The Effects of Social Networks on Employment and Inequality (2004) PDF
Document Details
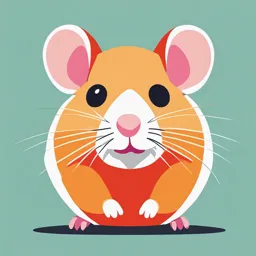
Uploaded by WealthyRiver3058
2004
Antoni Calvó-Armengol and Matthew O. Jackson
Tags
Summary
This paper models how social networks affect employment and inequality. It shows that employment is positively correlated over time and across agents in a network, and unemployment demonstrates duration dependence. The paper further examines how initial employment status differences between groups, coupled with labor market costs, can lead to persistent inequality in employment rates.
Full Transcript
The Effects of Social Networks on Employment and Inequality Author(s): Antoni Calvó-Armengol and Matthew O. Jackson Source: The American Economic Review, Vol. 94, No. 3, (Jun., 2004), pp. 426-454 Published by: American Economic Association Stable URL: http://www.jstor.org/stable/3592937 Accessed: 10...
The Effects of Social Networks on Employment and Inequality Author(s): Antoni Calvó-Armengol and Matthew O. Jackson Source: The American Economic Review, Vol. 94, No. 3, (Jun., 2004), pp. 426-454 Published by: American Economic Association Stable URL: http://www.jstor.org/stable/3592937 Accessed: 10/05/2008 00:17 Your use of the JSTOR archive indicates your acceptance of JSTOR's Terms and Conditions of Use, available at http://www.jstor.org/page/info/about/policies/terms.jsp. JSTOR's Terms and Conditions of Use provides, in part, that unless you have obtained prior permission, you may not download an entire issue of a journal or multiple copies of articles, and you may use content in the JSTOR archive only for your personal, non-commercial use. Please contact the publisher regarding any further use of this work. Publisher contact information may be obtained at http://www.jstor.org/action/showPublisher?publisherCode=aea. Each copy of any part of a JSTOR transmission must contain the same copyright notice that appears on the screen or printed page of such transmission. JSTOR is a not-for-profit organization founded in 1995 to build trusted digital archives for scholarship. We enable the scholarly community to preserve their work and the materials they rely upon, and to build a common research platform that promotes the discovery and use of these resources. For more information about JSTOR, please contact [email protected]. http://www.jstor.org The Effects of Social Networks on Employmentand Inequality AND MATTHEW0. JACKSON* By ANTONI CALVO-ARMENGOL We develop a model where agents obtain information about job opportunities throughan explicitlymodelednetworkof social contacts. Weshow that employment is positively correlated across time and agents. Moreover, unemploymentexhibits duration dependence: the probability of obtaining a job decreases in the length of time that an agent has been unemployed.Finally, we examine inequality between two groups. If staying in the labor marketis costly and one group starts with a worse employmentstatus, then that group's drop-out rate will be higher and their em- ployment prospects will be persistently below that of the other group. (JEL A14, J64, J31, J70) The importance of social networks in labor about jobs arrives randomly to agents. Agents markets is pervasive and well documented. who are unemployed and directly hear of a job Mark Granovetter (1973, 1995) found in a sur- use the informationto obtain a job. Agents who vey of residents of a Massachusetts town that are already employed, depending on whether over 50 percent of jobs were obtained through the job is more attractivethan their currentjob, social contacts. Earlier work by Albert Rees might keep the job or else might pass along (1966) found numbers of over 60 percent in a information to one (or more) of their direct similar study. Exploration in a large number of connectionsin the network.Also, in each period studies documents similar figures for a variety some of the agents who are employed randomly of occupations, skill levels, and socioeconomic lose theirjobs. After documenting some of the backgrounds.1 basic characteristics and dynamics of this In this paper, we take the role of social net- model, we extend it to analyze the decision of works as a manner of obtaining information agents to drop out of the labor force based on about job opportunities as a given and explore the status of their network. This permits us to its implications for the dynamics of employ- compare the dynamics of drop-out rates and ment. In particular, we examine a simple model employment status across groups. of the transmission of job information through a The fact thatparticipationin the laborforce is network of social contacts. Each agent is con- different across groups such as whites and nected to others through a network. Information blacks is well documented.For instance, David Cardand Alan B. Krueger(1992) see a drop-out * Calv6-Armengol:UniversitatAut6nomade Barcelona, rate 2.5 to 3 times higherfor blacks comparedto whites. Amitabh Chandra (2000) provides a Departmentof Economics, Edifici B, 08193 Bellaterra(Bar- celona), Spain (e-mail: [email protected];http://selene. breakdownof differences in participationrates uab.es/acalvo); Jackson: Division of the Humanities and by educationlevel and othercharacteristics,and Social Sciences 228-77, CaliforniaInstituteof Technology, finds ratios of a similar magnitude. Moreover, Pasadena, CA 91125 (e-mail: [email protected]; the analysis of James Heckman et al. (2000) http://www.hss.caltech.edu/-jacksonm/Jackson.html).We thankValentinaBali, Kim Border,Antonio Cabrales,Janet suggests thatdifferencesin drop-outratesare an Currie, Francois Fontaine, Isa Hafalir, Eddie Lazear, importantpartof the inequalityin wages across Massimo Morelli, David Perez-Castrillo,and Giorgio Topa races and that accounting for dropoutsactually for helpful conversations and discussions. We also thank increases the black-white wage gap.2'3 seminarparticipantsand three anonymousreferees for their comments and suggestions. We gratefullyacknowledge the financial supportof Spain's Ministry of Science and Tech- 2 nology under Grant Nos. SEC2001-0973 and BEC2002- Ignoring dropouts biases estimated wages upwards. 02130, and the Lee Center for Advanced Networking at Given much higher drop-outrates for blacks, this can bias Caltech. the wage differential. 1 See James Montgomery (1991) for furtherdiscussion 3 The persistentinequalityin wages between whites and and references. blacks is one of the most extensively studied areas in labor 426 VOL.94 NO. 3 AND JACKSON:EFFECTS OF SOCIALNETWORKS CALVO-ARMENGOL 427 One is then left to explain why drop-outrates race are dominant factors in explaining cor- should differ across races. An analysis of social relation patterns. networks provides a basis for observing both The positive correlationthat we establish be- higher drop-outrates in one race versus another tween the employment of agents in a network and sustainedinequality in wages and employ- then provides a basis for understandingthe sus- ment rates, even among those remainingin the tained difference in drop-outrates and resulting labor force, as follows,4 The starting point of inequality in employment rates. Consider two our model is that the better the employment identical networks, except that one starts with status of a given agent's connections (e.g., rel- each of its agents having a better employment atives, friends, acquaintances),the more likely status than their counterpartsin the other net- it is thatthose connectionswill pass information work. Now consider the decision of an agent to concerning a job opening to the agent. This either remainin the labormarketor to drop out. might be for any numberof reasons. One is that Remaining in the labor market involves some as the employment status of a connection im- costs, which could include things like costs of proves it is less likely that the connection will skills maintenance,education, and opportunity want to keep a new job for him or herself. costs. Agents in the network with worse initial Another is that as the employment status of starting conditions have a lower expected dis- other agents in the networkimproves, the more counted stream of future income from remain- likely that a given agent's connections will be ing in the network than agents in the network employed and a source of information,and the with better initial startingconditions. This mi- more likely thatthey will choose to pass news to nor difference might cause some agents to drop the given agent ratherthan to some other agent out in the worse network but remain in the who alreadyhas a job. This sort of information better network. The important observation is passing leads to positive correlation between that droppingout has a contagion effect. When the employment status of agents who are di- some of an agent's connections drop out, that rectly or indirectly connected in the network, agent's future prospects worsen since the within a period and across time, as we establish agent's networkis no longer as useful a source below. of job information. Thus, some agents con- Such correlation of employment is ob- nected to dropouts also drop out due to this served in the data, both in the indirect form of indirect effect. This can escalate, so a slight the inequality mentioned previously, and also change in initial conditions can lead to a sub- in terms of direct measured correlation pat- stantial difference in drop-out decisions. As a terns. Giorgio Topa (2001) demonstrates