Interpreting Statistics PDF
Document Details
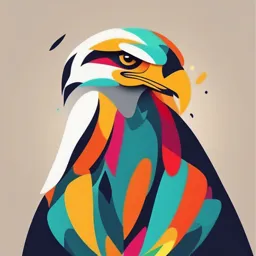
Uploaded by WorldFamousZombie1045
UCL
Tags
Related
- PTWE 5 Storyboard - Mental Health and Resilience Tools Final Copy PDF
- Mental Hlth PH Includes Hlthy Minds - Intro to PH Chpt 19 Fall 2024 PDF
- Suicide Statistics PDF - National Institute of Mental Health
- DSM-5-TR Diagnostic and Statistical Manual of Mental Disorders, 5th Edition, Text Revision PDF
- Neuropsicología de la Atención PDF
- PREP 4b Intro to Applied Stats - Defining the Data PDF
Summary
This document explains core principles of interpreting statistics in mental health research. It covers sampling distributions, standard deviations and errors, confidence intervals, p-values, and the normal distribution. It's geared towards an understanding of the statistical methods used in mental health.
Full Transcript
**[Core Principles in Mental Health Research:]** **[Interpreting Statistics]** [Learning Outcomes:] - Understand and explain sampling variety - List the key properties of sampling distributions - Understand how these properties allow researchers to draw conclusions about populations...
**[Core Principles in Mental Health Research:]** **[Interpreting Statistics]** [Learning Outcomes:] - Understand and explain sampling variety - List the key properties of sampling distributions - Understand how these properties allow researchers to draw conclusions about populations - Explain the similarities, differences, and relationship between standard deviation (SD) and standard error (SE) - Calculate and interpret a 95% confidence interval (CI) - Calculate and interpret a p-value [Sampling Variability & Standard Error] [Populations & Samples:] - Stats describe sample characteristics (e.g., mean) - Statistics estimate parameters - Parameters describe population characteristics **Stats is needed to describe a population** [Descriptive Statistics & Inferential Statistics:] - Descriptive Statistics: - Relates to samples - Used to describe a sample - Purpose: external validity, generalisability of study, - E.g., age & sex distribution of ppts - Inferential Statistics: - Relates to populations - Usually too expensive and time consuming to measure quantity of interest in whole population - Use a sample to make inferences about the population [Sampling Variation:] - The sample mean is unlikely to be exactly equal to the population mean - A different sample would have given a different estimate, the difference being due to sampling variation - Imagine we collected many independent samples of the same size from the same population and calculated the sample mean of each of them - We can draw a frequency distribution of these means and this is called the [sampling distribution of the mean ] [Sampling Distribution & Standard Error:] - It can be shown that: - The means would form a normal distribution - The mean of this distribution (the mean of the means) would be the population mean - The SD of this distribution would equal the population SD divided by the square root of the sample size - This is called the standard error (SE) of the sample mean [The Standard Error:] - The SE measures how precisely the population mean is estimated by the sample mean - The size of the SE depends on how much variation there is in the population and the sample size - The larger the sample size, the smaller the SE - We rarely know the population SD so we use sample SD to estimate the SE [Standard Deviation (SD) and Standard Error (SE):] - Standard Deviation (SD) - How much, on average, individual observations vary from the sample mean - Standard Error (SE) - How much, on average, mean scores from different sample vary from the true population mean [Repeated Sampling & Inference: ] - Under repeated random sampling, sampling distributions behave in predictable ways - Inference is possible because of the statistical properties of sampling distributions - This forms the basis of what are known as frequentist statistics **[The Normal Distribution:]** - In the general population, many variables approximate a normal or Gaussian distribution - Its frequency distribution is defined by the normal curve - As SD decreases, the bell becomes taller & narrower [Central Limit Theorem:] - Central role in stats as it can be shown that the sampling distribution of a mean is normal, even when the individual observations are not normal (provided the sample Is not too small) - This is the central limit theorem - It means that calculations based on the normal distribution are used to calculate confidence intervals and p-values - Statistical methods for proportions and rates are also based on approximations to the normal distribution [Standard Normal Distribution: ] - By a change of units, any