Inside the Black Box: A Simple Guide to Quantitative and High-Frequency Trading PDF
Document Details
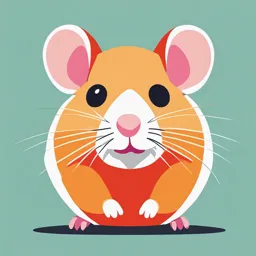
Uploaded by LovableZircon8781
University of East Anglia
2013
Narang, R. K.
Tags
Related
- High-Frequency Short Wave and Microwave Therapy (2023-2024) PDF
- Electrical Capacitive Transferrence and Ultra High Frequency (UHF) PDF
- Global Economy PDF
- Lecture 6: Market (micro)structure Regulation PDF
- Lecture 6 Key Market (Micro)Structure Rules PDF
- UNIBO_Valiante_Lecture_6_2024 PDF Lecture 6 Market (micro)structure Regulation
Summary
This book, Inside the Black Box, provides a guide to quantitative and high-frequency trading. It explores different aspects of quant trading strategies and the quant universe, offering insights into the strategies used and the market environment's impact on these strategies. The book includes real-life examples and case studies, aiming to provide practical knowledge for those interested in investment strategies.
Full Transcript
Chapter 1 Why Does Quant...
Chapter 1 Why Does Quant Trading Matter? Look into their minds, at what wise men do and don’t. —Marcus Aurelius, Meditations J ohn is a quant trader running a midsized hedge fund. He completed an undergraduate degree in mathematics and computer science at a top school in the early 1990s. John immediately started working on Wall Street trading desks, eager to capitalize on his quantitative background. After seven years on the Street in various quant‐oriented roles, John decided to start his own hedge fund. With partners handling business and operations, Copyright © 2013. John Wiley & Sons, Incorporated. All rights reserved. John was able to create a quant strategy that recently was trading over $1.5 billion per day in equity volume. More relevant to his investors, the strategy made money on 60 percent of days and 85 percent of months—a rather impressive accomplishment. Despite trading billions of dollars of stock every day, there is no shout- ing at John’s hedge fund, no orders being given over the phone, and no drama in the air; in fact, the only sign that there is any trading going on at all is the large flat‐screen television in John’s office that shows the strat- egy’s performance throughout the day and its trading volume. John can’t give you a fantastically interesting story about why his strategy is long this stock or short that one. While he is monitoring his universe of thousands of stocks for events that might require intervention, for the most part he lets the automated trading strategy do the hard work. What John moni- tors quite carefully, however, is the health of his strategy and the market environment’s impact on it. He is aggressive about conducting research on an ongoing basis to adjust his models for changes in the market that would impact him. 3 Narang, R. K. (2013). Inside the black box : A simple guide to quantitative and high-frequency trading. John Wiley & Sons, Incorporated. Created from uea on 2024-11-29 00:49:45. 4 The Quant Universe Across from John sits Mark, a recently hired partner of the fund who is researching high‐frequency trading. Unlike the firm’s first strategy, which only makes money on 6 out of 10 days, the high‐frequency efforts Mark and John are working on target a much more ambitious task: looking for smaller opportunities that can make money every day. Mark’s first attempt at high‐frequency strategies already makes money nearly 95 percent of the time. In fact, their target for this high‐frequency business is even loftier: They want to replicate the success of those firms whose trading strategies make money every hour, maybe even every minute, of every day. Such high‐ frequency strategies can’t accommodate large investments, because the op- portunities they find are small, fleeting. The technology required to sup- port such an endeavor is also incredibly expensive, not just to build, but to maintain. Nonetheless, they are highly attractive for whatever capital they can accommodate. Within their high‐frequency trading business, John and Mark expect their strategy to generate returns of about 200 percent a year, possibly much more. There are many relatively small quant trading boutiques that go about their business quietly, as John and Mark’s firm does, but that have demon- strated top‐notch results over reasonably long periods. For example, Quan- titative Investment Management of Charlottesville, Virginia, averaged over 20 percent per year for the 2002–2008 period—a track record that many discretionary managers would envy.1 On the opposite end of the spectrum from these small quant shops are the giants of quant investing, with which many investors are already quite familiar. Of the many impressive and successful