HLST 4200 Exam Review PDF
Document Details
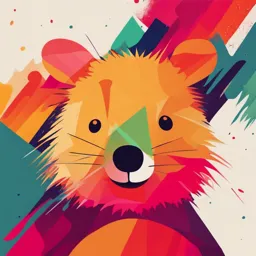
Uploaded by FineLookingSodalite
Tags
Summary
This document provides an overview of fixed designs, including experimental, quasi-experimental, single-case, and non-experimental designs. It also covers different types of surveys, interviews, and sampling methods, along with considerations for social desirability bias and maximizing response rates.
Full Transcript
HLST 4200 EXAM REVIEW WEEK 7 Different fixed designs Experimental design Quasi-experimental design Single case design Non-experimental fixed design o No active manipulation o Often looks at many more variables than an experiment/quasi-experiment Non-experimental fixed designs Might look at...
HLST 4200 EXAM REVIEW WEEK 7 Different fixed designs Experimental design Quasi-experimental design Single case design Non-experimental fixed design o No active manipulation o Often looks at many more variables than an experiment/quasi-experiment Non-experimental fixed designs Might look at: o Relationships between variables (relational design) E.g. relationship b/w physician age and type of care received o Differences between groups (comparative design) E.g. do females perform better than males in a course? o Trends over time (longitudinal design) Cross-sectional survey – look at many variables at once, most common tactic (method) for the above strategies (designs) o Gives you a picture of something on the inside but only seeing it at one point in time o E.g. surveys Main weakness: these are obtrusive data subject to social desirability bias (people will sometimes report things that make them look better) Types of surveys: when each are most appropriate Paper and pencil/online survey: most common in our work, identifiable list, more personal questions that require confidentiality or anonymity o anonymity can make people more honest – gives people permission to feel safe or to be honest Telephone interview: large sample surveys, use RDD or for targeted large sample surveys in appropriate populations o Maternity experience, Ontarians HC sat o 1-1, often done with random digit dialing In-person structured interview use for: o Simple short innocuous questions o Works well with populations with limitations (CAPI) o No list from which to ID people in advance (food services) o Can work well with short questions In-person interview surveys Good for non-threatening situations, need for fast data, no clear sample list o E.g. Sit at parking kiosk to get views on parking rates o Not if we’re asking really sensitive questions Main limitation: “the presence of the interviewer opens the doors for factors to do with the interviewer: skills, experience, personality, and degree of involvement in or alienation from the research” Multiple interviewers introduce additional variation into situations we try out best to control as researchers (less of a problem in less structured interviews) o Different interview styles, slighted changes to q wording or protocol o Differences in rapport with subjects Paper & Pencil/online surveys More often online now More common in research than ^ Key issues to consider with any survey (in person or not) o Instrument design and questions Are the questions good and reliable? o Sample to survey o Ensuring high response rates Still try to understand that certain things happen and that’s why we don’t always get results The value of survey research Researchers tend to have polarized views about surveys. Some see the survey as the central real-world strategy – others view them as generating large amounts of data, often of dubious value. Falsely prestigious b/c of their quant nature, the findings are seen as the product of uninvolved respondents whose answers are a mixture of politeness, and desire to be seen in a good light rather than their true feelings, beliefs or behaviour o Reflects social desirability issue o Value of survey data really depends on social desirability issue and maximize response rate – create environment where want to respond numerous opportunity for error to be introduced in the survey process E.g. different interpretation of question Data entry errors Accidentally filling in the wrong bubble SAMPLING What is generalizability? It reflects the extent to which findings from a study can be applied to or extended to (e.g. generalized to) those in the general population Our approach to sampling influences our ability to generalize Sampling Plan – FOUR questions you need to work through carefully to address sampling issues 1. What is the population you want to be able to comment on? 2. Will you use a census or sample approach? a. If using a sampling approach, identify your sampling frame b. E.g. will we test everyone or a sample? 3. Q3 a. need to determine sample size b. need to determine what type of sample Q2. Sample vs Census … practical questions help determine this think of o Feasibility (resources, practicality) E.g. who do you have access to? What resources do you have? Money? Too many people to study that it’s not practical? o Whether list is available (is the population a clearly definable group?) o How many people do we need to survey? Are there sub-groups we want to report on? When defining a sampling frame, must ask if it is representative of the population of interest o If it is not, you will have generalizability limitations Sampling frame = a list of those within a population who can be sampled Q3a. What should the size of your sample be? Hard question to answer You want large samples – the larger the sample, the better you will be able to generalize o A large sample = more representative of pop. w/in reason – there are limitation to getting large sample, e.g. cost Often what you are studying will determine how many you survey Rule of thumb of 10-15 cases per variable Large sample limit the influence of outliers or extreme observations – e.g. if you want to know avg age of York undergrads and I sample 3 people and get one mature student who is 42 the avg age of my sample will be farther from the pop avg than if I sample 10 or 20 or 200 Power Power is the main issue if you are looking for difference or at relationships o B/c it tells you if you will have a big enough sample to detect the difference/relationship you are looking for if it actually exists o Put differently, under-powered studies can fail to detect an effect that exists – not a large enough sample if you can’t see the relationship (type 2 error) o Type 1 error = detecting something that isn’t there o Type 2 error = not detecting something that is there o Power analysis will tell you if you have a big enough sample Power analysis helps determine sample sizes for studies where you have some data on the outcome variables you will measure In order to do a power calculation, it needs to be done with measures that have already been used Help you determine sample size ONLY for studies for which you already have some data on the measures you’re going to use Power analysis ensures you have a large enough sample to be able to detect the differences you are looking for if they exist Need to have previous data b/c you need to enter things like approx. std. deviation and pop. Mean based on other studies – this information