Econ 440: Lecture 3 PDF
Document Details
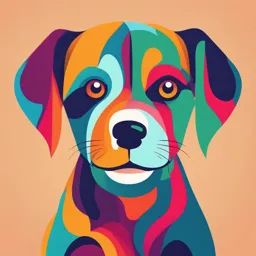
Uploaded by EnrapturedDragon
Texas A&M University
2024
Ragan Petrie
Tags
Summary
Lecture notes from a 2024 economics class at Texas A&M University. The lecture explores various biases and heuristics in decision-making, including confirmation bias, representativeness heuristic, and availability heuristic.
Full Transcript
Econ 440: Lecture 3 Prof. Ragan Petrie Texas A&M University August 27, 2024 Biases and Heuristics Biases and Heuristics Biases and Heuristics Confirmation bias ▶ Confirmation bias is the tendency to ignore information that does not confirm your beliefs about...
Econ 440: Lecture 3 Prof. Ragan Petrie Texas A&M University August 27, 2024 Biases and Heuristics Biases and Heuristics Biases and Heuristics Confirmation bias ▶ Confirmation bias is the tendency to ignore information that does not confirm your beliefs about the world ▶ How does this relate to Bayes’ Rule? ▶ Let B = climate change is caused by humans, A = some evidence for this ▶ Recall Bayes: P(A|B)P(B) P(B|A) = P(A) ▶ P(B) is called your prior belief, while P(B|A) is called your posterior belief (because it comes after the evidence has been seen) ▶ Consider climate denier (P(B) close to 0) and climate scientist (P(B) close to 1) ▶ Even if they agree on P(A|B), after seeing the same evidence they will still disagree on P(B|A) ▶ They have strong priors, i.e. priors close to 0 or 1 ▶ Confirmation bias is like a prior that is exactly 0 or 1 Kahneman and Tversky (1974) Kahneman and Tversky (1974) Kahneman and Tversky (1974) Motivation ▶ Probabilities underlie nearly all of our daily experiences and decisions ▶ What is the probability that inflation will stay below 3% over the next six months? ▶ What is the probability you will get a job that pays > $93,000/year when you are 30 years old? ▶ Even simpler, more objective probabilities are difficult for people to calculate (such as the cancer test example) ▶ Two motivating questions: ▶ How do people come up with probability assessments? ▶ Do these assessments violate our axioms and main theorems from probability theory? Kahneman and Tversky (1974) Leading up to Kahneman and Tversky ▶ Up until this point (and largely still), most research in economics assumed that individuals could calculate probabilities easily and accurately ▶ State of the art in economics and psychology on probability assessments at the time: ▶ Bayes’ rule ▶ von Neuman and Morgenstern’s (1944) expected utility theory ▶ Assumes decision-makers know underlying probabilities ▶ Also assume probability assessments follow independence axiom ▶ Savage’s (1954) subjective expected utility ▶ Decision-maker needs some probability assessment for EUT to make sense ▶ But these assessments can be subjective, i.e. reasonable people can disagree about the likelihood of certain events Kahneman and Tversky (1974) Methods ▶ KT report the results of a long list of experiments ▶ This is more typical of psychology papers ▶ Economists tend to run one experiment with many treatments ▶ Typical responders ▶ College students ▶ Often in psychology classes ▶ Typical sample size: less than 100 students ▶ Experiments usually delivered in terms of one or more vignettes ▶ Often followed by non-incentivized reporting of subjective probability assessments Kahneman and Tversky (1974) Theories ▶ Recall our first question: how do people make probability assessments? ▶ Heuristic is a decision rule: often well-adapted, sometimes maladapted ▶ KT suggest people make probability assessments using three heuristics, not by classical probability theory 1. Representativeness 2. Availability 3. Anchoring and adjustment ▶ We focus on the first two Representativeness Representativeness Representativeness Representativeness ▶ The representativeness heuristic: in assessing the probability that A is of class B, the decision-maker’s answer depends on how representative A is of B ▶ For example, the probability that Dick is an engineer is assessed at least partly by how representative Dick’s personality is of a stereotypical engineer ▶ This heuristic leads to biases (i.e. systematic mistakes) Representativeness Lawyer or Engineer? ▶ In one experiment by KT, subjects were given a vignette about one person randomly selected from 100 ▶ Asked for probability that person is lawyer or engineer ▶ Two treatments: told either 70 or 30 of 100 were engineers ▶ Ratio of assessments of two treatments should be 0.7 0.3 = 2.33 from Bayes’ Rule proof ▶ Instead ratio was close to 1 ▶ Subjects are ignoring the base rate – this is called base rate neglect ▶ Instead they answer depending on how representative vignette is of lawyer or engineer ▶ But when no vignette is given, report 0.7 or 0.3 correctly Representativeness Linda ▶ Another example: Linda the bank teller: Linda is thirty-one years old, single, outspoken, and very bright. She majored in philosophy. As a student, she was deeply concerned with issues of discrimination and social justice, and also participated in antinuclear demonstrations. ▶ Which