Chapter 8 - The Evolutionary Approach - Cognitive PDF
Document Details
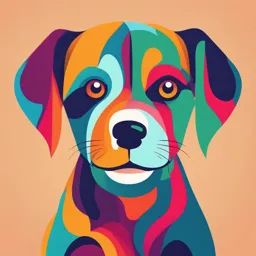
Uploaded by ImpeccableEpiphany1282
University of Gothenburg
Tags
Summary
This chapter details the evolutionary approach to cognition, exploring comparative cognition across different species and examining how environments influence cognitive skills. Evolutionary psychology is also discussed, looking at how the human mind originated. The chapter explores topics like natural selection, base-rate fallacy, and loss aversion.
Full Transcript
CHAPTER EIGHT THE EVOLUTIONARY APPROACH Change Over Time THE EVOLUTIONARY VIEW...
CHAPTER EIGHT THE EVOLUTIONARY APPROACH Change Over Time THE EVOLUTIONARY VIEW Learning Objectives Cognition doesn’t come out of “nowhere.” Natural forces create it. Animal and human cognition are products of these forces. If we After reading this chapter, wish to understand cognitive ability, then we need to understand you will be able to: how they work. These forces go by the name of evolution, a pro- cess that involves adaptation to changing environments. Animals, 1. Describe the process of including ourselves, have minds that allow us to think and survive in natural selection. a given environmental situation or niche. We have evolved capacities 2. List some cognitive skills that allow us to perceive, pay attention to, remember, and problem that are adaptive in certain solve so that we can acquire food, avoid predators, and mate and environmental niches. reproduce. All of our cognitive abilities are direct or indirect prod- 3. Discuss the role of brain– ucts of these earlier environments and have evolved in response to body size ratio in comparative such physical and social demands. cognition. In this chapter, we will examine the evolutionary approach and its relation to cognitive science from several perspectives. The first 4. List the six properties perspective is comparative cognition, where researchers examine of an evolved psychological the similarities and differences in cognitive ability between different mechanism. animal species. In this section, we will see how different environ- 5. Explain the base-rate ments have shaped different cognitive skills in animals. Birds that fallacy in judgment under need to store large numbers of seeds and nuts, for instance, have uncertainty. evolved special memory and search capacities to find their stored 6. Define loss aversion in caches of food. Animals that don’t have such needs may not develop behavioral economics. such special memory and search skills. So this section will show us how specific needs in particular environments give rise to particular 7. Discuss the evidence on sex differences in cognition. forms of cognition. The second perspective, evolutionary psychology (EP), is concerned with how the human mind came into existence. It attempts to describe the selection forces in our ancestral past that gave rise to our mental structures. Here, we will extend what we have learned in the comparative cognition section to people, show- ing which specific demands have driven different human cognitive skills. For example, we show that our ability to reason logically 229 seems to fail when a problem is described in abstract terms, but it is quite robust when couched in terms of a realistic social situation. Such results suggest that our reasoning capacity did not evolve in response to scoring higher on the SAT but in response to detecting cheaters in a group. A field that is related to EP is behavioral economics. It focuses on how evolution may have shaped the way we make decisions involving money, debt, and other resources. Behavioral economists apply evolutionary principles to the domain of economics. Humans are economic creatures. For many thousands of years, modern humans have lived in societies where we must produce, consume, and exchange goods and services. Living in these societies affects our reasoning with regard to money. Take, for instance, the situation where someone else is given money but only offers you a small amount. Most people under such circumstances refuse to accept it. This is an irrational decision as it is free money, but it makes sense in a society where people must share reciprocally to survive. The rejection is a statement of distrust and could even get the greedy lender kicked out of his group for failure to cooperate. In conclusion, we will wrap up the chapter with a brief discussion of sex differences in cognition and how evolutionary forces might or might not account for them. Other cognitive science approaches, in addition to those just mentioned, have adopted and used evolutionary principles. In the psychology chapter, we saw the func- tionalists asking the same questions that evolutionary psychologists ask. These function- alists wondered why we have certain mental abilities, for what purpose a given ability exists, and how a specific mental ability may have arisen. Evolutionary principles are also used in contemporary robotics, in which generations of robots are bred to produce robot offspring that are best adapted to the tasks that they have to carry out. A number of important themes are touched on in this chapter. Evolutionary psychol- ogists argue that many of our cognitive mechanisms are innate. This argument echoes the nativist position that we are born with knowledge. Evolutionary psychologists also argue that the mind consists of a set of special-purpose devices or evolved psychological mech- anisms that are activated only within specific environmental contexts. This runs counter to the tenet of artificial intelligence and cognition that states that the mind is a general- purpose computing device, capable of solving virtually all kinds of problems equally well. A LITTLE BACKGROUND: NATURAL SELECTION AND GENETICS Before delving specifically into the evolution of mental structures, it is helpful to review some basic concepts of biological evolution. The biologist Charles Darwin (Figure 8.1)—in his classic 1859 work, On the Origin of Species—outlines the basic prin- ciples of his theory of natural selection, which is a description of the process by which animal species change over time. Darwin noticed that animals vary (within a species as well as from species to species) in all sorts of ways—for example, they have longer or shorter legs, bigger or smaller ears, or different coloration. The fact that animals differ in their physical traits is known as variation. Second, parent organisms pass on some of their gene-based characteristics to their offspring. This process is called inheritance. 230 Cognitive SCienCe A change in environmental conditions can make it more likely that animals with a spe- cific trait will survive long enough to mate. Those without this trait might be more likely to die before mating. This process, whereby a particular attribute or attributes promote survival under altered conditions, is known as selection. The overall process by which animal species change in response to their environment is called adaptation. Variation, inheritance, and selection are the vital ingredients of species change. Looking at the fossil record, we can see that there had been alterations in the foot structure of horselike animals. Early progenitors of the horse had feet with several toes. Figure 8.2 shows that, over time, these toes became fused, and the hooves of modern-day horses came into being. Originally, the members of this progenitor species varied with respect to toe structure. Some animals had toes that were closer to each other and less distinct anatomically. These animals were perhaps better able to run in an emerging grassland environment, as their feet were likely to have given them better support and locomotive power on flat terrain. Presumably, these horses ran faster and so were able to escape predators more easily. They were more likely to survive and pass on their adaptive trait to future generations. Figure 8.1 The biologist Charles Darwin (1809–1882) proposed the theory of natural selection. Source: Photos.com/Thinkstock. Chapter eight the evolutionary approaCh 231 Natural selection is concerned with survival in a strict sense—that is, whether an organism simply lives long enough to reproduce, or not. Animals that possess an adap- tive trait are better equipped to deal with a changed or changing environment. They are better at escaping predators, locating food, and keeping warm or cool, and so they are likely to survive long enough to reproduce. Sexual selection, in contrast, refers to the adaptation of traits that improve the organism’s ability to attract a mate and, thereby, produce offspring. Some traits that are selected for naturally are not always selected for sexually. Females of some beetle species consider the presence of large horns on males of the species to be attractive. The males with these large horns mate more often and pass this trait to future generations more often. However, very large horns are also an impediment to survival. If they are too big, the males cannot easily escape predators such as birds. In this case, the optimum horn size represents a compromise between natural and sexual selection trading off against each other. Figure 8.2 Evidence from the fossil record showing the evolution of the hoof from a toed foot in horse species. (a) (b) (c) (a) Hyracotherium 55 – 45 million years ago (b) Merychippus 17 – 11 million years ago (c) Equus 5 million years ago COMPARATIVE COGNITION In this section, we will discuss evolution in broad terms of how it has affected cognition in nonhuman animals of different species. This will serve as an introduction to thinking about the relationship between evolution and cognition and will set the groundwork for our later discussion, where we will focus on human cognition only. 232 Cognitive SCienCe Cognitive Adaptation in Animals When looking at animals, we see that they are each adapted to a specific environment, what is called their ecological niche. Some birds migrate over vast distances and so need physical structures such as wings to enable them to fly, but they also need specific cognitive capacities that enable them to fly effectively. Studies show that carrier pigeons form an odor map of the environment that enables them to fly back more than 100 miles to a starting point after being released (Able, 1996; Wallraff, 1980). Pigeons may also be able to sense Earth’s magnetic field to navigate, in effect using a built-in “compass” to steer them in the right direction (Wiltschko, 1996). Some fish species, amphibians, and