will determine power Rule of thumb = 80% A study powered at 80% means you have an 80% chance of detecting a relationship if it exists o Don’t do 100% power b/c requires too large a sample size and we allow room for error Power relevant in all kinds of fixed studies – not just survey designs Allows you to know if you have an appropriate sample size to conduct a study – can just keep increasing the sample size until you hit at least 80% If it’s a new study, then you can’t do a power study b/c the measures haven’t been used before – a power calculation is how you can prove your study is worth doing with an appropriate sample size Q3b. What type of sample? Probability vs Non-Probability Sampling Probability (representative) sampling, you know the probability that any person will be included in the sample o Possible to generalize from sample to population (the sample is taken to be representative of the population) o The larger the sample, the lower the error in generalizing o Clearly defined sampling frame and able to say/identify the likelihood that a person will be selected – allows you to think about generalizability o E.g. 6000 ppl, 50% will be selected Non-probability sample, it is not possible to specify this probability … typically b.c the population is not clearly defined (not a population of hospital cafeteria users, it will change) o Becomes difficult or impossible to generalize from sample to population o Can’t specify the chance someone will be selected Types of Probability Samples Simple random sample o E.g. list of 6000 patients and randomly sample 2000 of them – computerized Systematic o When you can’t randomize – still trying to pick people in an unbiased way o E.g. take every 3rd person on a list when you can’t computerize Stratified o Recognize there’s groups/strata in each population and ensuring there are people from each group E.g. different staff groups at a hospital – sample enough people from each group/strata o Proportionate to size of the population E.g. population varies, but still use a 50% sample across each strata If goal is not to compare groups but paint a picture of something among (e.g. staff satisfaction) the groups, then you can use this since there is no oversampling issue o Disproportionate to the size of pop E.g. population 1000; sample 500 Pop 200; sample 100 Pop 100; sample 100 – oversampled this group, but used 50% from other groups Use this for comparison because you need to have a sufficient # of staff in each group o Depends on what your research purpose is Cluster (e.g. geographical cluster sampling) Types of Non-probability sampling Convenience – food services survey o E.g. just grabbing people that come out of the café Dimensional – choosing high learning organizations for FGs on learning from error o E.g. learning from failure study – choosing hospitals that are doing a lost to change safety culture already Purposive – in grounded theory to refine a theory you go out to more cases o Usually more in qualitative research Snowball – subjects you interview (or other people) suggest additional knowledgeable subjects Cluster sampling is typically used when the researcher cannot get a complete list of the members of a population they wish to study but can get a complete list of groups or 'clusters' of the population. It is also used when a random sample would produce a list of subjects so widely scattered that surveying them would prove to be far too expensive, for example, people who live in different postal districts in the UK. Generalizability – problems at several junctures need to be considered and discussed 1. Is the sampling frame representative of the population? 2. Is the sample truly representative of the sample frame? 3. Are respondents representative of those sampled? Enhancing response rates High RR is critical for increasing generalizability by reducing non-response bias Can increase it through: o Survey design o Survey length and content o Confidentiality or anonymity o Prenotification o Cover letter o Follow up o Timing Designing and testing the survey Layout is important o Appealing o Not too lengthy o Easy to complete Pretesting/piloting o Are questions clear and simple? o Is it a positive experience or nuisance? o Time to complete? Confidentiality or anonymity Difference? o Confidential – they know who you are e.g. name, ID number, etc. something that could link back to you – but there’s a promise of confidentiality o Anonymity – no unique identifiers Benefits/drawbacks o Confidentiality – drawbacks = sometimes people don’t know if it’s actually confidential especially with sensitive data Drawback = data may be used against you Sometimes you need to use confidential surveys b/c you need to link the data from one survey to another, one survey to a health record, or data in another database Usually used with experiments b/c you need to be able to track them over time or follow up after an intervention o Anonymous = allows participants to be more honest – more valuable results Consider o One off survey vs need for follow up o Track over time o Link to other data Cover letter: the musts Other things Title, investigators, sponsor, background, purpose What do I have to do Why was I chosen What are the risks Will I benefit Do I have to participate What else does my participation involve Will I be paid or do I have to pay Will be my records/info be kept private Agreement to participate statement Letterhead, personalize How long Sell them on importance Explain why ID numbers used More detail on who will see the detail, how it will be handled and where it’ll be stored Who to call with questions about why you received survey or ethics concerns Incentives? Thanks in advance Follow up procedures (Dillman) 1. Send first mailing 2. After one week, mail reminder postcard to everyone 3. After 3 weeks send another cover letter and questionnaire to those who haven’t responded 4. After 5 weeks, another full mailing to non-responders (many stop at the previous step) 5. Track all correspondence carefully (returned questionnaires, calls saying not working there or shouldn’t be on the list) 6. Summarize response rate Web-based surveys A host of different issues arise o Timeline shorter b/w stages o Accuracy of email addresses – same or different from mailing info o Do people pay more or less attn to email vs mail survey invitations? o Formatting of email communications – email clients are all different Timing Avoid christimas and summer holiday times Data collection with reminder and 1 follow up typically takes at least 2 months Ability to generalize Based on how you sample Based on your response rates and any non-response bias o Need to ask who non-respondents are and whether you would expect them to answer any differently WEEK EIGHT Questionnaire measures What makes for a good question on a questionnaire? Interpretation of a question the same – not open to multiple interpretations – has to be very clear Easy to recall – may forget the details of an answer – a good question would ask you to recall things that most people would be able to recall with reasonably good accuracy People have the knowledge or experience to answer the question Validity – making sure we are measuring/asking what we think we’re