quantitative firms Copyright © 2013. John Wiley & Sons, Incorporated. All rights reserved. in this category, the one widely regarded as the best is Renaissance Tech- nologies. Renaissance, the most famous of all quant funds, is famed for its 35 percent average yearly returns (after exceptionally high fees), with ex- tremely low risk, since 1990. In 2008, a year in which many hedge funds struggled mightily, Renaissance’s flagship Medallion Fund gained approxi- mately 80 percent.2 I am personally familiar with the fund’s track record, and it’s actually gotten better as time has passed—despite the increased competition and potential for models to stop working. Not all quants are successful, however. It seems that once every decade or so, quant traders cause—or at least are perceived to cause—markets to move dramatically because of their failures. The most famous case by far is, of course, Long Term Capital Management (LTCM), which nearly (but for the intervention of Federal Reserve banking officials and a consortium of Wall Street banks) brought the financial world to its knees. Although the world markets survived, LTCM itself was not as lucky. The firm, which aver- aged 30 percent returns after fees for four years, lost nearly 100 percent of its capital in the debacle of August–October 1998 and left many investors Narang, R. K. (2013). Inside the black box : A simple guide to quantitative and high-frequency trading. John Wiley & Sons, Incorporated. Created from uea on 2024-11-29 00:49:45. Why Does Quant Trading Matter? 5 both skeptical and afraid of quant traders. Never mind that it is debatable whether this was a quant trading failure or a failure of human judgment in risk management, nor that it’s questionable whether LTCM was even a quant trading firm at all. It was staffed by PhDs and Nobel Prize–winning economists, and that was enough to cast it as a quant trading outfit, and to make all quants guilty by association. Not only have quants been widely panned because of LTCM, but they have also been blamed (probably unfairly) for the crash of 1987 and (quite fairly) for the eponymous quant liquidation of 2007, the latter having se- verely impacted many quant shops. Even some of the largest names in quant trading suffered through the quant liquidation of August 2007. For instance, Goldman Sachs’ largely quantitative Global Alpha Fund was down an es- timated 40 percent in 2007 after posting a 6 percent loss in 2006.3 In less than a week during August 2007, many quant traders lost between 10 and 40 percent in a few days, though some of them rebounded strongly for the remainder of the month. In a recent best‐selling nonfiction book, a former Wall Street Journal reporter even attempted to blame quant trading for the massive financial crisis that came to a head in 2008. There were gaps in his logic large enough to drive an 18‐wheeler through, but the popular perception of quants has never been positive. And this is all before high‐frequency trading (HFT) came into the public consciousness in 2010, after the Flash Crash on May 10 of that year. Ever since then, various corners of the investment and trad- ing world have tried very hard to assert that quants (this time, in the form of HFTs) are responsible for increased market volatility, instability in the Copyright © 2013. John Wiley & Sons, Incorporated. All rights reserved. capital markets, market manipulation, front‐running, and many other evils. We will look into HFT and the claims leveled against it in greater detail in Chapter 16, but any quick search of the Internet will confirm that quant trading and HFT have left the near‐total obscurity they enjoyed for decades and entered the mainstream’s thoughts on a regular basis. Leaving aside the spectacular successes and failures of quant trading, and all of the ills for which quant trading is blamed by some, there is no doubt that quants cast an enormous shadow on the capital markets virtually every trading day. Across U.S. equity markets, a significant, and rapidly growing, proportion of all trading is done through algorithmic execution, one footprint of quant strategies. (Algorithmic execution is the use of computer software to manage and work an investor’s buy and sell orders in electronic markets.) Although this automated execution technology is not the exclusive domain of quant strategies—any trade that needs to be done, whether by an index fund or a discretionary macro trader, can be worked using execution algo- rithms—certainly a substantial portion of all algorithmic trades are done by quants. Furthermore, quants were both the inventors and primary innovators Narang, R. K. (2013). Inside the black box : A simple guide to quantitative and high-frequency trading. John Wiley & Sons, Incorporated. Created from uea on 2024-11-29 00:49:45. 