alternative is more probable? (a) Linda is a bank teller. (b) Linda is a bank teller and is active in the feminist movement. ▶ Probability theory says that (a) must be at least as likely as (b) ▶ However, KT report an experiment where 85% of undergraduates say the opposite ▶ Again, they are answering based on how representative the description is of someone who might be active in the feminist movement Availability Bias Availability Bias Availability Bias Availability ▶ The availability heuristic: Frequency or probability of event is assessed by how readily examples of this event come to mind. ▶ Example: assess national divorce rate by thinking of people you know who have been divorced ▶ Note that this is a very good heuristic in most cases, since things that occur frequently are often easier to remember ▶ However, this also generates biases in some cases Availability Bias Word Search ▶ Suppose I randomly select a word (of three or more letters) from the dictionary. Which is more likely? (a) The word begins with the letter r. (b) The word has the letter r as its third letter. ▶ Turns out that the answer is (b) ▶ KT report that in an experiment, most people say (a) ▶ This is because examples of (a) are more available: it is easier to think of words that start with a certain letter than those that have a certain letter as their third letter ▶ We say this is a bias due to effectiveness of the search set Availability Bias Concepts Representativeness Availability Short def’n “sounds like” “similar to” “examples of” Probabilty conditional unconditional Examples lawyer vs engineer, Linda word search Availability Bias Different Belief Biases ▶ Some belief biases are about things that are external to the decision-maker ▶ We already saw many examples of judgement biases in Kahneman and Tverksy (1974) ▶ Other beliefs biases are about things that are internal to the decision-maker ▶ Projection bias: biased belief about your utility ▶ Overconfidence: biased belief in your ability Projection Bias Projection Bias Projection Bias Motivation: Breakups ▶ Asked of people in romantic relationships: Imagine that you and the person you’re involved with break up within the next week. Using a scale from 1 to 7, where 1 is not happy and 7 is very happy, how do you think you would feel on a typical day two months from now? ▶ Asked of people with recent breakups: Using a scale from 1 to 7, where 1 is not happy and 7 is very happy, how happy would you say you are these days, on a typical day? ▶ Average responses: ▶ Anticipating breakup: 3.9 ▶ Recent breakup: 5.4 ▶ Any potential problems with this design? ▶ Unincentivized responses; experimenter demand effect; self-image; framing differences; renormalization of happiness scale Source: Gilbert, Pinel, Wilson, Blumberg, and Wheatley (1998), ”Immune Neglect: A Source of Durabiltiy Bias in Affective Forecasting,” JPSP Projection Bias Interpretation ▶ What is going on in the previous example? ▶ People may be underestimating how adaptable their preferences are ▶ What other situations might result in the same failure to predict resilience/adaptability? ▶ Moving to a new state ▶ Losing your job ▶ Getting bad grade or performance review ▶ Severe medical issue ▶ Winning the lottery ▶ This failure to predict one’s adaptability is a specific example of a more general bias: ▶ Projection bias: the tendency to overestimate the degree to which future tastes will resemble current tastes Projection Bias Shopping Lists ▶ Another manifestation of projection bias: current surroundings or state of mind have an undue impact on your planned consumption in the future ▶ Field experiment at grocery store ▶ 135 people entering grocery store without a shopping list ▶ Asked to fill out questionnaire with intended purchases ▶ Some subjects chosen at random for “taste test” of a muffin (real purpose was to make some people less hungry) ▶ After shopping, copies of receipts were collected ▶ Results: What percentage of items in shopping cart were unplanned purchases? ▶ Hungry shoppers: 51% ▶ Sated shoppers: 34% ▶ Interpretation: hungry people buy more because they think they will be more hungry in the future Source: Gilbert, Gill, and Wilson (2002), ”The Future is Now: Temporal Correction in Affective Forecasting” OBHDP Projection Bias Planning Ahead ▶ In the previous experiment, it is possible that hungry people are buying more because they are going to consume it right away ▶ We can get around this with a design that separates the purchasing and the consumption ▶ Experiment with 200 office workers (2 x 2 design): ▶ Workers asked to pick a snack to be delivered one week later ▶ Snacks could be either healthy or unhealthy (not described as such to participants, of course) ▶ Choices made right before or right after lunch ▶ Snacks delivered midafternoon or right after lunch ▶ Results: percent choosing unhealthy option: Receive midafternoon Receive right after lunch Choose before lunch 78% 56% Choose after lunch 42% 26% Source: Read and Van Leewen, ”Predicting Hunger”, OBHDP (1998) Projection Bias Winter Clothes ▶ Projection