measuring/asking Variability What makes for a good question? Follow this to get good, reliable, valid questions Length of the survey depends on the audience Use procedures that ensure comfort and trust Simple language Unambiguous Short, clear and to the point Not double-barreled o Two different things in the same question o E.g. not how are the lectures AND the readings Not leading o E.g. not – you love this course, don’t you? Don’t use negatives* o Confuses people but sometimes you want to use this o Cognitive speedbump – forces us to think before answering the question Avoid prestige bias o Questions that make people feel bad if they don’t answer a certain way o E.g. rate your education level; if you have a low education, you may feel bad Specific reference frame o E.g. making sure the timeframe is reasonable and you’re able to recall information Think carefully about sensitive questions Be explicit if you want one’s personal feeling o If you’re asking personal feelings, then tell them you’re looking for their opinion/perspective and not the truth/fact Avoid asking for personal details or do so with grouped option o Don’t ask income, age questions at the beginning and ask it in a sensitive way Behaviour focused questions Measuring ‘what we say’ vs ‘what we do’ o With surveys, we’re measuring what people say they do, not necessarily what they actually do Good response options Accurate/relative Exhaustive o Want to make sure there’s always a response for everyone o Good to include an ‘idk’ option o Frequency design, e.g. always, sometimes, never Mutually exclusive (except for all that apply) o Don’t want overlapping answers On the same dimension o Scale should make sense New vs existing measures Advantages o New o Existing – you can compare the data, e.g. comparing York experience data with other universities When it’s been used, you have data on whether the measure is valid and reliable – they’ve been tested, adjusted, etc. Main: already been shown to be a valid and reliable instrument Disadvantages o Existing – measures certain areas but may not exactly what you want May not be available in the public domain so they’re not available to be freely used Two most talked about aspects of good measures Reliability Construct Validity How consistently are we measuring Are we measuring what we think we are something – about consistency measuring? Test-retest reliability = when you test someone then test them again Internal consistency reliability = how each question is answered Often referred to as the psychometric properties of an instrument (the construction of instruments and procedures for measurement). Good psychometrics = good reliability and good validity Reliability Reflects the consistency with how we measure a construct o Is measurement consistent (e.g. stable over different situations [in workshop, at the office] and times [today and next week]) Same over two different time periods? Similar settings? o Are the items internally consistent with one another? E.g. answering questions about the same thing in a similar manner Reliability as a concept is: the correlation of an item, scale or instrument with a hypothetical one which truly measures what it is supposed to E.g. correlation of my weight on a scale with my “true” weight Since the “true” instrument is not available, reliability is estimated from: 1. Most common: correlation among the variables comprising the scale (Cronbach’s alpha a measure of internal consistency – above 0.7 is good) a. E.g. using 5 scales rather than 1 to estimate “true” weight – if all scales say 50 then can confident weight = 50 b. E.g. asking 5 questions about participative leadership rather than one question c. Using multiple tests/questions to answer one thing – gives you more confidence/reliability 2. Not as good: the correlation of two equivalent forms of the scale (split halves reliability) a. E.g. 10 questions, split into groups of 5, test correlation between the two groups 3. The extent to which an item, scale or instrument will yield the same score when administered in different times, locations, or populations, when the two administrations do not differ in relevant variables (e.g. test-retest reliability) The value of a reliability estimate (range 0-1) tells us the proportion of variability in the measure attributable to the true score o A reliability of .5 means that about half of the variance of the observed score is attributable to truth and half is attributable to error o A reliability of .8 means the variability is about 80% true ability and 20% error o There’s always measurement error, but a reliable one will likely give you less error, less than 20% Participative leadership defined A managerial style that invites input from employees on all company decisions Participative leadership or mentorship? 1. He backs up group members in their actions 2. He acts without consulting the group (R) 3. He provides career advice when asked - mentorship not PL 4. He gets group approval on important matters before going ahead 5. He encourages group members to look for the best career move - mentorship not PL Construct validity has to do with the logic of items which comprise measures of social concepts. A good construct has a theoretical basis which is translated through clear operational definitions involving measurable indicators Does it measure what you think it measures? Or is it tapping something slightly different? Establishing construct validity A definitional approach o Face validity – does it look right on the face of it? o Content validity – does the item’s content reflect the definition? A relational approach o Criterion-related validity – does it relate to other constructs in the way you would expect? (Includes predictive, convergent, and discriminant validity) Criterion-related validity Does it relate to other constructs in the way you would expect? Predictive validity – mentorship might predict length of relationship, PL unlikely to Convergent validity – the degree to which the measurement is related to variables it should be related to Discriminant validity – the degree to which the measurement is not related to variables it should not be related to Correlation should support both convergence and discrimination and therefore construct validity A self-report measure of clinicians’ orientation toward integrative medicine Interviews – what does integrative medicine mean to you? “free pile sorting” – method of grouping transcripts Identified key domains of integrative medicine (provider attitudes, referral, knowledge, practice) Developed 63 question statements to reflect these areas, used a ‘frequency’ response scale (never, rarely… always) Interviews to query item meaning and revise (pilot) Revised 56-item questionnaire sent out Used item-scale correlations with 4 predefined domains Revised to create 30-item, 5 scale survey 5 final domains on IM-30 o Awareness and openness to working with practitioners in other paradigms o Readiness to refer to other paradigms o Patient-centered care o Learning from alternate paradigms o Safety of integrative medicine Calculated Cronbach’s alpha (reliability) Used relational (criterion-related) approach to assess construct validity Relational approach to assess