few aquatic mammal species have evolved the capacity to perceive electric fields. This sense enables them to detect prey that would normally be difficult to find using more typical senses, such as vision. Weak electric fields are given off by the muscles in an animal’s body and can change when the animal moves, revealing its location. In most cases, this electroreception is passive, but the African ele- phantfish also has an organ in its tail that produces a weak electric field. Using this organ, the fish can send out an electrical signal that is then reflected back to the fish by objects in its immediate surroundings. Sensors on both sides of the fish’s body pick up this elec- trical echo and allow it to estimate the distance of prey it can eat or obstructions in the dark (Von der Emde, Schwarz, Gomez, Budelli, & Grant, 1998). Apes, monkeys, cats, dogs, and some birds can understand that an object, once it dis- appears from view, still exists. This ability is called object permanence and develops in human children at the age of 12 to 18 months (Piaget, 1938/1952). Object permanence is a primitive form of concept formation, because the animal must retain the idea that the object is still present in the absence of perceptual evidence. Gagnon and Dore (1994) found that dogs successfully look for a favorite toy that is hidden behind one of three boxes. In the natural world, having object permanence is crucial in locating animals that have disappeared behind obstacles such as rocks or trees. Even if a prey animal is not at the location where it disappeared, this is still a good starting point to continue the search. Memory is a crucial adaptive capacity. It enables animals to remember where they have looked for something so that they don’t have to expend valuable time and energy revisiting locations already searched. The Hawaiian nectar-feeding honeycreeper can remember which flowers it has collected nectar from and will avoid going back to those flowers if it has recently fed from them (Kamil, 1978). Memory also allows animals to recall where they have stored food. The Clark’s nutcracker bird can store 33,000 pine nuts at 6,000 different sites during the summer and remember all these locations when it comes back to eat them in the winter and spring! Many different animals have both short-term and long-term memories and demonstrate some of the same memory phe- nomena that people do, including primacy and recency effects and proactive and retro- active interference (Bolhuis & van Kampen, 1988). In addition to memory, foraging skills are also something that humans and other animals have in common. Whether searching for food that they’ve stashed away themselves or foraging for new food, various animals exhibit a particular statistical pattern in how they search an environment for food. For instance, spider monkeys, penguins, turtles, and tuna all show the same statistical pattern of making many short Chapter eight the evolutionary approaCh 233 movements in a small region while foraging, then occasionally making one long move- ment to a distant region where search resumes with more short movements (Kello et al., 2010). This same statistical pattern, sometimes called a Lévy walk or a power law, also shows up when humans move their eyes across a photograph of a city street (Rhodes, Kello, & Kerster, 2014) or when they play a foraging video game (Kerster, Rhodes, & Kello, 2016) or even when they try to recall as many vegetable names as they can (Rhodes & Turvey, 2007). This Lévy walk pattern of foraging in an environment is a highly efficient search strategy that is even found in ancient fossil records of the move- ment of sea urchins (Sims et al., 2014). Whether you are recalling category names from memory, looking at a complex scene, or hunting for your missing car keys, a style of foraging that involves intensive search in a narrow area followed by occasional extensive search across to a distant area appears to be evolutionarily conserved across humans and many other animals. Nonhuman animals are also able to reason and solve problems as people do. Remember from the psychology chapter that Kohler’s chimps demonstrated insight learning. They were able after some time to realize that a box and a stick could be used together to get at food. Another reasoning skill is transitive inference. This is equiv- alent to deductive reasoning, which we previously introduced. In a transitive inference problem, an animal must realize that if A is bigger than B and B is bigger than C, then A must be bigger than C. McGonigle and Chalmers (1977) presented squirrel monkeys with paired colored food containers, only one of which contained a hidden peanut. If the red container contained the peanut in one trial and the green did not, on the next trial, the green container would contain the peanut but the blue would not. Based on this training, the animals could eventually learn that the red container would have the peanut rather than the blue in a test trial. Later research has shown that pigeons and rats can also solve transitive inference problems (Davis, 1992; von Fersen, Wynne, Delius, & Staddon, 1991). What is the evo- lutionary advantage of such a skill? According to Wynne (2001), it allows an animal to grasp rank orderings. This is particularly important in social animals with a hierarchical power structure. If Chimp A is dominant over Chimp B and Chimp B is dominant over Chimp C, one can infer that Chimp A is dominant over Chimp C without having to directly test this relationship, which could result in a fight and physical injury. Table 8.1 shows the cognitive skills of some other animal species and the adaptive selection pres- sures that gave rise to them. In each of the cases outlined above, specific selection pressures have shaped par- ticular cognitive skills. Note that these skills are often shared between members of different species. The key difference here is in the adaptive problem that needs to be solved, not the species in question. We would expect that all animals, regardless of their genetic and physical structure, might evolve similar cognitive capacities to solve similar problems. However, this is not an ironclad rule, as it is also the case that species can develop different solutions to similar problems; witness the different ways in which a bird’s and bee’s wings operate. In concluding this section, we need to mention that we do not address animal linguistic abilities here because those will be covered in the next chapter on language. 234 Cognitive SCienCe Table 8.1 Cognitive skills for different animal species and the adaptive function they serve. Cognitive Adaptive Species Skill Behavior Function Reference lions Counting the greater the to avoid potentially McComb, packer, and number of lions in a harmful interaction pusey (1994) pride that are roaring, with other the less likely another competing groups pride will approach. new tool use they nibble strips to obtain food Bluff, Weir, and rutz Caledonian off the stiff edges of (2007) crows leaves so that they can stick them into holes to extract insects. Baboons Deception Subordinate males to gain access to Byrne (1995) groom females who females for sexual are hidden behind reproduction rocks out of view from dominant males. Chimpanzees Self-concept Chimps who have possible basis for gallup, anderson, colored marks applied intentionality and and Shilito (2002) to their faces while theory of mind anesthetized will touch these marks while looking in a mirror. INTERDISCIPLINARY CROSSROADS: EVOLUTIONARY PROCESSES AND ARTIFICIAL LIFE Artificial life (ALife) is the study of humanmade living systems (Langton, 1989). ALife researchers systems that have been designed to behave produce artificial “creatures” that inhabit virtual in ways that simulate the behavior of natural environments. They then study the behavior of (Continued) Chapter eight the evolutionary approaCh 235 (Continued) these creatures and how they interact with one values, are randomly determined and, as such, another and with aspects of their environments. give Animat no inherent advantage. These What makes ALife interesting and relevant to starting classifiers may be considered equiva- cognitive science is that although the rules that lent to the genetic variation that exists among govern the creatures are often quite simple, the individuals or the members of a species and is emergent behavior of these creatures is complex shaped by selection forces. As Animat moves and can be considered intelligent. We discuss around its little world, classifiers are chosen and ALife in this chapter because these creatures their strength values are altered on the basis of learn and adapt via the use of evolutionary prin- a system of reinforcement in such a way that ciples (e.g., Holland, 1992; Smaldino, 2014). survival-enhancing actions become more likely. A good starting point for a discussion of However, this system alone is not enough to ALife is Animat (Wilson, 1985). Animat is a sin- ensure Animat’s survival. What Animat needs is gle animal that lives in a simple environment. a way of creating new classifiers that will bet- Its world consists of a square computer screen ter attune it to its world. This is where evolution taken up by food items, which Animat can eat, comes in. and trees, which are obstacles Animat must nav- Wilson (1985) equipped Animat with an igate around. Animat can sense its environment; evolutionary algorithm. Two classifiers that cor- it is aware of the objects that are in its immedi- respond to the same general type of action are ate vicinity. It can also act on its environment selected. Individual components of these clas- by moving one step at a time in one of the eight sifiers are then swapped. Segments of each clas- directions that are specified by the points of a sifier are randomly picked and exchanged and compass (N, NE, E, SE, etc.). then used to create new classifier offspring. This Animat is initially endowed with a set of is, of course, analogous to sexual reproduction rules that govern its performance. These rules in which genetic material from both parents is are in the form of classifiers, templates that used to create children. Wilson, however, also match an environmental condition that Animat allowed for asexual reproduction. In this case, is subject to at a given moment in relation to an individual classifiers are cloned (reproduced in action. Classifiers are really a modified version their entirety) to have random mutations. of if–then production