Heteroscedasticity
Document Details
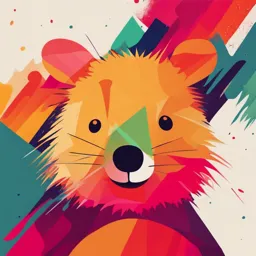
Uploaded by ClearerKoala
Ani Katchova
Tags
Related
- PCSII Depression/Anxiety/Strong Emotions 2024 Document
- A Concise History of the World: A New World of Connections (1500-1800)
- Lg 5 International Environmental Laws, Treaties, Protocols, and Conventions
- Ziraat Finans Grubu Uygulamaları
- Psychoanalytic Diagnosis: Understanding Personality Structure
- Dynamics of the Cell Membrane PDF - Istanbul Atlas University
Summary
This document provides an outline and overview of heteroscedasticity, its consequences, and various tests and methods for handling it. Topics covered range from definitions and tests to corrections with methods like weighted least squares and feasible generalized least squares.
Full Transcript
Heteroscedasticity Ani Katchova © 2020 by Ani Katchova. All rights reserved. Outline • Heteroscedasticity definition • Consequences of heteroscedasticity • Heteroscedasticity tests • Breusch-Pagan test • White test • Alternative White test • Corrections for heteroscedasticity • Robust stand...
Heteroscedasticity Ani Katchova © 2020 by Ani Katchova. All rights reserved. Outline • Heteroscedasticity definition • Consequences of heteroscedasticity • Heteroscedasticity tests • Breusch-Pagan test • White test • Alternative White test • Corrections for heteroscedasticity • Robust standard errors • Weighted Least Squares (WLS) • Feasible Generalized Least Squares (FGLS) 2 Heteroscedasticity • Homoscedasticity ???????????????????????????????????? ???????????? ???????????? ???????????? ?????????????????? ,???????????? ????????????2 , … ,???????????? ???????????????????????? = ???????????? 2 • The variance of the error term ????????????does not differ with the independent variables. • Heteroscedasticity ???????????????????????????????????? ???????????? ???????????? ???????????? ?????????????????? ,???????????? ????????????2 , … ,???????????? ???????????????????????? ≠???????????? 2 • The variance of the error term ????????????differs with the independent variables. 3 Consequences of heteroscedasticity • Under heteroscedasticity: • OLS estimators are still unbiased and consistent • R-squared is still valid • The variance formulas for the OLS estimators are not valid • The t- tests and F -tests are not valid • The OLS estimator is not the best linear unbiased estimator (BLUE). There maybe more efficient linear estimators. 4 Testing for heteroscedasticity • Hypothesis testing for heteroscedasticity: ???????????? 0 :???????????????????????????????????? ???????????? ???????????? ?????? ,???????????? 2 ,… ???????????? ???????????? = ???????????? 2 (homoscedasticity) ???????????? ???????????? :???????????????????????????????????? ???????????? ???????????? ?????? ,???????????? 2 ,… ???????????? ???????????? ≠???????????? 2 (heteroscedasticity) ???????????????????????????????????? ???????????????????????? =???????????? ???????????? 2 ???????????? − ???????????? ???????????????????????? 2 = ???????????? ???????????? 2 ???????????? • Under assumption 4 zero conditional mean, ???????????? ???????????????????????? =0.So the variance of ????????????is the expected value of ???????????? 2 . ???????????? 0 :The expected value of ???????????? 2 does not vary with the independent variables. (homoscedasticity) ???????????? ???????????? : The expected value of ???????????? 2 varies with the independent variables. (heteroscedasticity) 5 Testing for heteroscedasticity • Regression model ????????????= ???????????? 0 +???????????? Testing for heteroscedasticity • After the regression models for � ???????????? 2 are estimated: ???????????? 0 : ???????????? F-test for overall significance ????????????− ???????????????????????????????????????????????? = ???????????? � ???????????? 2 2 / ???????????? (??????−???????????? � ???????????? 2 2 ) /(???????????? −???????????? −??????) • LM-test ????????????????????????−???????????????????????? Heteroscedasticity-robust variance • If heteroscedasticity is found, then robust standard errors should be used. • Regression model ????????????= ???????????? 0 +???????????? ???????????????????????????????????? ̂ ???????????????????????? = ∑ ???????????? = 1 ???????????? ̂ ???????????????????????????????????? 2 � ???????????????????????? 2 ???????????????????????? ???????????? ????????????2 • These are called the White -Huber- Eickerstandard errors (or variance) • This formula is valid only in large samples (asymptotically) • The t-tests are valid asymptotically • Software programs can calculate robust standard errors. 