construct validity Examined the associations of the IM30 score with items assessing dual training, practice setting, prac type, and self-perceived integrativeness Hypothesized that practitioners who had received formal training in at least two healing paradigms* would score higher on IM30 than single trained Dimensionality of a construct Some constructs are one-dimensional and others have multiple dimensions Dimensions also called factors Is job satisfaction a one-dimensional construct? Or multi-dimensional? E.g. satisfaction w/ pay, peers, work, supervisor, promotion opportunities Using factor analysis to assess dimensionality Is safety culture unidimensional? o Use factor analysis to suggest the dimensionality of a construct … to uncover the underlying structure of a relatively large set of variables o FA = statistical technique that shows you whether there are different factors (dimensions) in the groups of items you are looking at o It shows you whether there are certain clusters of items that are highly correlated o EGA identifies diff factors and shows the extent to which items “load” on each factor Estimating reliability is doable Split-half reliability we randomly divide our items into two sets and calculate the total score for each randomly divided half. The split-half reliability estimate is simply the correlation b/w these two total scores There is a natural opportunity to establish test-retest reliability in experimental and quasi designs that use a no-treatment control group – how? Cronbach’s alpha looks at inter-item correlations (it is equivalent to the avg of all possible split half correlations); it can and should be run Establishing construct validity is challenging Face and content validity can and should be assessed Relational approach es (e.g. predictive, convergent and discriminant) can be more challenging o b/c you have to collect data on a bunch of other related concepts multitrait-multimethod matrix approach can achieve this using relational approaches and multiple methods Reliability vs Validity Related Imagine the center of the target is the concept you are trying to measure Each person you are measuring is a short at the target; measure perfectly and you hit the target center o Valid not reliable – answering things related to the target but it’s not consistent Developing a measure from scratch Scaling Different types of scaling are used for responses o Likert o Semantic differential Different adjectives on the extremes o Visual – analog Likert is by far the most common Floor and ceiling effects Generalizability “these hypotheses should be investigated in future studies conducted in other regions of the country” WEEK NINE Pilot testing – (testing out a survey/instrument before you send it out to your participants – small, convenient sample) a mechanism to assess how well a questionnaire works prior to administration Unclear questions? Unsure how to interpret? What did X question mean to you?... is that what the researcher intended? Any questions you did not answer? Important to you? Would you complete? If it’s not of interest to them, higher change they won’t complete it Time to complete Generate data for a power calculation Relatively easy and cheap thing too Often not done, but really important Interviews Individual Group (focus groups) A highly structured survey is a fixed design – close ended questions with a likert scale usually Cannot change order of questions, re-word questions, or express them differently Flexible Allowed to reword, reorder, etc. Depth interview – less questions – don’t do much of these unless you’re a pure qualitative researcher Logistics Time consuming to plan, record and analyze In-person 45 minutes – 1 hour o More ideal – better connection, rapport, better info from people, etc. Telephone – 20-30 minutes o Usually shorter b/c they’re sometimes less inclined to detail their answers o Sometimes interviewer is distracted Main steps Sign consents Questions No more than 5-6 main questions + Introduction – reiterate confidentiality Warm up (easy) Qs Main questions Cool down (easy Qs) Closure prompts and probes and open comment opportunity at the end o Prompts: list of possible answers o Probes: the “thoughtful listener”: conveying through silence, pauses and simple phrases (e.g. tell me more), repeating back what they said The “tell me more” Interviewers require skills Listening (more than talking) Clarity in explanation of info sought Mastery of the subject – the neurosurgeon tests me Convey warmth, compassion and enjoyment Pull more info from people Sense when you are too close Give participant valuable experience Direct discussion where you need it to go Take cues (seeing and hearing) Obtain closure ^ easier when face to face, which is why face to face > phone Group interviews can 1. Inform development of quantitative survey or other study (take place before) 2. Be stand alone source of info 3. Help understand quantitative results (take place after) 4. Be careful in using them to determine consensus in attitudes … why? 2 & 3 common for single interviews too Ensure you build survey questions that are actually important to the participants after doing a focus group Focus groups often used to create satisfaction surveys, etc. Shouldn’t assume everyone agrees Semi-structured interviews can elicit relational data across practitioners b/c the interviewer can deeply probe and openly explore practitioner attitudes and behaviours toward integrative medicine. This method was used instead of focus groups to maximize participant response variance. Focus groups suffer from lack of independence, among participants and unequal participation. Semi-structured interview is able to elicit complete and independent data from all participants Group interviews – logistics Time consuming to plan and analyze A lot to prepare (consents, guide, slides, or handouts, audio equipment, e.g.) Ideally want to have at least 2 members of the study team present o To help stay on track, provide clarification o One to take notes Audio recording is difficult – assess the environment o Buses and trucks and open windows o The paper rustler What do you know about group dynamics and how might this be a positive or negative force when conducting a force group? Positive: discussions, more ideas, etc. Negative: free riders who don’t answer, people who dominate the whole group, etc. Group interviews Plus side o Main advantage: synergy prompts new information Peer comfort, comments act as reminders o Can separate consistent themes from extreme views more easily than in single interviews Minus side o A few dominate and commandeer discussion o Added skills needed to be an effective moderator Practice and pilot test FG guide Harder to reign in many people than 1 person o Harder to control a group Role of FGs in survey development Want to ensure questions reflect things that are important to subject rather than researcher or the organization Particularly important in satisfaction surveys, QOL surveys o Palliative care survey development – tough but imperative compared to an ED experience survey FG Rules of Thumb Ideally: participants are homogenous strangers o e.g. don’t set up groups where there’s a different power structure, e.g. not