rules. Each classifier has The result of all this is that Animat’s behav- an associated strength or fitness. Classifiers with ior becomes gradually more adaptive. Rather greater strength values are more likely to be than modify the probabilities of its selections used by Animat, as they promote Animat’s sur- that represent its existing genetic endowment, it vival. Animat’s actions also have consequences. is able to generate entirely new actions, at least A good action is one that promotes its survival, some of which will have beneficial results. It such as its moving to a location where there is has been shown that these Animats, along with food. Good actions are rewarded by increases in some more recent versions, learn quite rapidly. the strengths of their classifiers. Neutral actions, In one simulation, Animat was able to find food ones that have no effect on the well-being of in only four steps compared with a chance-level Animat, such as bumping into a tree, engender performance in which it did the same in 41 steps. no change in classifier strength. Other ALife programs demonstrate greater Animat is “born” with a collection of classi- complexity. Ackley and Littman (1992) have cre- fiers. These classifiers, along with their strength ated an entire population of creatures. Some 236 Cognitive SCienCe are herbivores that eat plants, while others are accepted definition of intelligence; therefore, carnivores that hunt and eat herbivores. Over the issue of whether such creatures possess it time, the herbivores learned to “climb” trees to remains unresolved. avoid predators, while the carnivores learned to The field of ALife is closely tied to the cannibalize their fellow carnivores that died of study of artificial intelligence. One of the key starvation! Other emergent social behaviors of differences between the two fields is that ALife ALife creatures include parasitism, symbiosis, attempts to model the behavior of the entire and flocking. organism in an environment rather than just ALife simulations show that evolu- the functioning of the mind or some specific tion-based software entities interacting in a mental process (Beer, 1990). Because the behav- diversified environment are capable of com- ior of ALife creatures is mostly reactive, their plex behavior. If we define intelligence as one’s understanding of their world may be referred ability to modify one’s behavior in an advanta- to as “minimally cognitive.” However, a form of geous way over time in the pursuit of goals, then knowledge representation can still be identi- Animat and other ALife creatures may be labeled fied in their internal processes (Beer & Williams, as intelligent. There is, however, no generally 2015). Comparative Neuroscience A common question that may come to mind when thinking about cognitive differences between species is brain size. One might expect that “smarter” animals have bigger brains, or brains that differ qualitatively from less intelligent species. Researchers first examined the size issue by ranking animals in terms of their brain size. When this is done, we find that elephants and whales have the largest overall brains, in excess of 5 kilograms. Intuitively, this doesn’t seem right since we humans assume we are the most intelligent species and we have smaller brains than those animals. Larger animals gen- erally have larger brains because they have a greater body size. What we need, then, is a measure that expresses brain size in proportion to body size. This measure is called the cephalization index (expressed as the letter K). High K values indicate that an animal has a larger-than-average brain for its body size, while a low K value indicates the opposite. Now, we see that humans are at the top of the list with K values of 0.89. In second place are dolphins with a value of 0.64. Primates and whales come in third with values of 0.2 to 0.3, whereas other mammals fall below 0.2. This is depicted in Figure 8.3. However, we need to interpret this index with caution (Kaas, 2000). Other factors not related to intelligence influence brain size. Birds, for example, have evolved compact brains because extra weight interferes with flying. Dolphins, on the other hand, may have exceptionally large brains because weight is less of an issue in a buoyant aquatic environ- ment. Another complication is that not all parts of brains are involved in cognition. The hindbrain in many animals does little more than regulate basic physiological functions, such as respiration, heart rate, digestion, and movement. So if we were to make our Chapter eight the evolutionary approaCh 237 Figure 8.3 The cephalization index allows us to compare brain size relative to body size across different species. 1.0 0.9 0.8 Cephalization Index (K) 0.7 0.6 0.5 0.4 0.3 0.2 0.1 0 Rat Sheep Dog Fox Whale Elephant Monkey Chimp Dolphin Human Species Source: Russell (1979). comparison correctly, we would need to express cephalization only in terms of the cog- nitive brain areas rather than the entire brain. The brain structure that underlies most cognition is the neocortex. In humans, the neocortex constitutes 80% of total brain volume. For primates, this is 50%; in rodents, 30%; and for insectivores (mammals that eat insects), only 13%. However, even this measure has problems. To start, only mammals have a neocortex. Other species, such as birds, do not possess one, so this prevents us from comparing neocortex size across all species. Figure 8.4 shows the brains of several different animals. Not only can brain mass and neocortex mass provide insight into what makes certain species appear more intelligent, but the neural connectivity of those brains gives perspective on this as well. The neural connectome of humans shows more connections among nearby brain regions than is seen in monkey and cat brains (Van den Heuvel et al., 2016). That said, a mouse brain, with its large brain mass relative to body size, also has a rather dense neural connectome as well. 238 Cognitive SCienCe Figure 8.4 Brain size in several different animal species. Human Chimpanzee Mouse Another neural marker for intelligence may be von Economo neurons, also called spindle neurons. In humans and apes, these cells are found in the anterior cingulate cor- tex and the frontoinsular cortex. In people alone, they are also located in the dorsolateral prefrontal cortex (Fajardo et al., 2008). They are also found in beluga, humpback, killer, and sperm whales; bottlenose dolphins; and elephants. They allow for the fast transmis- sion of signals across larger distances as would be necessary in animals with larger brains. These cells, in conjunction with a small-world network architecture, could promote rapid global information flow. Chapter eight the evolutionary approaCh 239 The location of von Economo cells in the cingulate cortex is no accident. The ante- rior cingulate cortex plays a vital role in higher cognitive function. It is involved in focused problem solving, the recognition of having made a mistake, and the initiation of correcting a mistake (Allman, Hakeem, Erwin, Nimchinsky, & Hof, 2001). Neurons in this region become active in experiments where participants must differentiate a correct response from conflicting cues and when there is a discrepancy between a desired state and a current state. It has been hypothesized to play a role in self-control and in the regulation of emotion and drive states, all signs of higher intelligence. So what other measure can we use? One suggestion is the number of nerve cells. Unfortunately, the size and density of neurons in brains of different species differ. Some species, such as dolphins, have large cortical volumes but relatively few neurons. Other species, such as humans, have smaller volumes but more neurons. Although the compari- son between dolphins and humans on this measure may correspond to actual intelligence differences, we cannot be sure that some other neuron property such as the number of dendritic connections is confounded with number. Future possibilities might include physiological function measures rather than anatomical differences. It may turn out that evolved intelligence also correlates with the rate of cortical development in the young animal (Finlay & Workman, 2013), the ease with which new synaptic connections are forged (Anderson & Finlay, 2014), or the complexity of neural circuitry (Van den Heuvel et al., 2016). Evaluating the Comparative Approach Two methodological issues plague the comparative approach (Wasserman & Zentall, 2006). Many of the studies investigating cross-species animal abilities have used different techniques. This makes them hard to compare, since any differences can be attributed to the different ways in which the experiments were conducted. A second problem is that some of the studies are done in artificial laboratory settings, while others are based on the naturalistic observation of animals in the wild. The laboratory studies can control for extraneous variables or alternate explanations of behavior but may be looking at actions the animals wouldn’t normally engage in. The naturalistic observation studies yield nat- ural behavior but have less control over other factors. Perhaps one of the largest problems in this field is in how one attributes mental states to animals. People throughout history have had a tendency to attribute humanlike characteristics to animals, what is called anthropomorphism. Unfortunately, animal researchers are not immune to this tendency and sometimes ascribe more advanced cog- nitive abilities to animals than the data actually warrant. However, we must also guard against anthropodenial, which is a blindness to the humanlike characteristics of animals, or the animal-like characteristics of ourselves (De Waal, 1997a). We should not assume that humans are categorically unique in the arena of animal cognition. After all, we share a large number of brain structures and behaviors with many of them (Finlay & Work- man, 2014). According to this perspective, we are not so different from some animals, and it may be easiest and most accurate, at times, to describe their behavior in the same way that we would describe our own, in terms of internal intentions, desires, and beliefs. In fact, in much the same way that Howard Gardner (2011) suggested that there are 240 Cognitive SCienCe multiple types of intelligence (in Chapter 3), Frans De Waal (2016) suggests that multi- ple types of intelligence may be found in the evolutionary tree of various animal species. For example, some of the forms