From association to causation Binder.pdf
Document Details
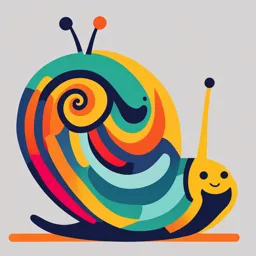
Uploaded by AmpleJudgment
Tags
Full Transcript
From Association to Causation Presentation By: Ann M Vuong, DrPH, MPH How do we establish cause and effect? How do we go from a proven relationship (association) to consider it a causal relationship? 2 Association versus Causation Association An identifiable relationship between exposure and dis...
From Association to Causation Presentation By: Ann M Vuong, DrPH, MPH How do we establish cause and effect? How do we go from a proven relationship (association) to consider it a causal relationship? 2 Association versus Causation Association An identifiable relationship between exposure and disease Implies that exposure causes disease Exposures associated with a difference in disease risk are often called risk factors Causation Implies that there is a true mechanism that leads from exposure to disease 3 The biggest problem is to establish causation The other problem is to explain causation after it occurs 4 Causation Animal Studies Can randomly assign animals to exposures In Vitro Studies Cell culture studies or other experiments Human Experiments Basic epidemiology can be used to understand the exposure-outcome relationship Unethical to expose people to a substance you believe causes harm 5 Causation Do risk factors have to be causal to be useful? Yes If we know what is causing disease, we can design treatments to cure or interventions to prevent No If a factor is predictive, it might give us just as much information as a truly causal factor, but be easier to measure or interpret 6 Types of Causal Relationship Direct Factor Indirect Factor Disease Step 1 Step 2 Disease 7 Components of Causation Necessary Cause Precedes the disease If the cause is absent, the disease cannot occur Sufficient Cause Precedes the disease If the cause is present, the disease always occurs 8 Types of Causal Relationship Necessary and Sufficient Necessary, but not Sufficient Sufficient, but not Necessary Neither Necessary nor Sufficient 9 Necessary and Sufficient Factor Disease Without the factor, the disease never happens With the factor, the disease always happens Rarely occurs 10 Necessary, but Not Sufficient Factor A Factor B Disease All factors required to produce the disease, often in a specified temporal sequence Multifactorial, multistage, “multi-hit” Factor C 11 Sufficient, but Not Necessary Factor A OR Factor B Disease Each factor, acting alone, can independently produce the disease OR Factor C 12 Neither Necessary Nor Sufficient Factor A Factor B OR Factor C Factor D OR Factor E Disease Factor F No single factor will cause disease alone (no sufficient causes), and not all are necessary for disease to occur 13 How do we assess whether a risk factor is indeed causal? 14 Hill’s Criteria for Causation Temporal Relationship Assessing Causation Strength of Association Dose-response Replication of Findings Biological Plausibility Consideration of Alternate Explanations Cessation of Exposure Consistency with Other Knowledge Specificity of Association Establishes causation 15 Hill’s Criteria for Causation Temporal Relationship Temporal Relationship Strength of Association Dose-response Replication of Findings Biological Plausibility Consideration of Alternate Explanations Cessation of Exposure Consistency with Other Knowledge Specificity of Association Factor must come before the outcome Important to consider latency of disease Latency for developing lung cancer is 15-20 years Exposure to asbestos last year is likely not the cause But, don’t assign causality simply because they exist beforehand Establishes causation 16 Hill’s Criteria for Causation Temporal Relationship Strength of Association Strength of Association Dose-response Replication of Findings Biological Plausibility Consideration of Alternate Explanations Cessation of Exposure Consistency with Other Knowledge Specificity of Association Usually measured by relative risk The larger the effect size, the more convincing the relationship Not all strong effects are causal Large-scale genetic studies sometimes have extraordinarily large effects that cannot be replicated Weak associations should not necessarily be dismissed Establishes causation Dose Response Hill’s Criteria for Causation Temporal Relationship Strength of Association Dose-response Replication of Findings Biological Plausibility Consideration of Alternate Explanations Cessation of Exposure Consistency with Other Knowledge Specificity of Association The more exposure, the more disease Amount cigarette smoking by number of cigarettes, years smoked The absence of a monotonic dose-response relationship does not necessarily rule out a causal relationship Threshold, U-shaped, J-shaped Establishes causation Hill’s Criteria for Causation Temporal Relationship Replication of Findings Strength of Association Dose-response Replication of Findings Biological Plausibility Consideration of Alternate Explanations Cessation of Exposure Consistency with Other Knowledge Specificity of Association Can results be repeated with another study population at another time? Expect it to be consistent across populations or within subgroups, unless there is reason to think there’s a difference Does it appear in several subgroups? Do the series of studies show consistency in findings? Establishes causation Hill’s Criteria for Causation Temporal Relationship Biological Plausibility Strength of Association Dose-response Replication of Findings Biological Plausibility Consideration of Alternate Explanations Cessation of Exposure Consistency with Other Knowledge Specificity of Association Does the association fit with what we know about the underlying biology? What we know can change over time Sometimes epidemiology can identify causal relationships in the absence of biological plausibility John Snow