doctors + nurses semi-structured interview, high moderator involvement 6-10 participants 3-5 groups/project When we observe people, sometimes what you see is what you hear Sometimes, the data is completely different than what you observe Ordinary vs “scientific” observation Social scientific observation – systematic and purposive nature o Study their surroundings regularly and repeatedly, with a curiosity spurred by theoretical questions about the nature of human action, interaction, and society Strengths of observation as a research method Enables the study of behaviour w/in subjects’ natural environment o What actually occurs in practice rather than what subjects might say or think they do Different observer roles may be adopted Can gain the trust of the researched group Access subjects not accessible via other methods Triangulation o Confirmatory or convergent function o Enhance completeness of understanding about the research phenomenon Challenges of observation as a research method Ethical issues Issues of rigour o Objectivity o Effect of researcher on setting Time implications o Tool development o Data collection o Data processing and analysis Financial implications 4 researcher roles according to the degree of observer participation Complete participant o Disguised entry to the field, challenges of maintaining a dual role, potential validity threats, e.g. recording data, ethical issues, e.g. subjects unaware of involvement, no informed consent Participant as observer o Identity of researcher known to subjects, challenges defining boundaries of researcher and participant role, temptation to “go native” Observer as participant (non-participant observation) o Observer present solely in research capacity, less risk of going native, risk of misunderstanding the observed Complete observer o Covert observation, ethical issues, e.g. invasion of privacy, no informed consent, eliminates Hawthorne effect Observer effect Effect of observer on subjects’ behaviour Contributing factors o Intrusiveness of observer o Observer and subject attributes o Explanation for observation Strategies to minimize observer effect Observer training Period of acclimatization Observation on repeated occasions Observing discreetly and unobtrusively Observer dress Problem of entry Any participant observer needs to gain access to the group, setting, or place to be studied May be difficult, time consuming; patience and sensitivity required Often achieved through formal and informal gatekeepers Official permission may be required “unstructured” data collection Descriptive observation Focused observation Be aware of observational biases o Selection attention o Selective encoding o Selective memory o Interpersonal factors Essential to have a system for recording E.g. leadership behaviours o Encouraging o Empowering o Demeaning, etc. Structured data collection Non-participant observation using a scale or other instrument Statistical analysis of data Positivistic perspective o Aspects of the construct are delineated in advance o Observation directed towards specific behaviour o Types of structured collection instruments include rating scales, checklists, event recorders o Convenient for recording observations o Facilitates standardization, permitting comparison of data within and between groups E.g. handwashing behaviours Culture of a neighbourhood starbucks 1. Observe and take notes 2. Look at (a) how customers interact with staff; (b) how customers interact with one another 3. Staff customer interactions a. Formal ----------- informal b. Highly personal --------- impersonal c. # of staff-customer interactions_____ d. # personalized:______ Structured observational sampling Time sampling o Occurrence or non-occurrence of behaviours during each time period Proportion of all hand washing during a shift that meets std of care Focal-subject sampling o All occurrences of behaviour of an individual or group are continuously observed Proportion of RNs where 90+% of hand washing meet std of care Observer performance Key challenge w/ interviews and questionnaires is what people choose to report (relying on self-reports) What is the corresponding (key) challenge w/ observation? What we see/notice/pay attention to Observer reliability: o Consistency of observer performance Observer training Inter-observer reliability testing Observer discussions throughout data collection period Observer drift o Instability in recording over repeated periods of observation due to forgetting, new learning, fatigue Observer training to agreed protocol Testing with an independent observer Observer field notes and discussions during the data collection period Archival/textual research Archival research o Using previously compiled info to answer a research question Types of archival research o Statistical records o Survey archives o Written records such as pt charts Textual data provides a different angle into what’s going on w/ an individual or in an organization Challenge: difficult to obtain; accuracy of info Texts and documents What’s the key limitation of textual data? “if it isn’t in the chart, it didn’t happen” “don’t put that in the minutes, put…” We need to ask o How are the documents written? Who reads them, for what purposes, how often? o What is recorded and what is omitted? o What is taken for granted? o What do readers need to know to make sense of them? Questions to consider 1. What advantage might document analysis have over interviews, FGs, or observation? 2. What advantage might interviews and FGs have over doc analysis and observation? WEEK TEN What are data? A representation of facts or concepts in an organized manner in order that it may be stored, communicated, interpreted, or processed Information collected by a researcher; any organized info… often thought of as statistical or quantitative, but they may take other forms as well – such as transcripts of interviews or videotapes of social interactions Not necessarily numbers – e.g. textual data – sometimes we reduce qualitative data to textual data for analysis but not always Many reasons qualitative approaches are used Qual approaches are often used to address broader research questions, rather than testing very specific hypotheses Qualitative approaches can be used for different reasons o Theory development o Theory testing, description, comparison o Instrument development Purpose of your research will determine details of the way you do your analysis o E.g. ethnographic studies or grounded theory approaches may be guided by diff principles and lenses, may involve far more data, several more iterations b/w analysis and data collection and less structure in the analysis than case studies Purpose of research is ultimately what dictates how we do analysis When the question is fairly clear, then you don’t need to do so much iteration between analysis and collection Template structure most common HC qualitative analysis that we do o Have some idea of the themes we’re expecting to see in our data and come up with codes for these themes in advance or as we do our initial data collection o Act as a template for our data – get a set of pre-defined questions that we expect to hear and slot our data into these ‘bins’ Quasi-statistical – open