geo- largerdrop-outrate in a networkleads to worse graphic correlation in unemployment across employmentstatusfor those agents who remain neighborhoods in Chicago, and also finds a in the network,we find that slight differencesin significantly positive amount of social inter- initial conditionscan lead to large differencesin actions across such neighborhoods. Timothy drop-outrates and sustaineddifferences in em- Conley and Topa (2001) find that correlation ployment rates.5 also exists under metrics of travel time, oc- Before proceeding to the model, let us also cupation, and ethnicity; and that ethnicity and mentiona fourthfeaturethatis also exhibitedby the model. Unemploymentexhibits durationde- pendence and persistence.That is, when condi- economics. James P. Smith and Finis R. Welch (1989), tioning on a history of unemployment, the using statisticsfrom census data,breakthe gap down across expected probability of obtaining a job de- a variety of dimensions and time. The gap is roughly on the creases in the length of time that an agent has order of 25 percent to 40 percent, and can be partly ex- been unemployed.Such durationdependenceis plained by differences in skill levels, quality of education, well documentedin the empirical literature,for and other factors [e.g., see Card and Krueger (1992); Chandra(2000)]. See Reynolds Farley (1990) for a com- example, in studies by StuartO. Schweitzerand parison of labor market outcomes for 50 racial-ethnic groups in the United States. 4 In this paper we focus on differences in drop-outrates 5 and employment, and we refer the reader to Calv6- Similarly, small differences in network architectures Armengol and Jackson (2003) for an analysis of wage may also lead to sharp differences in drop-out patterns inequality. across two groups with identical startingconditions. 428 THEAMERICANECONOMICREVIEW JUNE 2004 Ralph E. Smith (1974), Heckman and George mention the vast literaturein sociology.7 The Borjas (1980), ChristopherFlinn and Heckman contributionhere is that we are the firstto study (1982), and Lisa M. Lynch (1989). To get a an explicit network model and prove some of feeling for the magnitude, Lynch (1989) finds the resulting implications for the patterns and averageprobabilitiesof finding employmenton dynamics of employment, as well as the in- the order of 0.30 after one week of unemploy- equality across races. ment, 0.08 after eight weeks of unemployment, and 0.02 after a year of unemployment. I. A SimpleNetworkModel The reason that we see durationdependence in a networked model of labor markets is a The model we consider here is one where all simple one. A longer history of unemployment jobs are identical. We refer the reader to a is more likely to come when the direct and companionpaper,Calvo-Armengoland Jackson indirect connections of an agent are unem- (2003), for a more general model that nests this ployed. Thus, seeing a long spell of unemploy- model, and also looks at wage dynamics, and ment for some agent leads to a high conditional allows for heterogeneityin jobs, decisions as to expectation that the agent's contacts are unem- whether to switch jobs, repeated and selective ployed. This in turnleads to a lower probability passing of information, competing offers for of obtaininginformationaboutjobs throughthe employment,and other extensions of the model social network.This networkexplanationis or- presented here. In short, the results presented thogonal to standardexplanations such as un- here extend to wage inequality as well, and are observed heterogeneity. quite robust to the formulation. At this point, let us preview a policy predic- There are n agents. Time evolves in discrete tion that emerges from a networkedmodel. Due periods indexed by t. The vector s, describesthe to the networkeffects, improvingthe status of a employment status of the agents at time t. If given agent also improves the outlook for that agent i is employed at the end of period t, then agent's connections.This is the contagioneffect it = 1 and if i is unemployed then sit = 0. discussed above in reverse. As a result, in a A period t begins with some agents being networkedmodel there are local increasing re- employed and others not, as described by the turns to subsidizing education, and other poli- status st- 1 from the last period. Next, informa- cies like affirmativeaction.6One implicationis tion about job openings arrives. In particular, that it can be more efficient to concentratesub- any given agent hears about a job opening with sidies or programs so that a cluster of agents a probabilitya that is between 0 and 1. This job who are interconnectedin a network are tar- arrivalprocess is independentacross agents. If geted, rather than spreading resources more the agent is unemployed, then he or she will broadly. take the job. However, if the agent is already Before presentingthe model, we note that we employed then he or she will pass the informa- are certainly not the first researchersto recog- tion along to a friend, relative, or acquaintance nize the importanceof social networksin labor who is unemployed. This is where the network markets.Just a few of the studies of labor mar- pattern of relationships is important,as it de- kets thathave takennetworktransmissionof job scribes who passes informationto whom, which information seriously are Scott A. Boorman is ultimately crucial in determininga person's (1975), Montgomery (1991, 1992, 1994), Ken- long-term employment prospects. We now de- neth Arrow and Ron Borzekowski (2001), Topa scribe these network relationshipsand the pro- (2001), and Calv6-Armengol (2004)-not to cess of informationexchange. Any two people either know each other or do not, and in this model information only flows between agents who know each other. A graph 6 In our model, improving the status of one agent has g summarizes the links of all agents, where positive external effects on other agents' expected future gij = 1 indicates that i andj know each other, employment. There are, of course, other factors that might counterbalancethis sort of welfare improvement:for in- stance, the difficulty that an agent might have adapting to new circumstancesunderaffirmativeaction as discussed by 7 Some related references can be found in Montgomery George A. Akerlof (1997). (1991) and Granovetter(1995). VOL.94 NO. 3 AND JACKSON:EFFECTS OF SOCIALNETWORKS CALVO-ARMENGOL 429 and gij = 0 indicatesthatthey do not know each C) AikL 0 other. It is assumed that gij = gji, meaning that the acquaintance relationship is a reciprocal 1 3 one. FIGURE 1. NEGATIVE CORRELATION IN CONDITIONAL If an agent hears about a job and is already EMPLOYMENT employed, then this agent randomly picks an unemployed acquaintanceto pass the job infor- mation to. If all of an agent's acquaintanceare already employed, then the job informationis to characterize the behavior of this stochastic simply lost. The probabilityof the joint event process. that agent i learns about a job and this job ends The relationship between the one-period- up in agent j's hands, is described by pij(s), ahead employment status of an agent and his where pattern of connections, as described by the pij(s)'s above, is clear. Having links to em- Pij(s) ployed agents improves i's prospects for hear- ing about a job if i is unemployed. In addition, a if si = 0 and i = j, decreasing the competition for information aa from two-link-away connections is helpful. = IE gik if si = 1, sj = 0, and gi = 1; and That is, if friends of my friends are employed k:sk=0 rather than unemployed, then I have a higher 0 otherwise, chance of being the one that my friends will pass information to. Further indirect relation- and where the vector s describes the employ- ships (more than two-links away) do not enter ment status of all the agents at the beginning of the calculation for the one-period-aheadem- the period. ployment status of an agent. However, once we In what follows, we will also keep track of take a longer time perspective, the evolution of some indirect relationships, as friends of a employment across time depends on the larger friend will play a couple of roles. First, they are network and status of other agents. This, of competitorsfor job informationin the shortrun. course, is because the largernetworkand status Second, they help keep an agent's friends em- of other agents affect the employment status of ployed, which is a benefit in the longer run. We i's connections. say that i and j are path-connected under the We first present an example which makes it networkg if there exists a sequence of links that clear why a full analysis of the dynamics of form a path between i andj. employment is subtle. Finally, the last thing thathappensin a period is that some agents lose theirjobs. This happens Example 1 (Negative ConditionalCorrelation): randomly according to an exogenous breakup ConsiderFigure 1, a networkwith three agents, rate, b, between 0 and 1. This is the probability and suppose the employment from the end of that any given employed agent will lose his or the last period is st- = (0, 1, 0). In the picture, her job at the end of a given period, and this is a darkenednode representsan employed agent also independentacross agents. (agent 2), while unemployed agents (1 and 3) are representedby empty nodes. A line between II. The Dynamicsand Patternsof Employment two nodes indicates that those two agents are linked. As time unfolds, employment evolves as a Conditional on this state st_ , the employ- function of both past