figured out that the source was the pump water, even though current wisdom said cholera was due to vapors Establishes causation Hill’s Criteria for Causation Temporal Relationship Strength of Association Dose-response Replication of Findings Biological Plausibility Consideration of Alternate Explanations Cessation of Exposure Consistency with Other Knowledge Specificity of Association Consideration of Alternate Explanations If knowledge exists, then rule out or make sure studies took into account: Confounding Bias Poor study design Data errors Other potential explanations Establishes causation Hill’s Criteria for Causation Temporal Relationship Cessation of Exposure Strength of Association Dose-response Replication of Findings Biological Plausibility If remove (or reduce) exposure, does the disease go away (or become less common) Consideration of Alternate Explanations Cessation of Exposure Consistency with Other Knowledge Specificity of Association Establishes causation Hill’s Criteria for Causation Temporal Relationship Strength of Association Dose-response Replication of Findings Biological Plausibility Consideration of Alternate Explanations Cessation of Exposure Consistency with Other Knowledge Specificity of Association Consistency with other Knowledge If we have knowledge regarding a risk factor, then this comes into play Can bring in many pieces of evidence to bear on this questions Ecological Basic science – animal models, cell lines Previous epidemiological studies Clinical observations Trends over time Establishes causation Hill’s Criteria for Causation Temporal Relationship Strength of Association Dose-response Replication of Findings Specificity of Association If an exposure is associated with only one disease, it’s specific to that disease and may be more likely to be causal Biological Plausibility Consideration of Alternate Explanations Cessation of Exposure Consistency with Other Knowledge Specificity of Association Fits for infectious agents, but not necessarily with many risk factors for chronic illness Many causal factors with multiple effects Cigarette smoking Absence of specificity should not rule out causality Establishes causation How Do We Measure Cause before effect Relative Risk or Odds Ratio Dose Response Curve MetaAnalysis Disappearance Curve Temporality Strength of Association Dose Response Replication Cessation 25 Web of Causation CAUSAL AGENTS GENETIC FACTORS ENVIRONMENT PROTECTIVE FACTORS OTHER FACTORS 26 Population-based causation not equal to cause for an individual case Epidemiology works with population to infer causal pathway, but not able to describe that for an individual 27 Association is the starting point It may never be possible to prove causation in some complex diseases However, epidemiology can identify preventable risk factors Association to Causation In epidemiology, when we design our studies and run our analysis, the best thing that we can do is to establish whether an association exists between our exposure of interest and our outcome. But how do we establish cause and e;ect? Even if a statistically significant relationship may exist, how do we know that it is a real relationship? How do we move from an association between our exposure and outcome to a causal relationship? An association does imply that exposure causes disease, but that's it. Implying doesn't really mean anything. It's merely an identifiable relationship between exposure and disease. Exposures that are often studied in EPI are risk factors for disease develop. Because dictation, this term implies that there is a true biological mechanism for disease development after exposure occurs. The hardest thing to do is to actually establish causation. Then you still have to explain causation. That is why many times when we're talking about epidemiology, I'm trying to reiterate that in epidemiology, the only thing that we can do is to establish whether an association exists between exposure and outcome. We cannot say that exposure causes disease because we are observational in nature. However, there are a number of studies that canmore easily establish causation. These studies include animal models, toxicology studies where exposure can randomly be assigned. You have your control group and you can assign varying levels of exposure or dose. This way you can answer the questions whether exposure caused the disease. You can't really do this in human size for ethical reasons, but you can do it on a cellular level with individual cell. And since we can't expose people to something we think will cause harm, we need to rely on epidemiology to understand the exposure outcome relationship. One of the aims of epidemiology is to determine risk factors for developing disease. But do the risk factors have to be causal to be useful to us? Well, if we know that it's causal, we can use this information to design treatments to cure the disease. We can even design intervention programs to help prevent disease development. But that's not to say that the risk factors, which are not causal, are not helpful. Risk factors that are predictive can still give us a lot of information, and sometimes it's much more easily interpretable or measured. There are two types of causal relationships. The first one is called a direct causal relationship. They implies to intermediates because the risk factor is approximate cause of the outcome or disease. And the indirect causal relationship. There's a chain of events that goes from factor exposure of interest to the disease. This is the most common causal relationship, while there are other steps that must take place before disease development occur. When we assessed causation, we refer to two components, necessary causes and su;icient causes. They both occur prior to disease onset or di;erent with regard to their necessity for disease develop. The necessary cause is really important