ended questions but count how many times you hear certain things in responses – counting words/phrases Data analysis in the research process (more positivist tradition – linear) When we analyse our data we do so in the context of what we know in our existing literature And we say what’s supported and not supported in the existing lit Not used in qualitative analysis Data analysis in research process (qual/template tradition) Where repetition is seen Our analysis encourages us to go back to the literature and/or go collect more data if there’s still uncertainty Overlap in data collection and analysis Data analysis begins during data collection Careful note taking central to good data collection o Include your reflections as your record… continue to include your reflections (“memos”) as you go thru the steps of analysis o Add comments on non-verbal communication, setting, circumstances We take notes on what we hear and on our own reflection ^ this is analysis that takes place while you’re doing the collection Common features of qualitative analysis 1. Giving codes to initial materials collected (interviews, documents, obs, eetc.) 2. Adding comments/reflections (memos) 3. Combing materials for similar themes, relationships, group differences or whatever your focus 4. Taking the themes that emerge out to the field to structure next wave of data collection 5. Distill a few generalizations that reflect clear consistencies in your data 6. Link your generalizations back to existing lit and theory Its iterative b/c e.g. if you hear information from different groups of people where the info from each group were conflicting, you would want to go back out in the field and either phrase questions differently or go to different people for more info Adding to new studies and not building on what we already know Key goal of qualitative analysis = data reduction – literally try to reduce large amounts of (usually textual) data down to clear, key emerging consistent themes Focusing our data and abstracting themes that keep emerging and allow us to essentially tell a story b/c qualitative data provides more Data reduction = process of selecting, focusing, simplifying, abstracting, coding and transforming the raw data into info or themes that allow you to tell a story To code or not to code? Analytical choices, biases, expectations will shape the story your data tells Data reduction =/= reduce everything to numbers Must keep the context to retain the meaning … use quotations to illustrate your themes Make sure you understand the processes, don’t get wound up in the terminology Qualitative allows us to study things in context The analysis process: Pre-analysis: conversion of raw data Most sources of qualitative data are converted to text, including field notes, taperecorded interviews, video-taped activities, etc.. Transcription: interview time = 5:1 o When we’re transcribing data, can take hours Qualitative data management software provides you a way to organize, retrieve, to manage – your data – it does NOT analyze data for you o Softwares usually used to help with analysis – useful not essential but NOT to analyze data NUD*IST now NVIVO most widely used qual software package Step 1 in data analysis: Having transcribed your data 1. Get a sense of the whole a. Read through the data sets … or at least the first few interviews/FGs b. Write down broad ideas as you read – things that jump out at you, just write it down c. If you moderated, you will already have a good sense of this Step 2: Generate topics… if you had specific questions you were looking for answers for, list those as topics o Reading through again, ask yourself What is that about? Is it important (relative to research question)? Note topics and themes you think you see emerging under each topic (e.g. common answers around the questions that guided the FG) o You can focus your data around your topic o Try to code your data to fall under one of your topics Step 3: Develop themes more thoroughly o Make a more complete list of topics and their themes (as you go through more data) o For each topic and theme, create label that best represents its meaning o Theme under each topic o E.g. topic 1: the value of different kinds of events for learning Themes: preventable events, near misses, frequent events, etc. Step 4: Try out classification scheme o Go back to a transcript and code the data using your topics and themes Step 5: Refine themes o Do they fit the data? o How many topics are necessary? o What about leftovers? Relevant or irrelevant? o Repeat steps 4 (trying your classification scheme) and 5 (refining) as necessary You may through many cycles o Keep qualitative nature and “richness” of data (thick description) Step 6: Identifying patterns and themes o Patterns are the relationships among categories o We are starting to tell our story Pre-determined or developed on an ongoing basis Usually a combo of both This can be the basis of theory development Step 7: Drawing and verifying conclusions o Continuous process, based on: Summary of data Identifying trends Identifying associations – local causation Consider counteragruments o Constantly verifying by going back to the data, literature, and making sure they’re telling us what we think we’re seeing and we’re not imposing that on the data Step 8 Strategies to confirm findings o Consider representativeness of your themes – what have you chosen from the transcripts? What have you ignored? o Observer bias – do you see what I see? Use inter-coder reliability o Take your themes back out to the field for verification – do additional research Validation in group and individual discussions with informants o When you feel confident about your findings, you still want to confirm them o How representative are our data? Steps in data analysis – summary 1. Get a sense of the whole by reading through transcripts or being present at the data collection 2. Generate / list topics – based on questions 3. Develop themes 4. Try out classification scheme 5. Refine theme a. Were the initial themes more complex than you thought 6. Identifying patterns and themes 7. Drawing and verifying conclusiosn 8. Strategies to confirm findings Goal: reduction construction Analytic rigour Keep close to the data o Making sure we’re using the data to see things and not our own pre-conceived things or what other people are telling us o Documentation Let data speak to us and don’t force data to say something it’s not o Quotes to illustrate points Direct quotes help make our case Will be stronger and clearer if you use participants actual words Use other people’s arguments to make your point Negative case analysis o Making sure we don’t see quotations that disconfirm or make us question what we see in the analysis Acknowledge limitations b/c the qual data analysis process is so subjective, there are additional things we need to keep in mind to ensure the processes are rigorous Conclusion Analysis is a systematic, rigorous, thought-based process “analysis of analysis” is an important part of qual research (part of reflexivity) Remember qual research and analysis can involve description, theory building, testing, survey creation… The role of FGs in survey development Particularly important in