6 The Quant Universe of algorithmic trading engines. A mere five such quant traders account for about 1 billion shares of volume per day, in aggregate, in the United States alone. It is worth noting that not one of these is well known to the broader investing public, even now, after all the press surrounding high-frequency trading. The TABB Group, a research and advisory firm focused exclusively on the capital markets, estimates that, in 2008, approximately 58 percent of all buy‐side orders were algorithmically traded. TABB also estimates that this figure has grown some 37 percent per year, compounded, since 2005. More directly, the Aite Group published a study in early 2009 indicating that more than 60 percent of all U.S. equity transactions are attributable to short‐ term quant traders.4 These statistics hold true in non‐U.S. markets as well. Black‐box trading accounted for 45 percent of the volume on the European Xetra electronic order‐matching system in the first quarter of 2008, which is 36 percent more than it represented a year earlier.5 The large presence of quants is not limited to equities. In futures and foreign exchange markets, the domain of commodity trading advisors (CTAs), quants pervade the marketplace. Newedge Alternative Investment Solutions and Barclay Hedge used a combined database to estimate that almost 90 percent of the assets under management among all CTAs are managed by systematic trading firms as of August 2012. Although a great many of the largest and most established CTAs (and hedge funds generally) do not report their assets under management or performance statistics to any database, a substantial portion of these firms are actually quants also, and it is likely that the real figure is still over 75 percent. As of August 2012, Newedge estimates that the amount of quantitative futures money under Copyright © 2013. John Wiley & Sons, Incorporated. All rights reserved. management was $282.3 billion. It is clear that the magnitude of quant trading among hedge funds is substantial. Hedge funds are private investment pools that are accessible only to sophisticated, wealthy individual or institutional clients. They can pursue virtually any investment mandate one can dream up, and they are allowed to keep a portion of the profits they generate for their clients. But this is only one of several arenas in which quant trading is widespread. Pro- prietary trading desks at the various banks, boutique proprietary trading firms, and various multistrategy hedge fund managers who utilize quantita- tive trading for a portion of their overall business each contribute to a much larger estimate of the size of the quant trading universe. With such size and extremes of success and failure, it is not surprising that quants take their share of headlines in the financial press. And though most press coverage of quants seems to be markedly negative, this is not always the case. In fact, not only have many quant funds been praised for their steady returns (a hallmark of their disciplined implementation proc- ess), but some experts have even argued that the existence of successful Narang, R. K. (2013). Inside the black box : A simple guide to quantitative and high-frequency trading. John Wiley & Sons, Incorporated. Created from uea on 2024-11-29 00:49:45. Why Does Quant Trading Matter? 7 quant strategies improves the marketplace for all investors, regardless of their style. For instance, Reto Francioni (chief executive of Deutsche Boerse AG, which runs the Frankfurt Stock Exchange) said in a speech that algo- rithmic trading “benefits all market participants through positive effects on liquidity.” Francioni went on to reference a recent academic study show- ing “a positive causal relationship between algo trading and liquidity.”6 In- deed, this is almost guaranteed to be true. Quant traders, using execution algorithms (hence, algo trading), typically slice their orders into many small pieces to improve both the cost and efficiency of the execution process. As mentioned before, although originally developed by quant funds, these algo- rithms have been adopted by the broader investment community. By placing many small orders, other investors who might have different views or needs can also get their own executions improved. Quants typically make markets more efficient for other participants by providing liquidity when other traders’ needs cause a temporary imbalance in the supply and demand for a security. These imbalances are known as inefficiencies, after the economic concept of efficient markets. True ineffi- ciencies (such as an index’s price being different from the weighted basket of the constituents of the