GFQR 1026: Big Data Lecture 1 PDF
Document Details
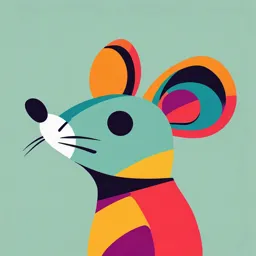
Uploaded by UsableLeibniz
Hong Kong Baptist University
Tags
Summary
This is a lecture on big data, covering its definition, volume, velocity, variety, and flow. It includes examples of big data applications and technologies.
Full Transcript
GFQR 1026: Big Data in “X” Lecture 1 : What is Big Data & Analytics Flow for Big Data? Page 1 Lecture 1: Outline What is Big Data? Big Data around you Global Trends of Big Data Types of Big Data...
GFQR 1026: Big Data in “X” Lecture 1 : What is Big Data & Analytics Flow for Big Data? Page 1 Lecture 1: Outline What is Big Data? Big Data around you Global Trends of Big Data Types of Big Data Analytic Flow for Big Data 2 2 Page What is Big Data? 3 3 Page What is Big Data? Collections of datasets whose volume, velocity, or variety is so large that it is difficult to store, manage, process, and analyze the data using traditional databases and data processing tools. (Bahga & Madisetti, 2016) 4 4 Page Three Vs The concept gained momentum in the early 2000s when industry analyst Doug Laney articulated the now-mainstream definition of big data as the three Vs: 5 5 Page Three Vs: Volume Organizations collect data from a variety of sources (e.g. business transactions, social media and information from sensor or machine-to-machine data) Typically, the term big data is used for massive scale data that is difficult to store, manage and process using traditional databases and data processing architectures – There is no fixed threshold for the volume of data to be considered as big data 6 6 Page Three Vs: Velocity Refers to how fast the data is generated Data generated by certain sources can arrive at very high velocities (e.g. social media data or sensor data) – High velocity of data results in the volume of data accumulated to become very large, in short span of time Some applications can have strict deadlines for data analysis (e.g. online fraud detection) and the data needs to be analyzed in real-time 7 7 Page Three Vs: Variety Refers to the types of the data Big data comes in different forms such as structured, unstructured or semi-structured Big data systems need to be flexible enough to handle such variety of data types 8 8 Page Three Vs: Variety Structured Data – Data that is located in a fixed field within a defined record or file – e.g. Point of Sales data, Financial data, student data Unstructured and Semi-structured Data – Represents all the data that cannot be so easily slotted into columns, rows and fields – It is difficult to analyze using traditional computer programs – e.g. Photos and graphic images, videos, websites, text files or documents such as email, PDF, blogs, social media posts, etc, and Powerpoint presentations 9 9 Page Three Vs: Variety 10 10 Page Additional Vs: Veracity / Validity Refers to how accurate is the data Data-driven applications can reap the benefits of big data only when the data is meaningful and accurate Cleansing of data is very important so that incorrect and faulty data can be filtered out 11 11 Page Additional Vs: Value Refers to the usefulness of data for the intended purpose Remember: The end goal of any big data analytics system is to extract value from the data More Vs will be coming…. 12 12 Page Let’s think about….. How do you use your mobile phone and How many – instant text messages do you send every day? – emails do you send every day? – photos do you take every day? – Facebook/Instagram posts you make every day? – “likes” you give in social media every day? – search you make on Google every day? All activities are contributing part of the Big Data! Page 13 How much data is generated every minute? What happens every minute? Americans use huge volume of internet data There are 231,000,000 emails sent There are 16,200,000 texts sent There are 625,000,000 videos watched on TikTok 14 14 Page Examples of Big Data Facebook generated 30+ petabytes of data per day 230+ millions of tweets are created every day. YouTube users upload 48 hours of new video every