of social intelligence displayed by nonhuman primates may have evolved into our own human versions of empathy, morality, and justice. However, we still have to be careful about potentially overinterpreting the behavior of nonhuman animals. Cheney and Seyfarth (1990) observed a low-ranking vervet mon- key named Kitui utter a leopard alarm call whenever a new male tried to join his group. The addition of a new male would lower his status and introduce more competition for him for food and mates. The call caused all the monkeys to scatter into the trees and prevented the entry of the new male. At first blush, Kitui’s action might make it seem as though he was capable of deceit and perhaps had a theory of mind, an understanding that the other members had beliefs. However, further study showed that he came down from the tree and uttered the cry again closer to the intruding male. By coming down from his hiding spot, Kitui would reveal to the others that he really didn’t believe a leopard was there. This would negate any deceitful action and cause the other group members to suspect him. So it is perhaps unlikely, given this behavior, that he actually intended to deceive or had a developed theory of mind. Other examples of overattributing sophisticated cognition to animals come from studies of imitative behavior. One reason an animal might imitate another is because it understands that the other animal is performing an action to achieve a desired goal, such as acquiring food. The animal in question would then copy this action to obtain the desired goal for itself. Studies of potato washing in Japanese macaques and following behavior in ducks have instead shown that imitation can be a consequence of stimulus enhancement, where attention simply has been drawn to the location or the behavior and there is no inference of desired goals. Many other abilities ranging from concept formation to self-perception can sometimes be accounted for by simpler explanations that don’t require sophisticated thought processes. Not only is this true for nonhuman animals, but it is true for humans as well. EVOLUTIONARY PSYCHOLOGY We humans don’t look like much. We are thin skinned—and almost gawky looking when we stand upright. To a passing predator, we must seem like a particularly easy kill. We have no armor, fangs, or claws with which to defend ourselves, nor are we particularly strong or fast in comparison with other animals. So what has enabled humans to survive and thrive in a harsh world? The answer, in a word, is our minds. The species Homo sapi- ens has the most sophisticated mental faculties of any species on Earth. Our capacities to think, to use language, to solve problems, and to strategically coordinate are greater than those of any other animal. An advanced mind allows for planning, communication, social cooperation, and the development of technology, which give us a tremendous competitive survival advantage. But how did the human mind come into existence? What processes formed it? These are some of the questions asked by the evolutionary approach. In evolutionary theory, changes in an environment give rise to corresponding physical changes in an Chapter eight the evolutionary approaCh 241 animal species that better enable it to survive. Giraffes, to use an often-cited example of adaptation, have developed longer necks over many thousands of generations to better enable them to get at treetop vegetation. Similarly, we can consider the mind to be such a body structure—comparable to the giraffe’s neck—that has been shaped by evolutionary processes. Evolutionary psychology (EP) not only attempts to describe these forces that are believed to have fashioned the human mind but also is concerned with understanding the mind’s organization and function and how interaction with an environment produces observable behaviors. Evolutionary psychologists face a fundamental problem. They need to under- stand the selection forces that gave rise to mental structures but cannot know pre- cisely what these were because they correspond to events that were taking place far back in human prehistory. Evolutionary psychologists believe that the period of time during which many human psychological mechanisms evolved was the Pleistocene era, which began approximately 2 million years ago. This period is referred to as the environment of evolutionary adaptation (EEA; Bowlby, 1967). Because we cannot go back in time to study what occurred during this period, we must make inferences about the physical and social environment of that time based on evidence of various types. Buss (1999) describes the types of evidence used to test evolutionary hypoth- eses. These include archeological artifacts and records, data from studying hunter– gatherer societies, systematic observation of human behavior, and self-reports in the form of interviews and questionnaires. EP also relies on a variety of methods for the testing of its hypotheses. Among these are comparisons across species, comparisons of males and females within a species, and experimentation (Buss, 1999). The evolu- tionary approach thus adopts a wide variety of methods rather than relying exclusively on the scientific method. Evolved Psychological Mechanisms Evolutionary biology focuses on all aspects of an animal’s makeup. It is concerned with how selection forces came to shape the individual parts of an organism and how these parts became integrated. The scope of EP is narrower—it examines the impact that these forces had on psychological mechanisms only. Whereas evolutionary biology looks at organs and organ systems, EP looks primarily at a single organ—the brain. This perspec- tive views the mind as a collection of psychological mechanisms that have evolved and attempts to describe how each came to be and how each operates. In the 1960s, the view in cognitive psychology was that the mind is a general- purpose processor. This processor can be “fed” a problem in any format and can come up with a solution by applying a known set of rules. Modern computers operate in this way. The advantage of this process is that it can solve all problems of a given kind, assuming that the correct values of the problem’s variables are mapped onto the algo- rithm designed to solve the problem correctly. The evolutionary psychology perspective adopts a very different view. It argues that the mind is not general in any way but, instead, a set of many specific capacities. Each of these capacities is called an evolved psycholog- ical mechanism. 242 Cognitive SCienCe Cosmides and Tooby (1992) refer to the mind as a “Swiss army knife.” In this anal- ogy, each part of the knife unfolds to solve a different problem: The corkscrew takes out corks, the scissors cut paper, and so on. Likewise, each evolved psychological mechanism is assumed to have evolved in response to the need to solve a specific problem of adapta- tion and is activated by the contextual information of that problem alone. They are thus examples of modules we discussed previously. The general characteristics of modules are discussed early in Chapter 4. Table 8.2 lists the six properties of evolved psychological mechanisms and illustrates them with an example. To sum up, EP views the mind not as a one-size-fits-all machine but as a collection of functionally distinct mechanisms. Evolutionary forces operating over vast periods of time created each of these mechanisms. Each evolved to solve a problem our ancestors faced during the EEA. It is important to keep this multiple-mechanism view in mind as we describe specific examples of cognitive processes. According to EP, for every possible cognitive process, it is not the brain as a whole that is acting (according to this perspec- tive) but a particular component of the brain. Thus, when we talk about the evolution of memory or logic, we are talking about specific mechanisms that may constitute only a part of the brain’s memory or logic systems. Table 8.2 The six properties of an evolved psychological mechanism. Property Explanation Example: Arachnophobia 1. purpose to solve a specific problem prevents being bitten by a of survival or reproduction poisonous spider 2. perceptual focus Mechanism triggered only by two body parts with eight a visual image of a specific legs stimulus 3. Communication input of mechanism informs organism knows adaptive the organism of the type of problem is one of death, not problem food selection or mating 4. output input produces a behavioral Scream or swipe spider off output or response body 5. type of output Can be physiological heart acceleration and activity, input to another sweating, decision to swipe psychological mechanism, or run away, resulting or a behavior behavior 6. Solution Mechanism evolved to removing spider from provide a solution to the immediate vicinity reduces problem threat Source: Adapted from Buss (1999). Chapter eight the evolutionary approaCh 243 Evolution and Cognitive Processes In the following sections, we will describe specific evolutionary hypotheses that pertain to several domains in cognition and the evidence that supports them (Gaulin & McBurney, 2001; Palmer & Palmer, 2002). These hypotheses concern cognitive processes only, which include categorization, memory, logical reasoning, judgment under uncertainty, and language. The motivation that underlies this research is the desire to arrive at an evolutionary account of how these cognitive processes arose. In other words, why do we categorize and remember things the way we do? Why are we so poor at reasoning log- ically and making judgments under uncertain conditions? How is it that human beings alone of all the species on Earth have developed such a complex linguistic ability? Categorization Research conducted by Eleanor Rosch (1973, 1975) shows that people form mental categories not in an “either–or” fashion but, rather, in a continuously graded way. Why should this be the case? Why do we not lump things together into one group on the basis of a single characteristic—for example, “red objects” or “curved objects”? The organi- zation of concepts, it turns out, is governed by “fuzzy” categories, whereby a particular item can be more or less representative of the category. In this section, we will exam- ine why humans form concepts in this way. Recall the finding—from the chapter on networks—that a bird such as a robin is thought of as being more representative or pro- totypical of the category of “birds” than another type of bird—for example, a penguin. This is because robins tend to come closer