and that if it's not there, the disease will not occur. Is su;icient cost isn't required like the necessary cost, but if it's present the disease will likely occur. From these we see four types of causal relationships, one that is necessary and su;icient, the second that is necessary but not su;icient, the third being su;icient but not necessary, and the last being neither necessary nor su;icient. And unnecessary su;icient relationship. You see a direct one going from factor to disease. So if the factor isn't there then the disease doesn't fall. If the factor is there, then the disease will occur. Remember that these types of relationships are very rare, though it does happen. An example would be genetic factors and development of sickle cell anemia or Down syndrome. Where it's necessary but not su;icient, the factors that are required for disease development are often appearing in a specified temporal sequence. It's more of a multifactorial multi stage disease development where all the factors need to be present. An example would be cancer, which requires an initiation, the latent. And a promoter. That is su;icient but not necessary. All factors can cause the disease and these relationships are independent of each. They do not need to be in succession like the previous relationship. The last relationship is neither necessary nor su;icient. Most of the time, risk factors combine in ways we know little about to produce disease, so no single one can cause disease alone. But not all are necessary for disease to develop. This is the most often the case for chronic diseases. Necessary and su;icient causes provide a theoretical framework for causation of diseases, but the question is how do we assess whether a risk factor is even causal? One way to assess causation is to use Austin Bradford Hills Criteria for causation, which he published in 1965 For chronic diseases. There are 9 criteria that he used to assess causality. Of the nine, only 5 established causation. The Russians explain causation. So if you take note, there is a Red Star next to five criteria listed on the left hand side and these are the criteria that actually establish causation. The ones that do not have a restar are ones that explain causation. So when you look at the criteria on the left hand side, there are some that look very familiar. Because we've talked about it throughout the course. The first one is temporality. We talked about temporality a lot so far. Exposure must precede disease. It's important to consider latency of disease development when thinking of exposure as well. For those diseases with a long latency period, exposures have occurred more recently are unlikely to be the source. Remember that just because exposure happened before disease development. It doesn't mean that it caused it. Thank you. Association is also important to look at. What is your measure of association. Let's say it's a relative risk if you have a cohort study. Four studies are able to establish temporality, while case controls are not. If your relative risk is a 1.5, it isn't as strong as a relative risk of 2.5. The larger the e;ect size, the more reason to believe that a causal relationship is actually there. But remember that not all strong e;ects are causal. If the study reports an excessively large relative risk, let's say a 30. But it doesn't mean for certain that there is a causal relationship. It could be because the sample size was small and the estimate is imprecise. And the association is small. It doesn't mean that the relationship isn't real. Think of the associations for active smoking or environmental tobacco smoke, or ETS. ETS will have a much smaller measure of association feared after smoking, but that doesn't mean that ETF isn't a problem and isn't a concern in the development of several adverse health conditions. Those response is another criteria that establishes causation that we've talked about, right. With increasing levels exposures there's increasing odds or increasing risk for disease development. So if you're looking at cigarette smoking as your exposure of interest, you can look at your in categories of exposure whether it be number of cigarettes or years of smoking. And then you can see what the relationship is with let's say, if your outcome is lung cancer. Now remember that just because there is no relationship in terms of a linear dose response between exposure outcome doesn't mean that there's no causal relationship. It does not need to be a monotonic dose response. It could be a threshold e;ect where. Up to a certain point of exposures, there is no increase of risk for a certain outcome, but after it reaches a certain point of level of exposure, the risk dramatically increases. The relationship can have a U shape distribution where the lower and upper ends of exposure are an increased risk for disease development, whereas those who have exposures within the middle range. Have lower risk or it could have a J shape which subdivision where the lower end slightly has an increased risk and those who are on the upper end have much higher risk of disease ability, so does not always have to be a linear dose response. With relationship is really caused open. There should be consistency between studies and this is what you guys are assessing in your projects. You have one research question you're comparing di;erent studies have been published determined whether the bulk of the findings indicate that there is indeed an association between your exposure and your outcome of interest. What you're doing is essentially performing a very mini literature review. Because you only need to select these studies, whereas in a real literature review or a meta analysis you would examine all the studies have been published thus far to see what the evidence supports. If there really is a causal relationship and the findings should be reported in