8 Weighted Least Squares (WLS) • If the heteroskedasticity form is known, the Weighted Least Squares (WLS) can be used to estimate the model. • If the heteroscedasticity is known up to a multiplicative constant: ???????????????????????????????????? ???????????? ???????????? =???????????? 2ℎ ???????????? =???????????? 2ℎ ???????????? • Multiplying the errors ???????????? ???????????? by 1/ ℎ ???????????? will make the errors homoscedastic. ???????????????????????????????????? ???????????????????????? ℎ???????????? = 1 ℎ ???????????? ???????????????????????????????????? ???????????????????????? = 1 ℎ ???????????? ???????????? 2ℎ???????????? = ???????????? 2 • Estimate a transformed model where all variables and the constant are multiplied by 1/ ℎ ????????????. ???????????????????????? ℎ???????????? = ????????????0 ℎ???????????? + ???????????????????????????????????????????????? ℎ???????????? + ????????????2????????????????????????2 ℎ???????????? + ⋯ + ???????????????????????????????????????????????????????????? ℎ???????????? + ???????????????????????? ℎ???????????? ????????????????????????∗ = ???????????? 0???????????????????????? Weighted Least Squares • To estimate the transformed model: • ???????????????????????? ℎ???????????? = ????????????0 ℎ???????????? + ????????????1????????????????????????1 ℎ???????????? + ????????????2????????????????????????2 ℎ???????????? +⋯ + ???????????????????????????????????????????????????????????? ℎ???????????? + ???????????????????????? ℎ???????????? • OLS will minimize the sum of squared residuals: • min ∑ ???????????????????????? ℎ???????????? − � ????????????0 ℎ???????????? − � ????????????1????????????????????????1 ℎ???????????? − � ????????????2????????????????????????2 ℎ???????????? − ... − � ???????????????????????????????????????????????????????????? ℎ???????????? 2 = ∑ ???????????? ???????????? − ̂ ???????????? 0 − ̂ ???????????? ?????????????????? ?????????????????? − ̂ ???????????? 2???????????? ????????????2 − ... − ̂ ???????????? ???????????? ???????????? ???????????????????????? 2 ?????? ℎ ???????????? • This is the same as estimating the original model ???????????? ???????????? = ???????????? 0 +???????????? OLS of the transformed model multiplying the variables by 1/ ℎ ???????????? is the same as estimating the original model using WLS with weight =1 /ℎ ???????????? • In WLS, observations with a higher error variance have less weight. OLS gives each observation equal weight. Feasible Generalized Least Squares (FGLS) • If the heteroscedasticity form is not known, the Feasible Generalized Least Squares (FGLS) transforms the variables to get homoscedasticity. Here, ℎwill be estimated as � ℎ and used in the FGLS. • The heteroscedasticity form is expressed as: ???????????????????????????????????? ???????????? ???????????? =???????????? 2ℎ ???????????? =???????????? 2exp (???????????? 0 + ???????????? ???????????????????????? +⋯ +???????????? ????????????????????????????????????) • Estimate the regression model: ????????????= ???????????? 0 +???????????? Alternatively, all variables and the constant get multiplied by 1/ � ℎ???????????? and the model is estimated by OLS. ???????????????????????? � ℎ???????????? = ????????????0 � ℎ???????????? + ???????????????????????????????????????????????? � ℎ???????????? + ????????????2????????????????????????2 � ℎ???????????? + ⋯ + ???????????????????????????????????????????????????????????? � ℎ???????????? + ???????????? ???????????? � ℎ???????????? Heteroscedasticity tests example • Example: ???????????? Testing for heteroscedasticity • After the regression models for � ???????????? 2 are estimated: ???????????? 0 : ???????????? F-test for overall significance ????????????− ???????????????????????????????????? ????????????= ???????????? � ???????????? 2 2 / ???????????? ( ?????? − ???????????? � ???????????? 2 2 ) /( ????????????− ????????????− ??????) • LM-test ????????????????????????