minute of the day. 294 billion emails are sent every day. Page 15 Examples of Big Data Internet of Things (IoT) – The Network of physical objects – devices, vehicles, buildings etc with electronics, software and sensors enable them to collect and exchange data via Internet – By the end of 2020, have 9.7 billion IoT devices. By 2022, number of IoT devices is estimated to reach 14.4 billion – smart TVs, Wearables like Apple watch, smart car(i.e.Tesla), smart air conditioner (Controlled by Apple HomeKit) – The total volume of data generated by IoT will reach 600 ZB per year by 2020 Page 16 Internet of Things - Number of Connected Devices Worldwide 2022-2033 Source: https://www.statista.com/statistics/1183457/iot-connected-devices- Page 17 worldwide/ Who have a lot of Big Data? …………………….. etc Page 18 How big is Zettabyte? Let’s have an idea how big is zettabyte One page One song One movie 6 million 55 storeys Data Data NSA of text books of DVD up to in data center 2003 2011 1.8 30KB 5 MB 5 GB 1 TB 1 PB 1 YB ZB 5 EB Byte Kilobyte Megabyte Gigabyte Terabyte Petabyte Exabyte Zettabyte Yottabyte KB MB GB TB PB EB ZB YB 1000 bytes 1000 KB 1000 MB 1000 GB 1000 TB 1000 PB 1000 ZB 1000YB 40 ZB = adding every single grain of sand on the planet multiplied by 75 Page 19 Size of the Global Datasphere from 2010 to 2025 ~175 zettabytes of data will be created globally in 2025 !!! Source: https://www-statista-com.lib- ezproxy.hkbu.edu.hk/statistics/871513/worldwide-data-created/ 20 20 Page Big Data is getting bigger? Global internet penetration sits at 66.2% in 2024 More data after we have the Internet !!! 21 21 Page Big Data is getting bigger during the pandemic period? 22 22 Page Domain Specific Examples of Big Data Web Healthcare – Web Analytics – Epidemiological – Performance Monitoring Surveillance – Ad Targeting & Analytics – Detecting Claim – Content Recommendation Anomalies – Real-time health Financial monitoring – Credit Risk Monitoring Internet of Things – Fraud Detection – Intrusion Detection – Smart Parking 23 23 Page Domain Specific Examples of Big Data Environment Industry – Weather Monitoring – Machine Diagnosis & – Forest Fire Detection Prognosis – Water Quality Monitoring – Production Planning & Control Logistics & Retail – Inventory Management Transportation – Customer – Real-time Fleet Tracking Recommendations – Shipment Monitoring – Store Layout – Route Generation & Optimization Scheduling 24 24 Page Datification: The new forms of Data Data is now being mined from: – Our activities – Our conversations – Photos and videos – Sensors – The Internet of Things 25 25 Page Analytics Flow for Big Data 1. Data Collection 2. Data Preparation 3. Analysis Types 4. Analytics Modes 5. Visualizations 26 26 Page 1. Data Collection Seven main ways to collect data: – Created data: Data that is created and captured manually, such as employee and customer surveys, focus groups. (Structured) – Provoked data: e.g. product review or rating. (Mostly structured) 27 27 Page 1. Data Collection – Transaction data: e.g. data generated every time a customer buys something online or in store. (Structure) – Compiled data: e.g. credit report which is compiled from many different sources outside the company. (Structured) 28 28 Page 1. Data Collection – Experimental data: usually a combination of created and transactional data. e.g. design an experiment and use a focus group (created), and then observe the data that is created (transaction). (Structured) – Captured data: e.g. google searches or GPS data at your phone. (Mostly unstructured) – User-generated data: e.g. facebook post, tweets, and videos posted on YouTube. (Unstructured) 29 29 Page 1. Data Collection Collect and ingest data into a big data stack The choice of tools and frameworks depends on the source of data and the type of data being ingested – e.g. messaging queues, source-sink connectors, database connectors, and custom connectors 30 30 Page 2. Data Preparation Involves various tasks, such as: – Data cleansing: detects and resolves issues such as corrupt records, records with