HTH SCI 3C04 Week 4 Appraisal of Intervention Studies (Part B) PDF
Document Details
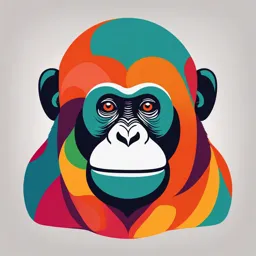
Uploaded by TransparentLemur
McMaster University
2024
Tags
Summary
This document is a lecture or presentation covering hypothesis testing, continuous outcome data analysis, and methodology in a nursing context. It includes examples to illustrate the statistical concepts.
Full Transcript
HTH SCI 3C04 Research Appraisal & Utilization in Evidence Informed Decision Making Week 4 Critical Appraisal of Intervention Studies (Part B) 4.1 – Hypothesis Testing Hypothesis Testing Quantitative research uses statistical measures to understand relationships between exposures/interventions and ou...
HTH SCI 3C04 Research Appraisal & Utilization in Evidence Informed Decision Making Week 4 Critical Appraisal of Intervention Studies (Part B) 4.1 – Hypothesis Testing Hypothesis Testing Quantitative research uses statistical measures to understand relationships between exposures/interventions and outcomes Answering research questions uses a process called hypothesis testing Process begins with a null hypothesis: “Null hypothesis” OR H0: there is no difference between the intervention(s) and control group(s) Hypothesis Testing Consider… We have a drug that might reduce hypertension: We give the new drug (Intervention) to a group of people with hypertension and the currently-prescribed drug (Control) to a similar group of people After 3 months, we look at the difference between the two groups in change in systolic blood pressure (SBP) from baseline to 3 months (Outcome Measure) Null hypothesis: There is no difference in the change in SBP between the Intervention Group and the Control Group Hypothesis Testing Null hypothesis: assumed to be true until analysis of data from a study suggests otherwise If the difference between the groups is large enough, we may reject the null hypothesis: If so, we “accept” the opposite, that is, there is a difference between the groups But, a study sample cannot provide 100% certainty – why? Hypothesis Testing: Two Errors Type I Error (⍺): “false positives” – claiming there is a difference between the groups when there isn’t one Type II Error (β): “false negative” – claiming there is no difference between the groups when there is one Both errors occur in a hypothesis test Type I & II Errors H0 HA Variance = 2 /n POWER= 0 1 DO NOT REJECT REJECT 1- Type I Error Type I Error: claiming there is a difference between the groups when there isn’t one. To limit this error, researchers declare a level of significance (⍺) for the hypothesis test ⍺ = probability researchers accept of making this error For hypothesis test with a 95% confidence level, ⍺ = 5% Type II Error Type II Error: Claiming there is not difference between groups when there is one. Researchers would like to limit this error too, but: Type I & II errors are related, decreasing one increases the other Type I error receives priority in hypothesis testing ⍺ of 5% equates to β of 20% Most common reason for Type II error: small sample size (smaller effects require larger samples to detect) 4.2 – Continuous Outcome Data Continuous Data Example: study testing a new weight loss drug compared to a currentlyprescribed drug (weight loss is measured in lbs) To analyze our results, we would look at the two groups as a whole by calculating the Mean and Standard Deviation (SD) for each group: New Drug (Intervention) Group of 25 people: mean weight loss of 14.4 lbs (SD=8.5) Existing Drug (Control) Group of 25 people: mean weight loss of 7.3 lbs (SD=4.5) It looks like there is a difference between the means, but is it a True difference? The t-Test (& ANOVA) We need a statistical test and appropriate confidence level to compare weight loss in the two groups: Confidence Level: 95% CI, ⍺ = 5% Statistical tests for comparing groups on a continuous outcome: T-Test is appropriate from comparing 2 groups ANOVA is appropriate for 3+ groups Both tests determine if the difference between mean values of the groups are statistically significant The t-Test (& ANOVA) Our weight loss example involves 2 groups, so we calculate the observed