employment status and ment states slt and s3t are negatively correlated. the network of connections which, together, This is due to the fact that agents 1 and 3 are randomly determine the new employment. "competitors"for any job news that is first Employment thus follows a finite state heard by agent 2. Markov process, where the state is the vector Despite this negative (conditional) correla- of agents' employment status at the end of a tion in the shorterrun, agent 1 can benefit from period and transition probabilities are depen- 3's presence in the longer run. Indeed, agent 3's dent on the network of relationships. We wish presence helps improve agent 2's employment 430 THEAMERICANECONOMICREVIEW JUNE 2004 g Prob(sl = 0) Corr(s, s2) Corr(si, 3) aggregated over the four agents is 10.75 per- 2 *3 cent. As we see from Figure 2, adding more 1o04 0.132 links furtherdecreases the unemploymentrate, but with a decreasing marginal impact. This 1 *4 0.083 0.041 makes sense, as the value to having an addi- 0.063 0.025 0.019 tional link comes only in providing job infor- mation when all of the existing avenues of 1t4 0.050 0.025 0.025 informationfail to provide any. The probability of this is decreasing in the number of FIGURE 2. CORRELATION AND NETWORK STRUCTURE I connections. The correlationbetween two agents' employ- ment is (weakly9) decreasing in the numberof status.Also, when agent 3 is employed, agent 1 links that each an agent has, and the correlation is more likely to hear about any job that agent 2 between agents' employment is higher for di- hears about. These aspects of the problem rect comparedto indirect connections. The de- counterthe local (conditional)negative correla- crease as a function of the number of links is tion, and help induce a positive correlationbe- due to the decreased importanceof any single tween the employmentstatus of agents 1 and 3. link if an agent has many links. The difference between direct and indirect connections in The benefits from having other agents in the terms of correlationis due to the fact that direct networkoutweigh the local negative correlation connections provide information,while indirect effects, if we take a long-run perspective. The connections only help by indirect provision of following examples illustrate the long-run be- information that keeps friends, friends of havior of the Markov process regulating em- friends, etc., employed. ployment as shaped by the underlyingnetwork Also, note thatthe correlationbetween agents of contacts between agents. 1 and 3 in the thirdrow of Figure 2 is positive (1.9 percent).Thus, even though agents 1 and 3 Example2 (Correlationand NetworkStructure): are in competition for information from both Consider an example with n = 4 agents and let agents 2 and 4 in the shorterrun, their employ- a = 0.100 and b = 0.015. If we think about ment is positively correlated in the long run. these numbers from the perspective of a time This will be truemore generally, as statedin the period being a week, then an agent loses a job propositionsbelow. roughly on averageonce in every 67 weeks, and Next, Figure 3 examines some eight-person hears (directly) about a job on average once in networks,with the same informationarrivaland every ten weeks. Figure2 shows unemployment job breakup rates, a = 0.100 and b = 0.015.'0 probabilities and correlations between agents' Here, again, the probabilityof unemployment employmentstatusesunderthe long-runsteady- falls with the numberof links, and the correla- state distribution.8 tion between two employed agents decreases If there is no networkrelationshipat all, then with the distance of the shortest path of links we see an average unemploymentrate of 13.2 (geodesic) between them. percent. Even moving to just a single link Also, we can see some comparisons to the (g12 = g21 = 1) substantially decreases the four-personnetworks:an agent has a lower un- probability(for the linked agents) of being un- employmentrate in a complete four-personnet- employed, as it drops by more than a third, to work than in an eight-person circle. In this 8.3 percent. The resulting unemployment rate example, the direct connection is worth more than a numberof indirectones. More generally, 8 The numbers for more than one agent are obtained from simulationsin Maple?. We simulatethe economy over 9 In some cases, the correlationsare indistinguishableto a large number of periods (hundreds of thousands) and the accuracy of our simulations. 10We know from calculate observed unemployment averages and correla- Propositions 1 and 2 that Corr(sl, s5) tions. The programs are available upon request from the is positive for the top networkin Figure 3. However, it is too authors. The correlationnumbers are only moderately ac- small to accuratelyreportits numericalvalue based on our curate, even after several hundredthousandperiods. simulations. VOL.94 NO. 3 CALVO-ARMENGOL AND JACKSON:EFFECTS OF SOCIALNETWORKS 431 > ProbQs'i 0) CornKsi, - ) Corr(sj,s) Corr('s,) Oo4r(,5) s, 4-Ebrab(s3 t U0,O)60 0.023 0.003 0.001 0.030 0.014 0.014 0.014 0.014 FIGURE 3. CORRELATION AND NETWORK STRUCTURE II 9 average path length average unemployrIent t+W1.571 's 0.048 [ 1L.786 0.049 FIGURE 5. PATH LENGTH MATTERS FIGURE 4. A NETWORK WITH A BRIDGE and 3, 4, 8, and 9 are the worst off. If we compareagent 3 to agent 1, we note the follow- ing: the average geodesic (minimumpath) dis- the trade-off between direct connections and tance between any two agents who are directly indirectones will depend on the networkarchi- connected to agent 3 is 4/3. In a sense, the tectureand the arrivaland breakuprates. In this agents that agent 3 is connected to are not "well example, agents rarelylose jobs, and hear about diversified." In contrast, the average geodesic them relatively frequently, and so direct con- (minimum path) distance between any two nections are employed with a high probability agents who are directly connected to agent 1 is regardlessof the numberof their neighbors,and 2. Moreover, agents 5 and 6 are only path- so indirect connections are less importantthan connectedthroughagent 1. In fact, 1 and 6 form direct ones. In situations with higher breakup what is referredto as a "bridge"in the social rates and lower arrival rates, indirect connec- networks literature.1 tions can become more important. Example 4 (StructureMatters: Densely Versus The model also provides a tool for analyzing Closely Knit Networks): The model can also asymmetriesin the network. show how other details of the networkstructure matter. Compare the long-run average unem- Example 3 (Bridges and Asymmetries): Con- ployment rates on two eight-person networks sider the networkin Figure 4. Again we calcu- with 12 links each. In both networks,all agents late employment from simulations using the have exactly threelinks. But, the averagelength same arrivaland breakuprates as in the previ- of the paths connecting agents is different ous examples. across networks. Again, we run simulations In this network the steady-state unemploy- with a = 0.100 and b = 0.015; see Figure 5. ment probabilitiesare 4.7 percent for agents 1 The averagepathlength is lower for the circle and 6, 4.8 percentfor agents, 2, 5, 7, and 10, and with diameters than for the circle with local 5.0 percent for the rest. While these are fairly close, simple differences of an agent's position in the networkaffects his or her unemployment 'l The lower unemployment(higheremployment)rateof rate, even though all agents all have the same these agents is then consistent with ideas such as Ronald S. number of connections. Here agents 1 and 6 Burt's (1992) structuralholes, althoughfor differentreasons have lower unemploymentrates thanthe others, than the power reasoning behind Burt's theory. 432 THEAMERICANECONOMICREVIEW JUNE2004 four-agents clusters, meaning that the latter is The propositionshows that despite the short- more closely knit than the former. Indeed, the run conditional negative correlation between average path length decreases when the span of the employment of competitors for jobs and network contacts spreads;that is, when the re- information, in the longer run any intercon- lationships get less introvertedor less closely nected agents' employment is positively corre- knit.12 The average unemployment increases lated. This implies that there is a clustering of with closed-knittedness,reflecting the fact that agents by employment status, and employed the wider the breadthof currentsocial ties, the workers tend to be connected with employed more diversifiedare the sources of information. workers,and vice versa. This is consistent with the sort of clusteringobserved by Topa (2001). The fact that the long-runemploymentstatus The intuition is clear: conditional on knowing of path-connected agents is positively corre- that some set of agents are employed, it is more lated in the above examples is something that likely that their neighborswill end up receiving holds generally. In particular,as we divide a informationaboutjobs, and so on. and b both by some largerand largerfactor-so Moreover, the positive correlationholds not that we are looking at arbitrarilyshort time only underthe steady-statedistribution,but also periods-then we can begin to sort out the across any arbitrarytime periods. That is, agent short- and longer-runeffects. We call this the i's employment status at time t is positively "subdivision"of periods. In the limit we ap- correlated with agent j's status at time t' for proximatea continuoustime (Poisson) process, general values of t and t'. which is the naturalsituation where such tem- porary competition for jobs is short lived and PROPOSITION2: Underfine enough subdivi- inconsequential,while the overall status of in- sions of periods, starting under the steady-state direct connections tells one a great deal about distribution,the employmentstatuses of any two the possible statusof directconnections,and the path-connectedagents are positively correlated longer-runeffects come to dominate. across arbitraryperiods.14 PROPOSITION1: Underfine enough subdivi- III. DurationDependenceand Persistencein sions of periods, the unique steady-state long- Unemployment run distributionon employmentis such that the employment statuses of any path-connected As mentioned in the introduction,there are 13 agents are positively correlated. some other patternsof unemploymentthat have been observed in the data and are exhibited by a networkedmodel. To see this, let us examine some of the serial patternsof employment that 12 Here, close-knittedness is measured by the average emerge. path length, which is just the average across all pairs of agents of the length of the shortestpath between them. An Again, considerjob arrivaland breakuprates alternative measure of the tightness of a network is, for instance, the clustering coefficient, which reflects the level of intraconnectednessamong agents with a common friend. Both measures are roughly equivalent, though in some cases, when network links are randomlyrewired, the aver- for all T > T', the employment statuses of any path- age path length drops sharply, in contrast with a lower connected agents are positively correlatedunder.T. correspondingdecrease of the clusteringcoefficient, a phe- 14 More formally,we show thatthereexists some T' such nomenon often termed a "small world" effect (see, e.g., that Cov(S,, St,,) > 0 for any path-connectedi and j and Duncan J. Watts and Steven H. Strogatz, 1998). periods t and t', under the Markov process 3MT for any 13 More formally, any networkg on the populationof n subdivision of T > T'. "Starting under the steady-state agents, arrivalprobabilitya E (0, 1) and breakdownprob- distribution"means thatthe startingstate is randomlydrawn ability b E (0, 1) define a finite-stateirreducibleand ape- according to the steady-statedistribution,and then all ex- riodic Markov process JM = (g, a, b) on employment pectationsaccountfor the dependenceof the process on this statuses. Denote by ,L the (long-run) steady-statedistribu- initial randomness.This is necessary, as Example 1 shows tion associated to this process, which is uniquely defined. that startingfrom some particularstates, one cannot escape By dividing a and b by some common T, we obtain an short-run negative correlation. Starting from the steady- associated Markov process EMT = (g, alT, blT), that we state distributionis "as if' our expectationsare taken where name the T - period subdivision of.M, with steady-state we let the process run for a long time and average over all distribution/T. We show thatthereexists some T' such that, dates that are t - t' apartfrom each other. VOL.94 NO. 3 AND JACKSON:EFFECTS OF SOCIALNETWORKS CALVO-ARMENGOL 433 g 1 period 2 periods 10 periods limiit ing the probabilitythat an agent will hear indi- rect news about a job. That is, the longer i has 1. *4 0.099 0.099 0.099 0.099 been unemployed, the higher the expectation 3 3 that i's connections and path connections are X1.4 0.176 0A175 0.170 0.099 themselves also unemployed. This makes it io4 0.305 0.300 0.278 more likely that i's connections will take any 0.099 informationthey hear of directly, and less likely FIGURE6. DURATIONDEPENDENCE that they will pass it on to i. In other words, a longer individualunemploymentspell makes it more likely that the state of one's social envi- of a = 0.100 and b = 0.015.15 Ask the follow- ronment is poor, which in turn leads to low ing question: suppose that a person has been forecasts of future employment prospects. unemployedfor at least each of the last X peri- As we mentioned in the introduction, this ods. What is the probabilitythat he or she will explanationfor durationdependenceis comple- be employed at the end of this period? We mentaryto many of the previous explanations. examine the answer to this question in Figure For instance, one (among a number of) expla- 6 as we vary the numberof periods of observed nations that has been offered for durationde- past unemployment and the network. The en- pendence is unobserved heterogeneity.16 A tries representthe probabilitythat agent 1 will simple variant of unobserved heterogeneity is be employed next period, conditional upon the that agents have idiosyncraticfeatures that are networkstructureand having been unemployed relevant to their attractivenessas an employee for at least the given number of consecutive and are unobservableto the econometricianbut previous periods. observedby employers.With such idiosyncratic In the case where there is no network, the features some agents will be quickly reem- probabilityof becoming employed is indepen- ployed while others will have longer spells of dent of the observed history-it is simply the unemployment,and so the durationdependence probabilitythat an agent hears about a job (and is due to the unobservedfeature of the worker. then does not lose it in this period). Once there While the networkmodel also predictsduration is a network in place, the length of the unem- dependence, we find that over the lifetime of a ployment history does give us information:it single worker, the worker may have different provides insight into the likelihood that the likelihoods (which are serially correlated) of agents' connections are unemployed. reemploymentdependingon the currentstate of The patternsobserved here are not particular their surroundingnetwork. So, it also predicts to the example but hold more generally. that controlling for the state of the network should help explain the durationdependence.In PROPOSITION3: Underfine enough subdivi- particular, it offers an explanation for why sions of periods and starting under the steady- workers of a particulartype in a particularlo- state distribution, the conditional probability cation (assuming networks correlatewith loca- that an individual will become employed in a tion) might experience different employment given period is decreasing with the length of characteristicsthanthe same types of workersin their observed (individual)unemploymentspell. another location, all other variables held con- stant.So for example, variablessuch as location Indeed, longer past unemploymenthistories thatcapturenetworkeffects should interactwith lead to worse inferences regardingthe state of one's connections and the overall state of the network.This leads to worse inferences regard- 16 Theoretical models predicting duration dependence, though, are a bit scarcer. In Olivier J. Blanchardand Peter Diamond (1994), long unemploymentspells reduce the re- 15 These calculations are also from simulations, where employmentprobabilitythrougha stigma effect thatinduces here we can directly calculate these conditional probabili- firms to hire applicantswith lower unemploymentdurations ties, by looking at conditional frequencies on observed (see also TaraVishwanath, 1989, for a model with a stigma strings of unemploymentof X periods long. The limit num- effect). In ChristopherA. Pissarides(1992), durationdepen- bers are obtained analytically, and are simply the same as dence arises as a consequence of a decline in worker skills having no network. during the unemploymentspell. 434 THEAMERICANECONOMICREVIEW JUNE 2004 TABLE 1-PROBABILITY OF FINDING EMPLOYMENT FOR AGENTS IN THE BRIDGE NETWORK Number of employed 0 1 2 3 4 5 6 7 8 9 a = 0.100; b = 0.015 10.0 10.4 12.0 14.5 17.9 20.7 25.4 25.7 28.7 34.4 a = 0.050; b = 0.050 5.0 5.9 6.2 6.9 8.6 9.3 11.3 12.2 15.0 18.5 other worker characteristic variables which become employed. Symmetrically, the lower would not be predictedby other models.17 the employment rate, the lower the probability that a given unemployed agent hears about a A. Commentson Stickiness in the Dynamics job.18 Although the process oscillates between of Employment full employmentand unemployment,it exhibits a stickiness and attractionso that the closer it The duration dependence for individuals is gets to one extreme (high employment or high reflective of a more general persistence in em- unemployment)the greaterthe pull is from that ployment dynamics. This persistence can be extreme. This leads to a sort of boom and bust understoodby first noting a simple feature of effect, as illustratedin Figure 7. our model. When aggregateemploymentis rel- Startingfrom full employment,Figure7 plots atively high, unemployedagents have relatively the dynamics of a simulation of aggregate em- more of their connections employed and face ployment over 100 periods for an empty net- relatively less competition for job information, work (the dotted line) and for the bridge and are more likely to hear about jobs. Con- networkof Figure 4 (the plain line). We ran the versely, when aggregate employment is rela- dynamics for two different parameter pairs. tively low, unemployed agents are relatively First, when a = 0.100 and b = 0.015, the less likely to hear aboutjobs. economy oscillates between full and high em- To illustrate this point, consider the bridge ployment in the bridge network while it oscil- network in Figure 4 that connects ten agents. lates more widely