same index) represent rare, fleeting opportunities for riskless profit. But riskless profit, or arbitrage, is not the only—or even primary—way in which quants improve efficiency. The main inefficiencies quants eliminate (and, thereby, profit from) are not absolute and unassail- able, but rather are probabilistic and require risk taking. A classic example of this is a strategy called statistical arbitrage, and a classic statistical arbitrage example is a pairs trade. Imagine two stocks Copyright © 2013. John Wiley & Sons, Incorporated. All rights reserved. with similar market capitalizations from the same industry and with similar business models and financial status. For whatever reason, Company A is included in a major market index, an index that many large index funds are tracking. Meanwhile, Company B is not included in any major index. It is likely that Company A’s stock will subsequently outperform shares of Company B simply due to a greater demand for the shares of Company A from index funds, which are compelled to buy this new constituent in or- der to track the index. This outperformance will in turn cause a higher P/E multiple on Company A than on Company B, which is a subtle kind of inef- ficiency. After all, nothing in the fundamentals has changed—only the na- ture of supply and demand for the common shares. Statistical arbitrageurs may step in to sell shares of Company A to those who wish to buy, and buy shares of Company B from those looking to sell, thereby preventing the di- vergence between these two fundamentally similar companies from getting out of hand and improving efficiency in market pricing. Let us not be naïve: They improve efficiency not out of altruism, but because these strategies are set up to profit if indeed a convergence occurs between Companies A and B. Narang, R. K. (2013). Inside the black box : A simple guide to quantitative and high-frequency trading. John Wiley & Sons, Incorporated. Created from uea on 2024-11-29 00:49:45. 8 The Quant Universe This is not to say that quants are the only players who attempt to profit by removing market inefficiencies. Indeed, it is likely that any alpha‐oriented trader is seeking similar sorts of dislocations as sources of profit. And, of course, there are times, such as August 2007, when quants actually cause the markets to be temporarily less efficient. Nonetheless, especially in smaller, less liquid, and more neglected stocks, statistical arbitrage players are often major providers of market liquidity and help establish efficient price discov- ery for all market participants. So, what can we learn from a quant’s approach to markets? The three answers that follow represent important lessons that quants can teach us—lessons that can be applied by any investment manager. The Benefit of Deep Thought According to James Simons, the founder of the legendary Renaissance Tech- nologies, one of the greatest advantages quants bring to the investment process is their systematic approach to problem solving. As Dr. Simons puts it, “The advantage scientists bring into the game is less their mathematical or computational skills than their ability to think scientifically.”7 The first reason it is useful to study quants is that they are forced to think deeply about many aspects of their strategy that are taken for granted by nonquant investors. Why does this happen? Computers are obviously powerful tools, but without absolutely precise instruction, they can achieve nothing. So, to make a computer implement a black‐box trad- Copyright © 2013. John Wiley & Sons, Incorporated. All rights reserved. ing strategy requires an enormous amount of effort on the part of the developer. You can’t tell a computer to “find cheap stocks.” You have to specify what find means, what cheap means, and what stocks are. For ex- ample, finding might involve searching a database with information about stocks and then ranking the stocks within a market sector (based on some classification of stocks into sectors). Cheap might mean P/E ratios, though one must specify both the metric of cheapness and what level will be con- sidered cheap. As such, the quant can build his system so that cheapness is indicated by a 10 P/E or by those P/Es that rank in the bottom decile of those in their sector. And stocks, the universe of the model, might be all U.S. stocks, all global stocks, all large cap stocks in Europe, or whatever other group the quant wants to trade. All this defining leads to a lot of deep thought about exactly what one’s strategy is, how to implement it, and so on. In the preceding example, the quant doesn’t have to choose to rank stocks within their sectors. Instead, stocks can be compared to their industry peers, to the market overall, or to any other reasonable group. But the point is that the quant is encouraged to Narang, R. K. (2013). Inside the black box : A simple guide to quantitative and high-frequency trading. John Wiley & Sons, Incorporated. Created from uea on 2024-11-29 00:49:45. Why Does Quant Trading Matter? 