bias seems to affect small purchases with tempting items like food, but will it affect purchases of more expensive, practical goods? ▶ Data from 2.2 million catalog purchases of cold-weather gear ▶ Note this is not an experiment ▶ Also in data set: temperature deviation on day item was ordered, relative to historical average temperature for that day ▶ Standard theory: current temperature deviations should not affect purchasing behavior, since gear would not arrive for several days ▶ Results: orders for winter gear went up on colder-than-normal days ▶ Any alternate explanations? ▶ Possible that colder weather increases salience, ie helps you remember to buy that coat you need ▶ Counter-argument: items bought on colder-than-normal days are more likely to be returned Source: Conlin, O’Donoghue, and Vogelsang,”Projection Bias in Catalog Orders,” AER (2007) Projection Bias More evidence ▶ What are the two biggest purchases that most of us will ever make? ▶ Car and house purchase ▶ Data: 40 million vehicle purchases across the US (again, not an experiment) ▶ Connected purchase behavior with abnormal weather in the area ▶ Weather acts like a coin flip in a lab experiment ▶ Keep in mind: the enjoyment of the car that day is miniscule compared to total lifetime of car ▶ Results: ▶ Temperature 20 degrees above average increases fraction of cars sold that are convertibles by 8.5% ▶ 10-inch snowstorm increases fraction of cars sold that have 4-wheel-drive by 6% Source: Busse, Pope, Pope, and Silva-Risso, ”Projection Bias in the Car and Housing Market” (2012) Projection Bias More evidence ▶ Data: 4 million homes sold at least twice between 1998 and 2008 ▶ Connected purchase behavior with weather in the area on that day ▶ Note: takes 30-60 days from purchase dates to actually getting keys ▶ Results: ▶ House with swimming pool sells for 0.4% more in summer than in winter ▶ That is a value difference of $1600 for the average house Source: Busse, Pope, Pope, and Silva-Risso (2012) Projection Bias Utility Function ▶ Will formalize the idea of projection bias in a moment ▶ But first, we need to establish the idea of a utility function ▶ Suppose there are several options to choose from, say x, y , z, etc ▶ Then a utility function is a function which takes in an option and returns a number such that u(x) > u(y ) if and only if I prefer x to y ▶ Standard economic theory says that I will pick the option with the highest utility number ▶ That is, I will maximize utility Projection Bias Maximizing Utility ▶ Suppose I have utility function u(x) ▶ What is amount of x that maximizes utility? ▶ Need to find first order condition of u(x) with respect to x: u ′ (x) = 0 ▶ Finally, solve this equation for x Projection Bias Theory Behind Projection Bias ▶ Individual has consumption c in state s ▶ Utility is u(c|s), i.e. utility of consumption (pool or no pool) depends on state (good or bad weather) ▶ Consumer tries to make prediction in state s ′ about utility in future state s: ūs ′ (c|s) ▶ Rational model: ūs ′ (c|s) = u(c|s) ▶ Projection bias model: ūs ′ (c|s) = (1 − α)u(c|s) + αu(c|s ′ ) ▶ Variable α determines your deviation from standard model ▶ Note that projection bias embeds standard model when α = 0 Overconfidence Overconfidence Overconfidence Motivation: Perceived Driving Ability ▶ College students asked to rate both their driving safety and driving skill relative to other people in an experiment ▶ Even if people’s estimate are noisy, the average self-ranking should be 50% ▶ Results: Self rating: Below 50% 50% to 80% 80 to 90% above 90% Safety 12.5% 27.5% 37.5% 22.5% Skill 7.2% 46.4% 26.8% 19.5% ▶ What could cause these patterns? ▶ Overconfidence ▶ Don’t want to admit weakness ▶ Different conceptions of what skillful or safe driving means Source: Svenson, ”Are we all less risky and more skillful than our fellow drivers?” AP (1981) Overconfidence What Might Cause Overconfidence? ▶ Consider the process of learning about one’s ability from observing your own successes and failures ▶ Decision makers may ascribe too much credit to their success and explain failures as bad luck ▶ This is a kind of attribution bias: failure to correctly attribute causes to their effects ▶ This is also a self-serving bias: a bias that makes the decision-maker feel better about themselves ▶ In turn assumes that ego enters the utility function ▶ Also call this ego defense Overconfidence Details on Lawyer-Engineer Vignette Calculations ▶ Let E stand for event that the person is an engineer ▶ Suppose we are told there are 70 engineers, then Bayes’ Rule says P(V |E )P(E ) P(V |E )0.7 P70 (E |V ) = = P(V ) P(V ) ▶ If instead we are told there are 30 engineers, then Bayes’ Rule says P(V |E )0.3 P30 (E |V ) = P(V ) ▶ Note than in both formulas P(V ) (the probability of getting this vignette when drawing randomly) is the same ▶ Key assumption: P(V |E ) = P(V | ∼ E ) (experimental design) ▶ P(V |E ) (the probability of getting this vignette from an engineer) ▶ Thus we can take the ratio of the two conditionals: P70 (E |V ) 0.7 = = 2.333 P30 (E |V ) 0.3 back