between high and low We calculate the (average) individualprobabil- employment in the empty network. An impor- ity that an unemployed agent finds a job within tant feature is that the spells of unemployment the currentperiod, conditionalon the total num- are shorterin the bridge network;this is reflec- ber of employed agents in the network. We tive of the fact that unemployed agents hear provide calculations for two different pairs of about jobs more quickly when the economy is parametervalues, a = 0.100, b = 0.015, and near full employment. The fact that the arrival a = b = 0.050. The probabilitiesare expressed rate is relatively high comparedto the breakup in percentageterms. rate means that the bridge network stays very When there is no networkconnecting agents, close to full employment most of the time. In the probabilitythat an unemployedagent finds a the second simulation, a = 0.050 and b = job is simply the arrivalratea. In contrast,when 0.050, and so the arrivalrate and breakuprates agents are connected through a network (here, are equal, and the economy oscillates more the bridge network of Figure 4), the probability widely between high and low employment in of finding a job varies with the employment both networks. Still the empty network experi- state. This conditional probability is a when ences lower average employment as we should everybody is unemployed, but then increases expect; but more importantly,the bridge net- with the number of employed agents in the work snaps back to full employment more network, as shown in Table 1. This state dependence of the probability of hearing about a job, then implies a persistence 18 We have not explicitly modeled equilibrium wages in aggregate employment dynamics. As a net- and the job arrival process. Incorporatingthese effects work gets closer to full employment, unem- might mitigate some of the effects our model exhibits. However, taking the arrivalprocess as exogenous helps us ployed agents become even more likely to show how the network pushes the process to have certain characteristics. See Randall Wright (1986) for a search model that generatesfluctuatingdynamics in a propermar- 17 We thank Eddie Lazear for pointing this out to us. ket setting. VOL.94 NO. 3 AND JACKSON:EFFECTS OF SOCIALNETWORKS CALV6-ARMENGOL 435 *'1 3 i i I , 20< 40 60 0 1N0 Aggregate employment of agents 1 to 5 versus agents 6 to 10 over time for a=-.050 and b=0.050 FIGURE 8. ASYNCHRONOUS PATTERNS OF EMPLOYMENT ACROSSNETWORKSECTIONS x Aggregate employment over time for a=0.100 and b=0.015 networksuch as the bridge networkfrom Figure 4. As a result temporal patternsmay be asyn- chronous across different parts of the network, with some parts experiencing booms and other parts experiencing recessions at the same time. This asynchronousbehavioris illustratedin Fig- ure 8, whichplots separatelyover 100 periodsthe aggregateemploymentof agents 1 to 5 (thedotted line) and thatof agents6 to 10 (the plain line) in the bridgenetworkfrom Figure4 from a simula- tion with a = 0.050 and b = 0.050. IV. Dropping Out and Inequality in Employment We now turn to showing how the network model has importantimplicationsfor inequality across agents, and how that inequality can Aggregate employment over time for a=0.050 and b-=0.050 persist. Our results so far show that an agent's em- FIGURE 7. TIME SERIES OF EMPLOYMENT FOR NETWORKED VERSUS DISCONNECTED AGENTS ployment status will depend in importantways on the status of those agents who are path- connected with the agent. This leads to some heterogeneity across agents, as their networks quickly when pushed away from it. In line with and the local conditions in their networks will the stickiness of the process, note that the only vary. Note, however, that in the absence of situation where more than two agents are un- some structural heterogeneity across agents, employed for more than five periods appears their long-runprospects will look similar. That between periods 60 and 80, and there we hit the is, if the horizon is long enough, then the im- lowest employment overall-which is illustra- portanceof the startingstate will disappear. tive of the relationshipbetween level of unem- However, expanding the model slightly can ployment and duration. introduce substantial and sustained inequality We also point out that employment need not among agents, even if their network structures be evenly spreadon the network,especially in a are identical.The expansionin the model comes 436 THEAMERICANECONOMICREVIEW JUNE 2004 in the form of endogenizing the network by ments, implying that the drop-out game is su- allowing agents to "drop out" of the network. permodular. That is, as more of the other This decision can be sensitive to startingcon- players decide to stay in, a given player's deci- ditions, and have lasting and far-reachingef- sion to stay in is increasinglyfavoredrelativeto fects on the network dynamics. Let us take a dropping out. The theory of supermodular closer look. games then guaranteesthe existence of a max- Suppose that agents have to decide whether imal Nash equilibrium in pure strategies. A to be in the labormarketnetworkor to dropout. maximal equilibrium is such that the set of We model this as a once-and-for-alldecision, agents staying in the marketis maximal, so that with no reentryallowed for agents choosing to the set of agents staying in at any other equi- drop out. Staying in the labor marketresults in libriumof the game is a subset of those staying an expected presentvalue of costs ci > 0. These in at the maximal equilibrium.We restrict at- include costs of education, skills maintenance, tention to such maximal equilibria.'9'20 opportunitycosts, etc. We normalizethe outside This supermodularaspect of the drop-outde- option to have a value of 0, so that an agent cisions is where we see the emergence of the chooses to stay in the labor force when the contagion effects discussed in the introduction. discounted expected future wages exceed the The fact that an agent drops out leads to worse costs. future employment prospects for that agent's There are two simplificationsmade here. One connections. This in turn increases the chance is that we model this as if all agents make their that those agents drop out, and so forth. Thus, decisions simultaneously. In reality although drop-out decisions are not independently and the bulk of a given agent's connections may be identically distributed (i.i.d.), even when the at similar stages of their lives, the agent may costs of staying in the labor force are i.i.d. also be connected to other agents at different across agents. This effect, as well as how the stages in their lives. The second simplification initial condition of the networkaffects drop-out is that the drop-outdecision is made just once. rates, are illustratedin the following example. Implicit in this is the idea that the bulk of the costs (education and opportunity)appearat an Example 5 (Initial Conditions, Dropouts, and early stage of an agent's career, and once those Contagion): To measure the contagion effect, costs are sunk there is little incentive to drop we first ask how many people would drop out out. These are both clearly crude approxima- without any equilibriumeffect, that is, if they tions, but reasonable startingpoints. each did the calculation supposing that every- There are some obvious comparativestatics. one else was going to stay in. Then we can Drop-out percentages will be decreasing in calculate how many people drop out in equilib- wages and increasing in costs. Drop-out deci- rium, and any extrapeople droppingout are due sions also depend on how well an agent is to somebody else dropping out, which is what connected. With better connections (for in- we attributeto the contagion effect. stance, larger numbersof links holding all else For these calculations, we take the cost of constant), there is a larger chance of hearing staying in the network, ci, to be uniformly dis- about jobs and so the future prospects of em- tributedon [0.8, 1] and fix the per period wage ployment are higher, leading to a higher thresh- to be w = 1. We do the calculations with old of costs where the agent would choose to complete networks, where each participating drop out. agent is directly linked to every other agent. We The partthatis less transparent,but still quite intuitive, is the interaction between the deci- 19 sions of differentagents. Positive correlationof Formally, let di E {O, 1} denote i's decision of whetherto stay in the labor market,where di = 1 standsfor employment for path-connected agents both staying in. A vector of decisions, d**, is a maximal equi- within and across periods implies that having librium if it is an equilibriumand, for every other equilib- more agents participate is better news for a rium d*, we have d** - d*, where - is the component- wise orderingon {O, 1}n. given agent as it effectively improves the 20 There is a coordinationgame going on (players may agent's network connections and prospects for all wish to drop out if all others do, etc.), and here looking future employment. Therefore,the decisions to at the maximal equilibriumeliminates coordinationerrors, stay in the labor force are strategic comple- and allows us to focus on the networkeffects of the model. VOL.94 NO. 3 AND JACKSON:EFFECTS OF SOCIALNETWORKS CALV6-ARMENGOL 437 TABLE 2-DROPOUTS AND CONTAGION-STARTING EMPLOYED So=(1,... 