HCNN_2024_2-31-67.pdf
Document Details
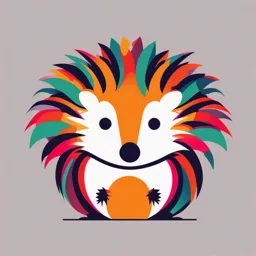
Uploaded by DaringRadon4272
Tags
Full Transcript
First, top layers of deeper CNNs predicted IT neural responses at the late phases (150–250 ms) significantly more than ‘regular-deep’ models such as AlexNet This observation suggests that deeper CNNs might indeed be approximating ‘unrolled’ versions of the recurrent circuits of the ventral stream....
First, top layers of deeper CNNs predicted IT neural responses at the late phases (150–250 ms) significantly more than ‘regular-deep’ models such as AlexNet This observation suggests that deeper CNNs might indeed be approximating ‘unrolled’ versions of the recurrent circuits of the ventral stream. Second, it was observed a reduced number of challenge images for deeper CNNs. Third, it was found that the images that remain unsolved by these deeper CNNs (that is, challenge images for these models) showed even longer OSTs in the IT cortex than the original full set of challenge images. This suggests that the newer, deeper CNNs have implicitly, but only partially, approximated—in a feedforward network—some of the computations that the ventral stream implements recurrently to solve some of the challenge images. 30 CORnet (2018), is a four-layered recurrent neural network model The top layer of CORnet (comparable to IT) has within-area recurrent connections (with shared weights). The model implements five time-steps (pass 1 to pass 5). CORnet had higher IT predictivity for the late-phase of IT responses. Pass 1 and pass 2 of the network are better predictors of the early time bins ( relevant for control images). Additionally, late passes (especially pass 4) are better at predicting late (170–200 ms) phases of IT responses (crucial for challenge images). Taken together, these results further argue for recurrent computations in the ventral stream. 31 32 Kubilius, J., et al. CORnet: modeling the neural mechanisms of core object recognition. biorXiv (2018) 33 These data do not yet explain the exact nature of the computational problem solved by recurrent circuits during core object recognition. Deeper CNNs such as inception-v3, v431, and ResNet-50, which introduce more nonlinear transformations to the image pixels compared to shallower networks such as AlexNet, are better models of the behaviorally critical late phase of IT responses. What computer vision has achieved by stacking more layers into the CNNs is a partial approximation of something that is more efficiently built into the primate brain architecture in the form of recurrent circuits. During core object recognition, recurrent computations act as additional nonlinear transformations of the initial feedforward. 34 Image completion and RNN 35 Recurrent computations for visual pattern completion In perception, pattern completion enables recognition of poorly visible or occluded objects. Tang et al. (2018) combined psychophysics, physiology, and computational models to test the hypothesis that pattern completion is implemented by recurrent computations. Tang et al., PNAS, 2018 36 The visual system is capable of making inferences even when only 10–20% of the object is visible. 37 Unmasked Masked Partial Occluded Novel In backward masking, processing of a visual stimulus is interrupted by the presentation of a second stimulus, the mask. 38 Tang et al., PNAS, 2018 Visual categorization of objects is robust to limited visibility Subjects robustly recognized partial and novel objects across a wide range of visibility levels despite the limited information provided. For whole objects and without a mask, behavioral performance was at 100% Backward masking disrupts recognition of partially visible objects. When an image is rapidly followed by a spatially overlapping mask, this interrupts any additional, presumably recurrent, processing of the image. 39 Tang et al., PNAS, 2018 40 Tang et al., PNAS, 2018 Standard feed-forward models are not robust to occlusion Performance of feed-forward models (AlexNet an 8-layer CNN trained via back-propagation on ImageNet) was evaluated using the same 325 objects (13,000 trials). Feed-forward CNN were comparable to humans at full visibility, hover performance declined at limited visibility. There was a modest but significant correlation at the object-by-object level between the latency of neural response and computational distance of each partial object to its whole object category mean for AlexNet pool5 and fc7 features. 41 Tang et al., PNAS, 2018 Recurrent Neural Networks improve recognition of partially visible objects Attractor networks can perform pattern completion. In the Hopfield network (1982), units are connected in an all-to-all fashion with weights defining fixed attractor points dictated by the whole objects to be represented. Images that are pushed farther away by limited visibility would require more processing time to converge to the appropriate attractor, consistent with the behavioral and physiological observations. AlexNet architecture was added with recurrent connections to the fc7 layer. with one attractor for each whole object. The RNNh model demonstrated a significant improvement over the standard AlexNet 42 Attractor networks can perform pattern completion. 43 Tang et al., PNAS, 2018 Temporal evolution of the feature representation for RNNh as visualized with stochastic neighborhood embedding. The representation of whole objects (open circles) showed a clear separation among categories, but partial objects from different categories (filled circles) were more similar to each other than to their whole object counterparts. Over time, the representation of partial objects approaches the correct category in the clusters of whole images. 44 Correlation (Corr.) in the classification of each partially object between the RNNh and humans. Over time, the recurrent model–human correlation increased toward the human–human upper bound. The RNNh model’s performance and correlation with humans saturates at around 10–20 time steps. A combination of feed-forward signals and recurrent computations is consistent with the physiological responses to heavily occluded objects arising at around 200 ms. 45 Tang et al., PNAS, 2018 Backward masking impairs RNN model performance If backward masking impairs performance by interrupting processing, this should be reproduced in the RNNh model. Presenting the mask reduced RNN performance from 58 ± 2% (SOA = 256 time steps) to 37 ± 2% (SOA = two time steps). 46 Tang et al., PNAS, 2018 Making inferences from partial information constitutes a critical aspect of cognition. During visual perception, pattern completion enables recognition of poorly visible or occluded objects. First, subjects robustly recognized objects even when they were rendered