) TRACE is basically the interactive activation model applied to speech perception Feature layer, phoneme layer, and a word layer ”Phonemes” refer to the basic sounds in a language Phonemes and letters are not necessarily the same thing! Letters can correspond to multiple phonemes The “c” in count is not the same as the “c” in cylinder One key difference: In reading, all of the letters are available simultaneously In TRACE, the phonemes are activated one at a time as the speech signal is processed IPA: International Phonetic Alphabet! What TRACE can explain Right context effects (Thompson, 1984) Many times spoken words have missing phonemes - they are either misheard or not pronounced, but we can understand words just fine! Gift being pronounced as ift – we likely still hear it as “gift” Because “ift” occurs after the letter “g”, it implies that what we hear in the present can alter our understanding of the past How does TRACE explain this? “Gift” may be the only word that “ift” can activate! “Gift” becomes activated and feeds back to /g in the phoneme layer What TRACE can explain Speech segmentation! Let’s take a pair of words like “Bob builds” – how do we segment this into two words? Word BOB Layer Phoneme layer \b \O \b Phoneme that is most recently heard is the most active Word BOB BUILDS Layer Phoneme \b \O layer \b \b \I As we begin hearing the word “BUILDS”, we begin getting information that is inconsistent with “BOB” – this gets more pronounced as time goes on! Word BOB BUILDS Layer Phoneme \b \b \O \b \I \L \d \z layer “BUILDS” is not only well supported by the incoming speech, but… Word BOB BUILDS Layer Phoneme \b \b \O \b \I \L \d \z layer Lateral inhibition at the word layer will allow “BUILDS” to suppress “BOB” even further What TRACE can’t explain Influence of semantics on word perception! Let’s say you hear “The _ing had feathers” Which word do you think this was? “WING” or “RING”? People generally think it’s “WING” because it’s semantically consistent with what came after (“feathers”: Szostak & Pitt, 2013) Another example: ”BIG GIRL” and “BIG EARL” can sound almost identical! How do we hear one but not the other? Linguistic context! We say “Big girls don’t cry”, not “Big Earls don’t cry!” TRACE would require some additional semantic layer to further constrain it What do these models tell us about language perception more generally? Reading and speech perception can be processed simply as an interaction between bottom-up perception and top-down knowledge The model can perceive these without using rules and exceptions! And this might be a good thing! Many linguists often discuss word perception involving rules, e.g., English words tend to end with hard consonants like /k But there are always exceptions – how does the system know how to manage both rules and the many exceptions that are present? What allows us to learn language? Language learning This is an extremely old question! Many philosophers have debated whether language is inborn or acquired BF Skinner in 1957 argued that language learning is learned via operant conditioning Example: If a child says “Mom can you give me milk?” and receives milk, there is reinforcement of the successful use of language Repeated reinforcement of successful uses of language lead to its acquisition Enter Noam Chomsky Chomsky, a linguist, wrote a scathing review of Skinner’s book He argued that it was virtually impossible for language to be learned via operant conditioning Key problem: the poverty of the stimulus Translation: Children just don’t exposed to that much language! Children often produce sentences that they have never even heard before A child saying “I hate you mommy!” Chomsky argued that language learning is innate and due to a universal grammar Impact of Chomsky’s critique Enormous! Led to a renewed interest in nativist accounts of language learning Many researchers have documented the extremely rapid rise in language use through the early years 5 years old learn on average of 2-3,000 words a year – many words a day! Impact of Chomsky’s critique Was also one of the cornerstones of the cognitive revolution in the 1960’s and the death of behaviorism Behaviorism was entirely about stimulus – response associations After the cognitive revolution, researchers began considering internal representations as a mediator between stimuli and responses These can take many forms! A universal grammar can be one – language that is mapped onto a grammar, comprehended, and an appropriate response is given Chomsky’s account of language comprehension Noam Chomsky argued that sentence comprehension is first and foremost dependent on syntax Syntax: rules for word order This is another example of a “classical” approach to language comprehension, also referred to as a “structural” approach Sentences are divided into their parts of speech and grouped into noun phrases and verb phrases The meanings of the words are only used to produce the meaning of the sentence after the syntax is processed Chomsky’s account of language comprehension Chomsky’s account of language comprehension Sentence (S) broken up into its parts of speech, such as adjectives (A), nouns (N), verbs (V), and adverbs Chomsky’s account of language comprehension Adjectives and nouns are grouped together into noun phrases (NP), and verbs and adverbs are broken up into verb phrases (VP) Chomsky’s account of language comprehension Meaning of a sentence can be produced by indexing the meaning of each word in the lexicon Problems with the classical account of sentence comprehension Sentence interpretations cannot always be recovered using rules! A great example: “The spy saw the policeman with binoculars” vs “The bird saw the birdwatcher with binoculars” The first case: the spy had the binoculars The second case: the birdwatcher had the binoculars What do these examples imply? The word meanings determine the structural interpretation, not the other way around Problems with the classical account of sentence comprehension Where do the syntactic rules come from? Chomsky assumed they were inborn But when in evolution did they arise? And which genes are responsible? Parallel distributed processing (PDP) accounts of language acquisition In the 1980’s, there were a number of neural network models of language acquisition that were developed These are also referred to as connectionist models The interactive activation model and TRACE are similar, but these models do not learn No changes in connections between words, letters, or phonemes occur during training