−???????????????????????? Graphs of residuals against an independent variable Residual for model for priceResidual for model for lprice (heteroscedasticity) (homoscedasticity) 14 -100 0 100 200 Residuals 1000 2000 3000 4000 size of house in square feet -1 -.5 0 .5 1 Residuals 1000 2000 3000 4000 size of house in square feet Graphs of residuals against fitted values Residual for model for priceResidual for model for lprice (heteroscedasticity) (homoscedasticity) 15 -1 -.5 0 .5 1 Residuals 5 5.5 6 6.5 Fitted values -100 0 100 200 Residuals 200 300 400 500 600 Fitted values 16 Model for price Breusch -Pagan test White test Alternative to White test VARIABLES priceuhatsq uhatsq uhatsq lotsize 0.002*** 0.202*** -1.860*** (0.001) (0.071) (0.637) sqrft 0.123*** 1.691 -2.674 (0.013) (1.464) (8.662) bdrms 13.8531,041.760 -1,982.841 (9.010) (996.381) (5,438.482) lotsizesq -0.000 (0.000) sqrftsq 0.000 (0.002) bdrmssq 289.754 (758.830) lotsizeXsqrft 0.000 (0.000) lotsizeXbdrms 0.315 (0.252) sqrftXbdrms -1.021 (1.667) pricehat -119.655** (53.317) pricehatsq 0.209*** (0.075) Constant -21.770 -5,522.795* 15,626.243 19,071.587** (29.475) (3,259.478) (11,369.411) (8,876.227) Observations 8888 88 88 R -squared 0.672 0.160 0.383 0.185 •Heteroscedasticity tests for price. • Model is estimated, then the squared residuals are regressed on the independent variables (Breusch -Pagan test), independent variables and their squares and interactions (White test), and the predicted values (Alternative White test). • The R -squared for the regressions for uhatsqare used to calculate the test statistics. • Several of the coefficients in the regressions for uhatsq are individually significant. Heteroscedasticity tests for price Breusch-Pagan test White testAlternative White test Observations ???????????? 88 8888 R- squared ???????????? � ????????????22 0.160 0.3830.185 k 392 F -stat (0.16/3)/((1-0.16) /(88- 3-1))=5.34 (0.383/9)/((1- 0.383) /(88- 9-1))=5.39 (0.185/2)/((1- 0.185) /(88- 2-1))=9.64 P- value for F- test 0.002 0.00001 0.0002 LM -stat 88*0.16=14.0988*0.383=33.73 88*0.185=16.27 P- value for LM test 0.0030.0001 0.0003 Conclusion heteroscedasticityheteroscedasticityheteroscedasticity 17 F-test for overall significance ????????????− ???????????????????????????????????????????????? = ????????????� ???????????? 2 2 / ???????????? ( ?????? − ???????????? � ???????????? 2 2 ) /(???????????? −????????????− ??????) LM -test ???????????????????????? −???????????????????????????????????????????????? = ???????????????????????? � ????????????22 ~ ???????????? ????????????2 All tests show heteroscedasticity in price. The regression for price needs correction for heteroscedasticity. 18 Model for log price Breusch -Pagan test White test Alternative to White test VARIABLES lpriceuhat1sq uhat1sq uhat1sq lotsize 0.000***0.000 -0.000 (0.000) (0.000) (0.000) sqrft 0.000***-0.000 -0.000 (0.000) (0.000) (0.000) bdrms 0.0250.020* 0.086 (0.029) (0.012) (0.072) lotsizesq 0.000 (0.000) sqrftsq 0.000 (0.000) bdrmssq -0.005 (0.010) lotsizeXsqrft 0.000 (0.000) lotsizeXbdrms -0.000 (0.000) sqrftXbdrms -0.000 (0.000) yhat -1.119 (1.280) yhatsq 0.095 (0.110) Constant 4.759***0.016 0.013 3.326 (0.094) (0.038) (0.150) (3.708) Observations 8888 88 88 R -squared 0.6220.040 0.082 0.012 •Heteroscedasticity tests for log price ( lprice). • Model is estimated, then the squared residuals are regressed on the independent variables (Breusch -Pagan test), independent variables and their squares and interactions (White test), and the predicted values (Alternative White test). • The R -squared for the regressions for uhatsqare used to calculate the test statistics. R -squared are much lower for lpricethan for price. • Only one of the coefficients in the regressions for uhatsqis individually significant. Heteroscedasticity tests for log price Breusch-Pagan test White testAlternative White test Observations ???????????? 88 8888 R- squared ???????????? � ????????????22 0.040 0.0820.012 k 392 F -stat (0.04/3)/((1-0.04) /(88 -3 -1))=1.17 (0.082/9)/((1- 0.082) /(88 -9 -1))=0.77 (0.012/2)/((1- 0.012) /(88 -2 -1))=0.53 P- value for F -test 0.32 0.640.59 LM- stat 88*0.04=3.5488*0.082=7.19 88*0.012=1.08 P- value for LM test 0.320.610.58 Conclusion homoscedasticityhomoscedasticityhomoscedasticity 19 F-test for overall significance ????????????− ???????????????????????????????????????????????? = ????????????� ???????????? 2 2 / ???????????? ( ?????? − ???????????? � ???????????? 2 2 ) /(???????????? −????????????