missing values, records with bad formatting – Data wrangling or munging: deals with transforming the data from one raw format to another (e.g. records from different sources may come across inconsistencies in the field separators) to make it into one consistent format – De-duplication: Remove records which are duplicated 31 31 Page 2. Data Preparation Involves various tasks, such as: – Normalization: when data from different sources uses different units or scales or have different abbreviations for the same thing (e.g. weather data in Celsius scale & Fahrenheit scale) – Sampling and Filtering: can be useful when we want to process only the data that meets certain criteria or to reject bad records with incorrect or out-of-range values 32 32 Page 3. Analysis Types To determine the analysis type for the application Possible options: – Basic Statistics, Regression, Recommendation, Dimensionality Reduction, Graph Analytics, Classification, Clustering, Time Series Analysis, Text Analysis, Pattern Mining… 33 33 Page 4. Analytics Modes Choose one: Batch, Real-time, or Interactive The choice of the mode depends on the requirements of the application – If your application demands results to be updated after short intervals of time (say every few seconds), then real-time analytics mode is chosen. – If your application only requires the results to be generated and updated on larger timescales (say daily or monthly), then batch mode can be used. 34 34 Page 4. Analytics Modes – If your application demands flexibility to query data on demand, then the interactive mode is useful. After you make a choice of the analysis type and analytics mode, you can determine the data processing methods that can be used. – e.g. for basic statistics as the analysis type and the batch analytics mode, MapReduce can be a good choice; for regression analysis as the analysis type and real- time analytics mode, the Stream Processing Method is a good choice 35 35 Page 5. Visualizations Static – it is used when you have the analysis results stored in a serving database and you simply want to display the results. Dynamic – if your application demands the results to update regularly, then you would require dynamic visualizations (e.g. live widgets, plots, or gauges). Interactive – if you want your application to accept inputs from the user and display the results then you would require interactive ones. 36 36 Page Big Data around you ! To be continued…. 37 37 Page Lecture 1: Summary What is Big Data? Big Data around you Global Trends of Big Data Types of Big Data Analytic Flow for Big Data 38 38 Page References 1. Why Big Data Keeps Getting Bigger (2019). Retrieved from https://www.visualcapitalist.com/big-data-keeps-getting-bigger/ on September 1, 2022. 2. Number of smartphone users in Hong Kong 2015-2020 with forecast until 2026 (2021). Retrieved from https://www-statista-com.lib- ezproxy.hkbu.edu.hk/statistics/494594/smartphone-users-in-hong-kong/ on September 1, 2022. 3. What happens in an internet minute: 90+ fascinating online stats (2023). Retrieved from https://localiq.com/blog/what-happens-in-an-internet-minute/ on August 21, 2024. 4. How much Data is collected every Minute of the Day (2019). Retrieved from https://www.forbes.com/sites/nicolemartin1/2019/08/07/how-much-data-is- collected-every-minute-of-the-day/#86901a23d66f on September 1, 2022. 5. Big Data Tutorial: All You Need To Know About Big Data! (2024). Retrieved from https://www.edureka.co/blog/big-data-tutorial on August 21, 2024. 6. With Internet of Things and Big Data, 92% of Everything We Do Will Be in The Cloud (2016). Retrieved from https://www.forbes.com/sites/joemckendrick/2016/11/13/with- internet-of-things-and-big-data-92-of-everything-we-do-will-be-in-the-cloud/#479cb6114ed5 on September 1, 2022. 39 39 Page References 7. Number of Internet of Things (IoT) connections worldwide from 2022 to 2023, with forecasts from 2024 to 2033 (2024). Retrieved from https://www.statista.com/statistics/1183457/iot-connected-devices-worldwide/ on August 21, 2024. 