satisfaction surveys, QOL surveys where we want to ensure questions reflect things that are important to subjects rather than researcher or the org Also important for ensuring questions reflect “reality” Documenting methods and analysis Describe process used to seek info Explain why process is suitable Document x3 Describe intra- and inter- interview process Describe recording and transcription processes Describe all data reduction techniques and decisions, including who and how many involved… use of inter-coder agreement techniques, etc. All of this will be needed for your method descriptions A lot of rigour comes from documenting E.g. what process did you use? Why was it suitable? How did you identify your sample? Who does the analysis? Who takes the notes? If you just use notes from an interview, then you don’t know what’s missing from the notes Does the study describe any processes they use b/w interviews? All data reduction techniques? Inter-coder agreement – how many times these people agreed on which theme a quote fits Deficiencies of the human as analyst Data overload – we have limited capacity o Limited capacity to remember and retain and organize and sort out data in our minds The problem with first impressions o They stick and hard to undo Positive instances – we are selective in the information we pay attention to o Psychology says we hold onto the good stuff which means we need to look out for the negative instances in our data We seek order – so tend to ignore or undervalue unusual info o This means we tend to ignore or undervalue things that don’t fit into our predetermined order Some sources are more valuable than others but we tend to ignore or not know how to handle this We are overconfident in our judgements of what we think we see o We see a pattern in a data that fits with the way we see the world and we o Tend to take the things that are consistent with what we see in the world and are overconfident in it We are inconsistent – repeated evaluations of the same data (inter and intra-person) tend to yield different results o E.g. reading something one day will probably influence how you analyse your data that day compared to how you analysed it last week – this is why we sometimes need to reanalyze our data up to four times Implications for the researcher It is “soft” science not “easy” science Very labour intensive – takes a long time to code qual data and tell a story Garbage in, garbage out still applies Requires well trained investigator – can’t farm out your analysis in most cases o Open mind, expert listening skills o Sensitivity to subjects and situations o Knowledge of the subject matter so you can probe o Awareness of biases o Detailed understanding of what you are looking for is nearly impossible to impart to others Qualitative analysis, with its close up look, can identify mechanisms, going beyond sheer association. It is unrelentingly local, and deals with the complex network of events and processes in a situation. It can sort out the temporal dimension, showing clearly what preceded what … it is well equipped to cycle back and forth between variables and processes – showing that ‘stories’ are not capricious [whimsical], but include underlying variables and that variables are not disembodied, but have connections over time Allows you to understand, in a holistic way, a particular phenomenon Can’t get the same thing with a more structured approach WEEK 11 Knowledge translation (KT) questions - A complex process b/c there are multiple potential types of researchers – wer’re trained to present our research for other rigorous researchers - We have a KT problem What is knowledge translation? The exchange, synthesis, and ethically sound application of research findings w/in a complex set of interactions among researchers and knowledge users … in other words, knowledge translation can be seen as an acceleration of the knowledge cycle; an acceleration of the natural transformation of knowledge into use It is about using research evidence in practice We have different types of research, different users, and different ways of actually using the research Used how? Direct use (instrumental) A concrete application of research findings to Indirect use (conceptual) Symbolic use Changes in Using research to awareness, validate positions knowledge, attitudes, already taken AKA make specific decisions or changes in policies, procedures, and programs E.g. I will be using this drug b/c there is clinical evidence Conceptual use AKA enlightenment, informing the debate, adopting ideas, concepts and language of the research E.g. make people aware of something, change their attitudes, change their way of thinking of something political or tactical or strategic use E.g. strategic use of research, e.g. being a big advocate for pub health and only finding research that supports that – serves people’s agendas Why the emphasis on KT How are the research results traditionally shared for use? What’s wrong with this process? Why aren’t research results (multiple studies that synthesized into evidence) used? Some of the most straightforward evidence is not used: using clinical research in practice Research has clearly shown the benefits of using aspirin and beta blockers following an MI and in patients with coronary heart disease Yet only 76% of pts with an acute MI who should receive this therapy (e.g. those w/o contraindications to aspirin therapy) receive such therapy at discharge Fewer than half of all post-MI patients receive beta blockers as LT therapy Suggests a massive underutilization of research findings Takes about 20 yrs between research evidence emergence and use/practice in the field 30% of pts who underwent underwent angioplasty had a contraindication for the procedure o This means there in 30% of cases they studied where angioplasty was used, there was generally agreement that it wasn’t useful or effective… and may have been harmful Gaps in use of this kind of clinical evidence is striking given its inherent usability compared to other research evidence Where EBM ties in EBM emerged in response to data showing huge (unjustified) practice variations in the 70s … in other words clinical research was underutilized EBM is “the conscientious, explicit, and judicious use of current best evidence in making decisions about the care of individual patients” Other evidence we ignore KT: drug companies spent 10x what research agencies on dissemination The Knowing-Doing gap = 20 years KT on the clinical side So we see efforts to translate clinical research into practice guidelines (CPGs) to make it easier to apply research evidence The translation of clinical research (evidence) into CPGs is one example of KT in the clinical domain Evidence is incorporated into flowcharts to walk clinicians through a care path Models of how knowledge is utilized 4 models of knowledge utilization o Science push o Demand pull o Dissemination model o Interaction model Others describe similar models using different terminology Science Pull Model Supply based model where researchers are the source of ideas Disinterested knowledge is produced by devoted scientists Users/society are passive receptacles for the results Useful application will derive from good