− ??????) LM -test ???????????????????????? −???????????????????????????????????????????????? = ???????????????????????? � ????????????22 ~ ???????????? ????????????2 All tests show homoscedasticity in log price. The regression for log price does not need correction for heteroscedasticity. OLS vs OLS with robust standard errors 20 OLSOLS with robust se VARIABLES priceprice lotsize 0.002***0.002 (0.001) (0.001) sqrft 0.123***0.123*** (0.013) (0.018) bdrms 13.85313.853 (9.010) (8.479) Constant -21.770-21.770 (29.475) (37.138) Observations 8888 R-squared 0.6720.672 •The coefficients are the same for OLS and OLS with robust standard errors, but the coefficient on lotsize became insignificant. • Using robust standard errors is the easiest solution for heteroscedasticity. • This is the model for price. The model for log price has homoscedasticity so robust standard errors are not needed. WLS 21 WLS VARIABLES price lotsize 0.002*** (0.001) sqrft 0.118*** (0.014) bdrms 10.607 (8.659) Constant 4.199 (29.698) Observations 88 R-squared 0.591 WLS VARIABLES pricestar lotsizestar 0.002*** (0.001) sqrftstar 0.118***(0.014) bdrmsstar 10.607 (8.659) constantstar 4.199 (29.698) Observations 88 R- squared 0.963 • The first regression ???????????? •In the second regression, all variables are multiplied by 1/ ???????????????????????????????????????????????????????????? , and model is estimated by OLS. ???????????????????????????????????????????????????????????? / ???????????????????????????????????????????????????????????? =???????????? 0/ ???????????????????????????????????????????????????????????? +???????????? ?????????????????????????????????????????????????????????????????? ???????????????????????? / ???????????????????????????????????????????????????????????? +???????????? 2???????????????????????????????????????????????????????????? / ???????????????????????????????????????????????????????????? +???????????? 3???????????????????????? ???????????????????????? ????????????/ ???????????????????????????????????????????????????????????? +????????????/ ???????????????????????????????????????????????????????????? • The results are identical. The heteroscedasticity form is assumed to be: ???????????????????????????????????? ???????????????????????? =???????????? 2???????????????????????????????????????????????????????????? FGLS with weights vs FGLS after transformation 22 FGLS VARIABLES price lotsize 0.004*** (0.001) sqrft 0.092*** (0.015) bdrms 6.175 (8.894) Constant 45.912 (30.824) Observations 88 R-squared 0.468 FGLS VARIABLES pricestar1 lotsizestar1 0.004*** (0.001) sqrftstar1 0.092*** (0.015) bdrmsstar1 6.175 (8.894) constantstar1 45.912 (30.824) Observations 88 R- squared 0.968• FGLS when the heteroscedasticity form is unknown. • The results are identical. • The first regression ???????????? In the second regression, all variables are multiplied by 1/ � ℎ????????????, and model is estimated by OLS. ???????????? ???????????????????????????????????????????????? / � ℎ???????????? = ???????????? 0/ � ℎ????????????+ ???????????? ?????????????????????????????????????????????????????????????????? ???????????????????????? / � ℎ????????????+ ???????????? 2???????????????????????????????????????????????????????????? / � ℎ????????????+ ???????????? 3???????????????????????? ???????????????????????? ????????????/ � ℎ????????????+ ????????????/ � ℎ???????????? OLS, OLS with robust standard errors, WLS, and FGLS 23 OLSOLS with robust se WLS FGLS VARIABLES pricepricepriceprice lotsize 0.002***0.0020.002*** 0.004*** (0.001) (0.001)(0.001)(0.001) sqrft 0.123***0.123***0.118***0.092*** (0.013) (0.018)(0.014)(0.015) bdrms 13.85313.85310.607 6.175 (9.010) (8.479)(8.659)(8.894) Constant -21.770-21.770 4.19945.912 (29.475) (37.138)(29.698)(30.824) Observations 88888888 R-squared 0.6720.6720.5910.468 •Robust standard errors do not change the coefficients, only the standard errors and significance. • The coefficients are different for OLS as compared to WLS and FGLS because of the use of weights. • Besides the loss of significance of one coefficient, the results are similar across all models, after correcting for heteroscedasticity. Review questions • Define heteroscedasticity. When heteroscedasticity is present, are the coefficients biased? Is the variance for the coefficients correct? Are the t- tests and F -tests valid? • Describe the 3 tests that are used to test for heteroscedasticity. Describe the procedures for each of these tests. What is the difference between them? • If the heteroscedasticity form is known, then what procedure is used? Describe the Weighted Least Squares procedure. What weights are used? • If the heteroscedasticity form is not known, then what procedure is used? Describe the Feasible Generalized Least Squares procedure. What weights are used? • Why is it equivalent to multiply each variable by 1/ ℎ , but the weight is 1/ℎ ? 24