normally distributed variable can be changed to a standard normal distribution - The mean of a standard normal distribution is 0 and the SD is 1 - This is done by subtracting the mean from each observation and dividing the SD [Z-Scores:] - an example for an individual with an IQ score of 125: - (125-100)/15 = +1.67 - Interpretation: an IQ of 125 is 1.67 standard deviations above the mean - A Z-score is a measure of how far an individual score is from the mean - Z-scores are measures in units of standard deviations - A Z-score is a measure of an observation relative to the other observations [Areas under the Normal Distribution curve:] - The standard normal distribution can be used to determine the proportion of the population with values in a specified range - Or equivalently: the probability that an individual observation from the distribution will lie within a specified range - This Is done by calculating the area under the curve (relevant to how we calculate CI and P-values) [Calculating Areas Under the Curve:] - The areas under the whole of the normal curve is 1 or 100% - The probability that an observation lies somewhere in the whole range of the curve is 1 or 100% - To calculate the proportion of people within a specified range, we use a computer or a look-up table - The rows of the table refer to z to one decimal place and the columns refer to the second decimal place [Properties of the Normal Distribution:] - Symmetrical - Completely described by Mean & SD - Shape always the same - 68.3 % of observations fall between -1 and +1 SD - 95.5% of observations fall between -2 and +2 SD - 99.7% of observations fall between -3 and +3 SD - Tails of the distribution - 5% of observations are \ +1.96 SD - 95% of observations fall between -1.96 and +1.96 SD [95% reference range:] - 95% of observations fall between -1.96 and +1.96 SD - (95.5% of observations fall between -2 and +2 SD) IQ in the general population - Mean = 100; SD = 15 - 95% reference range = mean +/- 1.96 x SD - 95% reference range = 100 - 29.4 to 100 + 29.4 - 95% reference range = 70.6 to 129.4 - 95% of the values in the population fall between 70.6 and 129.4 - 5% (2.5% in each tail) fall outside of these values [Confidence Intervals:] - In the sampling distribution of the mean, 95% of sample means lie within two SEs above or below population mean - The above statement can be written: - 95% CI: estimated mean +/- 1.96 SE  [Interpreting Confidence Intervals: sometimes MCQ question] - General interpretation: - Confidence intervals provide a range of likely values for the true value of the parameter we are trying to estimate - The data can be regarded as compatible with any value for the parameter which lies within the range of the 95% CI - Statistical Interpretation: - 95% of 95% CI for an estimate from the sample will include the true value of the parameter in the population - For any single sample, we can be 95% certain that the 95% CI contains the true value **[Calculating P-Value:]** [Defining P-Value:] - Probability of observing a difference between the two groups at least as large as that in our sample, if there was no effect in the population [Null Hypothesis & Null Values:] - We assume there is no association or difference between groups in the population - So, any observed difference between the means is due to sampling variation - Null Value for a difference = 0 (means, risk) - Null value for ratio = 1 (risk ratio, odds ratio) [Calculating a p-value for a difference:] - We use the fact that the sampling distribution of a difference is normal - If the null hypothesis is true, the mean of the hypothetical sampling distribution for the difference is zero - Our test statistic is the z score corresponding to the observed difference between means in the sample - The test statistic measures by how many standard errors the mean difference differs from the null value of zero - By convention, we use two-sided p-values - Our assessment of the probability that the result is due to chance is based on how extreme the departure is from the null rather than its direction - We include the probability that the difference might have been in the opposite direction - Normal distribution is symmetrical, so this probability is also 0.0082 - Two-sided p-value is 0.0082 + 0.0082 = 0.0164 [Test the hypothesis that CT can reduce psychiatric symptoms vs TAU:] Calculate the estimated difference between groups - Mean PANSS score: in CT group = 59 - Mean PANSS score in TAU group = 62 - Difference between means = -3 - Specify the null value - Expected difference if H0 is true = 0 - Find the standard error of the sampling distribution - Calculate the difference between the estimate and the null value in terms of SE (z score) - Find the p value