to our idea of what a bird is—something that is small, sings, flies, and so on. Robins are thus more typical of “birds” than are penguins (see Figure 8.5). Participants in experiments respond more quickly when they are asked to make a true/false judgment about the statement “A robin is a bird” compared to the statement “A penguin is a bird”—the so-called typicality effect (Rips et al., 1973). In fact, if they are responding to the word penguin with a computer mouse, their mouse movement may curve slightly toward the “mammal” response option before it finally lands on the “bird” response option (Dale, Kehoe, & Spivey, 2007). Categories of the sort found in nature are almost always continuous: They contain items that span the entire spectrum of typicality—from being very representative and prototypical to being quite unrepresentative and perhaps easily mistaken for items that belong to another category. The categories that result from human categorization pro- cesses, therefore, mirror the distribution of natural objects in the world. This suggests an evolutionary process whereby we came to acquire concepts and a sense of their relat- edness on the basis of how the items that these concepts represented were ordered in the natural environment. Further evidence in support of this notion comes from Boster and D’Andrade (1989). They found that professional ornithologists as well as those who had little experience and/or knowledge of birds all grouped stuffed bird specimens in similar ways. Their groupings demonstrated typicality and hierarchical effects reflective of what exists in nature. What purpose do typicality-based categories serve? They must have provided us with some sort of selective advantage—otherwise, they would not have arisen. The 244 Cognitive SCienCe Figure 8.5 Which of these birds looks more like a bird? What does this tell you about your concept of “birds”? advantage is this: If we know something about an item we have experienced, then it becomes possible to form judgments that have to do with related items (Rips, 1975). Imagine encountering for the first time a plant with four leaves and purple spots. After eating this plant, you become ill. Several months later, you stumble across a plant with three leaves and reddish-purple spots. Would you eat it? Probably not. There is a rea- sonable chance that the two species of plant, because they are similar, share properties. Typicality categories thus allow us to capitalize on past experience. We generalize from what we know to what we don’t know. Memory Other approaches in cognitive science tend to study basic memory processes. You will recall that cognitive psychologists are concerned with, among other things, how informa- tion is converted from short-term into long-term memory. Neuroscience can identify the brain structures that underlie this transformation. Connectionists can then create artificial networks to model the process. EP, on the other hand, cares less about memory function per se and more about the purposes our memories serve. EP researchers want to know why our memories are the way they are. Specifically, they want to know how the demands of our ancestral environment have shaped memory and have made our memories operate the way they do. In this section, we will examine one aspect of memory—the relationship between the frequency of contact with an item in one’s environment and recall. Every day, we are bombarded with a tremendous amount of information. Only a small fraction of it is actually remembered. This is a good thing, as most of the Chapter eight the evolutionary approaCh 245 information is trivial. For instance, can you remember the color of the shirt your best friend was wearing the previous Monday? It would make sense, then, for us to remember only the information that may be of some benefit to us and to forget that which doesn’t benefit us. But what constitutes beneficial information? What things should we be bet- ter at remembering? An evolutionary account avers that information that we encounter more often should be better remembered. The more often we encounter an item, the greater its relevance to our daily life and our survival. And the less often we encounter an item, the lesser its relevance to our survival. Remembering your roommate’s name is important—because he can help you in a variety of ways. You would also be likely to hear your roommate’s name quite often, as you see him practically every day. Remembering the name of your niece who lives in another country is less important to you, as you are far removed from interacting with her on a daily basis. Correspondingly, you would very rarely hear her name, assuming that it is not the same as that of someone else you know better. One way to test this hypothesis is to compare the frequency of our encounters with specific items with our ability to remember them. Clearly, this relationship will be positive: The more frequently we encounter items, the better our memory of them. In addition, the relationship is described by a power law function, similar to the statistical pattern seen with foraging skills in the comparative cognition section above. This spe- cific relationship is represented when we plot on a log–log graph our ability to remem- ber items versus how often we encounter them. Anderson and Schooler (1991) tested this relationship. They examined several real- world sources of information and determined for each type of information the statistical function for the frequency of occurrence and recall. That is, they determined the math- ematical relationship specifying how many times a piece of information occurred and how accurately it was remembered. They used newspaper headlines, utterances made by parents to their children, and names of the authors of e-mails that were received by one of the researchers over an approximate 4-year period. For all these sources, the probability that a particular utterance or word would crop up a second time was a decreasing function of the number of days since its previous appearance. For example, if the word north appeared in a newspaper headline yesterday, there would be a rela- tively good chance that it would appear again today, presumably because it was part of an ongoing news story. If north had been in the paper only in the previous week, the chances that it would appear again today would be much lower. The researchers then measured memory for these items and found that the function that described proba- bility of recall over time was in accordance with the probability of occurrence. This shows that our memory for items is directly proportional to how often we encounter them in the environment. Our memories are better for items that we encounter more often and poorer for those we encounter less often. This finding supports the notion that our memories have evolved such that they retain items only to the extent that we need them. From an evolutionary point of view, this makes sense. We should remember those things that we encounter frequently, as it is very likely that they are of importance to us, and forget those things that we encounter infrequently, as it is likely that they are not of much use to our survival. 246 Cognitive SCienCe Logical Reasoning Recall from the chapter on philosophy that deductive reasoning involves the application of logical rules to a set of premises. A conclusion is then derived: If the premises are true, then an application of the rules will always yield a correct conclusion. Deductive thought is thus a valid way of garnering new information about the world. Logic is used in other approaches to cognitive science too. Connectionists use the rules of logic to describe how neural units interact. Researchers in artificial intelligence create computer programs that use logic to generate new information from existing data and to solve problems. In the evolutionary approach, investigators want to know why we as humans are so bad at thinking logically and to relate this deficiency to the kinds of social condi- tions our early ancestors may have faced. If you have ever taken a course in logic, you may have wondered why the subject is so difficult. The rules of logic are straightforward. All one has to do is memorize the forms of these rules and then plug into them the relevant aspects of the problem. In this fashion, one can then apply those forms to any problem and be assured of obtaining a correct solution. Unfortunately, it is not as easy as it sounds. Most people find it difficult to reason logically. Interestingly, however, there is an exception. People can reason logi- cally under certain conditions. The evolutionary approach can tell us why we are so bad at logical reasoning in general and what the exception is. The Wason selection task is designed to measure a person’s logical thinking ability. Figure 8.6 gives an example of such a task. Take a minute now and try to solve this prob- lem. You probably found that it was quite difficult. Next, try to solve a different version of the problem, shown in Figure 8.7. When you have finished—or if you are having trouble—consult Figure 8.8 for the correct solutions. If you are like most people, you would have found the “bouncer” version of the problem to be much easier. Why is this? Both versions have the same underlying logical structure and should be of equal difficulty. Figure 8.6 You have been hired as a clerk. Your job is to make sure that a set of documents is marked correctly, according to the following rule: “If the document has an E rating, then it must be marked code 4.” You have been told that there are some errors in the way the documents have been coded and that you need to find the errors. Each document has a letter rating on one side and a numerical code on the other. Here are four documents. Which document(s) do you need to turn over to check for errors? E G 4 8 Chapter eight the evolutionary approaCh 247 Figure 8.7 You have been hired as a bouncer in a bar, and you must enforce the following rule: “If a person is drinking vodka, then he or she must be over 20 years old.” The cards depicted in the figure contain information about four people in the bar. One side of each card lists a person’s age, and the other side specifies what he or she is drinking. Which card(s) do you need to turn over to make sure that no one is breaking the law? vodka Pepsi 30 yrs 17 yrs Figure 8.8 Solutions to the “clerk” and “bouncer” problems. Solution to the “clerk” problem. Rule: E-rated documents must be marked code 4. Step 1: Check E-rated documents