science, use is responsibility of practitioners “if we build it, they will come” If we produce the research, it will be used Major problems No one responsible for transfer (which is not automatic) o No is responsible for making sure the research is understood, etc. Raw research info is not “usable” by most o Not written for the user audience Demand Pull Model Users become the main source of ideas for directing research Becomes much more of a customer/contractor relationship Idea is that useful knowledge is produced by giving constraints to scientists Practitioners/D-Ms should tell scientists what the problem is Use follows directly from the problem-solving nature of the research D-Ms = users of this research o You come with the research problem/question and the researcher answers it Major problem o Too much emphasis on users, not on good research o Timelines of researchers and DMs don’t match anyway Usually takes yrs to conclude a study Dissemination Model Adds a step to the research process that includes dissemination mechanisms to transfer knowledge to potential user(s) In more traditional dissem models, potential users not involved in the research or the selection of info for transfer Major problem o Reception of results does not mean it gets used o Reinforces the “2 communities” problem “researchers” and “DMs” Interaction model Knowledge is co-produced and co-interpreted by scientists and practitioners and high levels of interaction should be sought Argues that KU depends on sustained interaction and the more sustained and intense the interactions that more likely the utilization Can begin with needs of either group o Safety culture started with the researchers then led to demand from provider organizations … now they ask how to change it Greater attn to the relationship b/w researchers and users then to the nature and quality of the research Most challenging model for scientist and practitioners Good for getting buy-in, support and interest Not good for: negotiation of process, politics comes into research Demand Pull and Dissemination not really used Two Camps exist Model 1 – very similar to the Science Push Model Traditional mode of organizing unis and research Main objective is the production of new knowledge Research structured in established disciplinary boundaries Scientific achievement within these boundaries Scientists are the guardians of quality Knowledge diffusion via publication in peer-reviewed journals Application derives from this process but is not predominant goal Funding research is a public responsibility Universities are the main loci Model 2 – very similar to Interaction Model Problem-solving is the main objective including developing capability in society Heterogeneous team + unstable social structure Transdisciplinary Contextualization of research and the localization of research End of academic monopoly on assessment of research (e.g. Merit panel at CHSRF) Diversification and de-institutionalization of knowledge diffusion activities Terminology As models have evolved so has the terminology used to describe the process we are talking about Idea is that research in its raw form is not usable by most people hence the change in terminology – exchange between researchers and DMs Why bring DMs into the research process? What works? Bringing DMs who can use the results of a particular piece of research into its [the research’s] formulation and conduct is the best predictor for seeing the findings applied. The clearest message from evaluation of successful research utilization is that early and ongoing involvement of relevant DMs in the conceptualization and conduct of a study is the best predictor of utilization Bringing DMs in to the process is the best predictor to getting that research used o Bringing users and creators of knowledge together during all stages of the research cycle is fundamental to successful KT The Dissemination Effort Model (Huberman) Despite its name, this is a key interaction model Huberman argues: Interpersonal links, spread through the life of a given study, are the key to research use Although parsimony may not be a strength of his model, comprehensiveness is Interactionist: we need to pay attn to a # of things Important to understand user’s interests A bunch of aspects are important in the dissemination – e.g. use infographics, videos, etc. Why is interaction so important? What do we know about KU? 1. KU is a political process a. Consider results on a benefits of privatization … or societal impact of liberalizing abortion laws b. One recent study found that interaction didn’t influence utilization of study results b/c study findings were in conflict with DM’s organizational and political interests 2. KU involves cognitive processes susceptible to bias a. Information or research findings that are consistent with our expectations tends to be accepted while info that is inconsistent tends to be challenged, questioned, and ultimately disregarded b. In another study, research findings that confirmed existing pub health practices on breast cancer prevention approaches were used equally by groups who interacted with the researchers and those that did not 3. KU is a social process a. RU and KT are highly social processes that are more successful in the presence of positive social interactions b/w communities b. It is often suggested that relationships and face-to-face contact are more important to effective research utilization than the quality, methods, or content of a research study Why is interaction so important? b/c KU is political and susceptible to congnitive biases, the social piece that is central to interaction becomes critical o e.g. using face-to-face contact in DM when there are value conflicts helps BUT our Implementation Science paper asked if the interaction model goes too far in suggesting that early and sustained interaction is always warranted There is almost nothing in the published lit about the conditions under which various interactive approaches are more or less successful For what kind of studies do you think early, sustained, or late interaction might be most appropriate? o Depends on the type of study – do you want interaction early so stakeholders can identify proper interventions? Or later to interpret and plan for improvement? Early & sustained interaction can be expensive and onerous Might there be some cases where decision maker involvement is most appropriate only at the interpretation and dissemination stage (e.g. a retreat to older dissemination models)? Conditions for later interaction 1. Studies with multiple, diverse stakeholders a. Multiple stakeholders may have competing or inconsistent priorities which may complicate an already challenging exchange process 2. Fixed methods (e.g. replication studies a. Leave no room for DM input into early aspects of research 3. Highly public or politicized research a. Is likely to attract interest regardless of prior involvement in the process 4. Addressability/actionability of results Conditions where we DON’T need interaction before or during the study but at the end Conditions for sustained interaction … the link to CBR – community-based research o Community-situated: begins with a research topic of practical relevance to