1) n= 1 n= 2 n= 4 n= 8 n = 16 n = 32 n- o Drop-out percentage 58.3 44.5 26.2 14.7 9.7 7.8 6.8 Percentagedue to contagion 0 8.8 5.0 1.4 0.4 0.2 0 TABLE 3-DROPOUTS AND CONTAGION-STARTING UNEMPLOYED So = (0,..., 0) n= 1 n = 2 n= 4 n = 8 n = 16 n = 32 n ->oo Drop-outpercentage 100 97.8 92.9 82.2 68.0 60.6 56.8 Percentagedue to contagion 0 12.1 21.7 18.9 8.7 3.0 0 compute the percentage of dropoutsfor differ- to. Thus about 9.5 of the 11 people would ent values of n, and we also calculate the per- drop out even if all stayed in, and 1.5 of the centage of dropoutsdue to contagion.We do the 11 drop out because of the fact that some calculationfor two initial states:everybody em- others have dropped out. ployed, so = (1,..., 1), and everybody unem- Note that the contagion effect is larger for ployed, so = (0,..., 0). the worse starting state and is also larger for For Tables 2 and 3, the calculationsare done smaller networks (although not entirely for a discount rate of 0.9, where we simplify monotone). This is true because the impact of things by assuming that an agent starts in the someone dropping out is more pronounced in initial state, and thenjumps to the steady state in worse starting states and smaller networks. In the next "period."This just gives us a rough the limit, the impact of having people drop calculation,but enough to see the effects. So, an out is negligible and so the contagion effect agent who stays in gets a net payoff of O.lsi + disappears when agents have very large num- 0.9pi - ci, where Pi is the agent's steady-state bers of connections (holding all else fixed). employmentprobabilityin the maximalequilib- For n = 1, there cannot be a contagion effect, rium. We again set a = 0.100 and b = 0.015.21 so the number is 0 there as well. So, for instance, in Table 3, when n = 16 The nonmonotonicity of the contagion ef- and everybody is initially unemployed, we fect in n is a bit subtle. The possibility of have 68 percent of the people dropping out on contagion is initially nonexistent. It then in- average. This means that we expect about 11 creases as the number of connections in- people to drop out on average and about 5 creases, since there are more possible people to stay in. The 8.7 percent due to combinations of neighbor dropouts that can contagion means that about 1.5 (=0.087 X take place with three connections (when n = 16) of the people dropping out are doing so 4) than one connection (when n = 2), and because others drop out, and they would be any single dropout can then trigger another. willing to stay in if all the others were willing Eventually, with large numbers of connec- tions, the marginal impact of an additional 21 In these calculationswe estimatepi by an approxima- connection to a given agent is already very tion formula, to save on calculations, as we need to iterate low, and in fact becomes second order in the on both the ci's and the numberof agents. The approxima- number of agents. The fact that it shrinks so tion formulaleads to some slight biases. In the simulations, much means that eventually the contagion for any given n, we first randomly draw the ci's. We then calculate the pi's if all agents stay in. From this we can find effect disappears as even having some frac- out which agents would drop out. We can then recalculate tion of one's connections drop out is no the pi's for the new network,and see which agents drop out longer a problem if there are still a large with the new pi's. We continue this process until no addi- number of connections staying in. tional agents drop out. This provides the maximal equilib- rium for this draw of ci's. We then run iterations on this The previous example shows that different algorithmfor new draws of c's and calculate sample aver- social groups with identical network relation- ages over the iterations. ships but differingby their startingemployment 438 THEAMERICANECONOMICREVIEW JUNE 2004 state, have different drop-out rates. Because dropping out hurts the prospects for the group further, this can have strong implications for inequality patterns. We now show that this holds more generally. In analyzing the drop-out game for the proposition below we do not alter the network structurewhen an agent drops out; instead we simply set the dropout's employment status to be 0 forever. Thus a dropout's social contacts do not change and his or her contacts still pass FIGURE9. THE BRIDGE NETWORK WITH ASYMMETRIC on news about jobs. It is as if the agent's STARTING STATES connections still pass the agent information thinking that the agent is simply unemployed ing states emphasizes how important starting rather than a dropout. This might introduce a conditions can be.22 bias to the extent that information is no longer Just to show that the inequalitywe are seeing passed on to someone who has dropped out. is not due to the isolation of the groupsof agents Accounting for such effects would complicate with different startingconditions, let us exam- the analysis as one would need to keep track ine drop-out decisions when there is a bridge of any modifications of the network structure between two groups. as agents drop out. Example 6 (Connected Social Groups and PROPOSITION 4: Consider two social Dropouts): Consider the network structure groups with identical network structures. If from Example 3; see Figure 9. the starting state person-by-person is higher Agents 1 to 5 startemployed and agents 6 to for one group than the other, then the set of 10 startunemployed. agents who drop out of the first group in the We do drop-outcalculationsas in Example5. maximal equilibrium is a subset of their coun- We take the ci to be uniformly distributedon terparts in the second group. These differ- [0.8, 1], fix w = 1, use a discount rate of 0.9, ences in drop-out rates generate persistent and have agents who stay in get a net payoff of inequality in probabilities of employment in O.lsi + 0.9pi - ci, where Pi is the agent's the steady-state distributions, with the first steady-state employment probability in the group having weakly better employmentprob- maximal equilibriumof the drop-outgame, and abilities than their counterparts. There is a si is their startingemployment state. strict difference in employment probabilities The drop-out probabilities for the different for all agents in any component of the net- agents are illustratedin Table 4. work for which the equilibrium drop-out de- Note that the drop-outrates are significantly cisions differ across the two groups. higher for the agents who start in the unem- ployed state, even though the network is con- So we have established that a networked nected. The agent who forms the bridge from model can generate persistent differences the unemployed side is less likely to drop out among two social groups with identical eco- than the other unemployed agents: while the nomic characteristicsexcept that they differ in counterpartagent who forms the bridge from their starting state. As mentioned in the intro- duction, this is consistent with documenteddif- 22 ferences in drop-out rates among blacks and Differences in network structure,rather than differ- ences in startingconditions, can also lead to differentdrop- whites, as well as studies that show that ac- out decisions and sustained inequality. For instance, as we counting for voluntarydropoutsfrom the labor saw in Example 2, underexactly the same a and b and with force negatively affect the standardmeasuresof each agent having two links, the expected long-run unem- black economic progress (e.g., Chandra,2000, ployment of an agent in a network of four agents is 6.3 Heckmanet al., 2000). While this comparisonis percent while it is 6 percent for an agent in a network of eight agents. While the difference in this example is small stylized, the fact that we consider two com- (on the order of a 5-percent change in unemployment),it pletely identical networksexcept for their start- can easily become amplified throughthe contagion effect. VOL.94 NO. 3 CALVO-ARMENGOL AND JACKSON:EFFECTS OF SOCIALNETWORKS 439 TABLE4--DROP-OUTS RATESIN THEBRIDGENETWORKWITHASYMMETRIC STARTING STATES Agent 1 2 3 4 5 6 7 8 9 10 Drop-out rate 0.47 0.42 0.42 0.42 0.42 0.91 0.93 0.93 0.93 0.93 the employed side is more likely to drop out uals embedded in two societal groups with than otheremployed agents.23While this exam- different collective employment histories (or ple is clearly highly stylized, it does provide with different networks as discussed below) some intuitivepredictions,and shows that start- typically will experience different employment ing conditions can lead to sustaineddifferences outcomes. In other words, social networks in- across groups even in a connected network. fluence economic success of individualsat least in part due to the different composition and Let us discuss the relation of our analysis of history of individuals' networks.When coupled drop-out rates to theories of discrimination. with drop-out decisions, sustained inequality Classical theoriesof discrimination,such as that can be the result of differences in history. We of Gary Becker (1957) or Thomas C. Schelling discuss some policy implications of this net- (1971), postulatethatindividualshave an intrin- work viewpoint below. sic preferencefor individualsin their own soci- etal group.24Because of such preferences and V. A Look at Policy Implications externalities, individuals end up segregated in the workplace,and the resultingsortingpatterns Let us mention some lessons that can be by group affiliation can breed wage inequal- learned from our model about policy in the ity.25Our model offers an alternativeand novel presence of networkeffects, and some of which explanation for inequality in wages and em- we will illustrate with some examples below. ployment.26'27 Two otherwise identical individ- One obvious lesson is that the dynamics of the model show that policies that affect current 23 employment will have both delayed and long- There may also be differences between the drop-out lasting effects. rates of agents 2 and 3, or 8 and 9, as things are not Anotherlesson is thatthereis a positive exter- symmetric;but these differences are beyond the accuracyof our simulations. nalitybetweenthe statusof connectedindividuals. 