to see if they meet the coding criterion. Step 2: Check any documents not matching the coding criterion (e.g., code 8) to see if they are E-rated. No more checking necessary: G ratings (paired with any code) could not violate the rule. Code 4 documents do not have to be E-rated. Solution to the “bouncer” problem. Rule: Vodka drinkers must be over 20 years old. Step 1: Check vodka drinkers to see if they meet the age criterion. Step 2: Check anyone who does not meet the age criterion (e.g., 17 years old) to see if they are drinking vodka. No more checking necessary: Pepsi drinking (at any age) could not violate the rule. People over 20 years do not have to drink vodka. According to Cosmides and Tooby (1992), the bouncer problem is easy because it involves cheater detection, the ability to discern who has taken as his own something he does not deserve. They argue that during the EEA, human societies were characterized by reciprocal altruism, the sharing of hard-won resources among group members. In 248 Cognitive SCienCe this kind of social cooperative, it is important to detect “freeloaders”—those who take more than their fair share. Cosmides and Tooby believe that this social environment shaped our reasoning abilities such that when we are presented with a logic problem that involves cheating, we can solve it. If a logic puzzle possesses the element of a cheater, then the evolved psychological mechanism for cheater detection is activated. This mech- anism “understands” the rules of logic and can apply them, but only in this specific context. Note that this understanding stands in stark contrast to that which maintains that the mind is a general-purpose processor that can solve logic problems of any sort. There is abundant experimental evidence in support of the cheater detection model of reasoning. One study comes from Gigerenzer and Hug (1992). They presented par- ticipants with two Wason selection tasks, both of which entailed a social contract of the following sort: “If you stay overnight in a mountain shelter, you must help out by carry- ing up some firewood to add to the supply.” Both of these tasks were roughly equivalent with respect to content and logical structure. The only real difference between the two was that one of the tasks involved cheating, while the other did not. The results showed that 78% to 90% of the participants were able to solve the cheating version. Only about half as many participants were able to solve the noncheating version. This shows that the framing of a logic problem as a social contract alone is not enough to elicit the problem-solving skill; it is elicited by a social contract that specifically involves cheating. In another study, it was discovered that even children a little older than 2 years of age seem to have this cheater-detecting capacity (Cummins, 1999). Judgment Under Uncertainty In many situations in life, we have to make a decision in the absence of complete infor- mation. What are the chances that Mary will go out with me? What is the likelihood that I will make the college swim team? As is the case with logical reasoning, most people are quite poor at solving problems of this type—problems that involve probability (Nisbett & Ross, 1980; Tversky & Kahneman, 1974). Why is this? One reason is that humans facing these kinds of problems rely on heuristics. A heuristic is a mental “rule of thumb” or strategy that is a fast and easy way of solving a problem. The problem with heuristics is that they aren’t always right. Heuristics can lead us to commit fallacies—fundamental misunderstandings of statistical rules. The role that evolution has played in shaping our thinking in this situation is discussed below. Tversky and Kahneman (1974) were the first to study heuristics and fallacies. In one study, they gave the participants a description of a person. Participants were also told that this person belonged to a set of 100 people. There were two conditions in the experiment. In the first, the participants were told that the set consisted of 30 lawyers and 70 engineers. In the second, they were told that the set contained 70 lawyers and 30 engineers. Here is a sample description: Jack is a 45-year-old man. He is married and has four children. He is generally conservative, careful, and ambitious. He shows no interest in political and social issues and spends most of his free time on his many hobbies, including home carpentry, sailing, and mathematical puzzles. Chapter eight the evolutionary approaCh 249 Is Jack a lawyer or an engineer? The participants answered overwhelmingly that Jack was an engineer, even in the condition in which engineers made up only 30% of the set. Tversky and Kahneman (1974) argue that this kind of error is due to a representa- tiveness heuristic, the tendency to judge an item on the basis of its perceived similarity to other items. Because the description of Jack fits the stereotype of the engineer, we consider it very likely that he is one. The representativeness heuristic in this case is accompanied by the base-rate fallacy, ignorance of the base rates that define the set of 100 people. Here is another example of our difficulty in making judgments under uncertainty (Tversky & Kahneman, 1974). Consider the following description: “Linda is 31 years old, single, outspoken, and very bright. She majored in philosophy. In college, she was involved in several social issues, including the environment, the peace campaign, and the antinuclear campaign.” Which of these statements do you think is more likely: “Linda is a bank teller” or “Linda is a bank teller and is active in the feminist movement”? Most people overwhelmingly choose the latter sentence, even though it cannot possibly be the more likely of the two, since there are always going to be more tellers than feminist tell- ers (see Figure 8.9). Participants again rely on the representativeness of Linda’s descrip- tion, which fits the stereotype of the feminist. In this instance, participants ignore the conjunction rule, which states that the probability of encountering those who are both feminists and tellers is lower than the probability of encountering those who are one or the other. The making of this error is known as the conjunction fallacy. Figure 8.9 A Venn diagram illustrating the conjunction rule. Circle A represents all bank tellers. Circle B represents all feminists. Region C, the portion of overlap between the two circles, represents feminist bank tellers and will always be smaller than the regions that correspond to the number of bank tellers or feminists, considered separately. A C B Bank tellers Feminists Feminist bank tellers 250 Cognitive SCienCe So is it fair to say that humans cannot think in terms of probability or uncertainty? From an evolutionary standpoint, one would guess that this is not a fair statement, as uncertainty is an inherent characteristic of the natural world. Gigerenzer and Hoffrage (1995) argue that the difficulties that human beings have in this area, which are docu- mented in the literature, stem not from a fundamental inability on our part but from the way that these types of problems are usually structured. Many of these problems entail probabilities that are expressed as fractions or percentages rather than as frequencies, expressed as numbers of occurrences. Probabilities are more complex numerically, as they require dividing one number by another. They also are a rather recent invention in mathematics, just a few hundred years ago. By contrast, modern humans have been encountering frequency data as part of their daily existence for many thousands of years. We modern humans can easily think about how many times we’ve eaten today or how many rabbits we caught yesterday or how many games the New York Yankees have won this season. It makes sense, then, that the psychological mechanism that underlies uncertain reasoning should be sensitive to frequency data. It turns out that this is, indeed, the case. Gigerenzer and Hoffrage (1995) assessed a number of uncertainty problems and converted them from a percentage to a frequency format. They found that roughly three times as many participants answered correctly. Their finding, similar to the findings of the logical reasoning study illustrated earlier, is another example of how problem solving is narrowly tuned to a particular type of information. This attunement to a par- ticular type of information is the second major characteristic of evolved psychological mechanisms and again runs contrary to the notion of the mind as a general-purpose processor—at least within these problem-solving domains. Yet another example of poor probabilistic reasoning is the gambler’s fallacy. Do you think that someone who has been losing at a slot machine for hours is more likely to have a jackpot coming up soon than a person who has been losing at one for only 10 minutes? Do you think that a fair coin that has come up “heads” eight times in a row is more likely than usual to come up “tails” on the next toss? If you answered yes to either of these questions, then you have been taken in by the gambler’s fallacy. It is the belief that the probability of an event is influenced by how often it has occurred in the past—even when those events are independent of one another. If a particular outcome has turned up quite often in the recent past with a random chance event, people are apt to believe that there is an increased likelihood that a different outcome will occur next. In reality, the probability of each event in these gambling scenarios is independent. The chances of winning at slots the 100th time are exactly the same as for the first. The chance of getting a “heads” in a fair coin flip is 0.50 on every flip. Why, then, do we persist in holding such beliefs? One possible explanation is that independent outcomes are typically the product of artificially engineered devices that are not encountered in the natural world, such as dice or roulette wheels (Pinker, 1997). In nature, the past often is the best predictor of the future. If it was sunny yesterday, there is a good chance that it will be sunny today. If a herd of mammoth migrated through a particular region the previous year at this time, the chances are that they will do it again this year. Natural events tend to have regular time courses and shared causes; therefore, some knowledge about the way an event unfolded in the past is informative about the Chapter eight the evolutionary approaCh 251 way similar events will unfold in the future. One might think that this