8. Semantic Search: What It is & Why It Matters for SEO Today (2021). Retrieved from https://www.searchenginejournal.com/semantic-search-seo/264037/ on September 1, 2022. 9. Information Created Globally 2010-2015 (2019). Retrieved from https://www-statista- com.lib-ezproxy.hkbu.edu.hk/statistics/871513/worldwide-data-created/ on September 1, 2022. 10. Bahga, Arshdeep, and Madisetti, Vijay (2016), Big Data Science & Analytics: A Hands- On Approach, Arshdeep Bahga & Vijay Madisetti (pp.26-38). 11. Marr, Bernard (2015), Big Data: using Smart Big Data, Analytics and Metrics to make better decisions and improve performance, Wiley. (pp. 59-101). 12. Foster, A.L, and Brown, D.R. (2015), Big Data and its Impact on Society and Industry and the Growing Need for Big Data. International Advanced Research Journal in Science, Engineering and Technology, Vol. 2, Issue 12, 2015. Retrieved from https://www.iarjset.com/upload/2015/december-15/IARJSET%2032.pdf 40 40 Page Image Credits [Slide 2 & 43] https://www.kissclipart.com/instruct-clipart-clip-art-women-clip-art-zameoc/ [Slide 3] https://saultonline.com/2018/01/frog-or-inukshuk-hmmmm/ [Slide 5] https://www.rd-alliance.org/group/big-data-ig-data-development-ig/wiki/big-data-definition-importance-examples-tools [Slide 9] https://www.w3schools.in/data-structures-tutorial/intro/ [Slide 10] https://lawtomated.com/structured-data-vs-unstructured-data-what-are-they-and-why-care/ [Slide 13] https://www.irishtimes.com/news/science/study-shows-high-levels-of-mobile-phone-radiation-linked-to-tumors-in-rats-1.3379144 [Slide 15] https://npaworldwide.com/blog/2012/09/13/whatsapp-can-be-a-useful-tool-for-global-recruiting/ [Slide 16] https://localiq.com/blog/what-happens-in-an-internet-minute/ [Slide 17] https://www.dreamstime.com/social-media-networking-sign-logos-round-icons-vector-file-mostly-usable-signs-available-image107412123 [Slide 18] https://www.flickr.com/photos/134647712@N07/34817827783 [Slide 19] https://www.softwaretestinghelp.com/iot-devices/ [Slide 19] https://www.statista.com/statistics/471264/iot-number-of-connected-devices-worldwide/ [Slide 20] https://www.northernrockies.ca/EN/main/city/surplus.html [Slide 20] https://en.wikipedia.org/wiki/Censorship_of_Facebook [Slide 20] https://news.microsoft.com/en-gb/recent-news/ [Slide 20] https://multichannelmerchant.com/news/alibaba-considering-distribution-center-detroit/ [Slide 20] https://www.amazon.com/ [Slide 20] https://rapidtwitterfollowers.com/tweaking-the-likes-of-twitter-for-free/ [Slide 20] http://pngimg.com/imgs/logos/youtube/ [Slide 20] https://www.itpro.co.uk/strategy/28507/verizon-yahoo-acquisition-expected-to-close-in-june [Slide 20, 21&22] https://www.thesearchagency.com/2013/11/google-rolls-out-in-market-buyers-segment-on-gdn/ [Slide 21] https://zh.wikipedia.org/zh-tw/PageRank [Slide 24] https://www-statista-com.lib-ezproxy.hkbu.edu.hk/statistics/871513/worldwide-data-created/ [Slide 26] https://www.visualcapitalist.com/big-data-keeps-getting-bigger/ [Slide 26 & 27] https://datareportal.com/reports/digital-2020-april-global-statshot [Slide 28] https://www.websolutions.com/blog/9-web-analytics-metrics-you-need-to-know/ [Slide 28] https://www.zoho.com/analytics/financial-analytics.html [Slide 28] http://amipsyche.com/what-are-your-vital-signs/ [Slide 28] https://placetech.net/analysis/iot-grows-in-social-housing/ [Slide 29] https://www.baronweather.com/industries/insurance/claims/weather-monitoring/ [Slide 29] https://eu.clipdealer.com/vector/media/A:139372703 [Slide 30] https://semielectronics.com/sensors-lifeblood-internet-things/ [Slide 31] https://edkentmedia.com/difference-web-marketing-analytics/ [Slide 32] https://www.yotpo.com/glossary/product-ratings/ [Slide 33] https://time.com/nextadvisor/credit-cards/credit-report-vs-credit-score/ [Slide 35] https://miro.medium.com/max/700/1*MTWLp_V79poGSZ9W1bHGZA.png [Slide 37] https://www.qresearchsoftware.com/market-research-guide-data-preparation [Slide 32 - 41] https://www.qresearchsoftware.com/ [Slide 42] https://www.edureka.co/blog/big-data-applications-revolutionizing-various-domains/ 41 41 Page