24 There is also an importantliteratureon "statistical" So, for instance, if we consider improvingthe discriminationthat follows Arrow (1972), John J. McCall status of some number of individualswho are (1972), Edmond S. Phelps (1972), and others. Our work is scatteredaroundthe network,or some groupthat quite complementaryto that work as well. are more tightlyclustered,therewill be two sorts 25 We use the word "can"because it may be that some employers discriminatewhile the marketwages do not end of advantagesto concentratingthe improvements up unequal. As Becker (1957) points out, the ultimate in tighterclusters.The first is that this will im- outcome in the market will depend on such factors as the prove the transitionprobabilitiesof those directly numberof nondiscriminatingemployers and elasticities of labor supply and demand. involved,but the second is thatthis will improve 26 While we have not included"firms"in our the transitionprobabilitiesof thoseconnectedwith model, note that to the extent to which the job information comes initially from an employee's own firm, there would also be correlation patterns among which firms connected agents social backgroundis capturedby family income which then work for. That is, if an agent's acquaintanceis more likely determinesinvestmentdecisions in education.In Durlaufs to be getting informationaboutjob openings in the acquain- work, educationis modeledas a local public good, and com- tance's own firm,then that agent has a more than uniformly munity income, ratherthan family incomes, affects human random likelihood of ending up employed in the acquain- capitalformation.In bothcases, becausethe socialbackground tance's firm.This would producesome segregationpatterns imposesconstraintson humancapitalinvestment,income dis- beyond what one would expect in a standardlabor market paritiesare passed on across generations.In our paper, we model. focus insteadon the largersocietalgroupwithinwhich one is 27 Two other importantexplanationsfor inequalitycan be embedded,its networkstructure,collective employmenthis- foundin GlennC. Loury(1981) andStevenDurlauf(1996). As tory, and access to informationaboutjobs. This offers a com- in our model, both papersrelatesocial backgroundto individ- plementary,ratherthan competing,explanationfor sustained ual earningprospects.In Loury's paper, the key aspect of inequality. 440 THEAMERICANECONOMICREVIEW JUNE 2004 these individuals. Moreover, concentratedim- provementslead to a greaterimprovementof the 3 4 status of connections than dispersed improve- ments. This will then propagate through the network. To get a better picture of this, consider the drop-outgame. Suppose that we are in a situa- 5 2 tion where all agents decide to drop out. Con- sider two different subsidies: in the first, we pick agents distributedaround the network to subsidize; while in the second we subsidize a group of agents who are clustered together. In 6 the first case, other agents might now just have 1 one (if any) connection to an agent who is subsidized.This might not be enough to induce them to stay in, and so nobody other than the subsidized agents stay in the market. Here the main impact on the drop-out rate is directly throughthe subsidy. In contrast,in the clustered FIGURE 10. THESTARTING NETWORK STRUCTURE case, a number of agents now have several connections who are in the market. This may induce these other agents to stay in. This can then have a contagion effect, carrying over to we subsidize. In the network, each agent has agents connected with them and so on. Beyond four connections. The network structureis as the direct impact of the subsidy, this has an follows. Each agent has threelinks-two imme- indirect contagion effect that decreases the diate neighbors and one that is slightly further drop-outrate, and then improves the future sta- away. This is picturedin Figure 10. tus of all of the agents involved even further Table 5 provides the percentage of agents throughthe improved network effect. who stay in the networkas a function of who is Exactly how one wants to distributesubsidies subsidized (two agents in each case) and what to maximize their impact is a subtle matter.We the range of costs (randomlydrawn) are.29 look at an example to highlight the subtleties. There are some interestingthings to note. In the highest cost range, even having one A. Concentrationof Subsidies neighbor stay in is not enough to induce an agent to stay, and so the only agents staying in Let us again consider a society of eight indi- are the subsidized ones. Here it is irrelevant viduals, again where a = 0.100 and b = 0.015. which agents are subsidizedas they are the only Suppose the costs of staying in the network,ci, ones staying in. are drawn at random from a uniform distribu- In the lowest two cost ranges, having one tion with support[0.8, 1]. Initially,everybodyis neighbor stay in has a big impact, and so unemployed, so so = (0,..., 0). We work with spreading the subsidies out has the maximal drop-outdecisions when the discountrate is 0.9, impact. Agents 1 and 5 are on opposite ends as in the previous examples. of the circle and have no direct contact in The experiment we perform here is the fol- common. Subsidizing agents 1 and 5 thus lowing. In each case we subsidize two agents to amounts for spreading subsidies out, and it is stay in the market-simply by lowering their indeed the best policy in terms of maximizing cost ci to 0.28 The question is which two agents 28 This might in fact overestimatethe necessary subsidy dization would be a further reason for concentrating costs, as one might not need to lower ci by so much. subsidies. Moreover, we would end up with even lower subsidy costs 29Note that the different cases of who are subsidized by concentratingsubsidies which increases the futurepros- cover all possible configurations,up to a relabeling of the pects of the subsidized agents. Thus, lower costs of subsi- agents. VOL.94 NO. 3 AND JACKSON:EFFECTS OF SOCIALNETWORKS CALV6-ARMENGOL 441 TABLE 5-SUBSIDIZATION STRUCTURE AND PERCENTAGE OF AGENTS WHO STAY IN Cost range Agents subsidized 0.80 to 1 0.82 to 1 0.84 to 1 0.86 to 1 1 and 2 52.9 39.4 27.8 25.0 1 and 3 53.6 39.4 27.1 25.0 1 and 4 57.2 43.4 27.9 25.0 1 and 5 57.9 43.8 27.0 25.0 1 and 6 57.9 44.0 27.0 25.0 1 and 7 57.1 43.4 27.8 25.0 1 and 8 53.5 39.4 27.1 25.0 3 and 4 54.5 39.3 26.1 25.0 3 and 7 57.7 43.6 27.4 25.0 3 and 8 56.2 42.9 29.1 25.0 the number of agents who stay in the market VI. Possible Empirical Tests when the cost is at its lowest level.30 When the cost is in the 0.82 to 1 range, we begin to While as we have discussed, the model gen- see a slight change, where now subsidizing eratespatternsof employmentand dropoutsthat agent 1 and 6 is better, and these agents are are consistent with the stylized facts from a slightly closer together. number of studies, one might want to look at The places where things favor a different some additional and more direct tests of the sort of policy is in the middle range of costs. model's predictions. Here costs are high enough so that it is quite Note thatdrop-outratesand contagioneffects likely that an agent will drop out if she has depend both on the costs ranges and on the only one neighbor who stays in, but is less values for the arrival rate and breakup rate. likely if she has two neighbors who stay in, Some comparativestatics are quite obvious: (1) and so contagion effects are relatively high. as the expected cost increases (relative to Spreading the subsidies out to agents 1 and 5, wages), the drop-out rate increases; (2) as the or 3 and 7, etc., does worse than having them breakup rate increases, the drop-out rate in- close together (1 and 2, 1 and 3, 1 and 4) and creases; and (3) as the arrivalrate increases,the the best possible subsidy here is of the form 3 drop-outratedecreases. However, thereare also and 8. What matters is the number of contacts some more subtle comparisons that can be subsidized agents have in common. Agents 3 made. and 8 are well-placed since both 1 and 2 are For instance, let us examine what happensas connected to both of them. Thus, this concen- job turnoverincreases. Here, as the arrivaland trates subsidies in a way that provides a high breakup rates are both scaled up by the same probability that 1 and 2 will stay in. Without factor, we can see the effects on the drop-out such a concentration of subsidies, we get a rates. Note that such a change leaves the base higher drop-out rate. employmentrate (that of an isolated agent) un- What this suggests is that, in designing sub- changed, and so the differences are attributable sidy or affirmativeaction programs,attentionto entirely to the networkeffects. Table 6 pulls out network effects is important.Concentratingef- various rescalings of the arrival and breakup forts more locally can end up having a higheror rates for the two cost ranges when n = 4 and lower impact, depending on the network agents are related througha complete network. configuration. As before, the first figure is the drop-out rate and the second is the amount attributableto contagion effects. As we can see, higher turnoverrates (higher 30 It is almost a tie with 1 and 6, but slightly aheadin the rescalings of a and b) lead to higher drop-out next decimal. rates. The intuition behind this is as follows. 