evolutionary experience would cause the gambler’s fallacy to go in the opposite direction (i.e., a person who sees a coin flip result in “heads” eight times in a row would conclude that the next flip is more likely to be “heads” again). However, humans are accustomed to alternating cycles between opposing states, such as night following day or a half-moon following a full moon. Therefore, in considering opposing possibilities and taking the past into account, we sometimes fall prey to the gambler’s fallacy. Language Language is so important in cognitive science that it is a separate field of study. Linguists study the organizational rules of language and how language is acquired. They also construct models of language processing. From an evolutionary standpoint, the focus again is not so much on processing but on how language came to be. Because lan- guage possesses a social function, evolutionary researchers want to know what aspects of early human social life might have given rise to its development. One of the most obvious things that sets H. sapiens apart from other species is lan- guage. Other animals can communicate, and primates can be taught rudimentary lin- guistic skills, but nowhere else in the animal kingdom do we see such a sophisticated use of and widespread reliance on language as in the human species. The biological and cultural evolution of language is believed to have catalyzed the development of intelli- gence, social cooperation, and other phenomena, in the process of which human beings derived great benefit. Researchers in this area have argued that language is an evolved trait and have provided several theoretical explanations for how it could have arisen. Nonevolutionary accounts of language are discussed in Chapter 9. Converging lines of evidence point to the possibility that human language ability has evolved (Palmer & Palmer, 2002). First, languages share a number of universal com- monalities (Chomsky, 1986; Hockett, 1960). Among these are meaningfulness, the abil- ity of those who possess language to communicate about things not physically present, and the ability of those who possess it to produce an infinite number of new meanings via the combination of existing elements. Almost all human babies progress through the same developmental language stages, in the same order, and on roughly the same time schedule irrespective of which native language they are learning. Children also seem to acquire the rules of language faster than they could possibly acquire them through formal learning. Finally, there are specific brain areas devoted to language. Most indi- viduals are left hemisphere dominant with respect to language and have localized sites within this hemisphere that are devoted to language comprehension and language pro- duction. These observations suggest that language capacity may be prespecified and is present at birth. This, in turn, would mean that language capacity is genetically coded and has been shaped by selection forces that were operational at some time in our ancestral past. Robin Dunbar (1996) has developed a fascinating theory of language evolution. He notes that in hominids, there is a positive correlation between group size and the size of the neocortex. The neocortex underlies cognitive function and is quite large in humans. This brain region processes social information—for example, information that has to do 252 Cognitive SCienCe with which members of a group are our friends and which members are our enemies. These kinds of social computations become more complex with increases in group size. Most primates form alliances by grooming one another—that is, by picking par- asites and dirt from one another’s fur. This feels good to them and is a form of social bonding. But grooming, because it is a one-on-one activity, imposes a limit on how many individuals in a group can bond. Once a group becomes large enough, not every member of the group can be groomed. Language is also a form of social bonding and a way for individuals who possess this capacity to form alliances within groups. These alliances are forged primarily through the equivalent of gossip, wherein small groups communicate in both positive and negative ways about other group members. Because one can speak comfortably to a larger number of other individuals at once, language may have driven the formation of larger groups in early protohuman societies. The coming into being of larger groups prompted further innovations, which were adaptive in nature. Larger groups are better able to defend themselves against preda- tors in an open savanna environment. Language allows for complex, coordinated social behavior. Language would have facilitated hunting, foraging, child care, and other cru- cial survival behaviors. The development of language may have received an additional boost from sexual selection. Assuming that females recognize verbal skill as a rough indicator of intelligence, they might choose to mate with males who are good “talkers,” as their intelligence would make them better partners. Behavioral Economics: How We Think About Profit and Loss The discipline of behavioral economics is devoted to studying the basis of economic decision making. It applies scientific methods to understand the cognitive, emotional, and social influences on the way we think about things such as money, goods, services, buying, and selling. The classical view in economic theory is that people are rational decision makers. This implies that we reason logically when presented with choices, always picking outcomes that maximize our benefits and minimize our losses. In reality, it turns out that we are far from rational when it comes to making economic choices, and our motives in these situations often have evolutionary explanations. As was the case with judgment under uncertainty, our behavior stems from various heuristics. Framing, or the way a problem is presented, also has a powerful impact under these circumstances. Imagine that you are given $1,000 and that you can split it with somebody any way you want. This scenario in the lab is known as the ultimatum game. Being slightly selfish, you might decide to keep $900 and give $100 to the other person. Would your partner accept? If she were perfectly rational, she would. After all, it is a free hundred bucks. Surprisingly, though, in experiments such as this, people will turn down the offer and reject the money. In fact, they will reject it when the uneven split is 70/30 or more (Camerer, 2003). Why should this be? According to evolutionary theory, it is because people perceive the split to be unfair. Reciprocal altruism implies that if somebody does you a favor, then he or she is trustworthy. In the future, you could then return the favor and between the two of you establish a support network of mutual aid. This is one mech- anism by which hunter–gatherer societies could establish strong bonds and increase their chances of survival. Chapter eight the evolutionary approaCh 253 We can effectively sum up this principle as “I’ll scratch your back if you scratch mine” (Shermer, 2008). People are willing to accept help only if they perceive the split to be something approaching parity because this would signal an ally who could help them down the road. Interestingly, the effect isn’t found only in humans. De Waal (1997b) had pairs of capuchin monkeys perform a task. When one member of the pair was rewarded and did not share with its partner, the other expressed displeasure and failed to cooperate from that point on. This and other evidence suggest that our primate ancestors and we have evolved a sense of justice. The principle of loss aversion states that we are more sensitive to losses than to equivalent gains. In fact, empirical estimates show that people tend to fear losses about twice as much as they desire gains. Here’s an example: Would you prefer to receive a $5 discount or to avoid a $5 surcharge? Most people prefer the latter. Here is another example: You are on a team of experts that must decide what course of action to take in preventing a flu outbreak. Program A: Two hundred people will be saved. Program B: There is a one-third probability that 600 people will be saved and a two-thirds probability that no one will be saved. Of the participants, 72% chose Program A. Now consider the following rewording of the question: Program C: Four hundred people will die. Program D: There is a one-third probability that nobody will die and a two-thirds probability that 600 people will die. The outcomes in this second case are identical to that in the first, yet now the responses are reversed. Of the participants, 78% now chose Program D over Program C. These results demonstrate not just loss aversion but also framing effects. We prefer choices that are framed positively rather than negatively (De Martino, Kumaran, Sey- mour, & Dolan, 2006; Tversky & Kahneman, 1981). Framing effects are salient when we make financial decisions. Here is an example: Query A: The MePhone is available at Awesome Electronics for $100; five blocks away at DigiData, it is at half price for only $50. Would you make the short trip to save the $50? Query B: PowerPurchase offers the NetBook for $1,000; five blocks away, it can be had for $950 at CircuitCrazy. Would you make the short trip to save the $50? The majority of people, when presented with Query A, answer “yes.” They would be willing to walk the five blocks in that scenario. However, with Query B, even though the amount of money saved by the five-block walk is the same, many people respond “no.” 254 Cognitive SCienCe Why? According to the principle of mental accounting, we put money into different categories based on the context or frame. We tend to put smaller amounts of money into one category and larger amounts into another. A $50 savings seems greater compared with $100 than it does relative to $1,000. Generally, people are less likely to make efforts to save cash when the relative amount of money they are dealing with is large. Samuelson and Zeckhauser (1988) offered participants a choice between several financial investments varying in risk. Under those circumstances, individuals chose a variety of different investments based on how risk averse they were. Then, in a second condition, they told the participants that an investment had already been chosen for them, and they could either stick with this or switch to one of the alternatives. Now, they were far more likely to stick with the one that they had been assigned. Of these partici- pants, 