These networks embody the following principles Learning by the difference between predictions and what was heard On each iteration, the model makes a prediction of some kind If the prediction is in error, the connections in the network are modified to better predict future outputs Parallel distributed processing (PDP) accounts of language acquisition Knowledge is distributed across many connections, like in the human brain Knowledge is not stored in fixed units anywhere like in classical accounts of language This allows for graceful degradation If you cut certain units or connections in the network, they don’t lose entire words or phrases Each word is represented across many units, so losing a small number is not consequential The only learning that takes place is modifications of connections in the network – no new units are added Connections in the model can be thought of as associations or relationships The models learn relationships between different levels of language These can be relationships between the way words appear and how they sound (Plaut et al., 1996) This can also be relationships between words in a sentence (Elman, 1990) Parallel distributed processing (PDP) accounts of language acquisition The networks do not start with any knowledge! Models often begin performing very poorly They learn across many, many iterations – adjusting in response to the errors they make Performance gradually increases through the course of training until it approximates human performance Errors made during the course of training are another testbed for such models These errors should resemble the errors that humans make Rumelhart and McClelland’s (1986) model of Past Tense Acquisition Past tenses are of interest because of irregular verbs Most verbs are made past tense by adding “-ed” However, there are several other past tense verbs such as ran and went that don’t conform to this pattern Even crazier – children often go through a phase where they get worse in their use of irregular past tense forms E.g., a child will use the word “ran” at around age 3 Later, the child says “runned”! Eventually, the child properly uses both regular and irregular verbs Steven Pinker and others have argued that this is due to the usage of rules The erroneous “runned” reflects an overusage of the rule The exceptions to the rule are eventually learned and this allows children to perform well Rumelhart and McClelland’s (1986) model of Past Tense Acquisition The model: Present tense verb is presented to the network on the INPUT layer Word is converted into “Wickelfeatures” – trigrams of the letters The word “Foster” broken up into all consecutive three letter combinations: FOS-OST-STE-TER The Wickelfeatures of the present tense verb are used to produce a past tense version of the word Converted back into the letter string of the predicted past tense word How does the model learn to produce past tense verbs? Connections are present between each layer When an error is made, the connections are adjusted based on the difference between the current prediction and the correct past tense verb Rumelhart and McClelland (1986) Present tense The past tense verb is input over word is output here Errors are It is a corrected by distributed modifying the representation – connections many units are through the used to represent entire network it Irregular verbs became worse, and then improve! Plot of learning across many training iterations What does the success of this model tell us about language acquisition? Rumelhart and McClelland argued that there is a sufficiency in general learning mechanisms, rather than rules or syntax Their model – and other PDP models – merely learn on the difference between the correct input and the prediction from the network What does the success of this model tell us about language acquisition? Steven Pinker and colleagues heavily criticized this model on a number of grounds The model does not succeed on all exception words There are certain neurological double dissociations that support the idea that verb use is subserved by two systems “Double dissociations” – manipulation of a variable affects system 1 but not system 2, manipulation of another variable affects system 2 but not system 1 Patients with Alzheimer’s, who have LTM deficits, have difficulty with irregular past tense verbs Patients with Parkinson’s disease, who have damage to the basal ganglia, have difficulty with regular past tense verbs but not irregular ones Severing connections in connectionist models tends to affect the irregular verbs but not the regular ones Other criticisms of connectionist models They are sensitive to the training sets Very different behavior emerges from different training sets! They can learn things people can’t learn The learning algorithms are so powerful they can reproduce just about any patterns with enough training They are difficult to understand! If you don’t understand how the model worked, you’re not alone They often reproduce patterns of interest after very small incremental adjustments to connections across thousands of iterations of training Often the creators of the models cannot explain how the models succeed So who is right? The debate continues to this day! Modern neural network models: deep learning models, which are used by Google and others Neural network models with many layers (around 10-20 layers) These models are used a lot for web searches, face recognition, etc Modern language production models like GPT-3 are extremely impressive, but not without their criticisms! To close To say this was the tip of the iceberg was an understatement! You could spend not just an entire class, not just an entire major, you could spend an entire lifetime studying language and still not understand everything about it! Learning Outcomes Understand the lexical decision task and the trends that are often found in the data Understand the spreading activation account of priming effects Understand the word frequency effect in language and memory tasks along with the context variability effect Understand the interactive activation model and how context is used to process letters Understand the TRACE model and how it is used to segment speech Understand Chomsky’s criticisms of Skinner’s account of language learning Learning Outcomes Understand Chomsky’s account of language processing and its criticisms Understand the PDP criticisms of the classical account of language comprehension Understand the Rumelhart and McClelland network of past tense acquisition and its criticisms