442 THEAMERICANECONOMICREVIEW JUNE 2004 TABLE6-DEPENDENCE OFDROPOUTS ANDCONTAGION ONARRIVAL ANDBREAKUP RATES Scaled by a and b 1 3 5 7 9 0.05, 0.015 0.15, 0.045 0.25, 0.075 0.35, 0.105 0.45, 0.135 ci ~ [0.8, 1] 69:27 76:27 83:26 88:24 96:20 ci ~ [0.6, 1] 24:3 28:3 34:5 37:5 42:5 With higher turnover rates, when an agent the network as the local status of the connec- becomes unemployed it is more likely that tions changes. some of his neighbors are unemployed. This is actually quite subtle, as the base unem- VII. Concluding Discussion ployment rate has not changed. However, higher turnovermakes it more likely that sev- As we have mentionedseveral times, we treat eral agents lose theirjobs at the same time, and the network structureas given, except that we end up competing for information.This effect consider drop-out decisions. Of course, people then lowers the employmentrate, which in turn have more specific control over whom they feeds back and results in less valuable socialize with both in direct choice of their connections. friendships, as well as through more indirect This effect provides for a testable implica- means such as educationand careerchoices that tion: industries with higher turnoverrates, all affect whom they meet and fraternizewith on a else held equal, should have higher drop-out regularbasis. Examiningthe networkformation rates. Operationalizingthis requires some care, and evolution process in more detail could pro- however, as we do not model the careerchoices vide a fuller pictureof how the labormarketand for workers or an equilibriumin wages. None- the social structureco-evolve by mutually in- theless, it is clear that the predictionis that the fluencing each other: network connections wage to education cost ratio must be relatively shape the labor market outcomes and, in turn, higher in order to induce workers to enter ca- are shaped by them.31 reers where the turnoverrate is high compared In addition to furtherendogenizing the net- to those where it is low, even after correcting work, we can also look deeper behind the in- for any risk aversion or income-smoothing formation exchange procedure.32There are a motives. wide variety of explanations (especially in the Let us brieflymentionsome otherpossibleem- sociology literature,for instancesee Granovetter, piricaltests of the model. To the extentthatdirect 1995) for why networks are importantin job dataon networkrelationshipsis available,one can markets.The explanationsrange from assortive directlytestthe model.In fact, suchinformationin matching (employers can find workers with the form of survey data (the GeneralSocial Sur- vey) has been used extensively in the sociology literatureand also in conjunctionwith wage data 31 There is a growing literature on the formation of networksthat now provides a ready set of tools for analyz- (e.g., Troy Tassier,2001). There are also other tests that are possible. ing this problem.See Jackson(2004) for a survey of models applying to networks of the form analyzed here, as well as For instance, there is data concerning how the Sanjeev Goyal (2004), Frank Page (2004), and Anne van reliance on networks for finding jobs varies den Nouweland (2004) for issues relating to learning, far- across professions, age, and race groups, etc. sightedness, and cooperation structures. 32 Recall that the results stated so far extend to a frame- (see the table in Montgomery, 1991, for in- work more generalthan the simple communicationprotocol stance, to see some differences across profes- where only directcontactscan communicatewith each other sions). Our model then predicts that the and unemployed direct contacts are treated on an equal intensity of clustering, duration dependence, footing. In particular,the generalframeworkaccommodates and drop-outrates should also vary across these a priori ranking among contacts, indirect passing of job socioeconomic groups.Moreover,even within a information,heterogeneousjobs with differentwages, idio- syncraticarrivalandbreakuprates,informationpassing, and specific socioeconomic group, our model pre- job turnoverdependenton the overall wage distribution,etc. dicts differences across separatecomponentsof See Calv6-Armengoland Jackson (2003) for more details. VOL.94 NO. 3 AND JACKSON:EFFECTS OF SOCIALNETWORKS CALV6-ARMENGOL 443 similar characteristics by searching through nities and to the formation of unemployment them), to information asymmetries (in hiring traps.This is worthfurtherinvestigation. models with adverse selection), and simple in- Another important avenue for extension of surance motives (to help cope with the uncer- the model is to endogenize the labor market tainty due to the labormarketturnover).In each equilibrium so that the probability of hearing differentcircumstanceor setting, theremay be a about a job depends on the currentoverall em- differentimpetus behind the network.This may ployment and wages are equilibriumones. This in turn lead to different characteristicsof how would begin to give insights into how network the network is structuredand how it operates. structureinfluences equilibriumstructure. Developing a deeper understandingalong these Finally, we point out that althoughour focus lines might further explain differences in the in this paperis on labor markets,this model can importance of networks across different easily be adapted to other sorts of behaviors occupations. where social networksplay a key role in infor- Anotheraspectof changesin the networkover mation transmission.An example is whetheror time is that networkrelationshipscan change as not individuals take advantageof certain avail- workers are unemployedand lose contact with able welfare programs.Recent studies by Mari- former connections.Long unemploymentspells anne Bertrandet al. (2000) and Anna Aizer and can generatea desocializationprocessleadingto a Janet Currie (2002) point to the importanceof progressiveremovalfrom labor marketopportu- social networks in such contexts. APPENDIX We firstprovide some definitionsthat are necessary for the proofs that follow. Here we specialize the definitionsto randomvectors S = (S,..., Sn) whose components take on values of 0 or 1. We follow the convention of representingrandomvariablesby capital letters and realizationsby small letters. Association While first-orderstochastic dominance is well suited for capturing distributionsover a single agent's status, we need a richer tool for discussing interrelationshipsbetween a numberof agents at once, and this is needed in the proofs that follow. The following definition is first due to James D. Esary et al. (1967). Let ,L be a joint probabilitydistributiondescribing S. Lxis associated if Cov,(f, g) > 0 for all pairs of nondecreasingfunctionsf: {0, 1}n -R> and g: {0, 1} -> R, where Cov(f, g) is the covariance E,[f(S)g(S)] - E,[f(S)]E,[g(S)]. If S,..., S, are the randomvariablesdescribedby a measure/Lthat is associated,then we say that S,..., Snare associated.Note thatassociationof ,u implies thatSi and Sj are nonnegativelycorrelated for any i and1. Essentially, associationis a way of saying that all dimensions of S are nonnegatively interrelated. Strong Association As we often want to establish strictly positive relationships,and not just nonnegative ones, we need to define a strong version of association. Since positive correlationscan only hold between agents who are path-connected,we need to define a version of strong association that respects such a relationship. Consider a partitionI of { 1,... n} that captures which random variables might be positively related;which here will be determinedby the components of the graph g. A probabilitydistribution,L governing S is strongly associated relative to the partitionI if it is associated, and for any rrE I and nondecreasingfunctionsf and g 33 This is still a weaker concept than affiliation, which requiresassociation for all conditionals. It is imperativethat we work with the weaker notion as affiliation will not hold in our setting. 444 THEAMERICANECONOMICREVIEW JUNE2004 Cov,f g) > 0 whenever there exist i andj such thatf is increasingin si for all s i, g is increasingin sj for all s_j, and i andj are in 7r. One implicationof strongassociationis that Si and Sj are positively correlatedfor any i andj in vr. Domination Consider two probabilitydistributions,L and v. pIdominates v if : E,[f] E,[f] The dominationis strict if strict inequalityholds for every nondecreasingfunctionf: {0, 1}" -> DR. for some nondecreasingf. Domination capturesthe idea that "higher"realizationsof the state are more likely under p. than under v. In the case where n = 1, dominationreduces to first-orderstochastic dominance. LEMMA 5: Consider two probability distributionsJLand v on {O, 1 }n. pJdominates v if and only if there exists a Markovtransitionfunction 4 : {0, 1 }n _> ?({O, 1}n) [where P({O, 1In) is set of all the probability distributionson {0, 1}n] such that L(s') =EI Osv(s), s where 4 is a dilation (that is ,s,r> 0 implies that s' - s). Strict dominationholds if )ss, > 0 for some s' 4 s. Thus, p. is derived from