Introduction to Data Science Introductory Statistics/Probability Part II 2024 FM217 PDF
Document Details
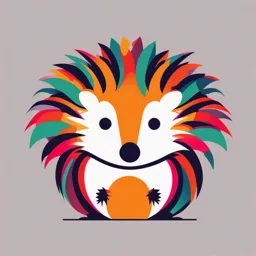
Uploaded by SecureConflict9348
2024
Tags
Related
- Probability & Statistics 2024-2025 PDF
- Statistical Methods of Data Science PDF
- Probability & Statistics for Engineers & Scientists PDF
- Statistics for Business Analytics & Data Science PDF
- Introductory Statistics for the Life and Biomedical Sciences PDF
- Statistics - Probability and Descriptive Statistics Course Book PDF
Summary
These are lecture notes on introduction to data science covering introductory statistics and probability, part II. The document discusses concepts like percentiles, quantiles, quartiles, and boxplots, as well as standardized variables, central moments, skewness, and kurtosis. It also covers covariance and correlation coefficient.
Full Transcript
Introduction to Data Science Introductory Statistics / Probability – Part II September 6, 2024 Percentiles Position of nth percentile = (Population Size) * n/100 Akin to dividing the sorted data into 100 buckets and picking the n th bucket. ...
Introduction to Data Science Introductory Statistics / Probability – Part II September 6, 2024 Percentiles Position of nth percentile = (Population Size) * n/100 Akin to dividing the sorted data into 100 buckets and picking the n th bucket. Quantiles A q-quantile splits the data into q equal parts. Akin to dividing the sorted data into q buckets and picking the nth bucket. Position of nth q-quantile = ? Quantiles A q-quantile splits the data into q equal parts. Akin to dividing the sorted data into q buckets and picking the nth bucket. Position of nth q-quantile = (Population Size) * n/q Quantiles - Example A q-quantile splits the data into q equal parts. X = {1,1,2,2,3,3,4,4,5,5} q= 5 : { 1,1, | 2,2 | 3,3 | 4,4 | 5,5 } q =2: { 1,1,2,2,3 | 3,4,4,5,5 } q=10 : { 1, | 1, | 2, | 2, | 3, | 3, | 4, | 4, | 5, | 5 } Quantiles Quantiles help get an idea of the spread of data at a required granularity. Quantiles Quantiles help get an idea of the spread of data at a required granularity. When q = 100, 100-quantiles = percentiles When q = 10, 10-quantiles = deciles When q = 4, 4-quantiles = quartiles : : Quantiles Quantiles help get an idea of the spread of data at a required granularity. When q = 100, 100-quantiles = percentiles When q = 10, 10-quantiles = deciles When q = 4, 4-quantiles = quartiles : : Q) What are the bounds on the values that q can take? Quartiles Quantiles with q = 4 Give a good idea about the centre as well as the spread of the data. X = [0,….Q1,….Q2,….Q3,….n ] Q1 = 1st quartile Q2 = 2nd quartile Q3 = 3rd quartile Quartiles Quantiles with q = 4 Give a good idea about the centre as well as the spread of the data. X = [0,….Q1,….Q2,….Q3,….n ] Q1 = 1st quartile = Lower quartile Q2 = 2nd quartile = Middle quartile Q3 = 3rd quartile = Upper quartile Quartiles Quantiles with q = 4 Give a good idea about the centre as well as the spread of the data. X = [0,….Q1,….Q2,….Q3,….n ] Q1 = 1st quartile = Lower quartile = 25th Percentile Q2 = 2nd quartile = Middle quartile = 50th Percentile Q3 = 3rd quartile = Upper quartile = 75th Percentile Quartiles Quantiles with q = 4 Give a good idea about the centre as well as the spread of the data. X = [0,….Q1,….Q2,….Q3,….n ] Q1 = 1st quartile = Lower quartile = 25th Percentile = Median of lower half of data Q2 = 2nd quartile = Middle quartile = 50th Percentile = Median Q3 = 3rd quartile = Upper quartile = 75th Percentile = Median of upper half of data Boxplots Boxplots Whiskers could end at: 1. Minimum and Maximum 2. 2nd Percentile and 98th Percentile 3. (Q1 - 1.5 IQR) and (Q3 + 1.5 IQR) 4. (Q1 - XYZ) and (Q3 + XYZ) 5. … Boxplots Boxplots Boxplots Box-and-whisker plots or Box plots display the centrality, dispersion and skew of data. Can also be used to highlight the outliers. Helpful in visually comparing (multiple aspects of) different datasets or distributions. Standardized Variable / Dataset Scaled version of the original variable/dataset such that Mean = 0 Standard Deviation = 1 Standardized Variable / Dataset Scaled version of the original variable/dataset such that Mean = 0 Standardized Variable / Dataset Scaled version of the original variable/dataset such that Mean = 0 Standard Deviation = 1 Standardized Variable / Dataset Scaled version of the original variable/dataset such that Mean = 0 Standard Deviation = 1 X = {10, 20, 30} After Standardization : {-SQRT(3), 0, SQRT(3)} Central Moments The ith Central Moment is the Expected value of the ith power of deviation from the mean. Central Moments The deviation from the mean. Central Moments The ith power of deviation from the mean. Central Moments The ith Central Moment is the Expected value of the ith power of deviation from the mean. Central Moments Standardized Central Moments Standardized Central Moments Third Standardized Central Moment Skewness Third Standardized Central Moment Skewness Right skewed or Left skewed or Positive skewed Negative skewed Fourth Standardized Central Moments Kurtosis Fourth Standardized Central Moments Kurtosis Covariance Cov(X,Y) = E[ ( X – E[X] ) ( Y – E[Y] ) ] = E[ XY – X E[Y] – Y E[X] + E[X] E[Y] ] = E[ XY ] – E[X] E[Y] – E[X] E[Y] + E[X] E[Y] = E[ XY ] – E[X] E[Y] Cov(X,Y) < 0 Cov(X,Y) = 0 Cov(X,Y) > 0 Correlation Coefficient The Correlation Coefficient is generally a scale independent version of the covariance. Hence, it is a better indicator of the correlation of the two variables or datasets. Pearson's Correlation Coefficient: ρX,Y = cov(X,Y) / (σX σY) ρX,Y = - 0.8 ρX,Y = 0 ρX,Y = 0.8