9 be intentional about these decisions by virtue of the fact that the computer will not fill in any of these blanks on its own. The benefit of this should be self‐evident. Deep thought about a strat- egy is usually a good thing. Even better, this kind of detailed and rigorous working out of how to divide and conquer the problem of conceptualizing, defining, and implementing an investment strategy is useful to quants and discretionary traders alike. These benefits largely accrue from thorough- ness, which is generally held to be a key ingredient to investment or trading success. By contrast, many (though certainly not all) discretionary traders, because they are not forced to be so precise in the specification of their strategy and its implementation, seem to take a great many decisions in an ad hoc manner. I have been in countless meetings with discretionary traders who, when I asked them how they decided on the sizes of their positions, responded with variations on the theme of “Whatever seemed reasonable.” This is by no means a damnation of discretionary investment styles. I merely point out that precision and deep thought about many details, in addition to the bigger‐picture aspects of a strategy, can be a good thing, and this lesson can be learned from quants. The Measurement and Mismeasurement of Risk As mentioned earlier in this chapter, the history of LTCM is a lesson in the dangers of mismeasuring risk. Quants are naturally predisposed toward con- ducting all sorts of measurements, including of risk exposure. This activity Copyright © 2013. John Wiley & Sons, Incorporated. All rights reserved. itself has potential benefits and downsides. On the plus side, there is a certain intentionality of risk taking that a well‐conceived quant strategy encourag- es. Rather than accepting accidental risks, the disciplined quant attempts to isolate exactly what his edge is and focus his risk taking on those areas that isolate this edge. To root out these risks, the quant must first have an idea of what these risks are and how to measure them. For example, most quant equity traders, recognizing that they do not have sufficient capabilities in fore- casting the direction of the market itself, measure their exposure to the mar- ket (using their net dollar or beta exposure, commonly) and actively seek to limit this exposure to a trivially small level by balancing their long portfolios against their short portfolios. On the other hand, there are very valid concerns about false precision, measurement error, and incorrect sets of assumptions that can plague attempts to measure risk and manage it quantitatively. All the blowups we have mentioned, and most of those we haven’t, stem in one way or another from this overreliance on flawed risk measurement techniques. In the case of LTCM, for example, historical data showed that certain scenarios were likely, others unlikely, and still others had simply never Narang, R. K. (2013). Inside the black box : A simple guide to quantitative and high-frequency trading. John Wiley & Sons, Incorporated. Created from uea on 2024-11-29 00:49:45. 10 The Quant Universe occurred. At that time, most market participants did not expect that a country of Russia’s importance, with a substantial supply of nuclear weapons and ma- terials, would go bankrupt. Nothing like this had ever happened before. Nev- ertheless, Russia indeed defaulted on its debt in the summer of 1998, sending the world’s markets into a frenzy and rendering useless any measurement of risk. The naïve overreliance on quantitative measures of risk, in this case, led to the near‐collapse of the financial markets in the autumn of 1998. But for a rescue orchestrated by the U.S. government and agreed on by most of the powerhouse banks on Wall Street, we would have seen a very different path unfold for the capital markets and all aspects of financial life. Indeed, the credit debacle that began to overwhelm markets in 2007 and 2008, too, was likely avoidable. Banks relied on credit risk models that simply were unable to capture the risks correctly. In many cases, they seem to have done so knowingly, because it enabled them to pursue out- sized short‐term profits (and, of course, bonuses for themselves). It should be said that most of these mismeasurements could have been avoided, or at least the resulting problems mitigated, by the application of better judg- ment on the part of the practitioners who relied on them. Just