t statistic and determine the p value for it, and compare it to our threshold ⍺ of 5% Result: t – 3.7, p 1, For Example: RR = 4.2 Correct: Intervention subjects had 4.2 times the risk of the outcome compared to Control subjects. Intervention subjects had a 320% more risk of getting the outcome compared to Control subjects. Incorrect: Intervention subjects had 4.2 times more risk of the outcome compared to Control subjects. ORs: use same wording as RRs, since these are interpreted as if they were RRs See: https://sphweb.bumc.bu.edu/otlt/MPH-Modules/EP/EP713_Association/EP713_Association3.html RRs: Interpretation, Pitfalls RR < 1, For Example: RR = 0.57 Correct: Intervention subjects had 0.57 times the risk of the outcome compared to Control subjects. Intervention subjects had a 43% less risk of getting the outcome compared to Control subjects. Incorrect: Intervention subjects had 57% less risk of the outcome compared to Control subjects. ORs: use same wording as RRs, since these are interpreted as if they were RRs See: https://sphweb.bumc.bu.edu/otlt/MPH-Modules/EP/EP713_Association/EP713_Association3.html 4.4 – Categorical Outcome Data: Dichotomous Outcomes Analyzed using a Modelling Approach Modelling Approaches vs 2x2 Contingency Table Analyses Modelling approaches use a statistical procedure to estimate the ORs and RRs Common statistical procedures include regression methods suitable for dichotomous outcomes, such as: logistic regression for estimating ORs log-binomial or (modified) Poisson regression for estimating RRs Modelling approaches increasingly used to estimate ORs and RRs in RCTs and observational studies - why: allow the use of categorical and/or continuous risk factors (exposures) (Note: a 2x2 table only applies to categorical factors) reduce risk of potential bias in RR and OR estimates by adjusting for confounding variables ORs and RRs generated by modelling methods interpreted the same way as those derived from 2x2 contingency tables Example: Framingham Heart 1 Study Large prospective cohort study (24 years of follow-up) Research Question: Does cholesterol level predict development of angina over 24-year follow-up? Outcome (dichotomous): developing angina during 24-year follow-up (Yes/No) Primary predictor of interest (continuous): serum cholesterol level (mg/dL) Potential confounding variables (categorical and continuous): sex, smoking status, diabetes diagnosis, age, BMI, heart rate Analysis (OR): logistic regression [Note: a 2x2 contingency table to estimate the OR cannot be used because: 1) primary predictor continuous, and 2) significant confounding may bias the OR estimate so should adjust] 1LaValley, MP. (2008). Statistical Primer for Cardiovasular Research - Logistic Regression. Circulation. Pg. 2395-2399. DOI: 10.1161/CIRCULATIONAHA.106.682658 Example: Framingham Heart Study – Cont’d1 Cholesterol (primary predictor of interest): unadjusted OR (95% CI): 1.412 (1.297, 1.537) adjusted OR (95% CI): 1.404 (1.284-1.535) 95% CIs for both ORs exclude 1, thus ORs statistically significant (higher cholesterol levels are associated with higher odds of developing angina) Not a large difference between unadjusted and adjusted OR for cholesterol, which means there is little confounding of the effect of cholesterol on angina as a result of the other predictors included in the adjusted model (larger confounding effects result in larger discrepancies between the unadjusted and adjusted ORs) 1LaValley, MP. (2008). Statistical Primer for Cardiovasular Research - Logistic Regression. Circulation. Pg. 2395-2399. DOI: 10.1161/CIRCULATIONAHA.106.682658 4.5 – Hazard Ratios (HRs) Hazard Ratios (HRs) compared to RRs & ORs HR: ratio of the rates of an event (hazard) in two groups takes timing into account tells us if an intervention/risk factor changes the rate at which an event occurs RRs and ORs: focus on the total number of events over entire study period do not take timing into account tells us if an intervention/risk factor changes the risk of the event (RR) or the odds of the event (OR) HRs, RRs and ORs: all are measures of “risk”, each suited to different study designs and research