47% stayed with the default compared with the 32% of those who had chosen it in the earlier scenario. The tendency to stay with what we have (the status quo) and to prefer it over other options has been dubbed the endowment effect. The evolutionary explanation for the endowment effect is straightforward. Back in the EEA, resources were usually scarce. Things such as food and tools took a long time and lots of effort to obtain. For this reason, it made sense to hoard or hold on to those things we already had, since replacing them would have been time-consuming and energy intensive. Those individuals who kept a tight grip on what they had would have been more likely to survive. However, the endowment effect can also backfire. Some- times, it does make sense to let go of what we have and switch to something different that may end up being more valuable or at least less costly. Unfortunately, we tend to overes- timate or focus on the amount of effort we’ve already put into something (its sunk cost) and use this to justify staying with it, which may blind us to choosing a better option. If this happens, then we have fallen victim to the sunk-cost fallacy (Sutton, 1991). Think for a minute about those things you may have held on to for too long. They may include staying with the same car, house, job, relationship, and so on. Sex Differences in Cognition Married couples may sometimes be aware of the existence of cognitive differences between the sexes. Perhaps one partner gets lost, while the other is forgetful. Research has shown that in the human species, there are some small but consistent cognitive differences between males and females. The evolutionary psychology approach tries to provide explanations for these differences in terms of the selection pressures that were acting on the two sexes during the EEA. According to evolutionary psychology, important cognitive sex differences can be attributed to an early human sexual division of labor, according to which men hunted and women gathered (Silverman & Eals, 1992; Silverman & Phillips, 1998). The hunt- ing process, which was apt to require navigation across long distances and novel terrain for days at a time, is believed to have selected for enhanced spatial abilities in men. Women, for whom communication with one another and with their children was a part of the gathering process, are believed to have developed superior verbal abilities. Indeed, such differences between the sexes are observable today. On average, males Chapter eight the evolutionary approaCh 255 outperform females on tests of spatial relations and image rotation (Levy & Heller, 1992). In these tests, a participant is usually asked to determine whether a letter of the alphabet, displayed in a variety of orientations, is the same as another. Females generally score higher than males on tests of verbal fluency, reading speed, reading comprehen- sion, and spelling (Notman & Nadelson, 1991). One might also deduce that as women were specialized at gathering, they should be adept at specific kinds of spatial tasks— namely, object–location memory. Memory for object location is imperative to the task of gathering, as one would need to recall the locations of perhaps widely disseminated food sources. Silverman and Phillips (1998) discovered that women outperform men in their ability to remember the locations of objects, both natural and artifactual (see Figure 8.10). Their study suggests that our conceptions of spatial and verbal abilities are crude and that perhaps multiple domains within these abilities perhaps may point the way to additional sex differences. A second theory that accounts for the differences between men and women with respect to spatial and verbal abilities has been advanced. According to this hypothesis, spatial ability became enhanced in males not as a result of hunting activity but, rather, as a result of long-standing mate-seeking practices. The males of polygynous animal species must travel comparatively greater distances to meet and mate with females. Data indicate that the males of polygynous species—for example, the meadow vole—make fewer errors than females when given maze navigation tasks (Gaulin & FitzGerald, 1986, 1989). These sex differences disappear in the prairie vole, which is a monogamous spe- cies and of which neither sex ranges very far. It should be noted that there is nothing special about “maleness” or spatial ability. Rather, it is the environmental demands that are placed on one sex or the other of a particular species that are pivotal. In the brown- headed cowbird, there is a greater need for spatial problem solving among females, who show evidence of spatial ability that is superior to that of the males (Sherry, Forbes, Khurgel, & Ivy, 1993). That said, it is important to be careful with claims about the evolutionary origins of human cognitive abilities, in general, given the fact that there is usually very little concrete evidence to back them up. The evolutionary scholar Stephen Jay Gould (1978) called these claims “just-so stories” because they sound intuitively compelling but have little or no evidence, and an alternative invented story can often sound just as compel- ling. For example, Western thought has tended to assume that the reason men domi- nate in mathematical and scientific careers is because boys innately place more focus on objects and their mechanical and mathematical relationships, whereas girls innately focus on emotions and personal relationships (Baron-Cohen, 2003; Pinker 2003). On the face of it, this sounds like it might be a reasonable explanation. But the evidence actually does not support this just-so story. Developmental psychologist Elizabeth Spelke (2005) points out that if these sex differences are due to genetic biases, then we should be able to find evidence for them in infants. Although some studies show that older children and adults exhibit a sex difference in speeded quantitative reasoning tests, studies with infants do not show any advantage for males in processing objects, space, or number. Thus, many of the sex differences that show up in older children and adults may be due to cultural biases in how these skills are taught, rather than genetic biases. 256 Cognitive SCienCe Figure 8.10 A stimulus set that can be used to test for object and location memory. If you studied this for a minute, would you be able to recall what objects were next to the pelican? Chapter eight the evolutionary approaCh 257 EVALUATING EVOLUTIONARY PSYCHOLOGY EP as a formal discipline is a recent addition to the ranks. As such, it has received both fervent acclaim and fervent criticism. Its strengths are its methodological variety and its attempt to develop an objective science of human behavior that is grounded on a single, powerful theoretical model. The evolutionary model already has proved to be fruitful in biology, and its use in psychology links the two disciplines. The evolutionary perspective also offers to reshape psychology such that it becomes a unified discipline, as opposed to the disjointed one that it tends to be currently (Buss, 1999). Modern psychology con- sists of multiple disciplines, each characterized by a unique theoretical perspective and a unique focus. For example, cognitive psychology focuses on information processing, social psychology focuses on interpersonal relationships, and developmental psychology focuses on growth and change in an organism over its entire life span. EP cuts across these different theoretical perspectives and tries to provide a coherent, unified approach to the study of mind and behavior. But EP has its problems. Even adherents of the theory of natural selection and of biological evolution have attacked its assumptions. One of the fundamental assumptions behind the evolutionary approach is that evolved psychological mechanisms are adaptive responses to survival-related or repro- duction-related problems. But this need not always be the case. At least two other kinds of phenomena, in addition to selective processes, can produce novel biological func- tion (Dover, 2000). The first of these is exaptation, or neutral drift. In this instance, random mutations in an organism’s genome result in new genes that have little or no consequence for reproduction. These genes can become disseminated in a population and at some point may assume a new adaptive function that represents a response to a change in the environment. The second is molecular drive, in which a copy of a gene can mutate. There are known cases in which genes that code for proteins become dupli- cated; the duplicated genes then diverge to serve another function. These genes and the characteristics they give rise to have not been selected for. Stephen Jay Gould (2000) proposed that many of an organism’s attributes may arise via nonselectionist mechanisms. He refers to these attributes as “spandrels.” A span- drel is the triangular space between the exterior curve of an arch and the rectangular frame that encloses the arch (see Figure 8.11). A spandrel serves no design purpose; it exists solely as a consequence of an architect’s surrounding an arch with a frame. Simi- larly, many psychological mechanisms may themselves be spandrels—they may be by- products of an adaptation that was subsequently co-opted for useful purposes. The ability to read and write are probably by-products of an earlier linguistic capacity—the capacity to comprehend and produce speech. Once these earlier capacities are in place, neural connections to visual and manual parts of the brain that were already in existence would then have enabled the later arriving capacities. Cognitive scientist Michael L. Anderson (2014) calls this “neural re-use,” where perceptual and cognitive mechanisms that were naturally selected for in our ancestors now get used for new and different learned functions—often for functions that have not been around long enough for nat- ural selection to have evolved genes for them in our DNA. The job of the evolutionary psychologist is a difficult one, as he or she must start with a given mental mechanism and come up with a purpose for it. The purpose 258 Cognitive SCienCe Figure 8.11 Spandrels in a cathedral in Granada, Spain. Spandrels corresponds to the adaptive problem the mechanism was intended to solve. This process is called reverse engineering because one begins with the final product and thinks back to what it was that may have compelled its genesis. This is more difficult than the con- ventional engineer’s job, which consists