as one cannot justifiably blame weather‐forecasting models for the way that New Orleans was impacted by Hurricane Katrina in 2005, it would not make sense to blame quantitative risk models for the failures of those who created and use them. Traders can benefit from engaging in the exercise of understanding and measuring risk, so long as they are not seduced into taking ill‐advised actions as a result. Copyright © 2013. John Wiley & Sons, Incorporated. All rights reserved. Disciplined Implementation Perhaps the most obvious lesson we can learn from quants comes from the discipline inherent to their approach. Upon designing and rigorously test- ing a strategy that makes economic sense and seems to work, a properly run quant shop simply tends to let the models run without unnecessary, arbitrary interference. In many areas of life, from sports to science, the hu- man ability to extrapolate, infer, assume, create, and learn from the past is beneficial in the planning stages of an activity. But execution of the resulting plan is also critical, and it is here that humans frequently are found to be lacking. A significant driver of failure is a lack of discipline. Many successful traders subscribe to the old trading adage: Cut losers and ride winners. However, discretionary investors often find it very difficult to re- alize losses, whereas they are quick to realize gains. This is a well‐documented behavioral bias known as the disposition effect.8 Computers, however, are not subject to this bias. As a result, a trader who subscribes to the aforementioned Narang, R. K. (2013). Inside the black box : A simple guide to quantitative and high-frequency trading. John Wiley & Sons, Incorporated. Created from uea on 2024-11-29 00:49:45. Why Does Quant Trading Matter? 11 adage can easily program his trading system to behave in accordance with it every time. This is not because the systematic trader is somehow a better person than the discretionary trader, but rather because the systematic trader is able to make this rational decision at a time when there is no pressure, thereby obviating the need to exercise discipline at a time when most people would find it extraordinarily challenging. Discretionary investors can learn something about discipline from those who make it their business. Summary Quant traders are a diverse and large portion of the global investment uni- verse. They are found in both large and small trading shops and traffic in multiple asset classes and geographical markets. As is obvious from the magnitude of success and failure that is possible in quant trading, this niche can also teach a great deal to any curious investor. Most traders would be well served to work with the same kind of thoroughness and rigor that is required to properly specify and implement a quant trading strategy. Just as useful is the quant’s proclivity to measure risk and exposure to various market dynamics, though this activity must be undergone with great care to avoid its flaws. Finally, the discipline and consistency of implementation that exemplifies quant trading is something from which all decision makers can learn a great deal. Copyright © 2013. John Wiley & Sons, Incorporated. All rights reserved. Notes 1. M. Corey Goldman, “Hot Models Rev Up Returns,” HFMWeek.com, April 17, 2007; Jenny Strasburg and Katherine Burton, “Goldman Sachs, AQR Hedge Funds Fell 6% in November (Update3),” Bloomberg.com, December 7, 2007. 2. Gregory Zuckerman, Jenny Strasburg, and Peter Lattman, “Renaissance Waives Fees on Fund That Gave Up 12%,” Wall Street Journal Online, January 5, 2009. 3. Lisa Kassenaar and Christine Harper, “Goldman Sachs Paydays Suffer on Lost Leverage with Fed Scrutiny,” Bloomberg.com, October 21, 2008. 4. Sang Lee, “New World Order: The High Frequency Trading Community and Its Impact on Market Structure,” The Aite Group, February 2009. 5. Peter Starck, “Black Box Trading Has Huge Potential—D. Boerse,” Reuters.com, June 13, 2008. 6. Terry Hendershott, Charles M. Jones, and Albert J. Menkveld, “Does Algorith- mic Trading Improve Liquidity?” WFA Paper, April 26, 2008. 7. www.turtletrader.com/trader‐simons.html. 8. Hersh Shefrin and Meir Statman, “The Disposition to Sell Winners Too Early and Ride Losers Too Long: Theory and Evidence,” Journal of Finance 40, no. 3 (July 1985). Narang, R. K. (2013). Inside the black box : A simple guide to quantitative and high-frequency trading. John Wiley & Sons, Incorporated. Created from uea on 2024-11-29 00:49:45. Copyright © 2013. John Wiley & Sons, Incorporated. All rights reserved. Narang, R. K. (2013). Inside the black box : A simple guide to quantitative and high-frequency trading. John Wiley & Sons, Incorporated. Created from uea on 2024-11-29 00:49:45.