di;erent populations at di;erent time periods, it will be present in various subgroups, meaning both genders and various races. Increase and so forth. Our logical possibility is the criteria, Underhill that does not establish causation. As you can see, there's no start. But it helps explain conversation. It's the underlying biological mechanism involved. There's the studies finding align with what we know happens at the biological level. At the cellular level. We draw from what we know has been published in cellular studies in animal model. That is why when you read the discussion section of most EPI papers. You see, the findings are reported and after that most of the investigators will support their findings with evidence from animal models. They also explain why they found what they found. But sometimes we may find something that goes against what we know, and that's OK. In several of my papers, I may find some conflicting results from what has been published so far, and we don't necessarily know why that is. Remember our lecture on EPI history and how several prominent figures reported some significant finds? But it went against everything that was known at the time. John Snow, the cholera epidemic. He didn't know exactly what was happening under the surface, but he was right. Lynch and his discovery of treating those out at sea with citrus fruit to treat scurvy, He didn't know what vitamin C. Because we found a true causal relationship. Now, that's not to say that if the study found something that goes against what we found that it found something groundbreaking and it's right. It could just be a poorly designed study and they found something that was di;erent and it's wrong. So you have to assess the study. You have to critically examine it. Was there confounding that was not taken into account? Is there any bias that may have resulted in the findings? Going away or toward the null? Was the study designed itself to support. Did they include people that they should have excluded? Maybe their recruitment strategy was inappropriate. Maybe they analyze their data in a way that resulted in their inaccurate findings where their data errors. Think of everything that could be involved and why they found what they found. The last criteria under Hills criteria that establishes causation is succession of exposure, which just means that if we take out the exposure, does the disease go away or become less common? Think back to John Snow again. He didn't know for certain that the water was a source of the cholera outbreak, but he decided to remove the exposure by. Removing the water handle from the Broad Street pump. And then he saw the numbers of collar cases decrease. Consistency with other knowledge. This is a criteria that explains causation, does not establish it. Do the findings align with what we know so far? We can further support and explain the causation if there are ecological studies that found what we found is the basic science supports it, if others at these studies support it, and so on. The last criteria is one that explains causation. It refers to specificity of the association. How specific is the exposure to the disease? If the exposure is associated with only one disease, then it's likely to be causal. This fits well with pathogens for infectious diseases, but falls apart when you think about exposures or risk factors for many chronic disease. So we think of risk factors for chronic diseases, let's say high cholesterol or obesity. These factors are risk factors for many diseases and condition. So they are not specific to 1. Even if there is factor isn't specific to one disease. Does it mean that there's less language about the relationship is causal? The five criteria field that's establishes causality are listed on the bottom of the screen using the same ones we saw in the previous slides that had a Red Star next to them. On the top are ways that we can actually measure them for temporality. Make sure exposure is before outcome or strength of association. Refer to your measure of association, whether it be a relative risk or an odds ratio or dose response. Refer to your dose response curves. You can break your exposure down to categories. Turtles. Quartiles. Wind towers just depends on what you want to do and the number of people you have in your study. Then you can run them as continuous variables and test whether the P value is less than 0.05. This is your P value for trend. You can also check to see if there is non monotonicity. Run a generalized additive model. For replication you can check what has been published in the past and complete a meta analysis. For secession you can remove the exposure. You can see this with a disappearance curve. When you look at causation, there are many interacting factors. When we investigate a question, it's generally an isolation because we can't examine everything in its totality. Outside of your causal agents, your exposure of interest, there's genetics, the environment, and other protective factors as well. It's a very complex relationship. In epidemiology, we examine population health. We're not examining research questions at the individual level. Because of that, we cannot say that population level causation is the same as individual level because everyone may have slightly di;erent causal pathways. Their genetics and everyone's environment is di;erent. Their risk factors are di;erent. Each of us represent amalgam of di;erent exposures. That make us more or less susceptible to di;erent diseases and conditions. Associations starting point for establishing causation. The cause of criteria are helpful but not a doctrine and reasoning disease causes prevention doesn't have to wait. If causation is not firmly established, it may never even be possible to completely prove causation in some complex diseases, but EPI can help identify some preventable risk.