questions all are ratios all have CIs, if CI excludes 1 the HR/RR/OR is statistically significant Hazard Ratios (HRs) HRs used with modelling approach called survival analysis, yields survivorship curves that show: occurrence of some event over time within a group vertical axis corresponds to proportion experiencing the event of interest within each group horizontal axis corresponds to time Interpretating HR: HR curve shows the rates that event occurs within a group, can compare rates for different groups HR = 1 indicates that the event occurs at the same rate in each group at any given time interval HR ≠ 1 indicates that the event occurs at a different rate in each group at any given time interval HR = RR? Some prominent sources state that the two measures are more or less equal, e.g., glossary at British Medical Journal website: “Hazard ratio (HR): Broadly equivalent to relative risk (RR); useful when the risk is not constant with respect to time. It uses information collected at different times. The term is typically used in the context of survival over time.” [https://bestpractice.bmj.com/info/us/toolkit/ebm-tools/a-glossary-of-ebm-terms/] But, this is a broad equivalence - there is a difference (Stare & Maucort-Boulch, 2016): RR = ratio of two probabilities (probability of the event in one group compared to probability in the other group) calculated for the entire study period With survival (used with HR), we need to state in which time interval the probabilities are calculated, thus the hazard can differ depending on the time interval chosen HR for entire study period = weighted RR during the entire study period For short time intervals, the RR and HR similar, but as interval lengthens the measures diverge Example: Survival Rate in Cirrhosis Patients with ≤ 90 vs > 90 day Hospital Readmission2 Large retrospective cohort study Research Question: Is early hospital readmission associated with survival rate in patients with cirrhosis? Outcome (dichotomous): died (Yes/No) Primary predictor of interest (categorical): early (≤ 90 days) vs late (>90 days) hospital readmission Potential confounding variables (categorical and continuous): age, sex, ethnicity, income, Charlson score (mortality index) Analysis (HR): survival analysis 2 Mah, JM, Dewitt, Y, Groome, P. et al. Early hospital readmission and survival in patients with cirrhosis: A population based study. Canadian Liver Journal. Vol. 2, No. 3, 2019, pg 109-120. DOI: 10.3138/canlivj.2018-0025 Example: Survival Rate in Cirrhosis Patients with ≤ 90 vs > 90 day Hospital Readmission – Cont’d2 Hospital readmission (primary predictor of interest): unadjusted HR (95% CI): 1.75 (1.72, 1.78) adjusted HR (95% CI): 1.56 (1.53, 1.59) 95% CIs for both HRs exclude 1, thus HRs statistically significant (early hospital readmission [≤ 90 days] is associated with higher risk of death compared to late [> 90 days] hospital readmission) difference between unadjusted and adjusted HRs indicates confounding effect due to other predictors included in the adjusted model 2 Mah, JM, Dewitt, Y, Groome, P. et al. Early hospital readmission and survival in patients with cirrhosis: A population based study. Canadian Liver Journal. Vol. 2, No. 3, 2019, pg 109-120. DOI: 10.3138/canlivj.2018-0025 Additional Resources (HRs) Helpful references: course textbook (Chapter 9, pg. 91-92, Included in Required Reading) article by George, Stead & Ganti (2020), supplemental reading for those interested in learning more References George A, Stead T S, Ganti L (August 26, 2020) What’s the Risk: Differentiating Risk Ratios, Odds Ratios, and Hazard Ratios?. Cureus 12(8): e10047. DOI 10.7759/cureus.10047 LaValley, MP. (2008). Statistical Primer for Cardiovasular Research - Logistic Regression. Circulation. Pg. 2395-2399. DOI: 10.1161/CIRCULATIONAHA.106.682658 Mah, JM, Dewitt, Y, Groome, P. et al. Early hospital readmission and survival in patients with cirrhosis: A population based study. Canadian Liver Journal. Vol. 2, No. 3, 2019, pg 109-120. DOI: 10.3138/canlivj.2018-0025 Stare, J, Maicort-Boulch, D. Odds Ratio, Hazard Ratio, and Relative Risk. Metodolo.ski zvezki, Vol. 13, No. 1, 2016, 59-67